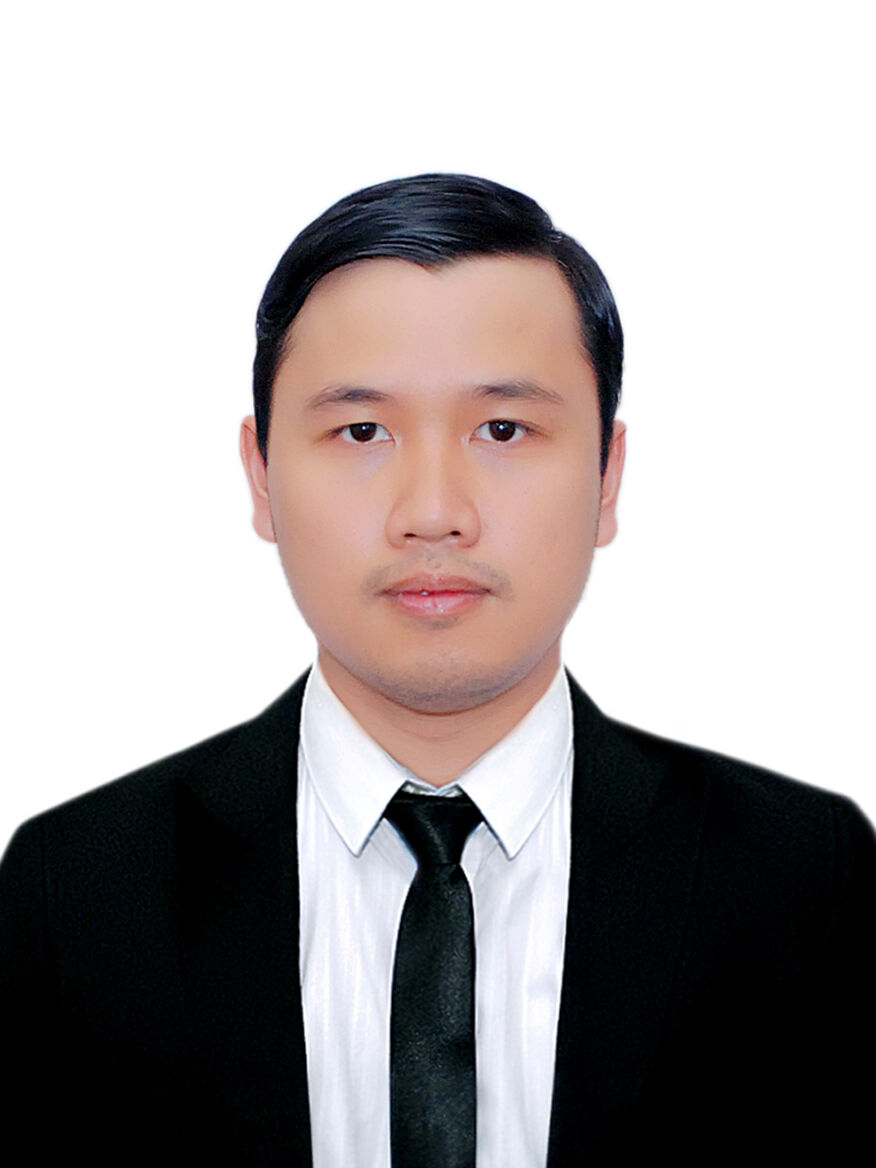
IMPROVING THE PERFORMANCE OF WI-FI INDOOR LOCALIZATION IN BOTH DENSE AND UNKNOWN ENVIRONMENTS
Indoor localization is important for various pervasive applications, garnering considerable research attention over recent decades. Despite numerous proposed solutions, the practical application of these methods in real-world environments with high applicability remains challenging. One compelling use case for building owners is the ability to track individuals as they navigate through the building, whether for security, customer analytics, space utilization planning, or other management purposes. However, this task becomes exceedingly difficult in environments with hundreds or thousands of people in motion. Conversely, the need to track oneself’s location is also meaningful from the perspective of individuals traversing in crowded spaces. These use cases are pertinent, such as meeting friends or reaching a preferred store in random malls or shopping centers. Nonetheless, addressing these use cases requires solutions that can be applied in unknown environments without pre-existing knowledge of those environments. Consequently, solutions should not necessitate the installation of complex devices, require extensive maintenance efforts, or rely on detailed environmental knowledge.
This thesis will address two particularly challenging environments: dense environments with thousands of moving people in non-overlapping areas and unknown environments with no maps, fingerprints, or pre-existing knowledge. The proposed system for dense environment, named DenseTrack, connects devices reported by a Wi-Fi location system with specific video blobs obtained through computationally efficient video data analysis. The experiment results indicate that DenseTrack acquires an average match accuracy of 83% within a 2-person distance, with an average latency of 48 seconds in dense environments. For unknown environments, the thesis presents empirical findings derived from experiments utilizing one-sided RTT, aiming to assess the feasibility of this approach for indoor localization. By addressing the challenges posed by both dense and unknown environments, a comprehensive solution for indoor localization in practical scenarios is achieved.
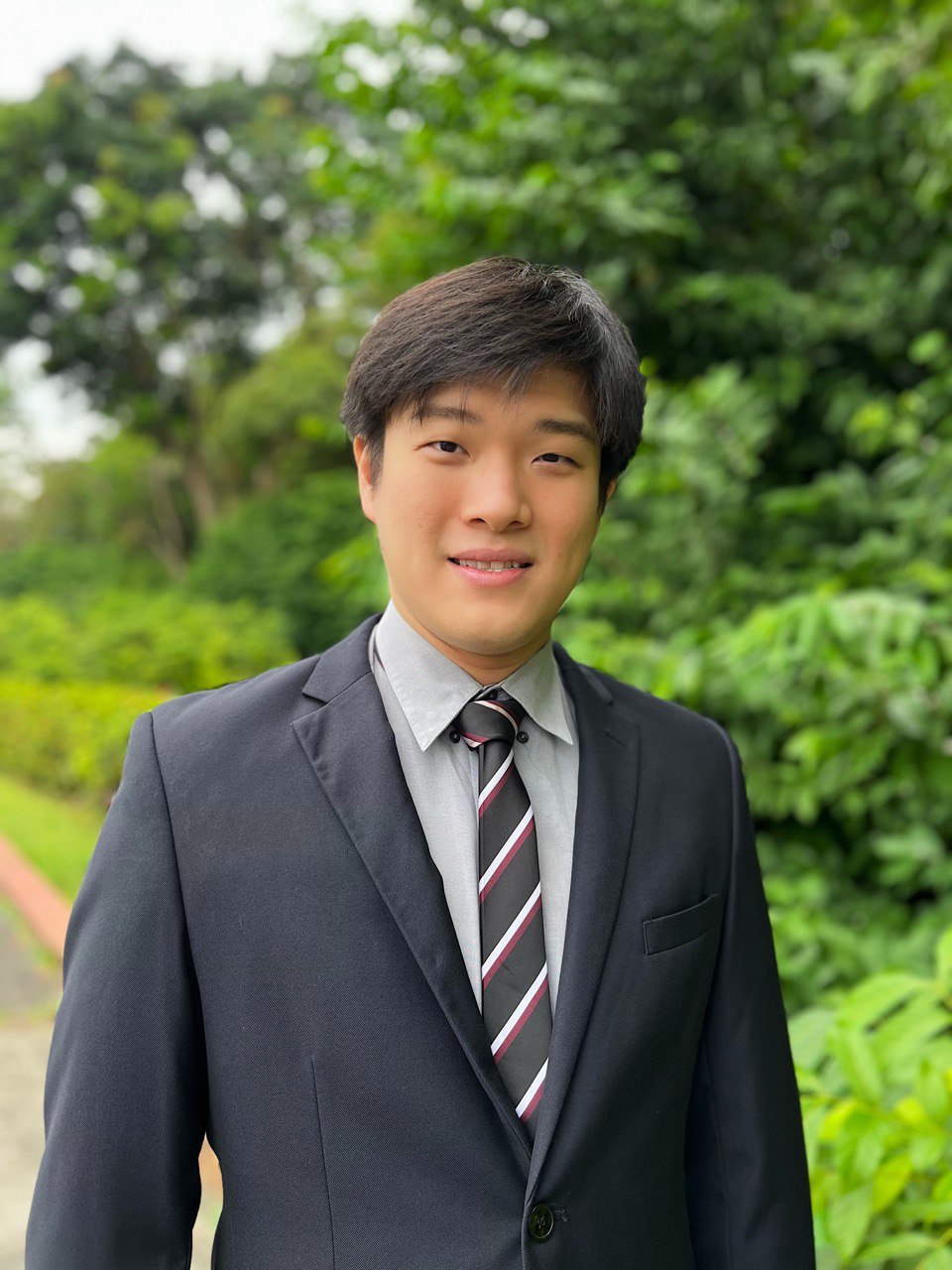
Essays on Weak Identification
This dissertation consists of two chapters that deal with the estimation and inference of weak instrumental variable (IV) models. The first chapter considers a linear combination of jackknife Anderson-Rubin (AR), jackknife Lagrangian multiplier (LM), and orthogonalized jackknife LM tests for inference in IV regressions with many weak instruments and heteroskedasticity. Following I.Andrews (2016), weights are chosen in a linear fashion based on a decision-theoretic rule that is adaptive to the identification strength. Under both weak and strong identifications, the proposed test controls asymptotic size and is admissible among certain classes of tests. Under strong identification, the linear combination test has optimal power against local alternatives among the class of invariant or unbiased tests which are constructed based on jackknife AR and LM Tests. Simulations and an empirical application confirm the good power properties of the test. The second chapter deals with the AR Tests developed separately to conduct weak-identification-robust inference when the number of IV is fixed or diverging to infinity with the sample size, respectively. These two tests compare distinct test statistics with distinct critical values. To implement them, researchers first need to take a stance on the asymptotic behavior of the number of IVs, which is ambiguous when this number is just moderate. Instead, in this chapter, I propose two analytical and two bootstrap-based weak-identification-robust AR Tests, all of which control asymptotic size whether the number of IVs is fixed or diverging - in particular, I allow but do not require the number of instruments to be greater than the sample size. Monte Carlo studies and empirical applications are conducted to illustrate the performance of the proposed algorithms and estimators.
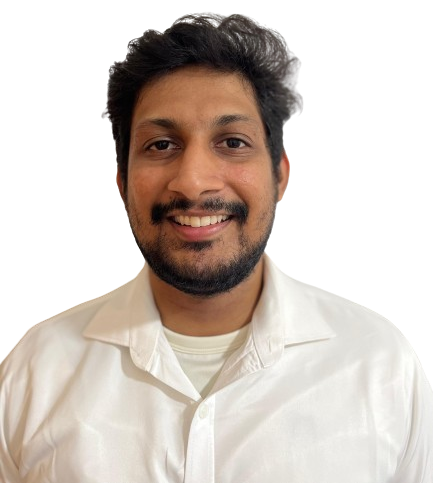
Natural human-human interaction is inherently multi-modal, as we use a variety of modalities including verbal commands, gestures and facial expressions, visual cues, gaze and even vocal nuances (e.g., tone and rhythm) to mutually convey our intent. Motivated by such human-human interaction scenarios, this thesis broadly investigates some methods to enable multi-modal sense-making for human-AI interaction tasks in resource-constrained wearable and edge devices. In particular, we consider object acquisition as an exemplary task for human-AI collaboration that can benefit from enabling naturalistic multi-modal interaction. To address this, we leverage Referring Expression Comprehension (REC) or Visual Grounding models developed in computer vision and NLP literature. These models, when provided with an image along with verbal and/or gestural inputs, identify the bounding box of the referred object. We then introduce a number of sense-making models and optimization techniques to support low-latency execution of such models for inferencing on pervasive devices.
In this thesis, our emphasis will be predominantly on exploring diverse dynamic optimizations for the comprehension of task instructions. Throughout these investigations, we rely on a common guiding principle that underscores our approach: the acknowledgement that not all instructions pose the same level of task complexity. To illustrate, consider the varying complexities introduced by different types of instructions. In a cluttered environment, identifying a target object often necessitates a more intricate execution pipeline to ensure accurate identification. Users may employ a combination of language instructions and pointing gestures, which can aid the model in disambiguating among closely situated objects. Consequently, the presence of multiple modalities helps alleviate task complexity. Conversely, in a less cluttered space, a simple pointing gesture may suffice for object identification, requiring a less complex execution pipeline. This nuanced understanding of task complexities serves as the foundation for the dynamic optimizations explored in this thesis.
This dissertation is organized into two parts. Part 1 focuses on studying model optimizations applied to REC models, which process a single static image along with language and, optionally, gestural modalities. In Part 2, we extend our methodologies to more complex scenarios involving videos as vision input, moving beyond single static images.
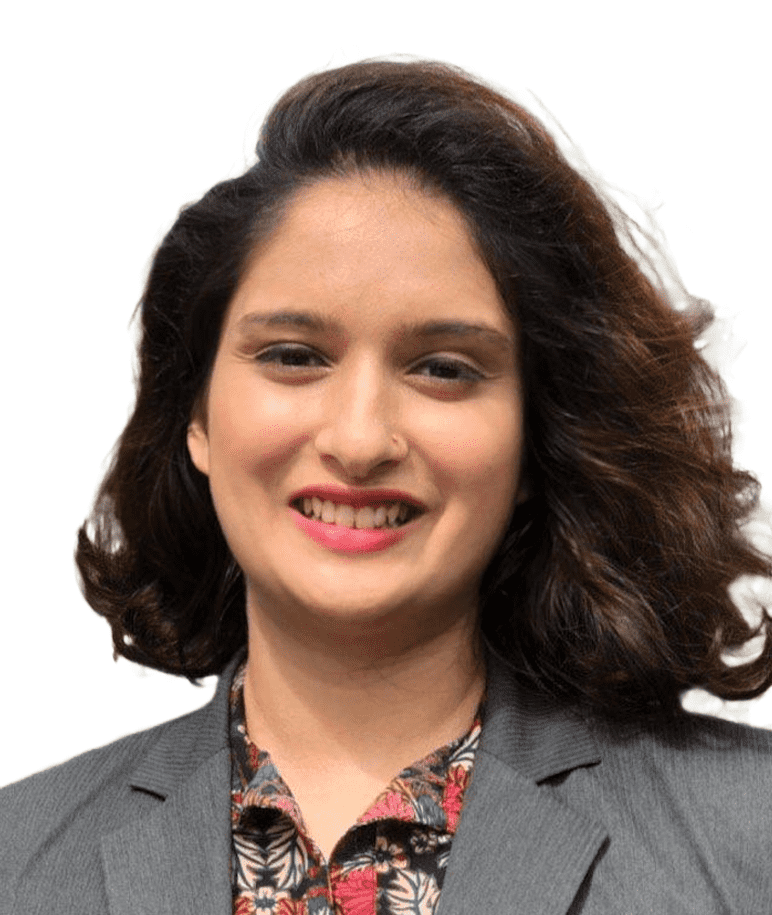
Cyber-physical systems and applications have fundamentally changed people and processes in the way they interact with the physical world, ushering in the fourth industrial revolution. Supported by a variety of sensors, hardware platforms, artificial intelligence and machine learning models, and systems frameworks, CPS applications aim to automate and ease the burden of repetitive, laborious, or unsafe tasks borne by humans. Machine visual perception, encompassing tasks such as object detection, object tracking and activity analysis, is a key technical enabler of such CPS applications. Efficient execution of such machine vision perception tasks on resource-constrained edge devices, especially in terms of ensuring both high fidelity and processing throughput, remains a formidable challenge. This is due to the continuing increase in resolution of sensor streams (e.g., video input streams generated by 4K/8K cameras and high-volume event streams generated by emerging neuromorphic event cameras) and the computational complexity of the Deep Neural Network (DNN) models that underpin such perception capabilities, which overwhelms edge platforms, adversely impacting machine perception efficiency. This challenge is even more severe when a perception pipeline operating on a single edge device must process multiple concurrent video streams for accurate sense-making of the physical world. Given the insufficiency of the available computation resources, a question then arises on whether parts of the perception task can be prioritized (and executed preferentially) to achieve highest task fidelity while adhering to the resource budget. This thesis introduces the paradigm of Canvas-based Processing and Criticality Awareness to tackle the challenge of multi-sensor machine perception pipelines on resource-constrained platforms. The proposed paradigm guides perception pipelines and systems on "what" to pay attention to in the sensing field and "when", across multiple camera streams, to significantly increase both perception fidelity under computational constraints and achievable system throughput on a single edge device. By creating spatial and temporal degrees of freedom for stimuli/regions of interest from their original video streams, such a perception pipeline can "pick and choose” which stimuli to ascribe more priority for preferential DNN inference over time, thereby reducing the total computational load. The thesis explores how such prioritized and selective processing, across multiple RGB and event sensor streams, needs to be designed to support both non-streaming and streaming perception tasks. With multiple strategies for fine-tuning such a perception pipeline for real-world deployment characteristics such as bandwidth constrained wireless networks, variable workloads at the edge, spatial overlap between cameras, this thesis demonstrates that it is possible to achieve multiplicative gains in processing throughput with no cost to DNN task accuracy, across multiple concurrent RGB and event camera streams at the resource-constrained edge. The proposed techniques are especially applicable for real-time multi-sensor machine perception tasks such as drone-based surveillance and multi-camera traffic analysis.
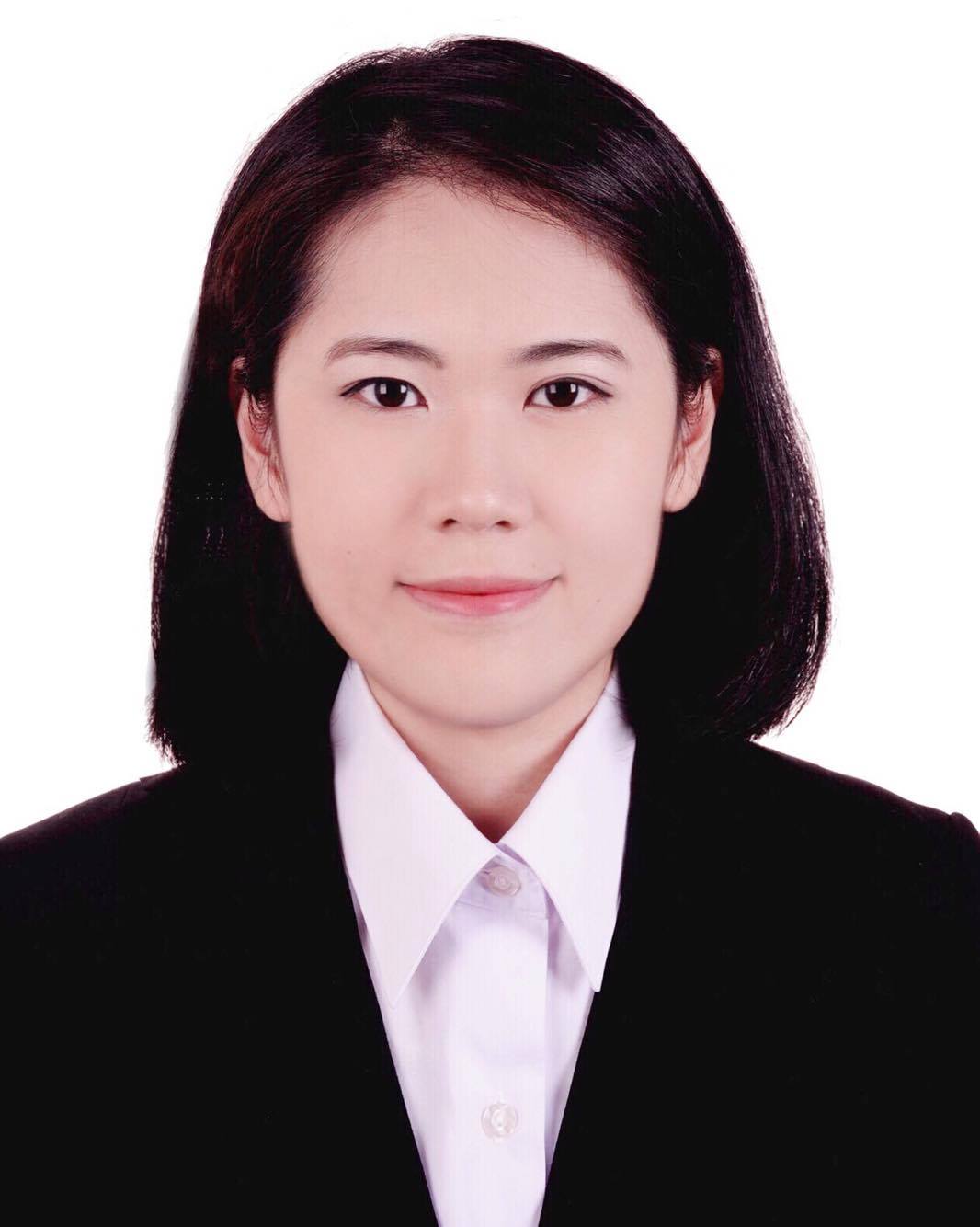
This study examines whether mandatory ESG disclosure encourages publicly traded firms to go private due to an increase in proprietary costs. I find that firms affected by the European Union’s Non-Financial Reporting Directive 2014/95/EU are more likely to go private after the passage of the directive. The main results are more pronounced for firms with fewer peers who voluntarily disclose ESG information, operating in industries with a higher rate of new entrants, having higher R&D expenditures, and exhibiting lower dependence on external financing. In summary, my findings suggest that mandatory ESG disclosure can encourage the decision to go private due to concerns about proprietary costs for public firms.
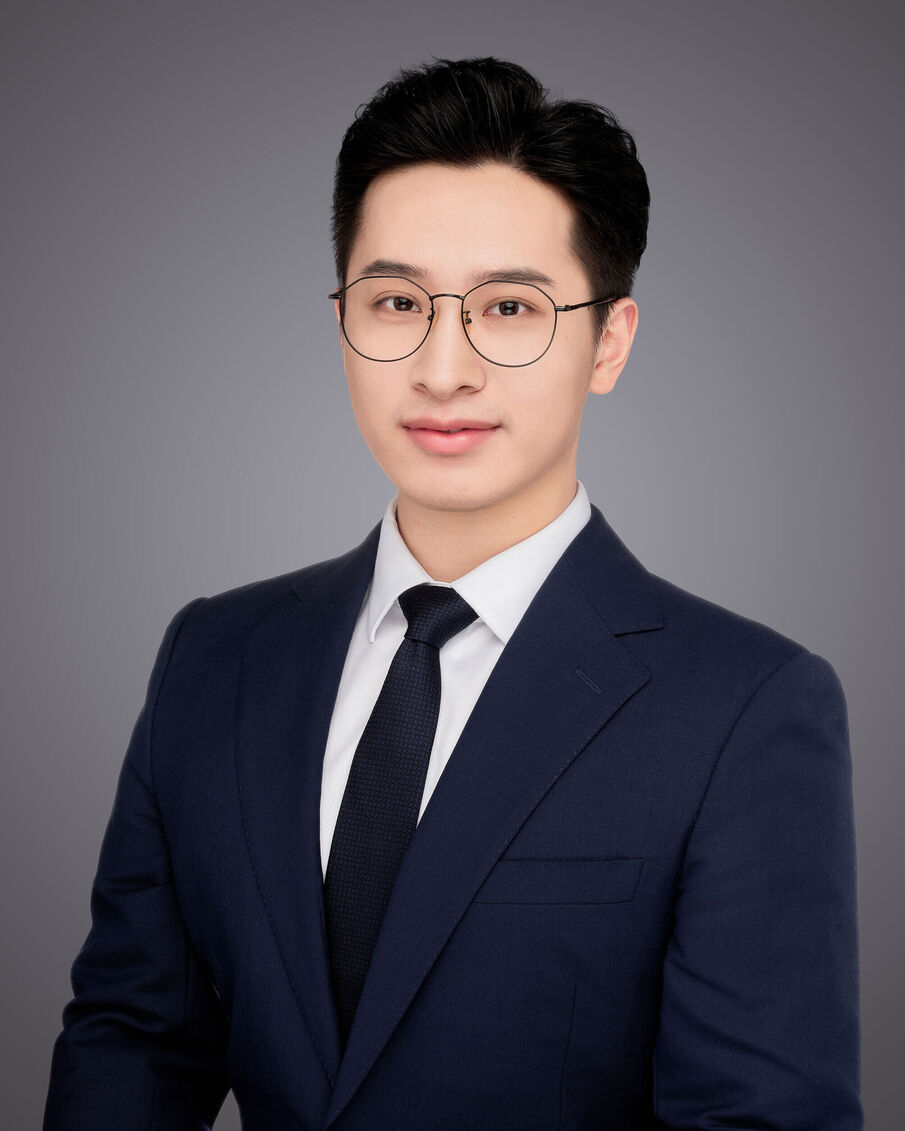
Essays on High-Frequency Financial Econometrics
My dissertation consists of three papers that contribute to the estimation and inference theory of high-frequency financial data.
In the second chapter, we present a general framework for optimal nonparametric spot volatility estimation based on intraday range data, comprised of the first, highest, lowest, and last price over a given time-interval. We rely on a decision-theoretic approach together with a coupling-type argument to directly tailor the form of the nonparametric estimator to the specific volatility measure of interest and relevant loss function. The resulting new optimal estimators offer substantial efficiency gains compared to existing commonly used range-based procedures.
The third chapter, extends the previous one by addressing the multiple candlesticks case. Specifically, we propose a computationally more efficient and accurate algorithm for optimal spot volatility estimation when dealing with more than one candlestick. In addition, we introduce an exact simulation scheme that overcomes the one-sided bias issue inherent in Euler discretization schemes, particularly when handling supremum and infimum. This exact simulation scheme not only allows for more precise risk comparison of estimators, but also facilitates further analysis involving extreme values of Brownian motions.
In the fourth chapter, we address the uniform inference problem for high-frequency data that includes prices, volumes, and trading flows. Such data is modeled with a general state-space framework, where latent state process is the corresponding risk indicators, e.g., volatility, price jump, average order size, and arrival of events. The functional estimators are formed as the collection of localized estimates across different time points. Although the proposed estimators do not admit a functional central limit theorem, a Gaussian strong approximation, or coupling, is established under in-fill asymptotics to facilitate feasible inference. We apply the proposed methodology to distinguish the informative part from the Federal Open Market Committee speeches, and to analyze the impact of social media activities on cryptocurrency markets.
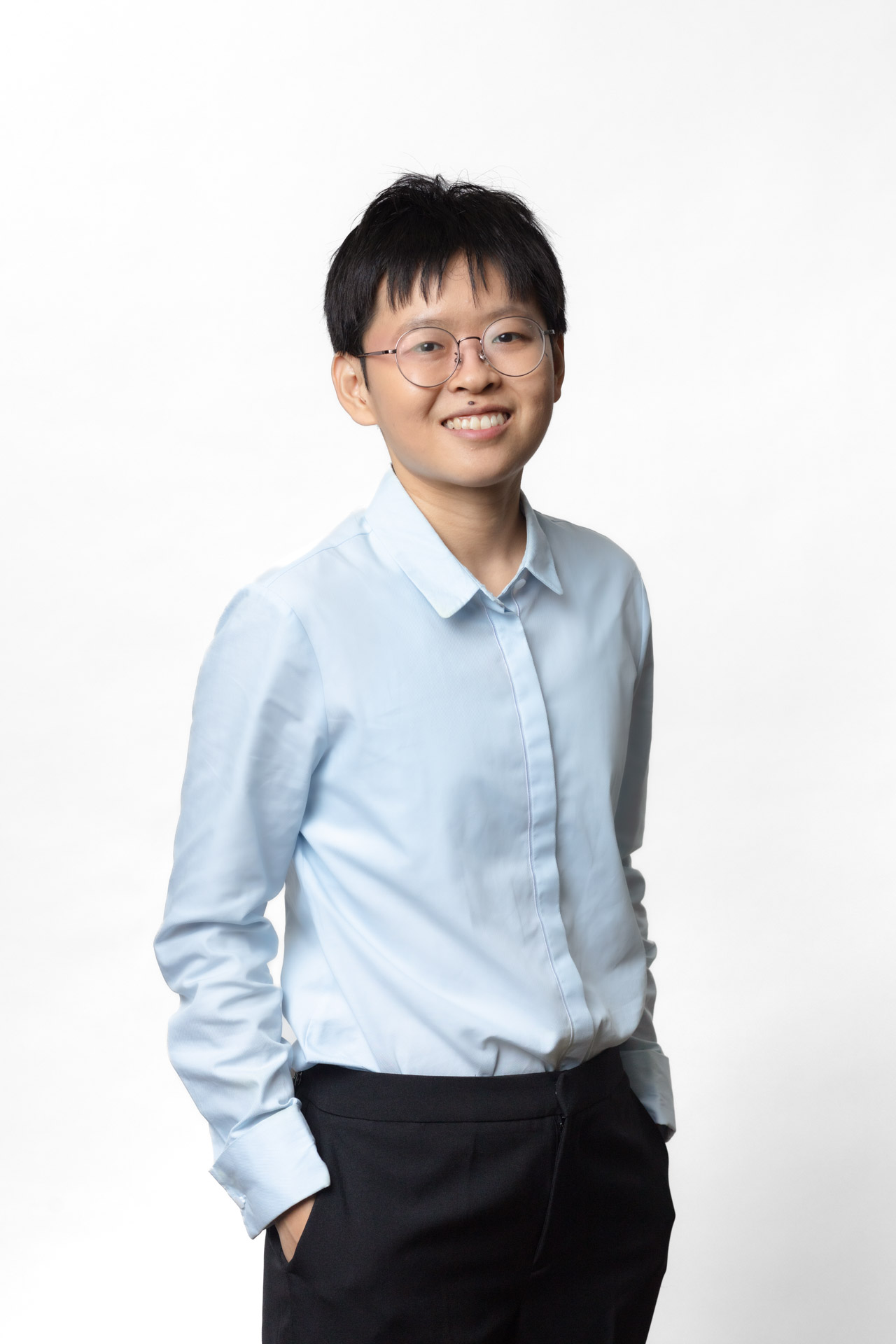
I examine how the hierarchical structure of a firm’s accounting function influences its financial reporting quality. Using information from accounting employees’ online resumes to infer the hierarchical layers in a firm’s accounting function, I find that a firm with a more hierarchical accounting function exhibits higher financial reporting quality. Further analysis shows a hierarchical accounting function is associated with a reduced likelihood of internal control weaknesses, in particular internal control weaknesses in the segregation of duties and accounting personnel matters. These findings suggest that a hierarchical accounting function enhances financial reporting quality through improving internal control. These effects are more pronounced when a firm’s accountants are of a lower level of education and when a firm’s accounting function spans a smaller geographical region. Collectively, my findings underscore the importance of the organizational structure of a firm’s accounting function in shaping its financial reporting quality.
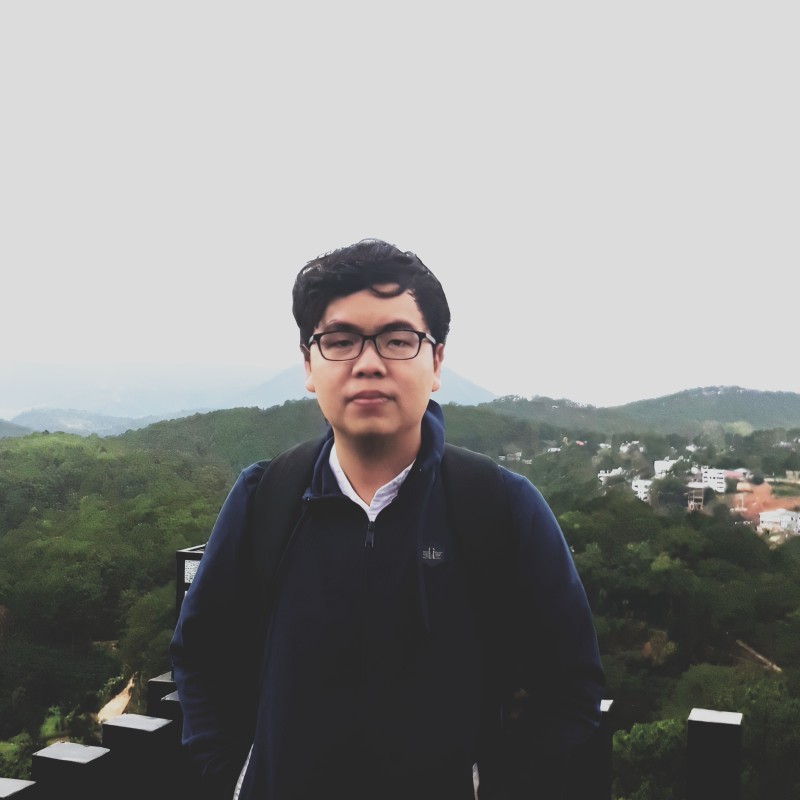
Towards Securing Smart Contracts Systematically
Smart contracts are a groundbreaking technique that allows users to programmatically modify the state of the blockchain. They are essentially self-enforcing programs that are deployed and executed on top of the blockchain. In recent years, we have witnessed various smart contract incidents that led to substantial financial losses and even business closures. These incidents mainly arise from design flaws in Solidity, a dominant programming language for writing smart contracts, which complicates the process of detecting and repairing vulnerabilities. Furthermore, there is a growing interest in attacking smart contracts by the attackers. This thesis is dedicated to developing effective methods to ensure the safety and correctness of smart contracts systematically. Our methods have two parts: vulnerability detection and smart contract repair. While the goal of vulnerability detection is to aggressively uncover bugs, smart contract repair eliminates detected bugs by adding safety constraints.
In the first part of the thesis, we primarily concentrate on vulnerability detection. We start by building a grey-box fuzzing engine for detecting common vulnerabilities like reentrancy and arithmetic vulnerabilities. The main contribution is an algorithm, inspired by search-based software testing (SBST), to improve the quality of the test suite. Subsequently, we design a formal verification framework to guarantee the correctness of smart contracts. The framework provides an expressive verification language and a functional verification engine that aims to eliminate global analysis and reduce false positives.
In the second part of the thesis, we propose repair algorithms to systematically eliminate detected vulnerabilities in smart contracts. We first design a novel approach to patch vulnerable implementations by analyzing control and data dependencies in their bytecode. Each vulnerability is defined in the form of dependencies and is patched using the corresponding templates. The patched contracts are proven to be free of vulnerabilities and incur low gas overhead. After that, we develop an algorithm to repair bugs in user-developed specifications in the form of a precondition/post-condition for each function. The algorithm is inspired by abductive inference and constraint-solving. It first automatically discovers inconsistencies between the specification and the implementation and then generates recommendations for repairing specifications. With vulnerability detection and contract repair, this thesis paves the way for achieving smart contract security systematically.
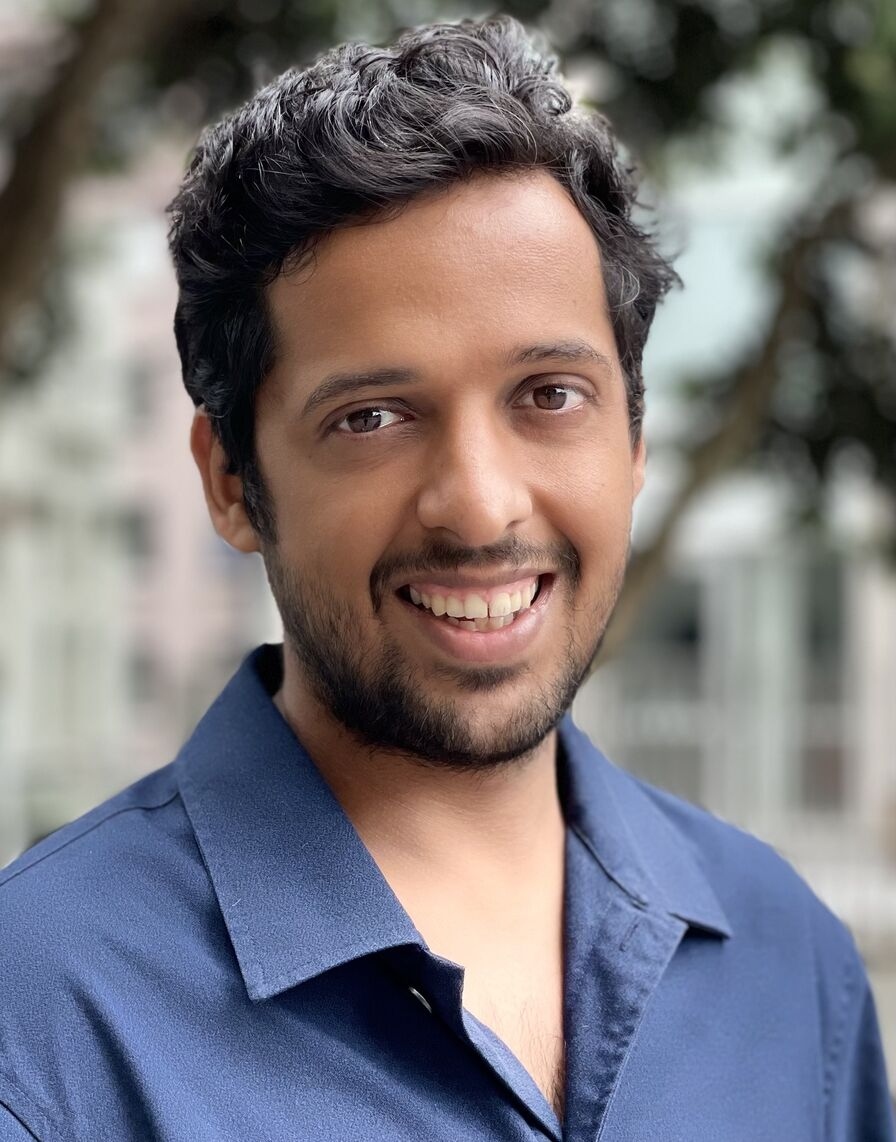
In modern collaborative work environments, interactions with colleagues span a spectrum from close relationships to those perceived as socially distant. While close relationships are traditionally emphasized for their benefits on well-being, distant ties often go overlooked despite their prevalence and ease of maintenance. Building on construal-level theory, I first propose a theory of relational construing, emphasizing how the perceived psychological distance with colleagues influences mental representations and shifts in these representations, termed mindful construing. Leveraging the full spectrum of workplace relationships, from close to distant, can enhance well-being by alleviating the burdens of maintaining solely close connections. I then use empirical studies to test the influence of social distance on construal level and subsequent effects on eudaimonic and hedonic well-being and a deep and broad sense of belonging. Using multilevel analysis in two datasets collected using experience sampling and daily reconstruction methods, respectively, I find some support for my hypotheses.
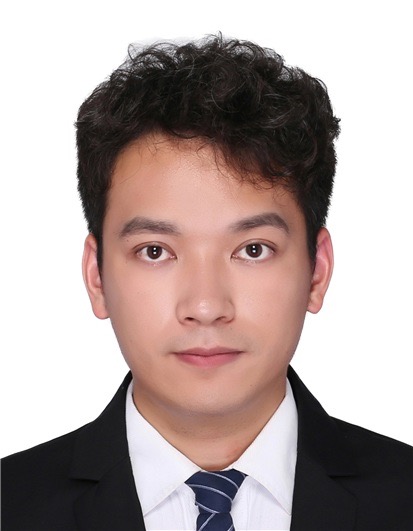
The recommender system is a crucial component of today's online services. It helps users navigate through an overwhelmingly large number of items and discovering those that interest them. Unlike general recommender systems, which recommend items based on the user's overall preferences, sequential recommender systems consider the order of user-item interactions. Sequential recommendations aim to predict the next item a user will interact with, given a sequence of previously interacted items, while considering the short-term and long-term dependencies among items.
In this thesis, we focus on sequential recommendation methods: from representation learning to large language model (LLM)-based reasoning. On the one hand, representation learning-based sequential recommendation methods usually feed ID embeddings of interacted items into models, such as deep neural networks, to generate user representation vectors. They then rank candidate items to create a recommendation list based on the similarity between user representation vectors and candidate item vectors. On the other hand, the LLM-based reasoning approach mainly depend on the LLM's strong reasoning ability and rich world knowledge. When using LLM-based reasoners, it requires carefully designed prompts and/or demonstration examples considering the task complexity and prompt length constraint.
This thesis consists of three parts. In the first part, we aim to improve representation learning for sequential recommendation and present our efforts in building an explanation-guided contrastive learning sequential recommendation model. In the second part, we investigate how we can build sequential recommendation models based on the recent success of LLMs. In particular, we introduce two new research directions for LLM-based sequential recommendation: 1) zero-shot LLM-based reasoning of recommended items and 2) few-shot LLM-based reasoning of recommended items. In the final part, we address the explanation generation task and the evaluation of explanation for sequential recommendation results using LLMs. Specifically, we introduce a framework for LLM-based explanation that can support an automatic evaluation of an LLM's ability to generate plausible post-hoc explanations from the content filtering and collaborative filtering perspectives.
Essays on empirical asset pricing
The dissertation consists of three chapters on empirical asset pricing. The first chapter examines the market return predictability of media coverage on climate change. We introduce a comprehensive media climate change concern (ΔCMCCC) index, derived from unexpected climate change coverage across diverse media channels, including print (newspapers), voice (radio), and video (television). Our findings show that this index negatively predicts stock market returns, both in-sample, and out-of-sample, offering potential gains for investors. The second chapter conducts an in-depth analysis of how trading volume can either alleviate or exacerbate stock mispricing. Our research findings highlight that the impact of trading volume on mispricing is contingent upon the predominant source of mispricing, whether it stems from limited attention or investor biases and the volume state (high or low). The third chapter proposes a novel psychological explanation that anchoring on the psychological barrier of 52-week extreme price, to elucidate the observed heterogeneity in the risk-return trade-off. Specifically, we find a negative risk-return relation among stocks with prices far from their 52-week high prices and a positive risk-return relation among stocks with prices near their 52-week high prices. In summary, the dissertation contributes to the field of empirical asset pricing through its rigorous analyses and innovative insights.
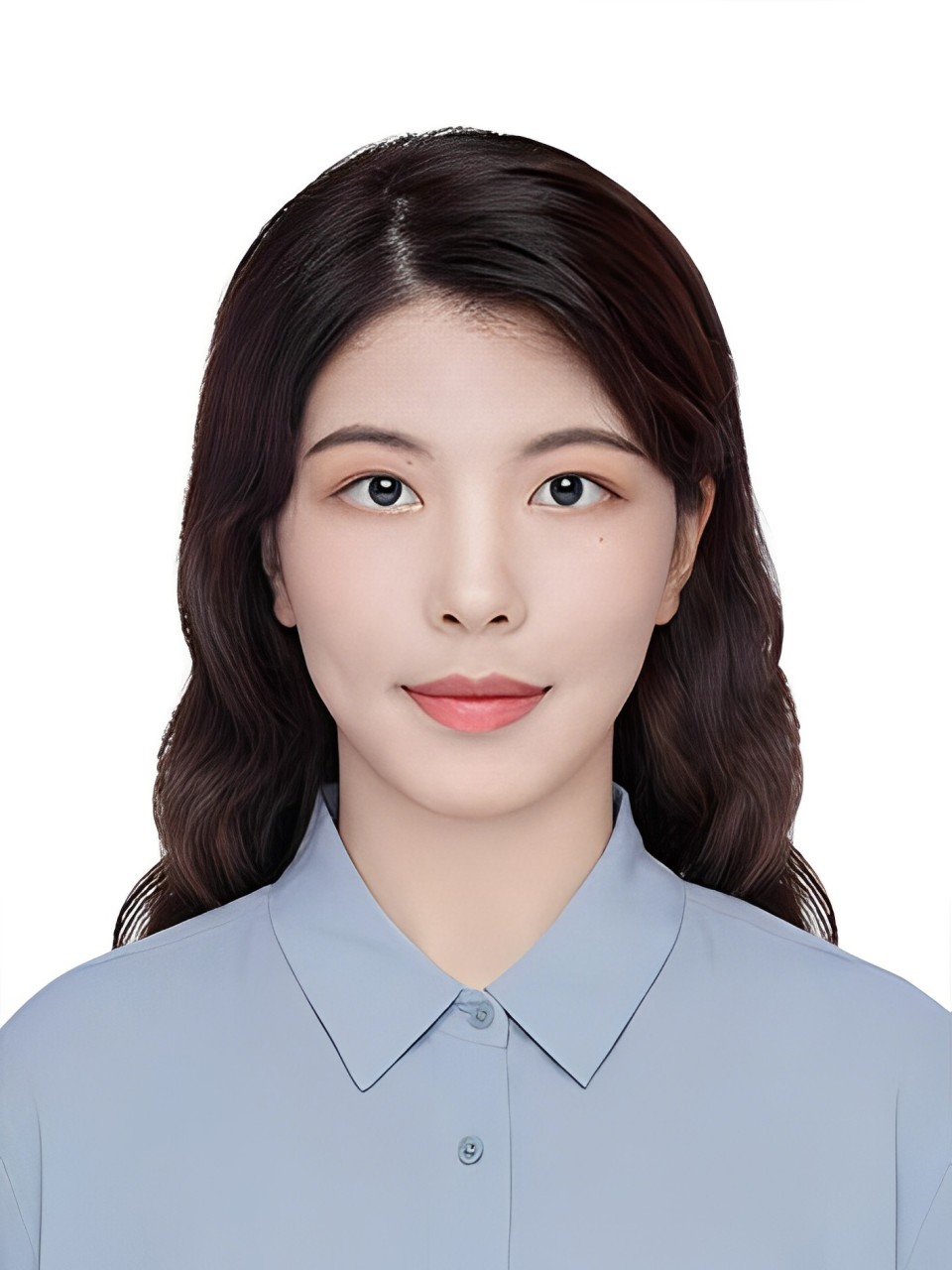
I examine whether and how firms incorporate retail customers’ environmental preferences into their pollution decisions. Leveraging the staggered revelations of firms’ environmental negative news and the granularity of household grocery shopping records, I quantify local customers’ heterogeneous environmental preferences based on the extent of product sales declines following the news events. In line with the conjecture that firms factor in rewards and penalties from customers and strategically reduce their pollution, I find a significant improvement in air quality near event firms’ facilities located in markets where local customers reveal the strongest environmental preferences. This effect is more pronounced when news events are more salient and when ex-ante information frictions between firms and customers are greater. Furthermore, I find no changes in firm-level pollution, and air quality significantly worsens in facilities located in markets where customers have weaker environmental preferences, corroborating firms’ pollution-shifting strategy. Overall, my findings shed light on retail customers’ role in firms’ environmental resource allocation.
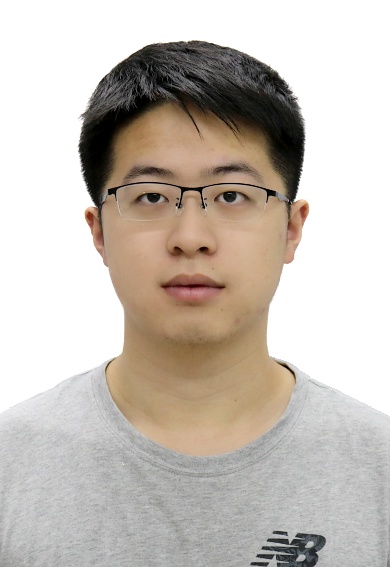
Mandatory ESG disclosure makes it possible to incorporate ESG information into stock prices, incentivizing firms to “do good”. This channel, however, may lead to suboptimal investments, according to disclosure theories. This study investigates the changes in firms’ investment in innovation activities following the staggered introduction of mandatory ESG disclosure around the world. Using a sample of corporate patents filed by listed firms across 58 countries from 2000 to 2022, I find that the introduction of mandatory ESG disclosure is associated with less corporate innovation. The effect is mainly driven by countries that mandate ESG disclosure within corporate financial reports, when the market force channel is more likely to work (i.e., when ESG information is more likely to be incorporated into stock prices). To shed light on the underlying mechanism, I document a less sensitive market response to financial information, measured by a reduction in earnings response coefficients (ERCs) and the main effect is mainly driven by countries with a greater reduction in ERCs. In addition, the main effect is more pronounced in countries with stronger environmental preferences. The main effect is partially mitigated in countries with stronger external financing. Collectively, this paper suggests that mandatory ESG disclosure leads to an unintended cost for corporate innovation.
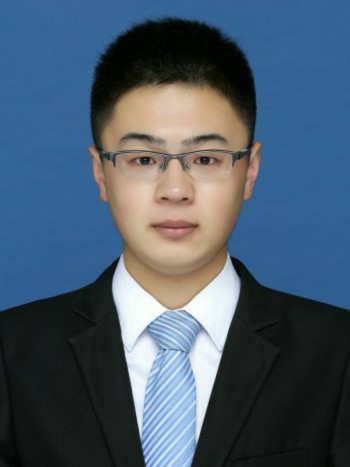
Recommender system design and multi-channel pricing: Personalization strategies for online platforms
The advancement of mobile technology and rising consumer demand have contributed to the unprecedented growth of online platforms. Entertainment platforms host a vast amount of user-generated content (UGC). The unique feature of UGC entertainment platforms is that creators’ content generation and users’ content usage can influence each other. However, traditional recommender systems often emphasize content usage but ignore content generation, leading to a misalignment between these two goals. To address this challenge, we propose a new framework to balance content generation and usage through personalized content recommendation and display decisions. In addition, an increasing number of e-commerce platforms introduce multiple sales channels, which facilitate consumers to search products across multiple channels and leave their footprints. Optimizing multiple-channel prices based on consumers’ footprints is vital and challenging for these platforms. Thus, we design an innovative pricing method based on consumers’ multi-channel footprints. In conclusion, this thesis designs novel multistakeholder recommendation and multi-channel pricing strategies for online platforms.
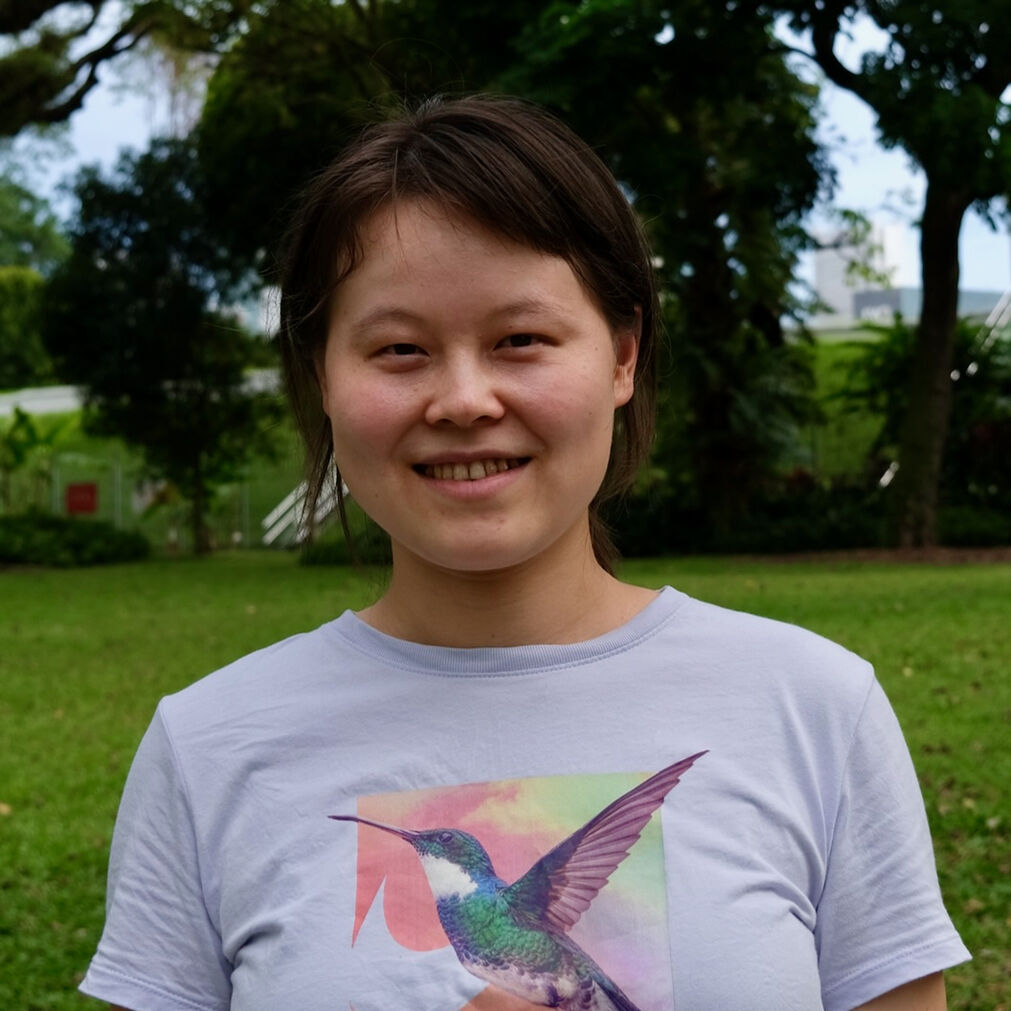
In recent years, software engineering (SE) has witnessed significant growth, leading to the creation and sharing of an abundance of software artifacts such as source code, bug reports, and pull requests. Analyzing these artifacts is crucial for comprehending the sentiments of software developers and automating various SE tasks, ultimately leading to more human-centered automated SE and enhancing software development efficiency. However, the diverse and unstructured nature of software text poses a significant challenge to this analysis. In response, researchers have investigated a variety of approaches, including the utilization of natural language processing techniques. The advent of large language models (LLMs), ranging from smaller-size LLMs (sLLMs) like BERT to bigger ones (bLLMs) such as LLaMA, has ignited a growing interest in their potential for analyzing software-related text.
This dissertation explores how LLMs can automate different SE tasks involving classification, ranking, and generation tasks. In the first study, we assess the efficacy of sLLMs, such as BERT, in SE sentiment analysis, comparing them to existing SE-specific tools. Furthermore, we compare the performance of bLLMs with sLLMs in this context. In the second study, we address the issue of retrieving duplicate bug reports. First, we create a benchmark and then use bLLMs to enhance the accuracy of this process, with a specific focus on employing GPT-3.5 for suggesting duplicate bug reports. In the third study, we propose to leverage sLLMs to create precise and concise pull request titles.
In conclusion, this dissertation contributes to the SE field by exploring the potential of LLMs to support software developers in understanding sentiments and improving the efficiency of software development.
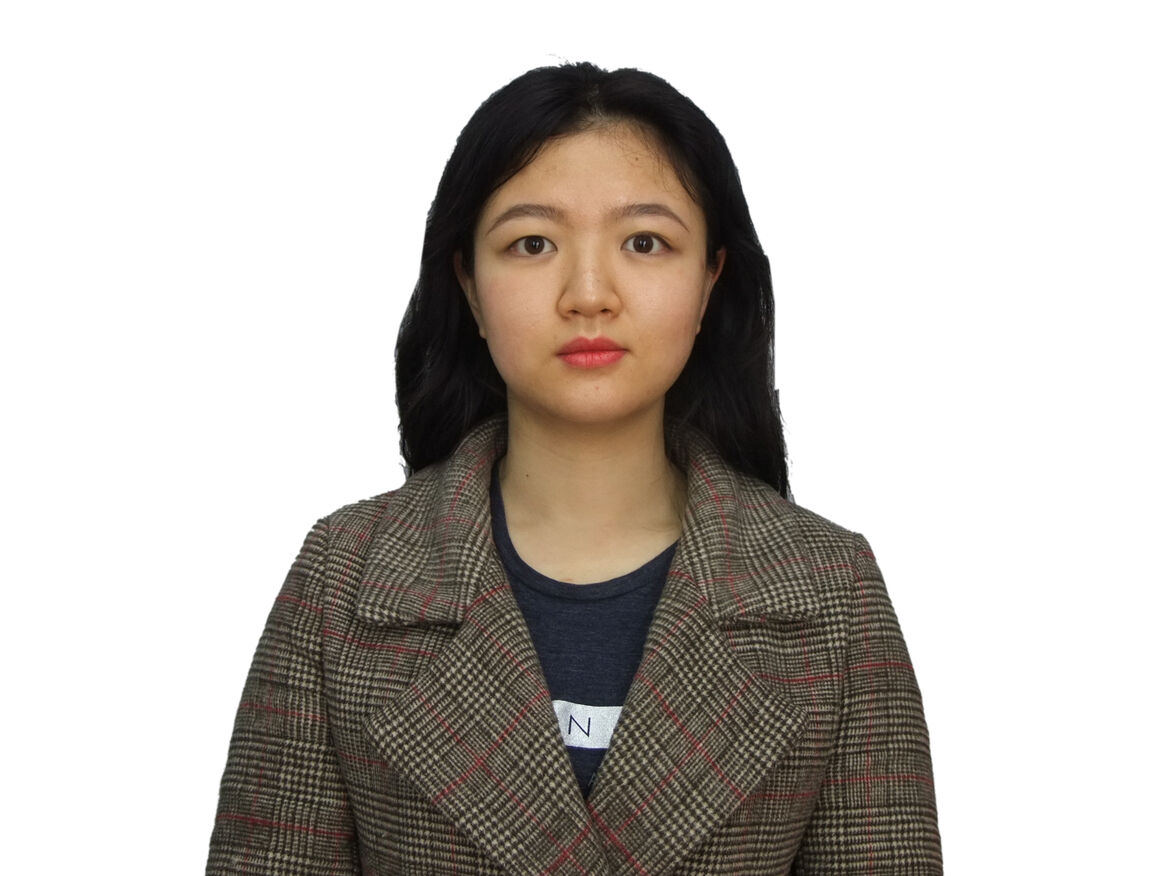
In recent years, remarkable progress has been made in Artificial Intelligence (AI),
with an increasing focus on integrating AI systems into people’s daily lives. In the
context of our diverse world, research attention has shifted towards applying AI to
multimodal understanding tasks. This thesis specifically addresses two key modalities, namely, vision and language, and explores Vision-Language Understanding
(VLU).
In the past, addressing VLU tasks involved training distinct models from scratch
using task-specific data. However, limited by the amount of training data, models
may easily overfit the training data and fail to generalize. A recent breakthrough
is the development of Pre-trained Models (PTMs), which are trained on extensive
datasets to acquire universal representations. Leveraging these PTMs for VLU tasks
has become a prevalent approach.
The use of PTMs for VLU tasks can be divided into two paradigms: (1) finetuning PTMs with downstream task data, and (2) zero-shot transfer or few-shot learning based on frozen PTMs. However, existing methods under these two paradigms
suffer from a few limitations: direct fine-tuning of PTMs may overlook the unique
characteristics of the downstream tasks; the zero-shot and few-shot performance of
PTMs on some tasks may be poor; and complex VLU tasks may require multiple
reasoning skills that a single PTM may not possess.
In the thesis, we aim to address the limitations above by optimizing the utilization
of PTMs for VLU tasks. Our work can be organized based on whether we leverage
fine-tuning or zero-shot / few-shot learning, and whether we adopt a single PTM or a
composition of PTMs. When tuning a single PTM, we explore how to incorporate
task-specific components to better cater to downstream tasks (Tuning-Single). For
VLU tasks where frozen PTMs are not ideal solutions due to poor performance, we
investigate using a single frozen PTM to facilitate sub-steps in these tasks (Frozen-Single). On the other hand, we also study how to compose a set of tuned PTMs,
each capable of a reasoning skill, to improve the performance on these tasks in the
low-resource setting (Tuning-Composition). As VLU tasks may involve multiple
skills and multiple reasoning steps, we consider a composition of frozen PTMs
and assign reasoning tasks to proper frozen PTMs without requiring any adaptation
(Frozen-Composition).
Specifically, in this thesis, we narrow down our scope to two VLU tasks, Hateful
Meme Detection (HMD) and Visual Question Answering (VQA). HMD classifies a
given multimodal meme as either hateful or not hateful, while VQA aims to answer
questions related to a given image. The decision to focus on these two tasks stems
from their importance in real-world applications. Furthermore, both tasks present
non-trivial challenges that demand innovative solution approaches.
For the HMD task, most existing work has primarily focused on direct fine-tuning
of PTMs, treating HMD as a general multimodal classification task and overlooking
its unique characteristics. We address the limitation by integrating task-specific
components with PTMs and tuning them end-to-end. We proposed DisMultiHate,
which is based on a PTM but learns to disentangle representations of hate speech related target entities in memes to enhance hateful content classification. Additionally,
HMD often requires external background knowledge for meme comprehension, yet
there are no dedicated knowledge bases constructed for this purpose. In light of this,
we explore leveraging knowledge in Pre-trained Language Models (PT-LMs). We
propose PromptHate, which prompts PT-LMs and utilizes their implicit knowledge
for HMD. Since PT-LMs are inherently textual, PromptHate involves converting
images into textual captions with a frozen pre-trained vision-language model (PTVLM).
Though achieving good detection performance, PromptHate suffers from noninformative captions. Generic image descriptions may lack crucial details, such as
race and gender information, vital for detecting hateful content. To address this, we
proposed Pro-Cap, which leverages a frozen PT-VLM to complement PromptHate. Specifically, we prompt a frozen PT-VLM with hateful content-related questions
and use the answers as image captions (termed Pro-Cap), ensuring that the captions
contain critical information for hateful content detection.
While these methods exhibit commendable performance, they heavily rely on
extensive supervised learning, demanding large volumes of annotated data. This
process is both costly and time-consuming. In response, we further introduce ModHATE, which harnesses the power of a composition of tuned PTMs, each of which
possesses an essential reasoning capability for HMD. To the best of our knowledge,
Mod-HATE represents a pioneering exploration of hateful meme detection tailored
to the few-shot learning setting.
For VQA, we study it under the zero-shot transfer setting. Notably, previous
zero-shot VQA models overlooked the explicit consideration of multi-step reasoning
chains inherent in VQA. To address this oversight, We introduce a modularized
zero-shot network that explicitly decomposes questions into sub-reasoning steps,
converts sub-reasoning tasks to objectives suitable for PTMs, and assigns tasks to
appropriate PTMs without adaptation.
Expanding our investigation, we delve into a specific VQA scenario known as
knowledge-based VQA (K-VQA). In K-VQA, apart from an image, external knowledge is indispensable for answering the given questions. Recent approaches have
utilized pre-trained large language models (LLMs) as both a knowledge source and
a zero-shot QA model for K-VQA. However, these recent methods lack explicit
demonstration of the knowledge needed to answer questions and thus lack interpretability. To rectify this deficiency, we propose KGENVQA, which first generates
knowledge from a frozen LLM and subsequently leverages another frozen LLM for
question answering with the incorporation of the generated knowledge.
Finally, we conclude the thesis with a summary of our contributions and a
discussion of potential future directions regarding the application of PTMs to VLU.
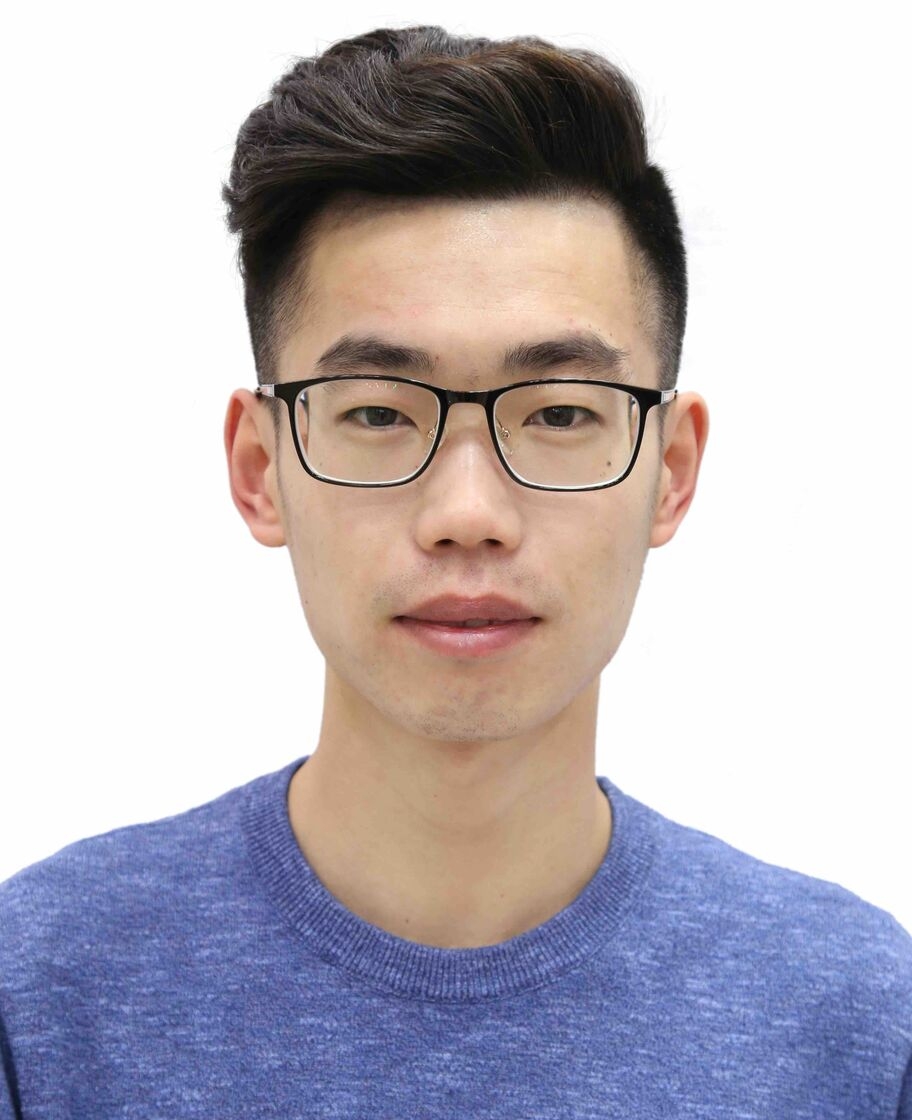
Weakly-Supervised Semantic Segmentation
Semantic segmentation is a fundamental task in computer vision that assigns a label to every pixel in an image based on the semantic meaning of the objects present. It demands a large amount of pixel-level labeled images for training deep models. Weakly-supervised semantic segmentation (WSSS) is a more feasible approach that uses only weak annotations to learn the segmentation task. Image-level label based WSSS is the most challenging and popular, where only the class label for the entire image is provided as supervision. To address this challenge, Class Activation Map (CAM) has emerged as a powerful technique in WSSS. CAM provides a way to visualize the areas of an image that are most relevant to a particular class without requiring pixel-level annotations. However, CAM is generated from the classification model, and it often only highlights the most discriminative parts of the object due to the discriminative nature of the model.
This dissertation examines the key issues behind conventional CAM and proposes corresponding solutions to generate complete CAM. Furthermore, it explores the applicability of the recent visual foundation models, such as the Segment Anything Model (SAM), in the context of WSSS. This exploration provides insights into the potential and challenges of deploying visual foundation models for WSSS, facilitating future developments in this exciting research area.
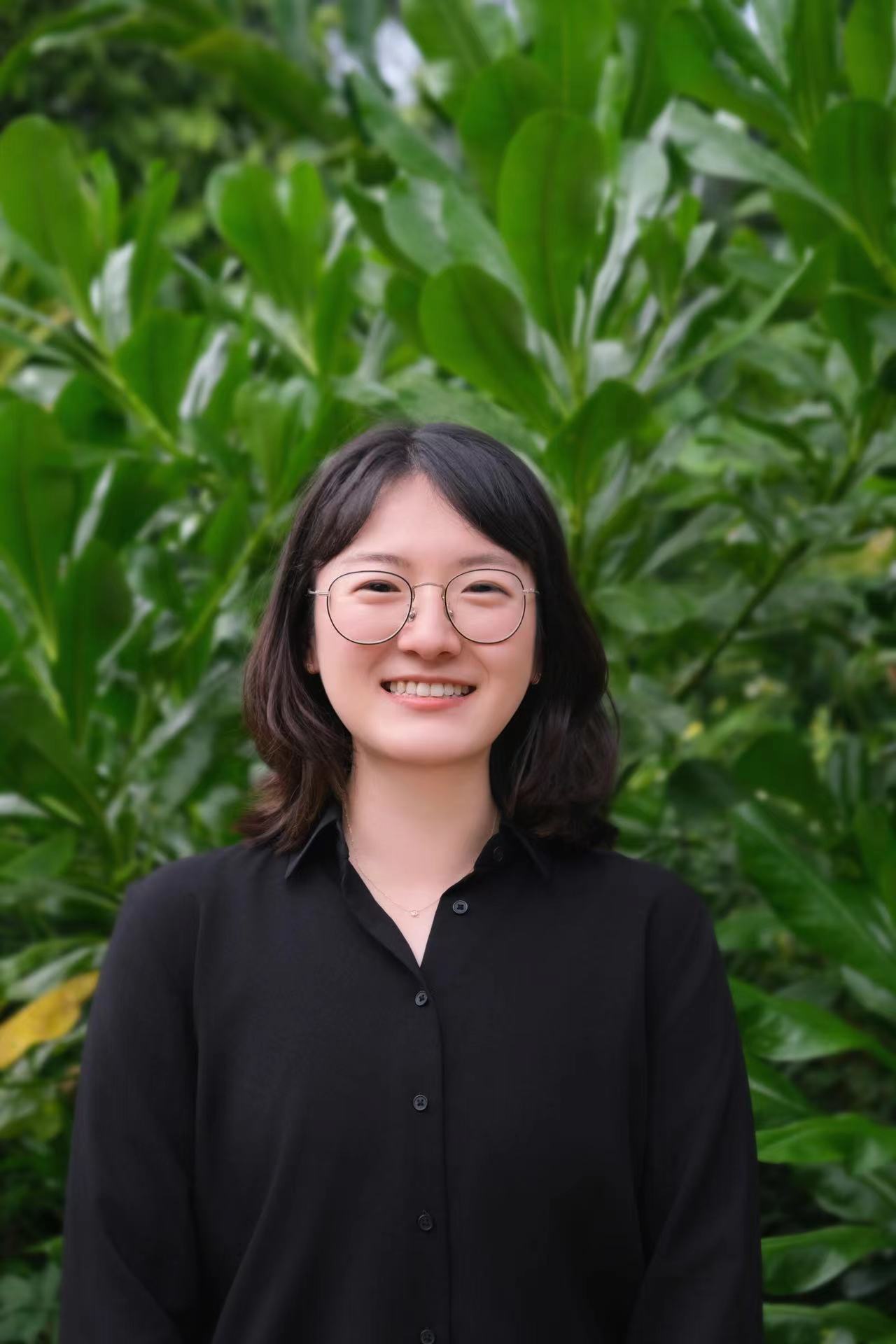
This dissertation consists of three papers that delve into the topic of spatial economics and international trade. The first paper focuses on spatial inequalities. Educational resources are distributed unevenly across space and could contribute to spatial inequality. The first paper develops a dynamic spatial model with life-cycle elements to study the impacts of location-specific educational resources.In the model, individuals determine whether and where to attend college, weighing factors such as distance from home, the expected value of education, and the educational resources available at different destinations. Locations with more colleges attract more students. Moreover, as mobility costs increase with age, many college graduates opt to stay in the city where they studied, leading to long-term changes in skill composition.The model is quantified to the context of China, and the cost of obtaining a college degree in each location is structurally estimated. It's shown that the college expansion between 2005 and 2015 had minimal impacts on welfare and skill composition, as it primarily diverted resources towards locations already well-endowed with colleges. More evenly distributed colleges could improve aggregate welfare and reduce spatial inequality simultaneously.
The second paper considers the U.S.--China trade war. U.S. President Joe Biden has maintained Trump tariffs on Chinese imports, despite the promise to remove them before the 2020 presidential election. The hypothesis that these tariffs can serve as leverage in future tariff negotiations with China is investigated using a quantitative model that incorporates U.S. regions and international trade linkages. After estimating the bargaining power of the U.S. and China, their cooperative tariffs starting from the 2017 baseline and 2019 trade-war equilibrium are computed separately. Simulation results show that, regardless of the relative bargaining power of the U.S., the trade war always improves U.S. welfare in the post-negotiation cooperative equilibrium. With an estimated Nash bargaining weight between 0.47 and 0.70, the trade war with China yields a post-negotiation welfare improvement of 0.04% to 0.05% for the U.S.
The third paper is on the topic of trade policy and considers trade sanctions. Trade sanctions are a common instrument of diplomatic retaliation. To guide current and future policy, the inquiry is: What is the most cost-efficient way to impose trade sanctions against Russia? A quantitative model of international trade with input-output connections is built. Sanctioning countries opt for import tariffs to simultaneously maximize their income and minimize Russia's income, with different weights assigned to these objectives. It is found, first, that for countries with low willingness to pay for sanctions against Russia, the most cost-efficient sanction is a uniform tariff on all Russian products of about 20%. Second, if countries that are willing to pay at least US\$0.70 for each US\$1 drop in Russian welfare, an embargo on Russia's mining and energy products -- with tariffs above 50% on other products -- is the most cost-efficient policy. Finally, if countries target politically relevant sectors, an embargo on Russia's mining and energy sector is the cost-efficient policy, even when there is low willingness to pay for sanctions.
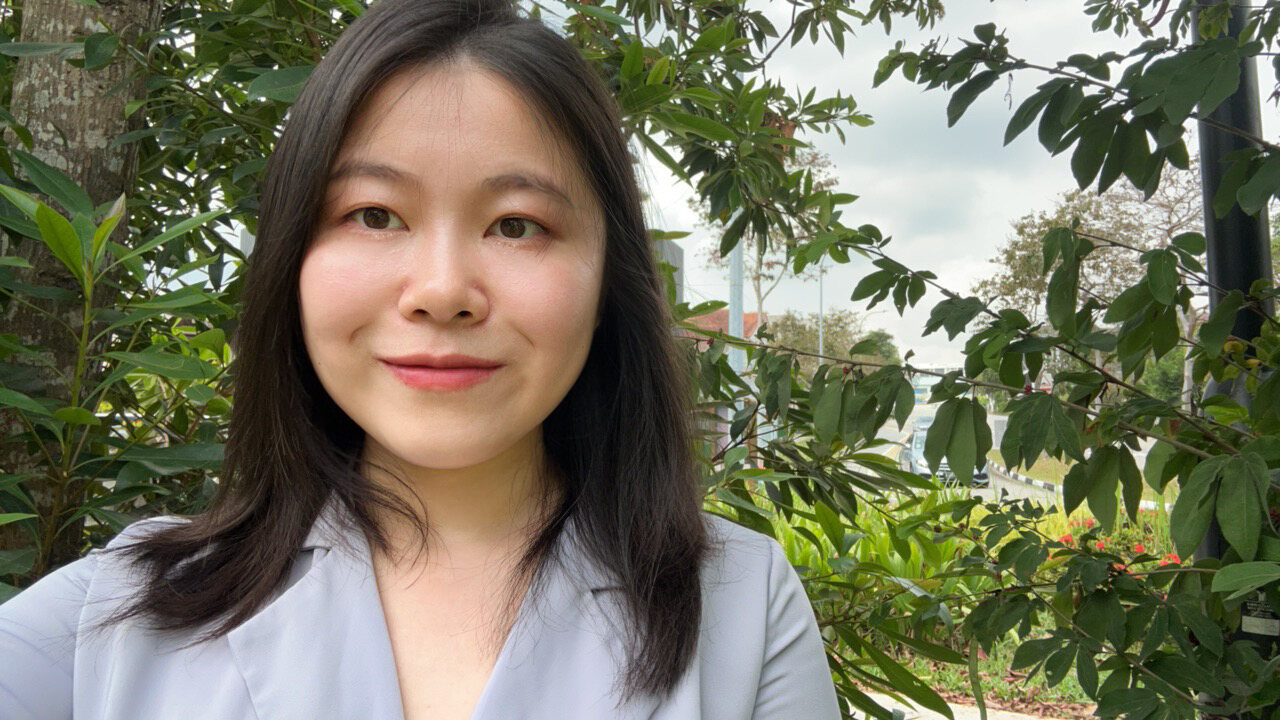
Essays on Foreign Trade Zones
In 1934, in the midst of the Great Depression, the US Congress enacted the Foreign Trade Zones (FTZs) Act, and all states have at least one zone. Zones play a significant role of sourcing foreign products, accounting for about 12% of all imports entering the US and over 28% of total taxable goods during the US-China Trade War. Up to 2021, there were 258 approved FTZs and 442 production firms. Roughly 85% of the outputs are domestically consumed and producers distribute across 144 6-digit NAICS sectors, with 95% belonging to the manufacturing category. 73.3% production firms have local headquarters, Japan accounts for the largest portion of foreign investments. The US government set three main objectives of FTZs, including facilitating international trade, fostering domestic economic activities, and attracting foreign investments. This thesis firstly quantitatively measures the effectiveness of zones with regards to the first two objectives and applies model to propose insights for the government. Secondly, I identify the determining factors in drawing in both domestic and international investors.
Enabling deferral or elimination of duty payments, the US FTZ displayed significant “Cushion Effects” for producers within the zones during the US-China trade war. The first chapter studies these protections of zones in facilitating international trade and domestic production within zones. The first source of “Cushion Effects” resulted from the over 28% increase in the zones' export volume during the tariff war, measured by the extra duties directly exempted. The effect amounted to about 883 million dollars in 2019. In addition, the FTZ demand for sanctioned components used in the production of domestically sold products was less affected due to the deferral and efficiency of duty payments, providing the second source of “Cushion Effects”. I applied the rarely quantitatively analyzed FTZ import data from USITC and compiled trade volumes from the zones' annual reports. The empirical identification results show that tariff shocks triggered more sales of FTZ firms to both foreign and domestic markets at both intensive and extensive margins. This is especially pronounced at the extensive margin: the entrance of 120 new firms was positively correlated with extra tariffs. The supplementary duties that were exempted, temporarily deferred, and non-paid by the year's end quantify “Cushion Effects”. Under the protection, FTZ firms' tendency to pre-storage when anticipating new tariffs or substitute the domestic and non-affected foreign sources of inputs for their sanctioned Chinese counterparts is less pronounced, as the FD and DID models estimate. For the cutting-edge technology inputs of List 2 issued in Section 301 Act, which were also included in the “Made in China 2025” program, the imposed tariff shocks generated positive impacts on FTZ producers' import volumes. Lastly, the empirical observations are mapped theoretically to a two-tier Melitz model, and the counterfactual comparative statistics derived provide a constructive suggestion that the government can enhance the protection by relaxing the criteria of entry into the zones.
The second chapter focuses on the determinants of FDI entry into US FTZs. Up to 2021, a total of 442 production firms have been established within the US Foreign Trade Zones (FTZs), encompassing a diverse spectrum of 144 6-digit NAICS sectors, predominantly affiliated with the manufacturing industry. By examining the ownership structure of FTZ producers at the headquarter level, I find that approximately 26% of them are subsidiaries of foreign parent companies, with Japan representing the largest source of FDI among these entities. To account for the inherent heterogeneity of foreign investments across industries within the zones, this study presents a comprehensive model that encompasses variations in headquarter service intensity, foreign component intensity, and productivity. By employing the compiled dataset, empirical verification is conducted to validate the propositions implied by the model. The findings demonstrate that non-US headquarters exhibit a stronger propensity to enter FTZs when operating within sectors characterized by intensive usage of dutiable inputs. In contrast, the entry of US producers displays stronger responses to sectoral productivity enhancements.
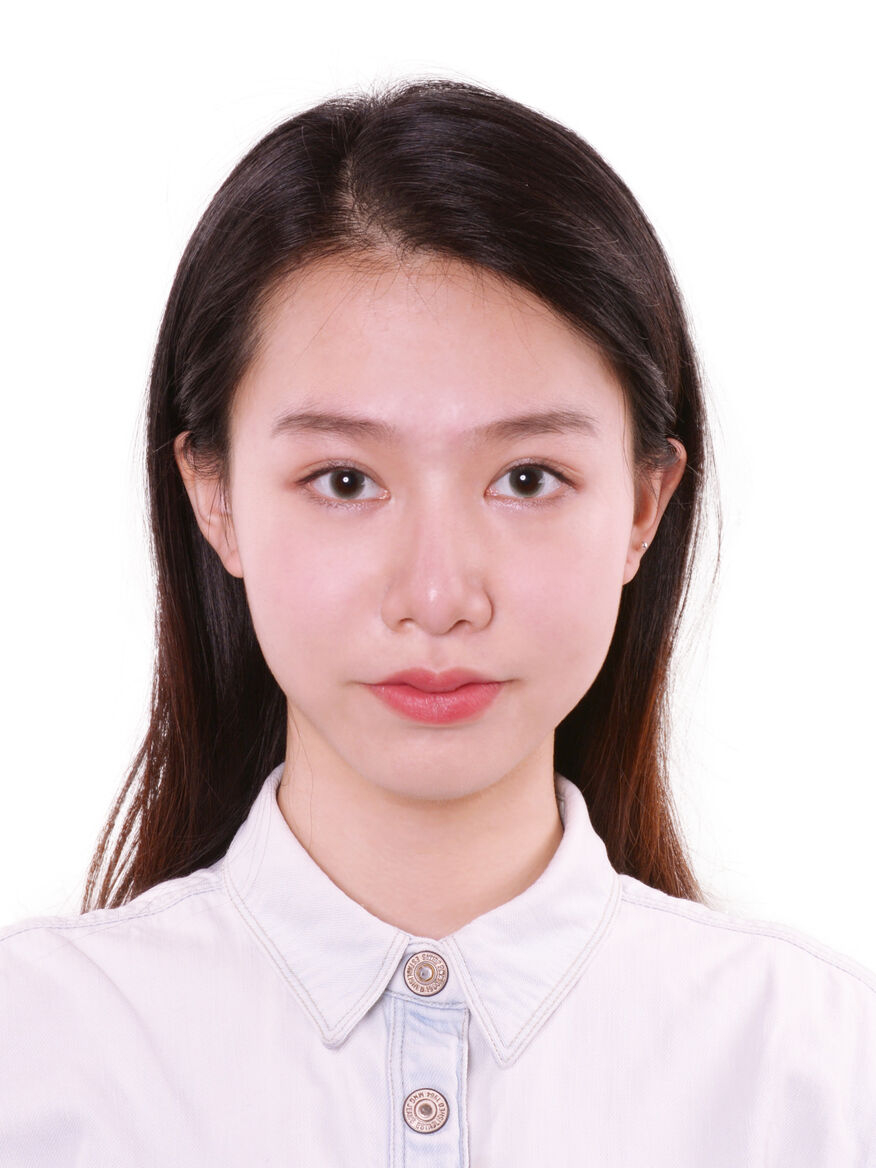
Towards Explainable Neural Network Fairness
Neural networks are crucial in addressing real-world problems but suffer from vulnerability to attacks, opacity, and fairness concerns. Discrimination has been observed in various machine learning models, including Large Language Models (LLMs), which calls for systematic fairness evaluation before their deployment in ethic-relevant domains. When bias is detected, we must methodically enhance fairness.
My dissertation develops techniques to detect and rectify fairness issues in neural networks in a transparent, systematic manner. Initially, we created a method to explain neural network decisions through simple, insightful rules, tackling the "black-box" nature that can lead to bias. The second study introduces a rule-based framework, TestSGD, to pinpoint and measure hidden group discrimination, characterized by interpretable rules and quantified by a fairness score with theoretical error bounds. The third study develops an approach which explore the causes of fairness issue and mitigate them systematically. After evaluating the efficacy of existing fairness methods, which are often ineffective, we present an adaptive method, guided by causality analysis, to choose the most suitable fairness enhancement tactic based on the distribution of biased neurons and attributes. Finally, we present a method which would allow us to extend our fairness mitigation approach to LLMs. We propose a non-intrusive bias mitigation strategy for LLMs, leveraging a parameter-efficient debias adapter that systematically mitigate biases and offers theoretical guarantees during the debiasing process. This approach is non-intrusive which does not require accessing or modifying the internals of LLMs.
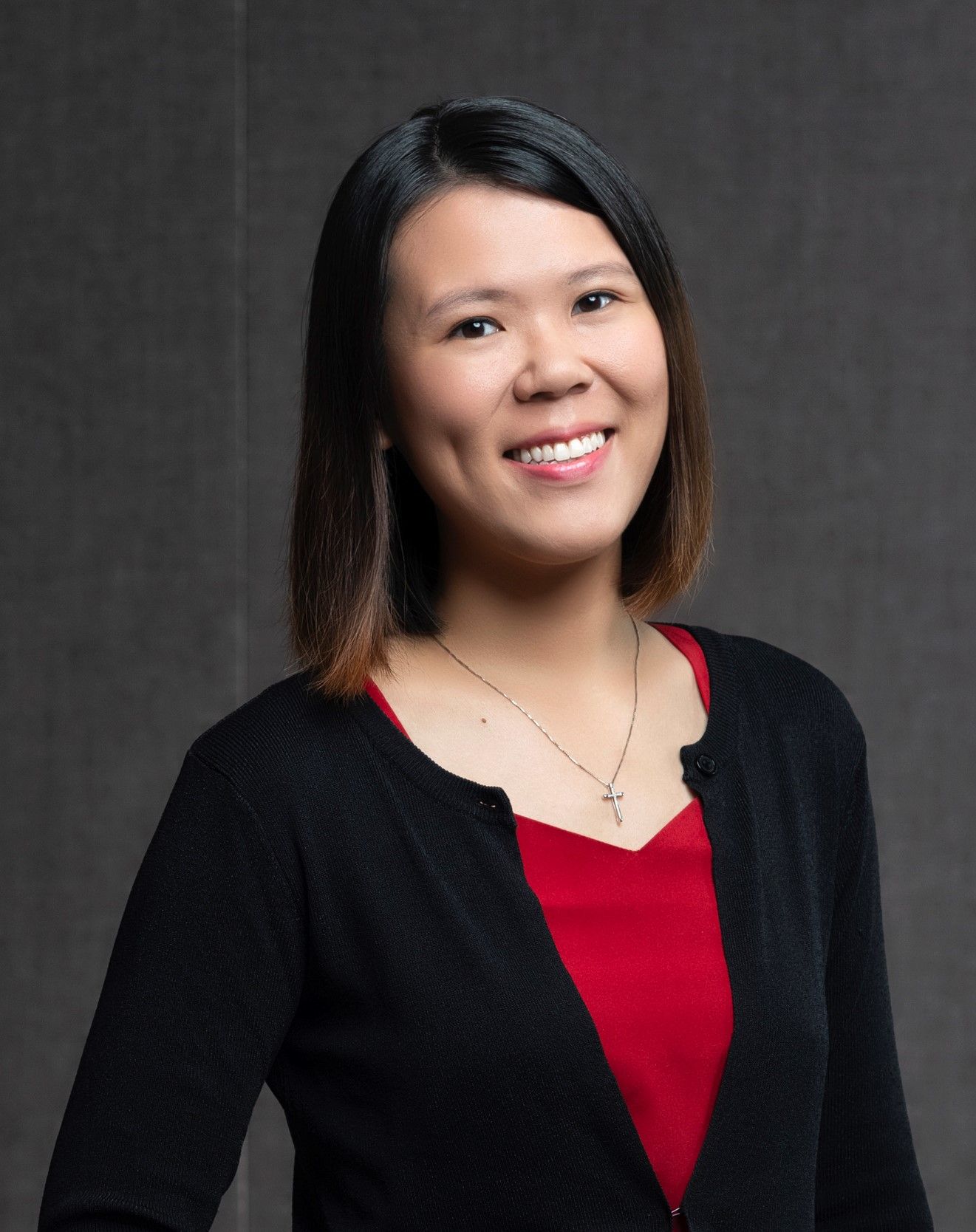
The Role of Disclosure in DeFi Markets
Decentralized Finance (DeFi) platforms use self-executing smart contracts to provide financial services and are programmed to automatically post all information on the public blockchain. Notwithstanding this public availability of blockchain information, DeFi platforms also extract public blockchain data and disclose the summarized blockchain information on their Twitter accounts. This paper studies whether and how voluntary disclosure of blockchain information plays a role in the transparent DeFi market. I find that the number of blockchain-related tweets is associated both with an increase in the platform’s Total Value Locked (TVL) and with an increase in the total number of platform users. The relationship between blockchain-related tweets and TVL is strengthened when the tweets have greater information content and when users face higher information processing costs. This suggests that public blockchain transactions are too costly for users to process such that they rely on the platform’s disclosure of blockchain information. Overall, my results show that DeFi platforms can help users process and understand blockchain transactions by summarizing and disclosing them on Twitter.
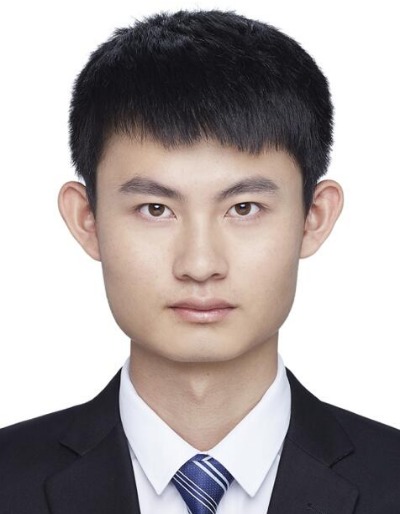
Document graph representation learning
Much of the data on the Web can be represented in a graph structure, ranging from social and biological to academic and Web page graphs, etc. Graph analysis recently attracts escalating research attention due to its importance and wide applicability. Diverse problems could be formulated as graph tasks, such as text classification and information retrieval. As the primary information is the inherent structure of the graph itself, one promising direction known as the graph representation learning problem is to learn the representation of each node, which could in turn fuel tasks such as node classification, node clustering, and link prediction.
As a specific graph data, documents are usually connected in a graph structure. For example, Google Web pages hyperlink to other related pages, academic papers cite other papers, Facebook user profiles are connected as a social network, news articles with similar tags are linked together, etc. We call such data document graph or document network. To better make sense of the meaning within these text documents, researchers develop neural topic models. By modeling both textual content within documents and connectivity across documents, we can discover more interpretable topics to understand the corpus and better fulfill real-world applications, such as Web page searching, news article classification, academic paper indexing, and friend recommendation based on user profiles, etc. However, traditional topic models explore the content only, ignoring the connectivity. In this dissertation, we aim to develop models for document graph representation learning.
First, we investigate the extension of Auto-Encoders, a family of shallow topic models. Intuitively, connected documents tend to share similar latent topics. Thus, we allow Auto-Encoder to extract topics of the input document and reconstruct its adjacent neighbors. This allows documents in a network to collaboratively learn from one another, such that close neighbors would have similar representations in the topic space. Extensive experiments verify the effectiveness of our proposed model against both graphical and neural baselines.
Second, we focus on dynamic modeling of document networks. In many real-world scenarios, documents are published in a sequence and are associated with timestamps. For example, academic papers published over the years exhibit the development of research topics. To incorporate such temporal information, we introduce a neural topic model aimed at learning unified topic distributions that incorporate both document dynamics and network structure.
Third, we discover that documents are usually associated with authors. For example, news reports have journalists specializing in writing certain type of events, academic papers have authors with expertise in certain research topics, etc. Modeling authorship information could benefit topic modeling, since documents by the same authors tend to reveal similar semantics. This observation also holds for documents published on the same venues. We propose a Variational Graph Author Topic Model for documents to integrate both topic modeling and authorship and venue modeling into a unified framework.
Fourth, most previous topic models treat documents of different lengths uniformly, assuming that each document is sufficiently informative. However, shorter documents may have only a few word co-occurrences, resulting in inferior topic quality. Some other previous works assume that all documents are short, and leverage external auxiliary data, e.g., pretrained word embeddings and document connectivity. Orthogonal to existing works, we remedy this problem within the corpus itself by meta-learning and proposing a Meta-Complement Topic Model, which improves topic quality of short texts by transferring the semantic knowledge learned on long documents to complement semantically limited short texts.
Fifth, we explore the modeling of short texts on the graph. Text embedding models usually rely on word co-occurrences within the documents to learn effective representations. However, short texts with only a few words may influence the learning process. To accurately discover the main topics of these short documents, we propose a new statistical concept, i.e., optimal transport barycenter, to incorporate external knowledge, such as pre-trained word embedding on a large corpus, to improve topic modeling. The proposed model shows state-of-the-art performance.
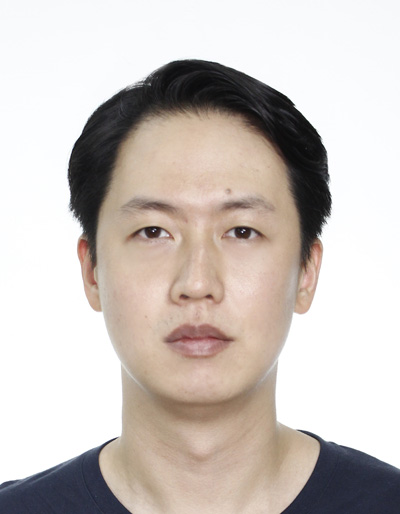
In this presentation, we will discuss various aspects of semantic data representations, which we broadly group into two categories. First, effective semantic representations focuses on aspects generally related to the capabilities of these representations, such as task performance and interpretability. Next, efficient semantic representations encompasses aspects which generally related to the utilization of these representations, such as their storage size as well as generalizability across multiple tasks.
Our discussion revolves around two primary forms of data, textual data as well as knowledge bases. For textual data representations, we introduce a novel approach that improves efficiency through discarding representations, while limiting the impacts on downstream task effectiveness. For knowledge base representations, we explore a novel measure of node importance in knowledge graphs, and present a heuristic approach for selecting such nodes in large knowledge graphs.
We also discuss the use of semantic representations in real world applications, and propose a novel approach for the cold-start problem when training Large Language Models in the legal domain.
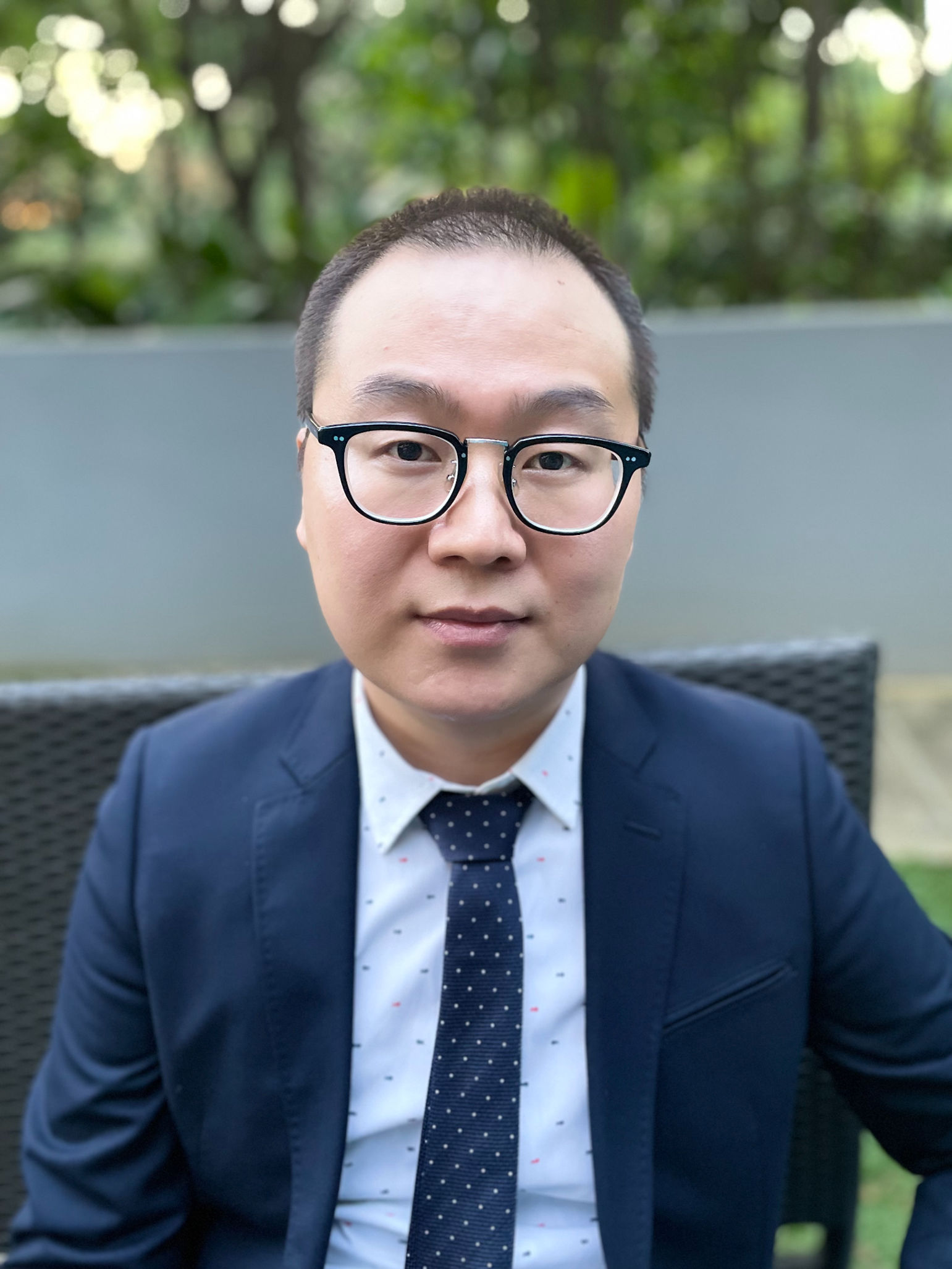
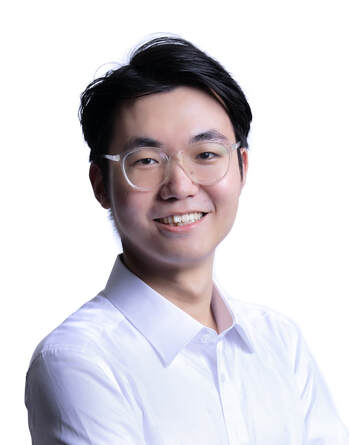
Essays on stakeholder economy
The dissertation consists of two chapters on stakeholder economy. It looks at how firms interact with the stakeholders, including not only investors, employees, customers, governments, but also the broader community and society at large, and examines how such interactions affect corporate behavior in China and the global setting. The first chapter studies how societal culture shapes firm behavior and growth by analyzing the trade-off of relying on trust in acquiring stakeholder resources, and testing with data on the number of historic Confucian schools surrounding a current firm’s location in China. Companies more exposed to Confucianism have greater social contributions and stakeholder protection, and more business courtesy expenses, patents, and trade credits, which match the five basic virtues of Confucianism: benevolence, righteousness, courteousness, wisdom, and trustworthiness. Our results cannot be explained by other cultural traits and are robust to using the distance to the prototypical Confucian academies in the Song Dynasty and the intensity of rivers in the local region as instrumental variables. The effects are likely to be transmitted via a firm’s interaction with market participants, politicians’ ideology, and board of directors. Stronger Confucianism is associated with greater profitability and growth. Our paper contributes to the literature by providing more granular evidence on how culture affects economic activities through firm-level channels, which have not been systematically explored in the literature.
In the second chapter, we employ a novel firm-level dataset on monetized value of unpriced earnings losses due to climate-related transition risks to study the magnitudes, determinants and consequences of a firm’s carbon earnings risks across different scenarios based on national pledges to Paris Agreement targets and different time horizons. We find carbon earnings risks on average account for about 15 percent of a firm’s total earnings and are largely driven by unobservable industry- and firm-level heterogeneities. We also find that companies with greater carbon earnings risks tend to have more green innovations, discretionary accruals, and outsourced productions. We use the staggered introduction of country-level carbon tax and emission trading system, as well as state-level climate-related disasters as instrumental variables to address potential endogeneity issues. Our findings highlight the importance of accounting for transition risks in a firm’s financial statements. Our work complements the growing climate finance literature on the effect of climate risks on corporate policies by providing more comprehensive evidence on the motivation of corporate reaction, driven by material carbon earnings risks that are reflected on a firms financials.
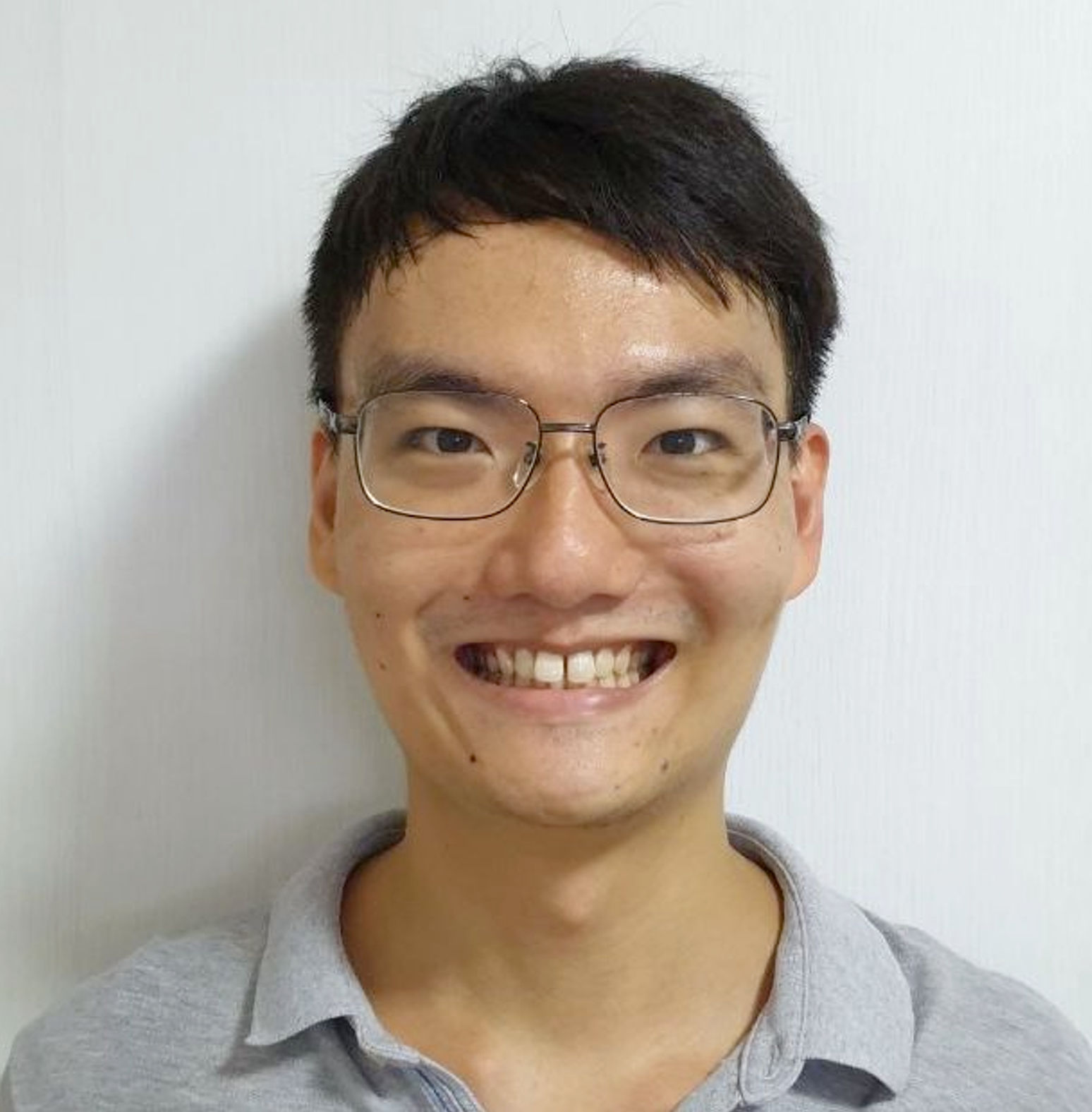
Fortifying the seams of software systems
A seam in software is a place where two components within a software system meet. There are more seams in software now than ever before as modern software systems rely extensively on third-party software components, e.g., libraries. Due to the increasing complexity of software systems, understanding and improving the reliability of these components and their use is crucial. While the use of software components eases the development process, it also introduces challenges due to the interaction between the components.
This dissertation tackles problems associated with software reliability when using third-party software components. Developers write programs that interact with libraries through their Application Programming Interfaces (API). Both static and dynamic analysis of API-using code require knowledge of the API and its usage constraints. Hence, we develop techniques to learn and model the usage constraints of APIs. Next, we apply the insights gleaned from our studies to support bug-finding techniques using static and dynamic analysis. Then, we look into larger software systems comprising multiple components. We propose techniques for mining rules to monitor the joint behaviors of apps, and for exploiting known library vulnerabilities from a project importing a library. These techniques aim to assist developers to better understand third-party components, and to detect weaknesses in software systems.
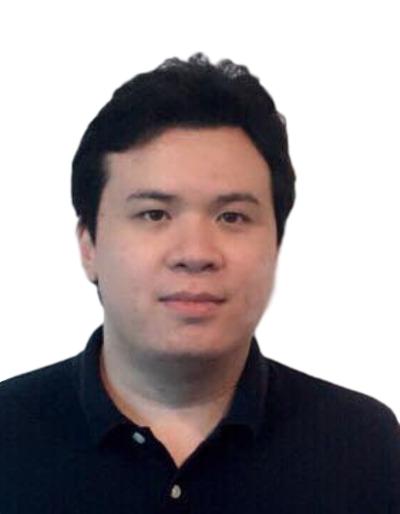
Continual Learning with Neural Networks
Recent years have witnessed tremendous successes of artificial neural networks in many applications, ranging from visual perception to language understanding. However, such achievements have been mostly demonstrated on a large amount of labeled data that is static throughout learning. In contrast, real-world environments are always evolving, where new patterns emerge and the older ones become inactive before reappearing in the future. In this respect, \emph{continual learning} aims to achieve a higher level of intelligence by learning online on a data stream of several tasks. As it turns out, neural networks are not equipped to learn continually: they lack the ability to facilitate knowledge transfer and remember the learned skills. Therefore, this thesis has been dedicated to developing effective continual learning methods and investigating their broader impacts on other research disciplines.
Towards this end, we have made several contributions to facilitate continual learning research. First, we contribute to the classical continual learning framework by analyzing how Batch Normalization affects different replay strategies. We discovered that although Batch Normalization facilitates continual learning, it also hinders the performance of older tasks. We named this the \emph{cross-task normalization phenomenon} and conducted a comprehensive analysis to investigate and alleviate its negative effects.
Then, we developed a novel \emph{fast and slow learning} framework for continual learning based on the \emph{Complementary Learning Systems}~\cite{kumaran2016learning,mcclelland1995there} of human learning. Particularly, the fast and slow learning principle suggests to model continual learning at two levels: general representation learning and learning of individual experience. This principle has been the main tool for us to address the challenges of learning new skills while remembering old knowledge in continual learning. We first realized the fast-and-slow learning principle in Contextual Transformation Networks (CTN) as an efficient and effective online continual learning algorithm. Then, we proposed DualNets, which incorporated representation learning into continual learning and proposed an effective strategy to utilize general representations for better supervised learning. DualNets not only addresses CTN's limitations but is also applicable to general continual learning settings.
Through extensive experiments, our findings suggest that DualNets is an effective and achieved strong results in several challenging continual learning settings, even in the complex scenarios of limited training samples or distribution shifts.
Furthermore, we went beyond the traditional image benchmarks to test the proposed fast-and-slow continual learning framework on the online time series forecasting problem. We proposed Fast and Slow Networks (FSNet) as a radical approach to online time series forecasting by formulating it as a continual learning problem. FSNet leverages and improves upon the fast-and slow learning principle to address two major time series forecasting challenges: fast adaptation to concept drifts and learning of recurring concepts. From experiments with both real and synthetic datasets, we found FSNet's promising capabilities in dealing with concept drifts and recurring patterns.
Finally, we conclude the dissertation with a summary of our contributions and an outline of potential future directions in continual learning research.
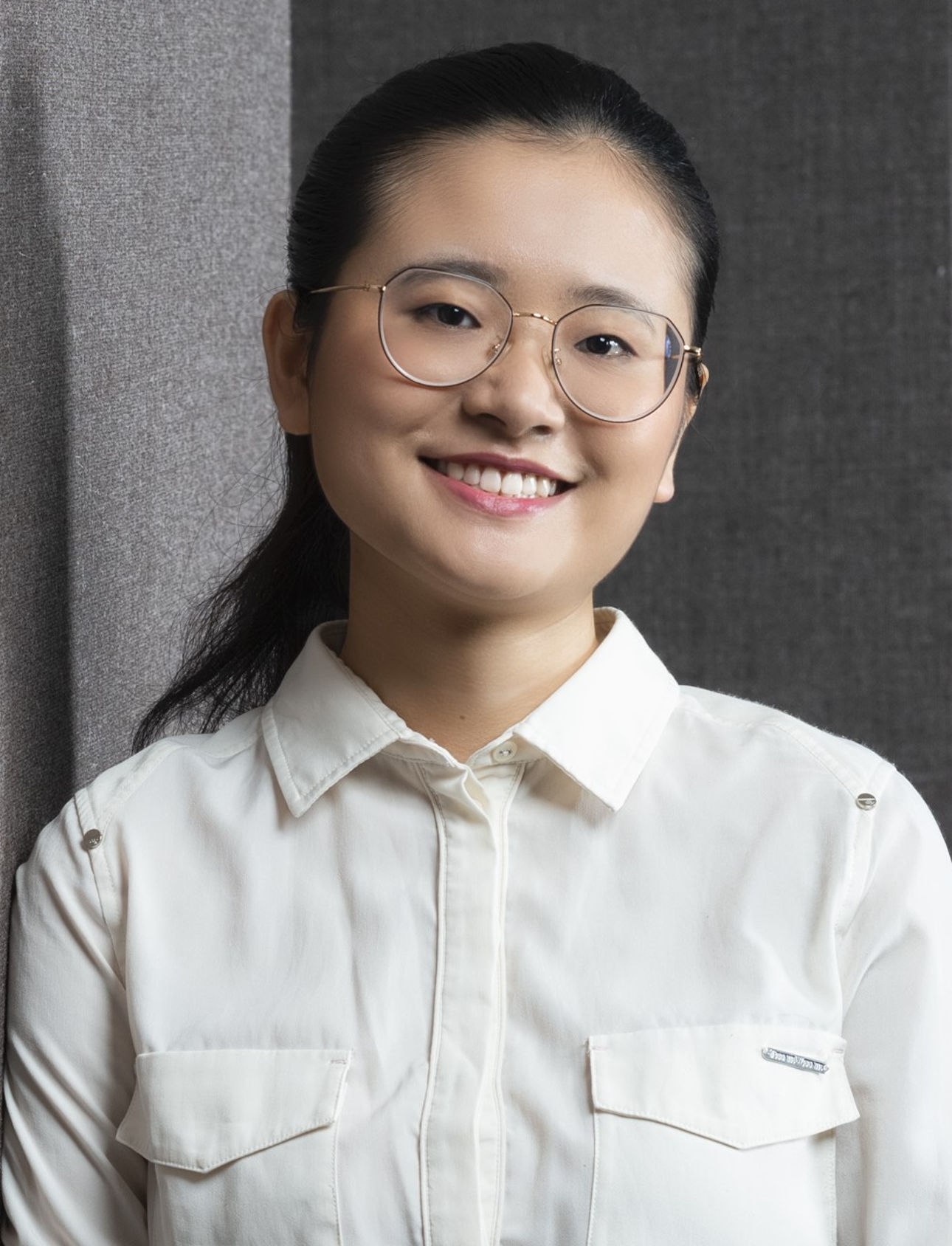
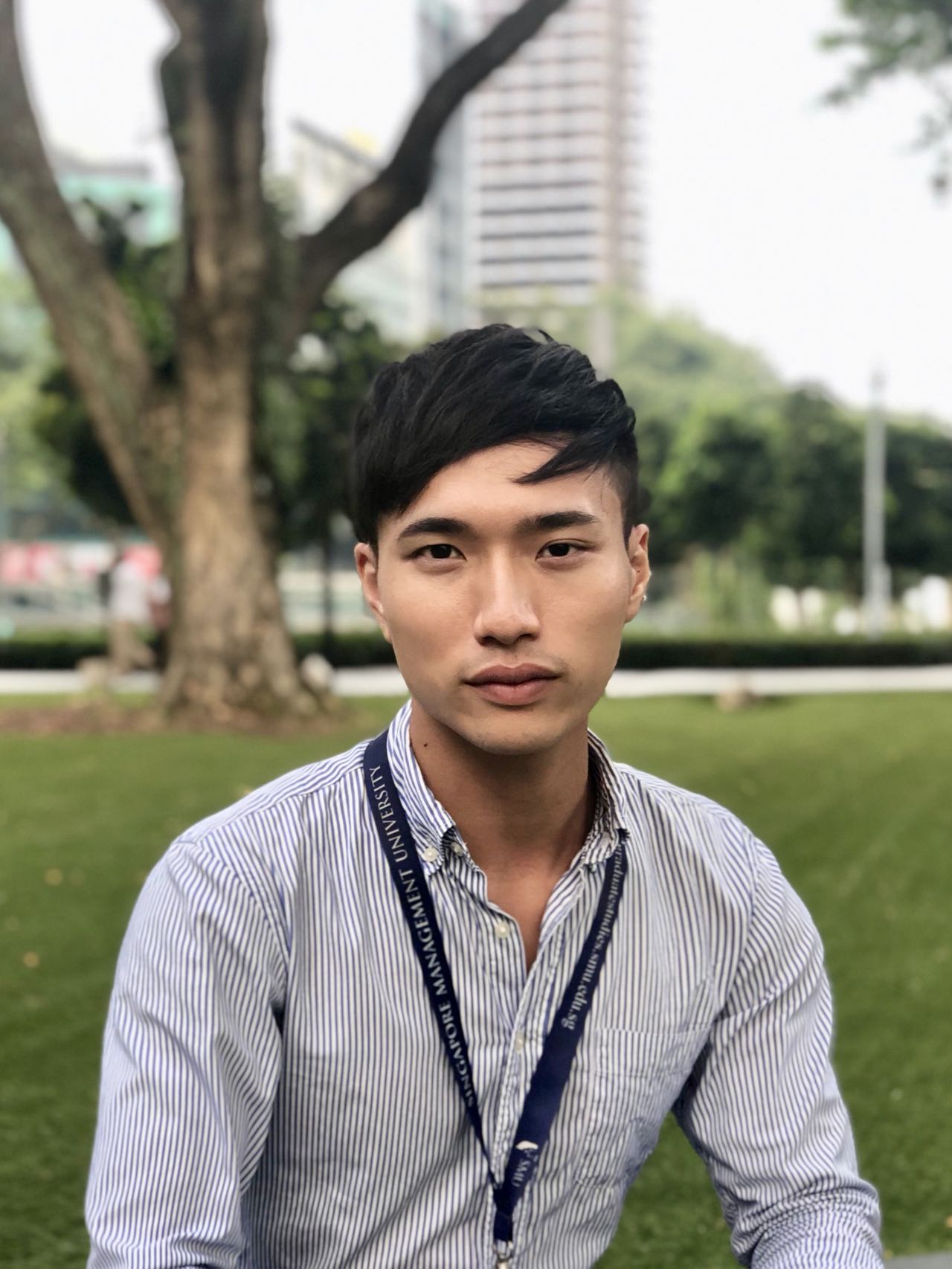
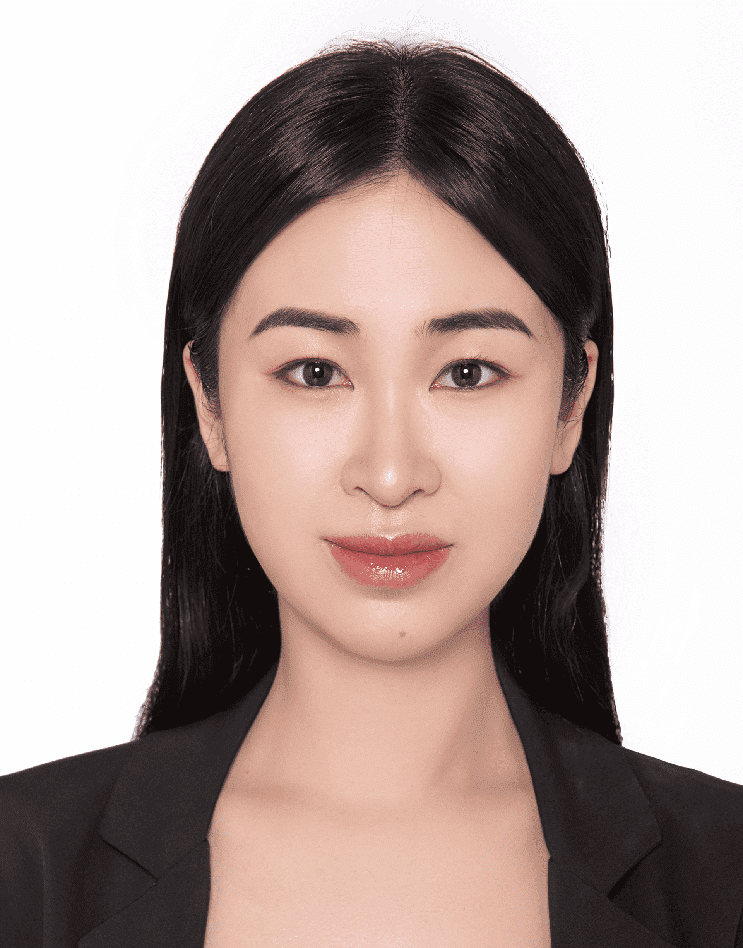
This dissertation investigates the impact of acquisition activities on the current partners of the involved firms and the restructuring of their alliance portfolios. The first essay examines how acquisitions by a firm’s current alliance partners influence this firm’s subsequent alliance formation. The literature suggests that if a focal firm’s current alliance partners acquire targets that are in the same industry as the focal firm, the focal firm would be concerned about these alliance partners’ commitment, their increased bargaining power, and opportunistic behaviors. This essay contends that in response, the focal firm will form alliances with new partners to mitigate concerns about potential reduction in resource capture in its current alliances and to reduce dependence on current partners. This essay also theorizes how the focal firm’s status relative to its partners and its ego network density mitigate this tendency. This essay expands the knowledge about how firms react to alliance partners’ strategic activities. The second essay explores how acquisition premiums influence the acquirers' subsequent alliance formation. It reveals that acquirer firms paying higher acquisition premiums tend to engage in fewer new alliances afterward. However, this tendency diminishes when the relational embeddedness between the acquirer and the target increases, or when the acquirer holds a higher centrality or brokerage position. This essay expands the existing literature on acquisition premiums by shedding light on their influence on acquirers' external interorganizational relationships. The third essay examines the impact of acquisitions on the economic gains experienced by the common partners of both the acquirer and target firms, as evidenced by market reactions to the announcement of the acquisition. The hypothesis posits that acquisitions have a negative effect on the stock market returns of these common partners, attributed to a decrease in bargaining power. Additionally, this essay proposes that factors such as the number of other common partners and previous alliance experiences between the acquirer and target may mitigate this negative impact. This essay enriches the existing literature on acquisitions by providing new insights into implications for third parties and interorganizational relationships.
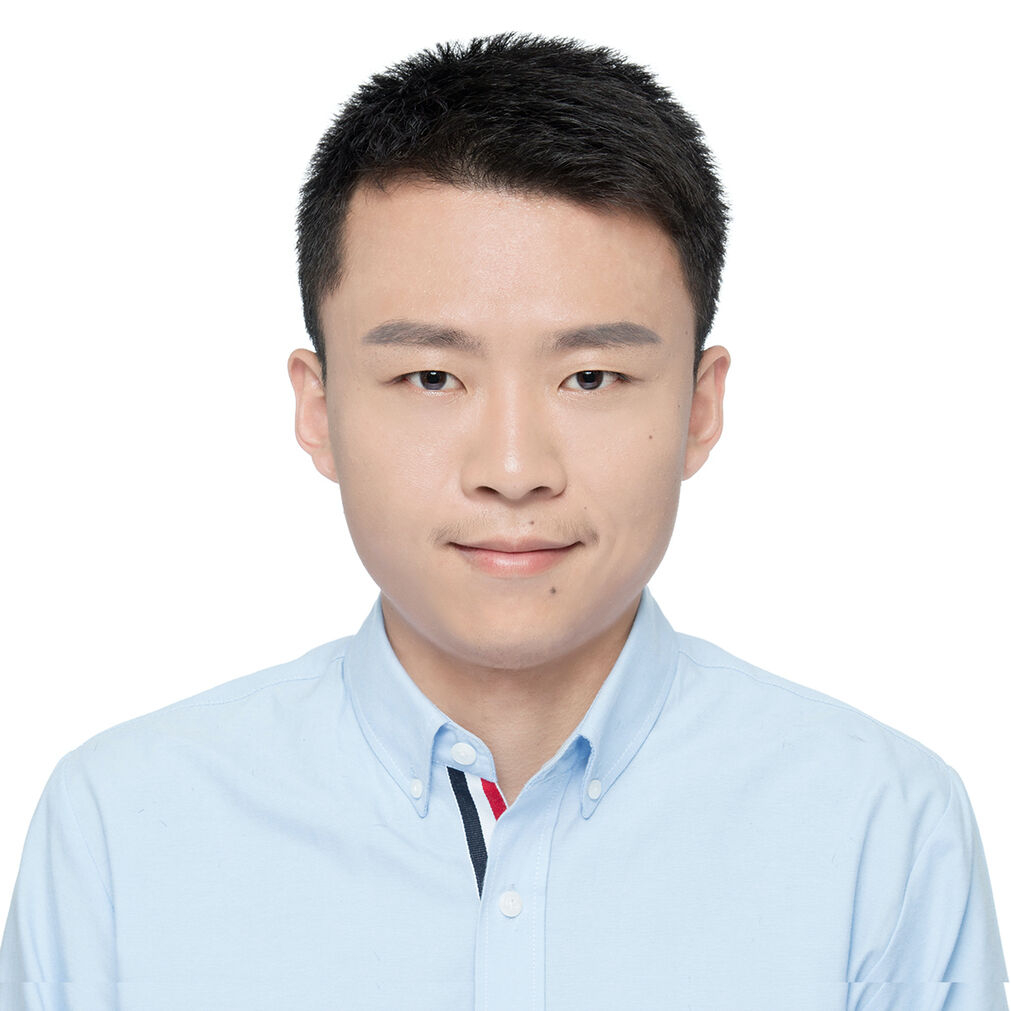
Given the rapid pace of urbanization, there is a pressing need to optimize urban logistics delivery operations for enhanced capacity and efficiency. Over recent decades, a multitude of optimization approaches have been put forth to address urban logistics challenges, encompassing routing and scheduling within both static and dynamic contexts. In light of the rising computational capabilities and the widespread adoption of machine learning in recent times, there is a growing body of research aimed at elucidating the seamless integration of data and machine learning within conventional urban logistics optimization models. Additionally, the ubiquitous utilization of smartphones and internet innovations presents novel research challenges in the realm of urban logistics, notably in the domains of last-mile delivery collaboration and on-demand food delivery services.
My PhD research is driven by these new demands, exploring how data-driven methods can improve urban logistics. This thesis will encompass a comprehensive discussion of my research conducted in three key domains: (1) collaborative urban delivery with alliances; (2) dynamic service area sizing optimization for on-demand food delivery services; and (3) optimization of dynamic matching time intervals for on-demand food delivery services.
This dissertation consists of three chapters on Search Models of Money.
The first chapter is a review of recent advances in Search Models of Money. It reviews the Lagos and Wright (2005) framework which is the workhorse of many modern search models with applications to models with Competing Media of Exchange to Fiat Currency, and models with Money and Credit. We trace the history of the development of search models of money from the first generation to present day. We highlight recent developments that address puzzles such as the coexistence of money in an environment where an asset serves as both an alternative means-of-payment and a superior store of value. We look at search models of money with credit which address the fact that in the original LW framework, credit could not exist because agents are anonymous in the decentralized market while in the centralized market all agents can work with linear utility in hours rendering credit unnecessary.
The second chapter explores the adoption and acceptance of alternative means-of-payment to fiat currency. We determine the inflation rate and transaction costs of adoption that encourage the adoption of an alternative means-of-payment. However, the buyer’s bargaining power must also be high enough for money and the asset to co-exist as means of payment, otherwise buyers will choose to use money only for low inflation and asset only for high inflation. We observe that when inflation is low, for a given fraction of acceptance of the alternative means-ofpayment by sellers, and the cost of holding money is not great so the benefit of using the asset as an alternative means-of-payment to the buyer is negative or zero, and buyers will not adopt the asset. At high inflation when the asset is adopted and accepted as an alternative means-of-payment, when acceptance rate is low, welfare gains are limited because agents do not use too much of the asset as an alternative means-of-payment. However, when the acceptance rate is high, the welfare gains are much higher. In equilibria where money and the asset co-exist as means of payment, increasing the seller’s acceptance rate of the asset as means-of-payment encourages the adoption of the asset as means-of-payment at lower inflation rates.
The third chapter investigates consumer behaviour in an environment with two types of credit – secured and unsecured credit, and with four types of agents – (1) low-income agents with high consumption needs, (2) high-income agents with high consumption needs, (3) low-income agents with low consumption needs, and (4) high-income agents with low consumption needs. Given each agent has a strictly less than one probability of access to financial markets or credit, this gives rise to a total of eight heterogenous agents. As inflation increases, the cost of money increases resulting in agents carrying less fiat currency and relying more on credit to finance their consumption needs. Low-income agents with high consumption needs are always the first to require credit while in most situations, high-income agents with low consumption needs never need credit. Credit relaxes liquidity constraints of agents and as inflation increases, welfare decreases because agents carry less money and rely on credit to finance consumption needs. At high levels of inflation, agents start to have insufficient liquidity to obtain the optimal DM quantity of good. Calibrating to US data, we find welfare loss range from 1% to 4% for every 0.1% increase in inflation. Because of our diverse types of agents, we are able to show that inflation affects high consumption agents the most, especially those without access to credit.
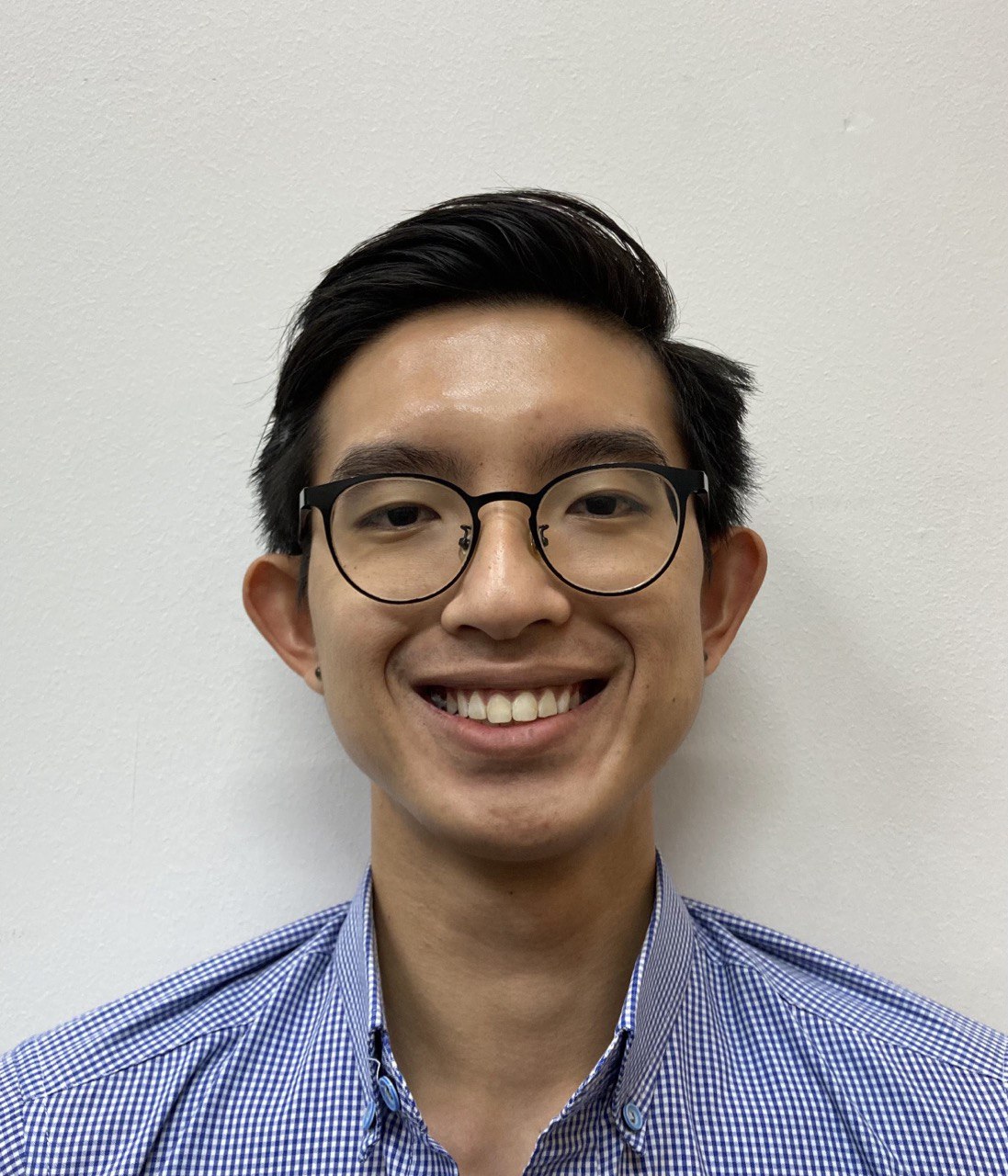
Recent literature indicates that a lack of personal control negatively predicts (social) cynicism, a negative view of others as self-interested and exploitative (Stavrova & Ehlebracht, 2018a, 2019). Despite the ostensibly robust nature of this relationship, I propose that the strength of the link between personal control and cynicism could be more variable than extant findings have suggested. In particular, I argue that variability in the controlcynicism link may be tracked (i.e., moderated) by the extent to which actors in a situation have corresponding or conflicting interests, with the effect of control on cynicism being attenuated when actors are perceived to have corresponding (vs. conflicting) interests. Furthermore, I reason that perceptions of vulnerability to exploitation should mediate the effect of control (and interests) on cynicism. Overall, the present research hypothesized a moderated mediation model linking personal control, interests, vulnerability, and cynicism. Four studies were conducted: three experiments that employed economic games (Study 1) and vignettes (Study 2 and 3), and one large-scale, cross-cultural correlational study (Study 4). Findings were broadly consistent with the theoretical model: the link between control and cynicism was mediated by perceptions of vulnerability and was attenuated in situations with corresponding (vs. conflicting) interests. The implications and limitations of the current research are discussed. Overall, the findings suggest that shaping people’s perceptions of interests in a situation can be one useful way to help stem the cynicism that arises from a lack of personal control.
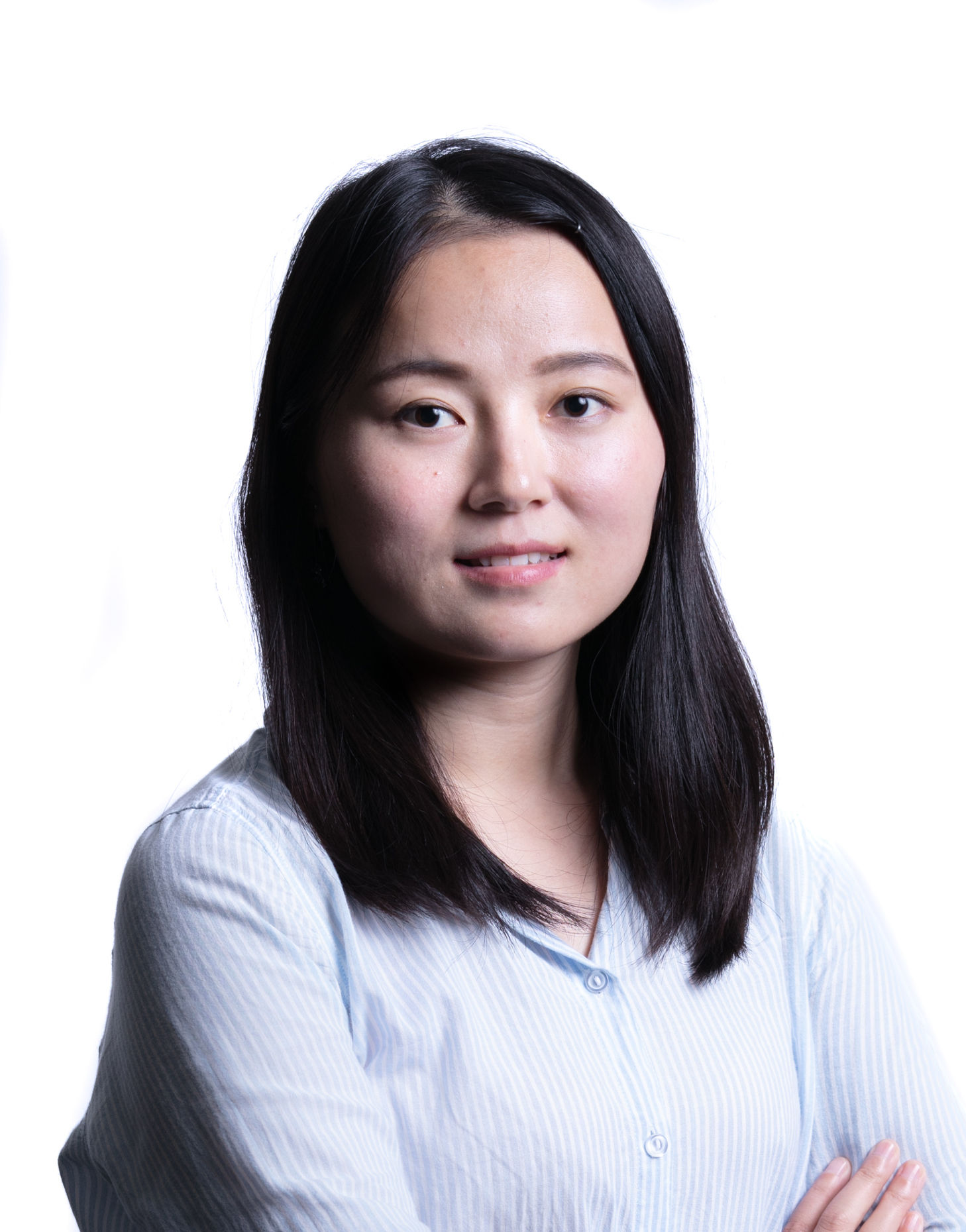
Essays on new business models in operations
This dissertation consists of three essays about problems of managing operations with emerging new business models that are broadly related to anti-counterfeiting, car subscription programs, and on-demand ride-hailing services. In the following three chapters, each studies one type of new business model with opportunities and challenges, and builds analytical models to explore the implications on firms' operational decisions.
Chapter 2 studies the emergence of “super fakes,” and investigates the effectiveness of the new anti-counterfeiting measure — converting counterfeiters to authorized suppliers. We employ a game-theoretic model to examine interactions between a brand-name firm with its home supplier, and a counterfeiter who produces high-quality counterfeits and can be potentially converted to an authorized overseas supplier. We demonstrate that it is easier for the brand-name firm to combat counterfeiting through conversion than by driving the counterfeiter out of the market. We examine the impact of this new measure on consumer and social surplus, and find that it may hurt consumer surplus and does not always improve social surplus.
Chapter 3 studies flexible versus dedicated technology choice and capacity investment decision of a two-product manufacturing firm under demand uncertainty in the presence of subscription programs. The key feature of subscription programs is that a proportion of customers that are allocated a particular product later switches to using the other product (if available). We build a two-stage stochastic program to study the optimal technology choice and capacity investment decision, and the subsequent product allocation and reservation for each product. We investigate how the demand correlation and the switching proportion affect the profitability with each technology, and shape the optimal technology choice decision.
Chapter 4 studies an on-demand ride-hailing platform partnering with traditional taxi companies for expanding the supply of drivers, and the government’s regulation problem of access control of taxi drivers to on-demand ride-hailing requests under such emerging partnership. We examine the conditions under which taxi drivers participate in providing both street-hailing and on-demand ride-hailing services. We investigate whether and how the government should make regulatory decisions to maximize social welfare. We find that advocating their partnership by allowing taxi drivers to get ``full access" to the platform may not be optimal and the regulation is needed.
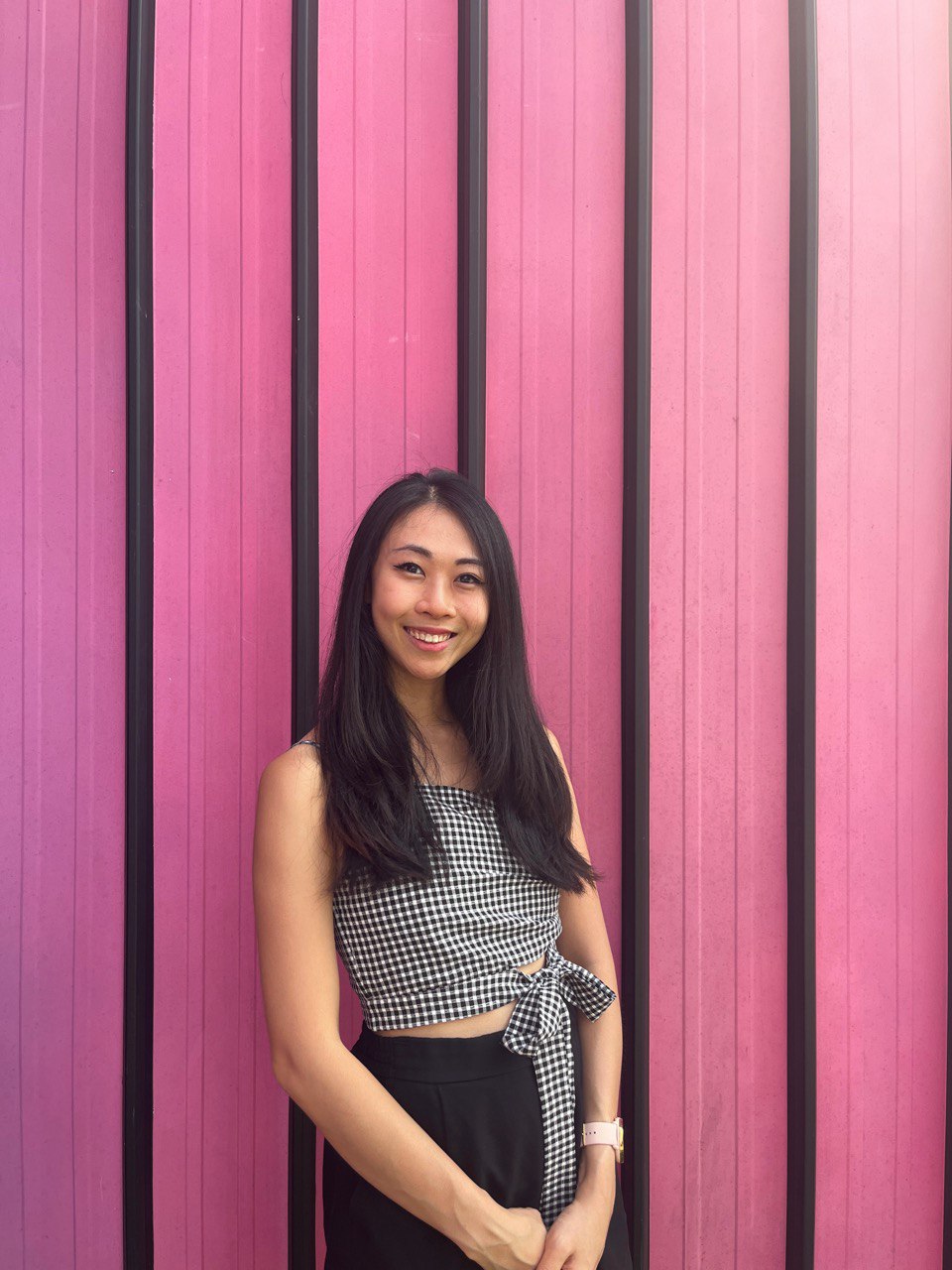
Is this Behaviour Impressive or Repulsive? The Influence of Our Ecology on Our Social Evaluations
Sexual unrestrictedness, impulsivity, and a short-term orientation—these traits generally carry negative connotations and tend to be frowned upon. However, are they necessarily maladaptive? Evolutionary psychologists map these traits onto a behavioural cluster known as a fast life strategy. While a wide body of work has examined many types of prejudices (e.g., sexism, ageism, racism, classism, attractiveness bias etc.) the literature has yet to examine prejudices against behaviours that lie on the life history strategy continuum. I propose that in our modern world where life is relatively predictable and mortality rates are lower than in ancestral times, there exists a general negative bias towards fast (versus slow) life strategy traits (H1). Further, I expect that this bias would be attenuated by perceptions of ecological harshness (i.e., mortality threats) because a fast strategy offers adaptive value under conditions of threat (H2). I test these hypotheses across several studies (Total N = 1500 participants from the USA). Study 1 assesses affective reactions that people have towards descriptions of a fast (vs slow) life strategy. Study 2 provides a high-powered replication while examining an exploratory mediator, net perceived affordance. Study 3 adopts a full factorial experimental design, manipulating ecology perceptions and the life strategy of the target. The results generally support our hypotheses that people hold unfavourable views toward fast (versus slow) strategy behaviours, but this can be mitigated by ecology perceptions.
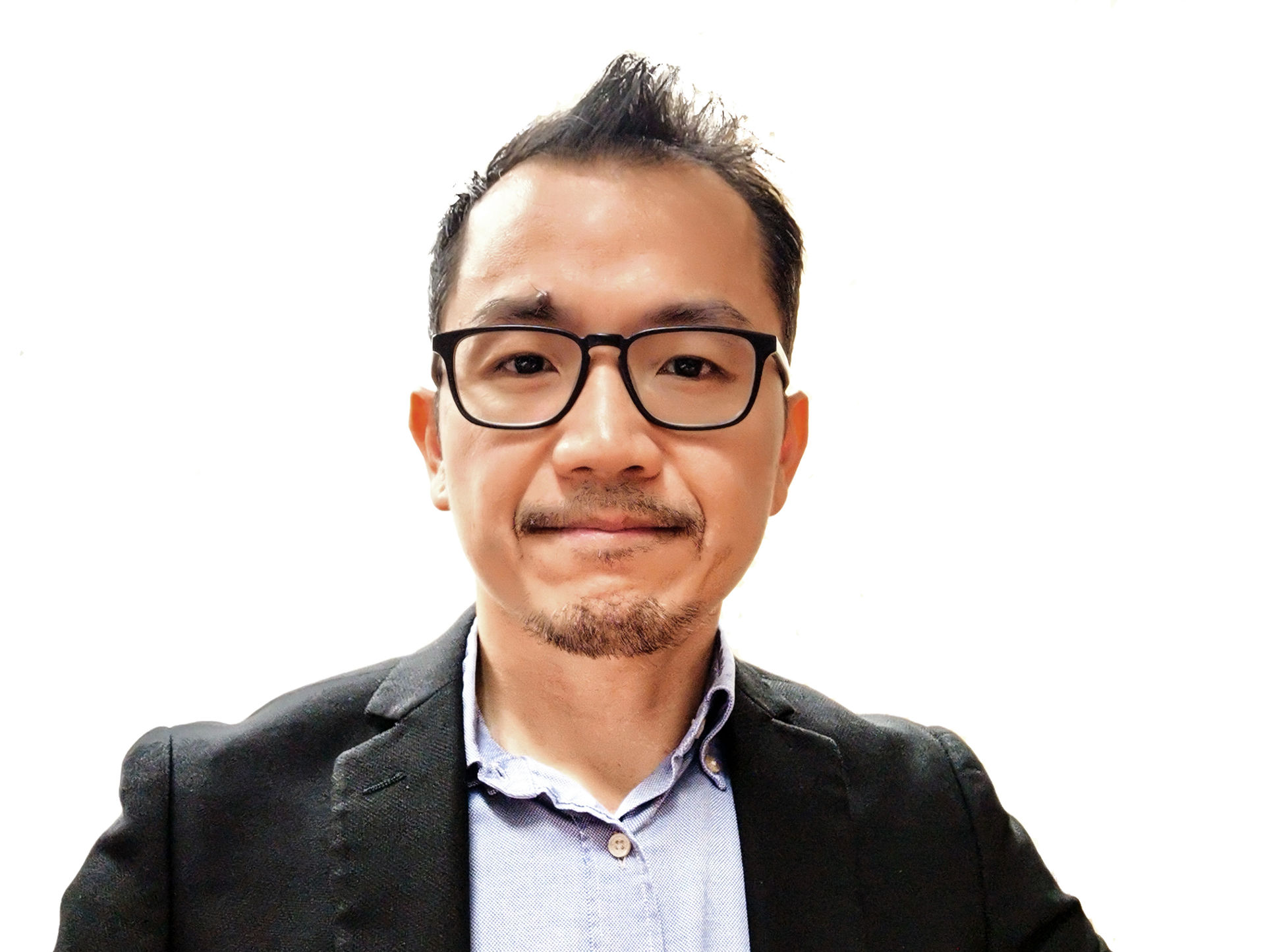
Learning dynamic multimodal networks
Capturing and modeling relationship networks consisting of entity nodes and attributes associated with these nodes is an important research topic in network or graph learning. In this dissertation, we focus on modeling an important class of networks present in many real-world domains. These networks involve i) attributes from multiple modalities, also known as multimodal attributes; ii) multimodal attributes that are not static but time-series information, i.e., dynamic multimodal attributes, and iii) relationships that evolve across time, i.e., dynamic networks. We refer to such networks as dynamic multimodal networks in this dissertation.
An example of a static multimodal network is one that consists of user interface (UI) design objects (e.g., UI element nodes, UI screen nodes, and element image nodes) as nodes, and links between these design objects as edges. For example, the links between UI screen nodes and their constituent UI element nodes are part of the edges between the respective nodes. The design objects may be associated with visual and element images, text, numerical values, and categorical labels as attributes. An example of dynamic company networks with dynamic multimodal attributes may involve relationships between company nodes that evolve across time (i.e., evolving commercial relationships between company nodes), and the company nodes may be associated with time-series of numerical stock prices, textual news, and categorical event attributes.
While there has been significant progress in the area of network or graph learning, most existing works do not focus on modeling such dynamic multimodal networks nor static networks with static or dynamic multimodal attributes.
In the first part of this dissertation, we focus on modeling networks with multimodal attributes. We develop four models that jointly capture static networks comprising different node and/or edge types with static multimodal and positional information. For model interpretability, we propose attention weight-based and learnable edge mask-based methods that enable end-users to understand and interpret the contribution of different parts of the network and information from different modalities. We show that our proposed models consistently out-perform other state-of-the-art models on six datasets across an extensive set of UI prediction tasks.
Next, in the second part of the dissertation, we focus on networks with dynamic multimodal attributes. We propose two models that jointly capture static networks comprising the same or different node types with dynamic attributes, i.e., time-series attributes, from different modalities, e.g., numerical stock price-related and textual news information, which may be local in nature (directly associated with specific nodes), or global in nature (relevant to multiple nodes). To address the noise inherent in multimodal time-series, we also propose knowledge-enrichment and curriculum learning methods. We show that our proposed models out-perform state-of-the-art network learning and time-series models on eight datasets across an extensive set of investment and risk management tasks and applications.
In the third and final part of the dissertation, we focus on modeling dynamic networks with dynamic multimodal attributes. We propose three models that capture dynamic implicit networks and/or dynamic explicit networks. The network nodes may be associated with local or global dynamic multimodal attributes that may be of varying lengths and frequencies. To address noisy and non-stationary dynamic networks and dynamic multimodal attributes, we also propose self-supervised learning and concept learning methods. Aside from applying the proposed models for dynamic networks with dynamic multimodal attributes to investment and risk management tasks and applications on another four datasets, we further apply our proposed models for dynamic networks with dynamic multimodal attributes to environmental, social, and governance rating forecasting tasks on six datasets, and demonstrate that our proposed models out-perform state-of-the-art models on these tasks.
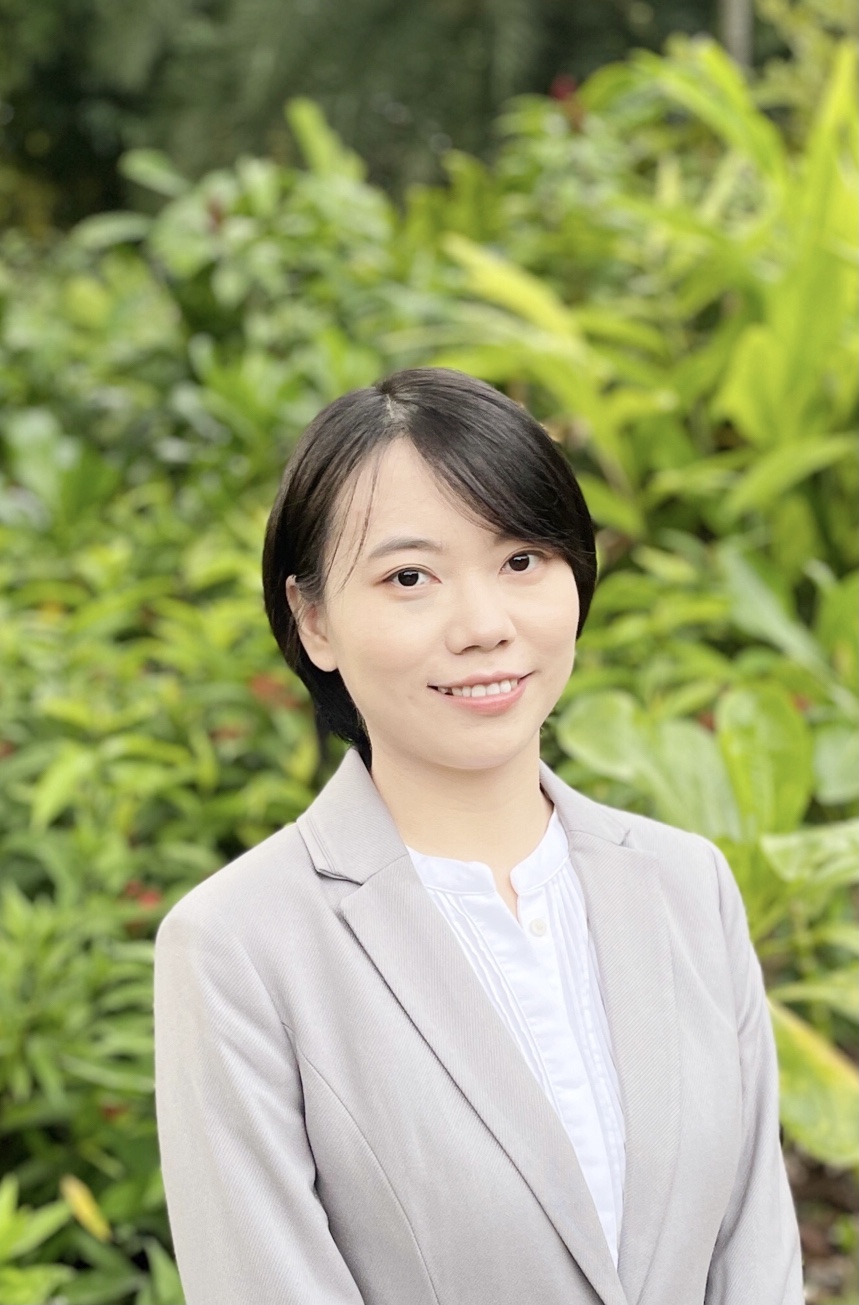
Essays on culture, institutions, and development
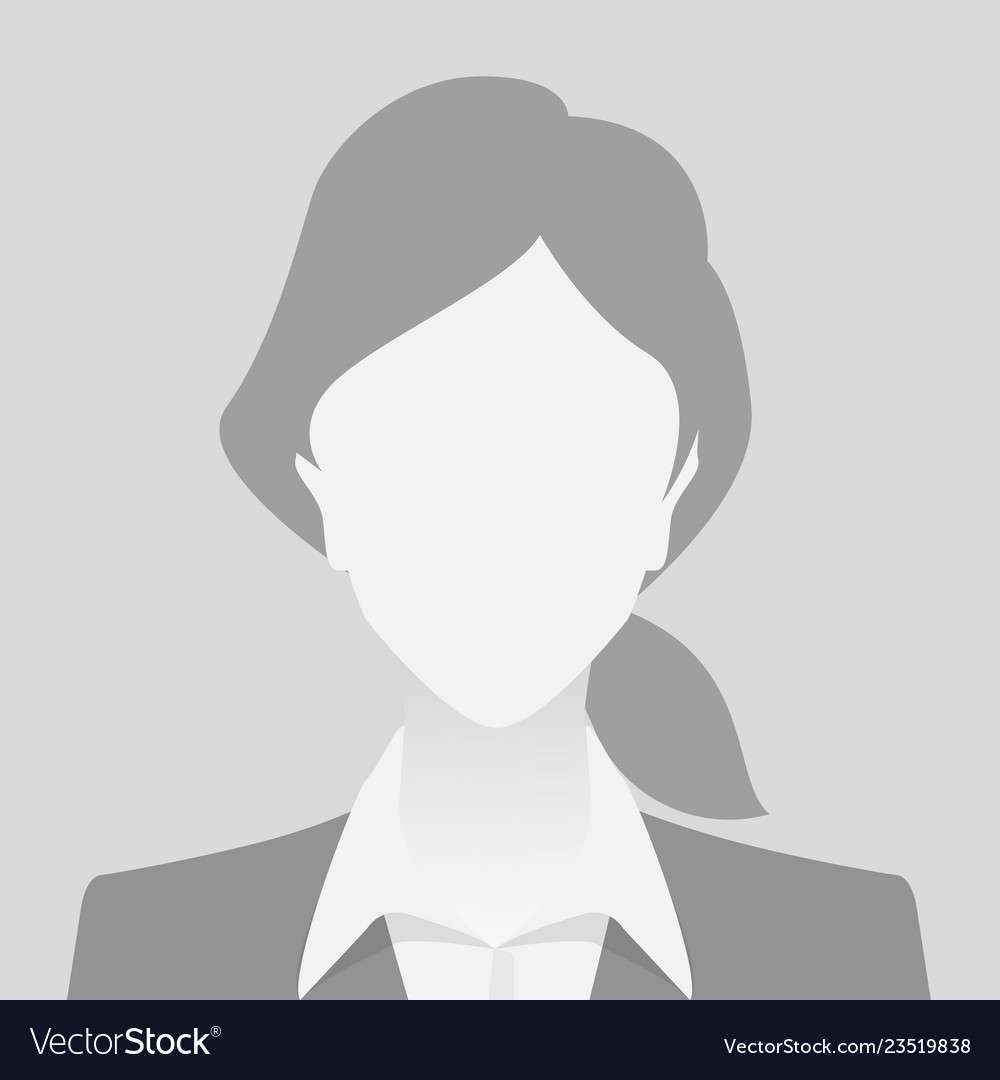
The interactive effects of societal and organizational cultural tightness on employee work related outcomes
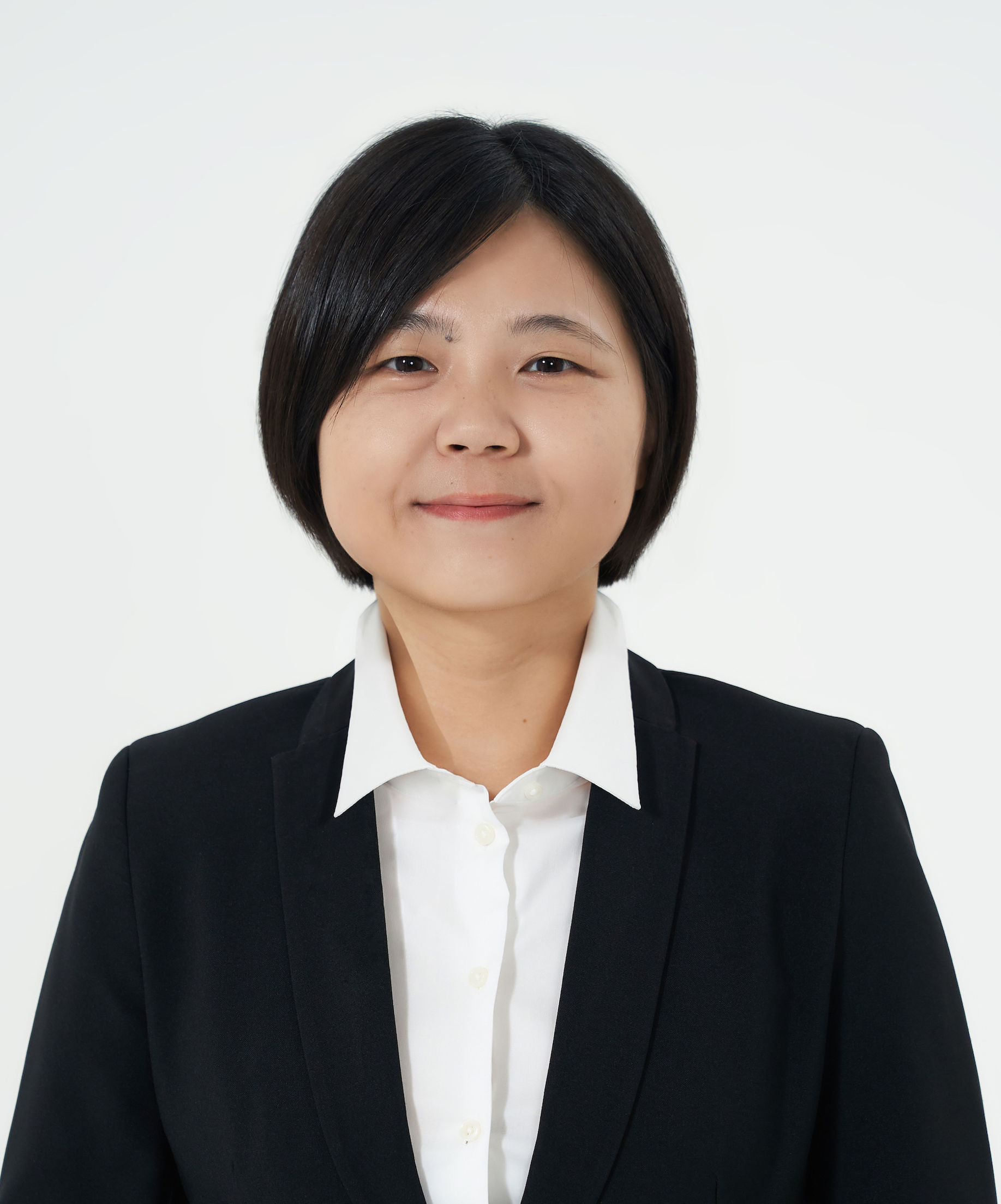
There are many information retrieval tasks that depend on knowledge graphs to return contextually relevant result of the query. We call them Knowledgeenriched Contextual Information Retrieval (KCIR) tasks and these tasks come in many different forms including query-based document retrieval, query answering and others. These KCIR tasks often require the input query to contextualized by additional facts from a knowledge graph, and using the context representation to perform document or knowledge graph retrieval and prediction. In this dissertation, we present a meta-framework that identifies Contextual Representation Learning (CRL) and Contextual Information Retrieval (CIR) to be the two key components in KCIR tasks.
We then address three research tasks related to the two KCIR components. In the first research task, we propose a VAE-based contextual representation learning method using a co-embedding attributed network structure that co-embeds knowledge and query context in the same vector space. The model shows superior downstream prediction accuracy compared to other baseline models using VAE with or without using external knowledge graph.
Next, we address the research task of solving a novel IR problem known as Contextual Path Retrieval (CPR). In this task, a knowledge graph path relevant to a given query and a pair of head and tail entities is to be retrieved from the background knowledge graph. We develop a transformer-based model consisting of context encoder and path encoder to solve the CPR task. Our proposed models which include the proposed two encoders show promising ability to retrieve contextual paths.
Finally, we address the Contextual Path Generation (CPG) task which issimilar to CPR except that the knowledge graph path to be returned may require inferred relation edges since most knowledge graphs are incomplete in their coverage. For the CPG task, we propose both monotonic and non-monotonic approaches to generate contextual paths. Our experiment results demonstrate that the non-monotonic approach yields better-quality resultant knowledge graph paths.
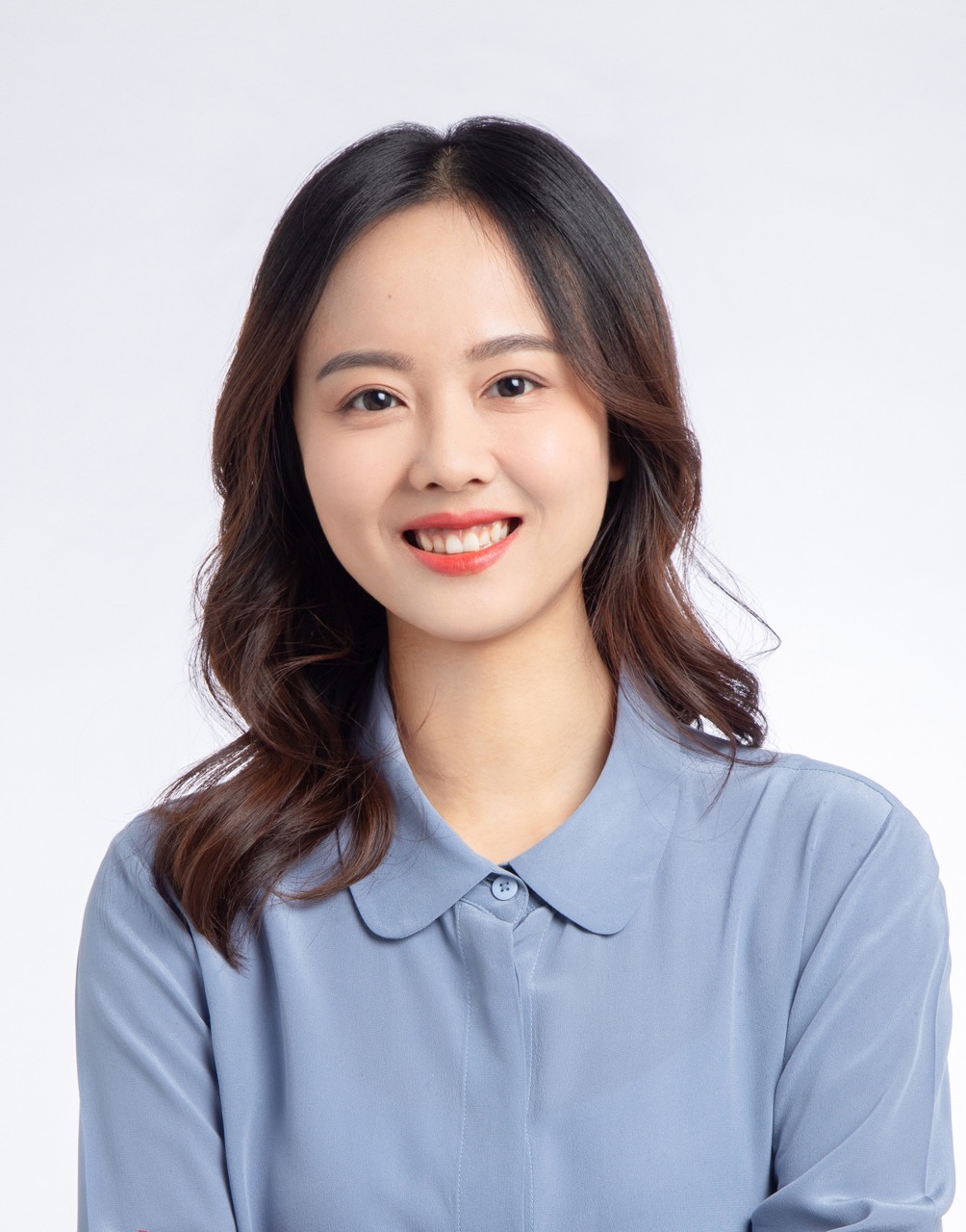
Essays on corporate social (ir)responsiblity, alliance formation and stock market reaction
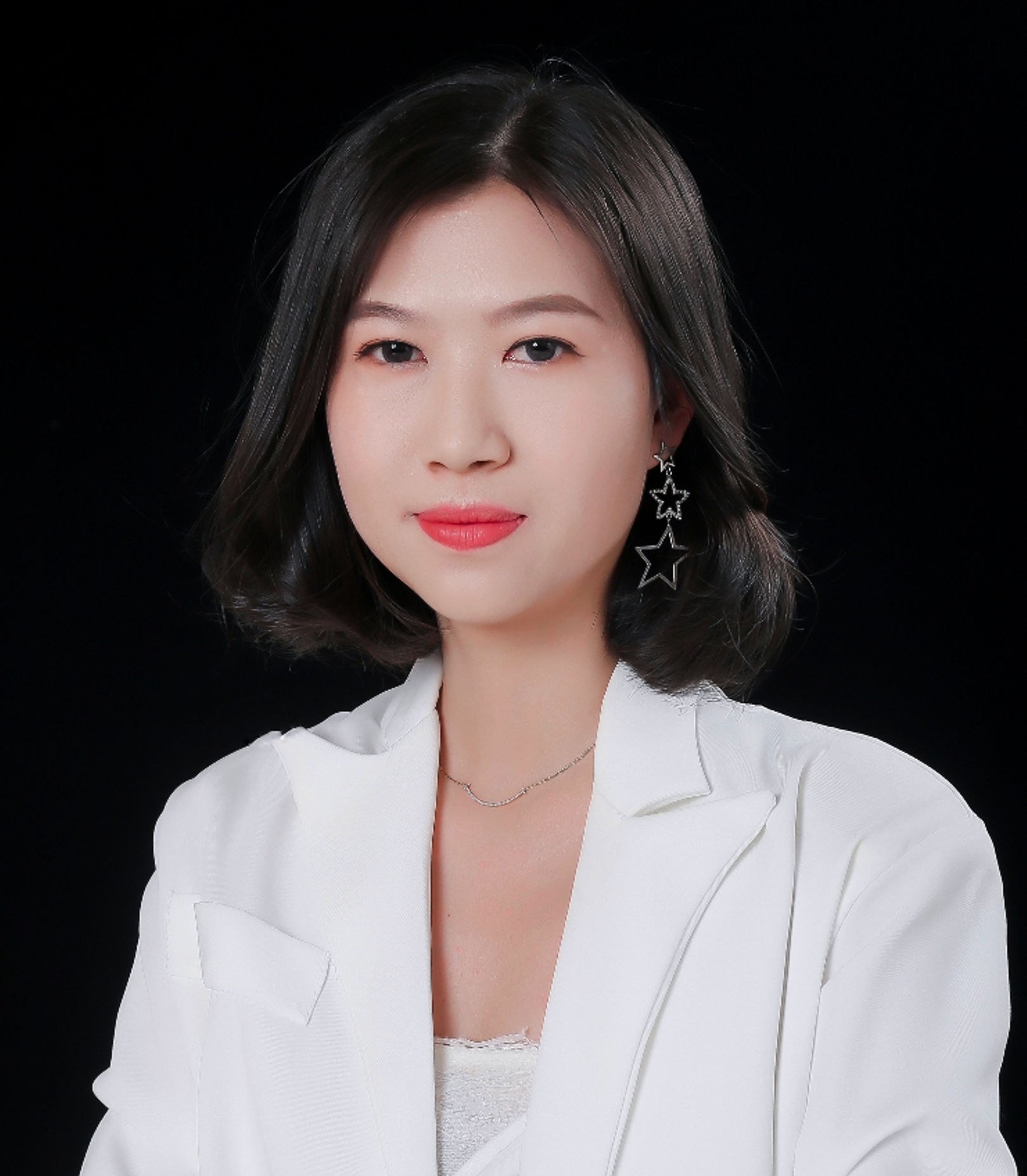
Customers' waiting experiences are crucial in service and retail systems, and this thesis investigates their impact in various contexts. In the service system, long waiting time causes customers' no-show behavior, and negative feedback from existing customers, which in turn results in low conversion and loss of revenue for service providers. However, waiting is not always a negative presence. In the online retail system, with the innovation of the sales model, long waiting can earn more time for online retailers to ease the logistics pressure although it may reduce customers' willingness to pay at the same time. Against this backdrop, in the first essay, we investigate the influence of customers' waiting preference and no-show behavior on appointment systems in the service system. Secondly, this dissertation looks at the pricing incentivization of customers' waiting in online retail systems. Finally, we empirically measure the impact of financial incentives on last-mile operations to reduce customers' expected waiting time for delivery.
In the first essay, we conduct two lab experiments and build models to examine the impact of waiting on customer's appointment selection and no-show behavior in appointment systems. Appointment systems are widely adopted in many service organizations. The simplest and most common format is the Equally-Spaced (ES) system, in which the inter-appointment times between consecutive arrivals are equal. One major drawback of such a system is the long expected waiting time for later arrivals, which makes later appointment positions unappealing to customers. As a result, customers who take these positions are more likely to abandon their appointments, leading to a higher no-show rate. To address this issue, we examine a novel Equal-Waiting (EW) scheduling system under which the expected waiting times are equal across appointments. Through a series of controlled lab experiments, we establish that the EW system increases the attractiveness of later appointments and that customers who are willing to take these appointments are more likely to show up. We then incorporate this individual-level preference and no-show behavior into models to evaluate its impact on the system-level performance. We find that, compared with the traditional ES system, the EW system can significantly increase customers' show-up rate and improve system utilization.
In the second essay, we focus on the pricing incentivization of customers' waiting in a new flash-sale model, which is widely used by many platforms such as JD.com, and Lazada on seasonal promotions like Double 11. In flash sales, customers first pay the deposit and then wait several days to make the final payment. The product will be shipped out after the final payment is made. The deposit determines the discount strength that the customer can enjoy due to the Double Deposit Inflation and provides a signal to the retailer on potential demands, allowing the retailer to reduce the logistical cost incurred from bottlenecked demand surges. The waiting occurring during the transaction process may reduce customers' willingness to pay. However, it can earn more time for the online retailer to ease the logistics pressure so that the logistics cost can be further reduced. Considering these important features in flash sales, we propose a pricing optimization model and jointly decide the optimal deposit and the product’s full price. We identify the value of introducing the flash-sale channel for the retailer and the conditions under which the value can be realized. We also provide the optimal flash-sale duration. In addition, our findings indicate the importance of considering the production cost in the optimal pricing strategy, especially for the linear demand function. In the case study, we calibrated our model with real data from an e-commerce company in China, and the results from a 5-fold cross-validation show that our model can predict demand well. Besides, by applying the pricing strategy proposed in this paper, we can dramatically improve the profit.
The third essay delves into the impact of financial incentives on last-mile operations. Riders' responsiveness is crucial for service quality in last-mile delivery. To address the frequently-occurred low responsiveness due to driver shortage or order congestion, most delivery platforms adopt financial incentives to attract more drivers. However, empirical research on the effectiveness of financial incentives and their spillover effects is lacking. Thus, the third essay examines the impact of financial incentives on last-mile operations, using transactional datasets obtained from a crowdsourced delivery platform.
Specifically, we employ a regression discontinuity design to identify the causal influence of financial incentives on drivers' order acceptance speed. Our results show that financial incentives significantly reduce the driver's order acceptance duration by 16.6%. Furthermore, temporal effects suggest the platforms can strategically terminate financial incentives ahead of schedule, as the impact will persist for a certain period of time. From a network perspective, we also examine the spillover impact of the neighboring stores' financial incentives on the performance of the focal store. Interestingly, our findings reveal opposing impacts that depend on the focal store's status. Specifically, the nearest store's financial incentives cause longer driver's order acceptance duration at the focal store without financial incentives; however, the opposite spillover effect is observed when the focal store also offers financial incentives. To better understand the underlying mechanisms, we identify the siphon effect and clustering effect as the key drivers of this phenomenon. This study contributes both theoretical and practical implications to the field of last-mile delivery.
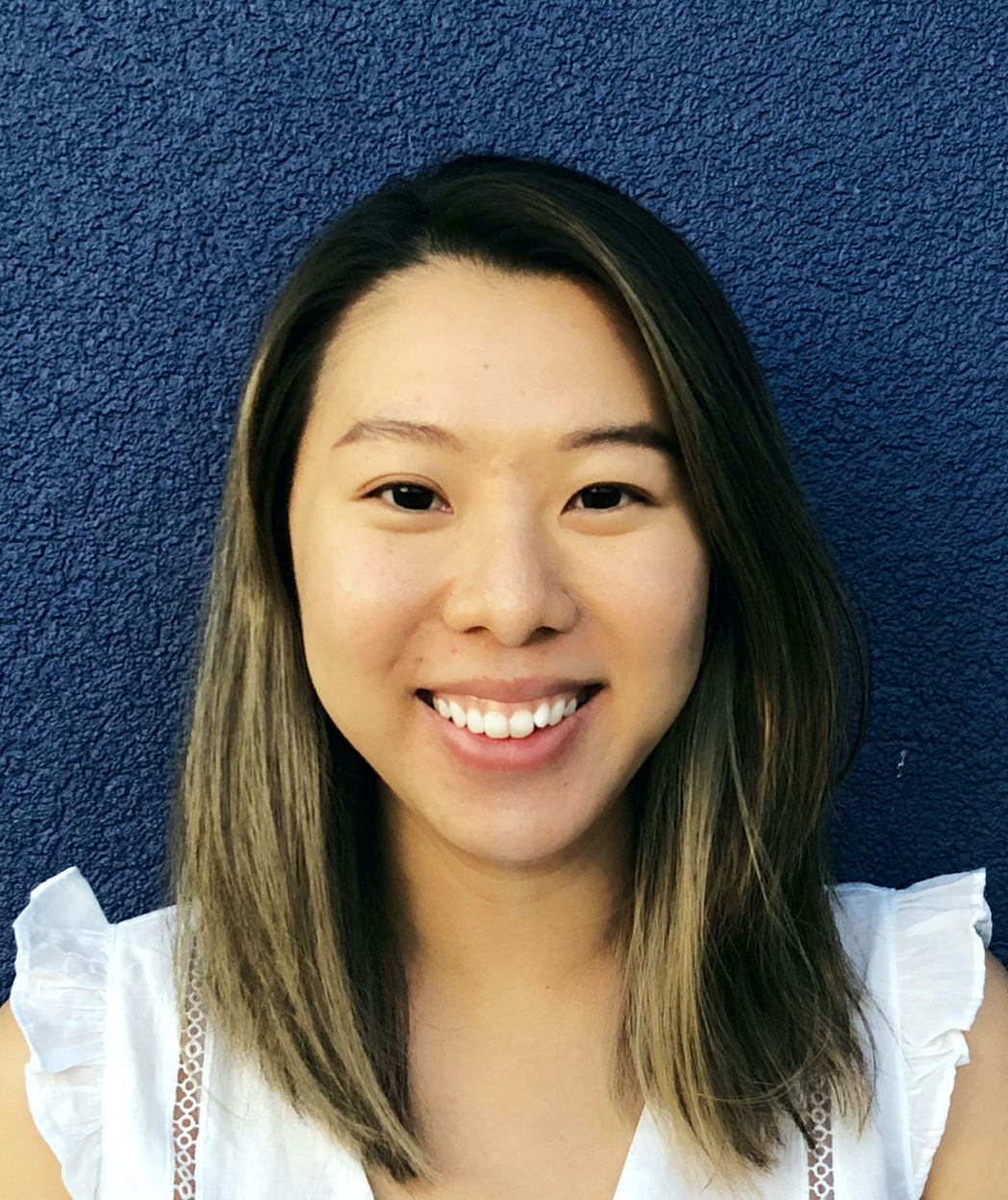
Existing research on multiracials has examined how multiracials develop different racial identities. However, empirical research on how multiracial manage and integrate their identities as well as its impact are limited. This dissertation examined key antecedents and consequences associated with the unique process that multiracials undergo to achieve a positive identity via Multiracial Identity Integration (MII). In Study 1, we examined the link between MII, psychological well-being, and cognitive capacity. Results revealed a positive association between MII and psychological well-being as well as some cognitive capacity outcomes. Study 2 replicated the same relationship between MII and psychological well-being/cognitive capacity outcomes. Additionally, multiracials’ experiences with identity denial and identity inquiry were negatively associated with multiracials’ MII. The relationship between identity denial and psychological well-being/cognitive capacity outcomes were mediated by MII. Studies 3 and 4 examined if MII would moderate the interpretation of identity-related questions and if manipulated experiences of identity denial and identity inquiry would impact multiracials’ MII respectively. The findings from both studies were nonsignificant. Together, this dissertation illuminated the antecedents and consequences associated with a healthy multiracial identity via MII. Theoretical and practical implications are discussed.
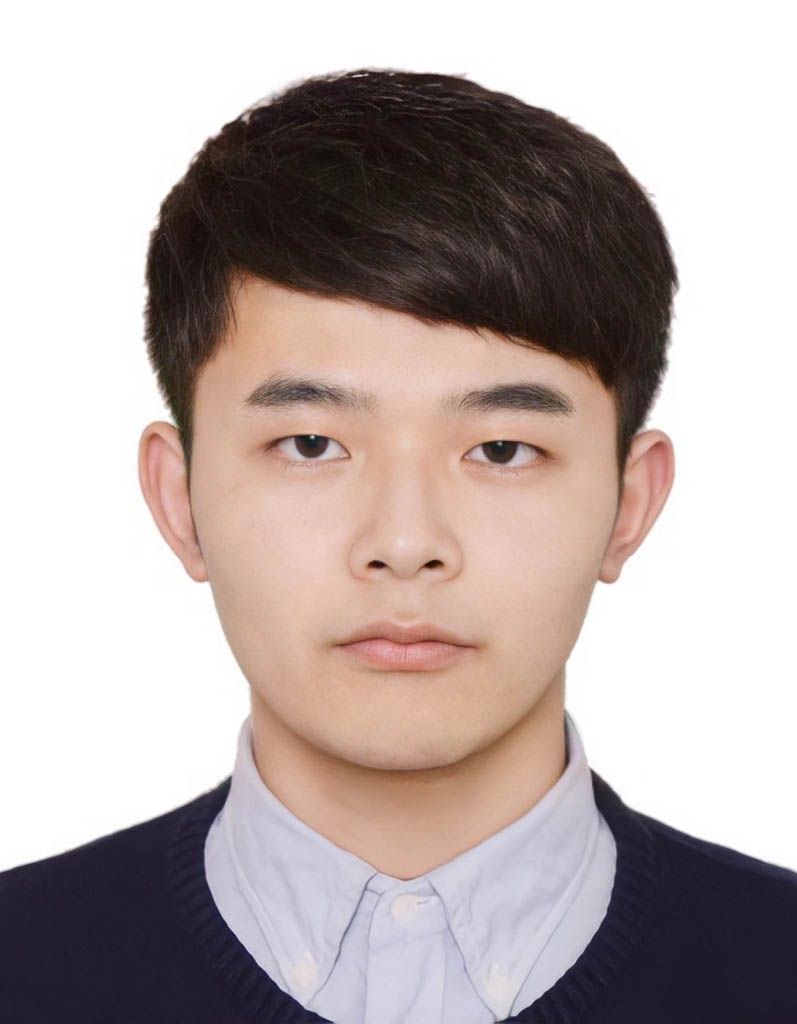
Most traditional machine learning or deep learning methods are based on the premise that training data and test data are independent and identical distributed, i.e., IID. However, it is just an ideal situation. In real-world applications, test set and training data often follow different distributions, which we refer to as the out of distribution, i.e., OOD, setting. As a result, models trained with traditional methods always suffer from an undesirable performance drop on the OOD test set. It's necessary to develop techniques to solve this problem for real applications. In this dissertation, we present four pieces of works in the direction of OOD in Natural Language Processing (NLP) which can be further grouped into two sub-categories: adversarial robustness and cross-lingual transfer.
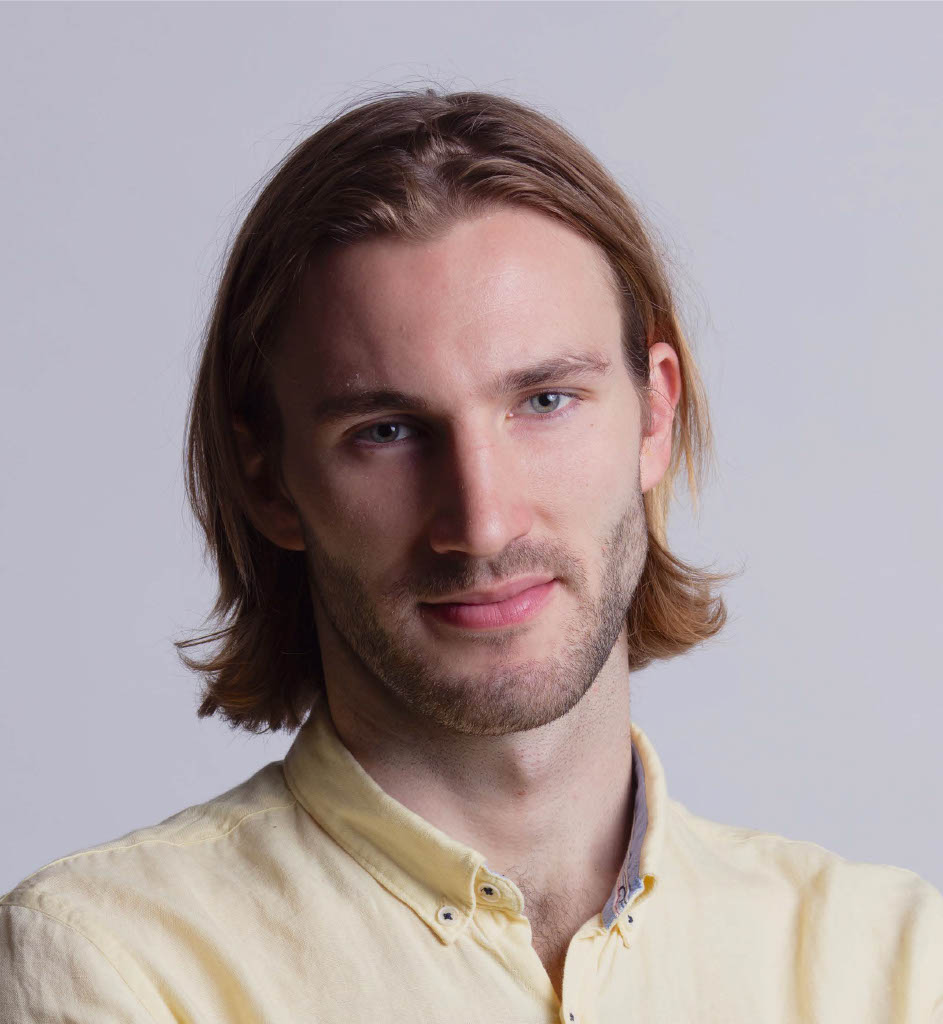
Social Attention in Realistic Work Environments
Social attention – the process by which individuals select which aspect of the social world to mentally process – is a key antecedent to all organisational behaviour in groups. This central role of attention has long been appreciated by organisational theorists, but our understanding of this core cognitive process has been hampered by a lack of empirical evidence. To create a method through which organisational scholars can study social attention, this dissertation combines cognitive science measures of attention with recent innovations from social and applied psychology using virtual reality to study naturalistic social behaviour (Chapter 1). This method is then applied to investigate the factors that determine whether individuals can capture the attention of their audience at work – e.g., charismatic job candidates receiving more attention than non-charismatic job candidates – and the downstream effects this has on individual-level outcomes (Chapter 2). These biases in social attention are then incorporated into models of group decision-making to demonstrate how micro-level attentional biases in group decision-making scenarios can translate into macro-level decision biases and thus sub-optimal decision outcomes (Chapter 3). The dissertation concludes with an inductive theory of “Socially Bounded Rationality” that hopes to spur future research on this topic.
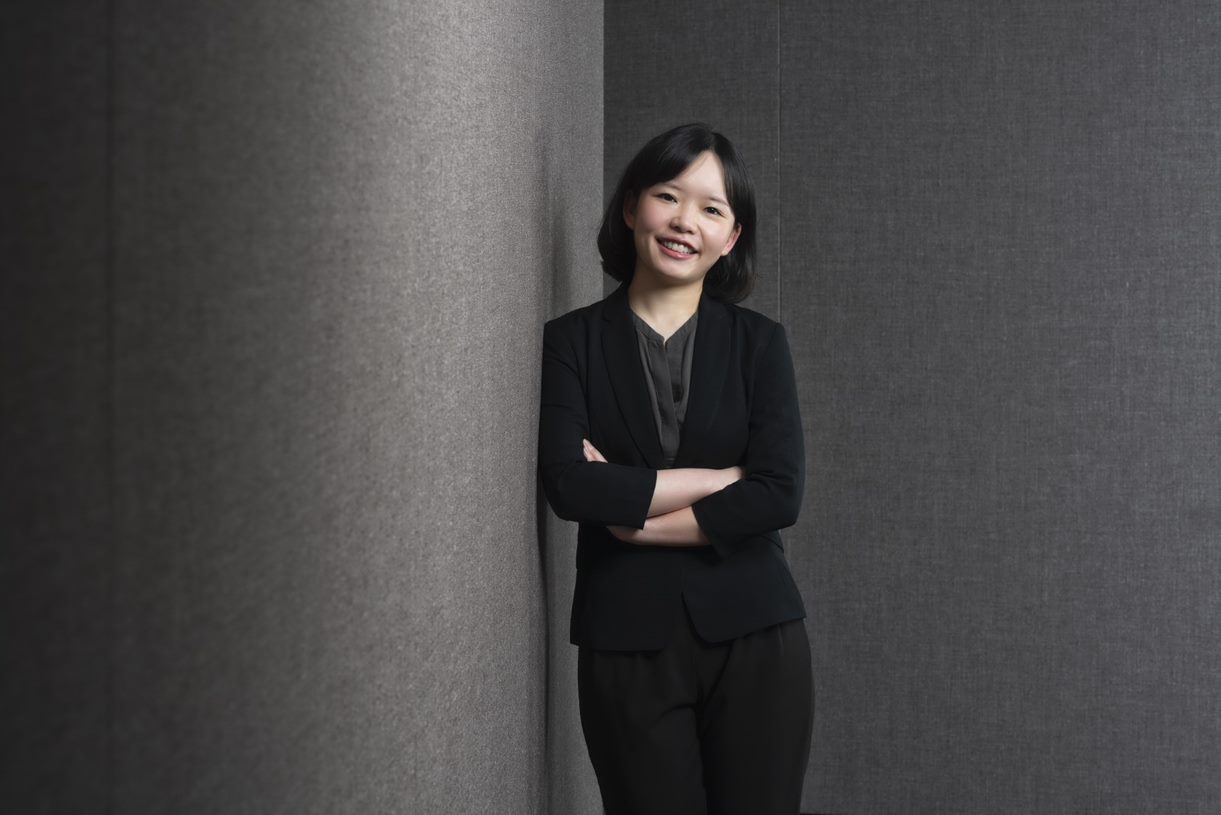
Regulating by new technology: The impacts of the SEC data analytics on the SEC investigations
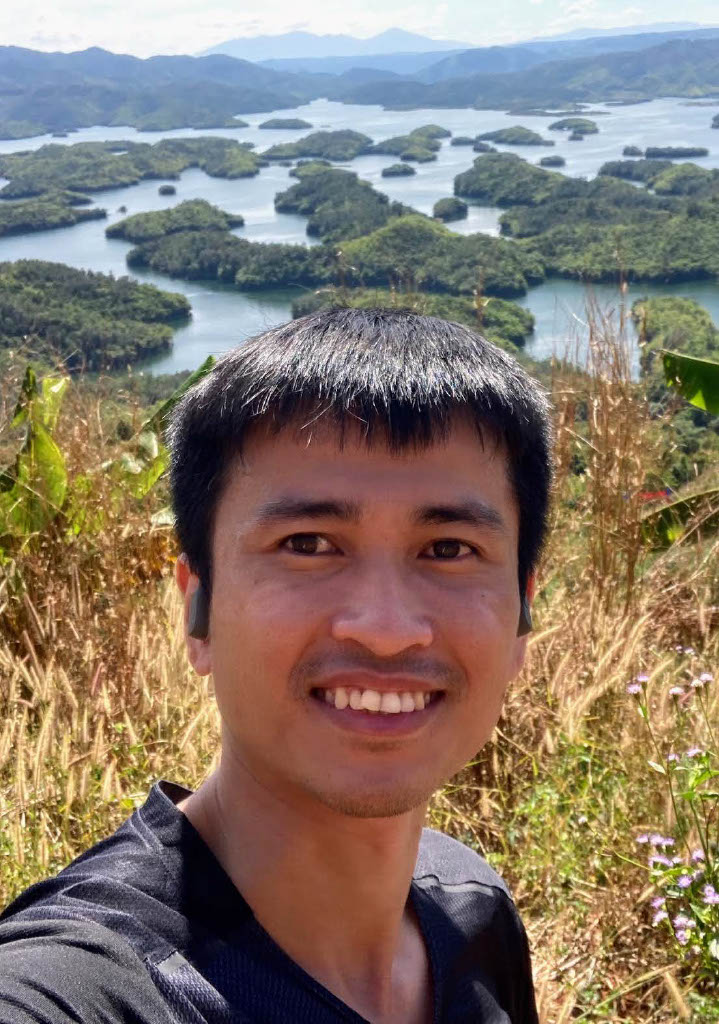
Recommendation explanations help to make sense of recommendations, increasing the likelihood of adoption. Here, we are interested in mining product textual data, an unstructured data type, coming from manufacturers, sellers, or consumers, appearing in many places including title, summary, description, review, question and answers, etc., can be a rich source of information to explain the recommendation. As the explanation task could be decoupled from that of recommendation objective, we can categorize recommendation explanation into integrated approach, that uses a single interpretable model to produce both recommendation and explanation, or pipeline approach, that uses a post-hoc explanation model to produce explanation for recommendation from a black-box or an explainable recommendation model. In addition, we can also view the recommendation explanation as evaluative, assessing the quality of a single product, or comparative, comparing the quality of a product to another product or to multiple products. In this dissertation, we present research works on both integrated and pipeline approaches for recommendation explanations as well as both evaluative and comparative recommendation explanations.
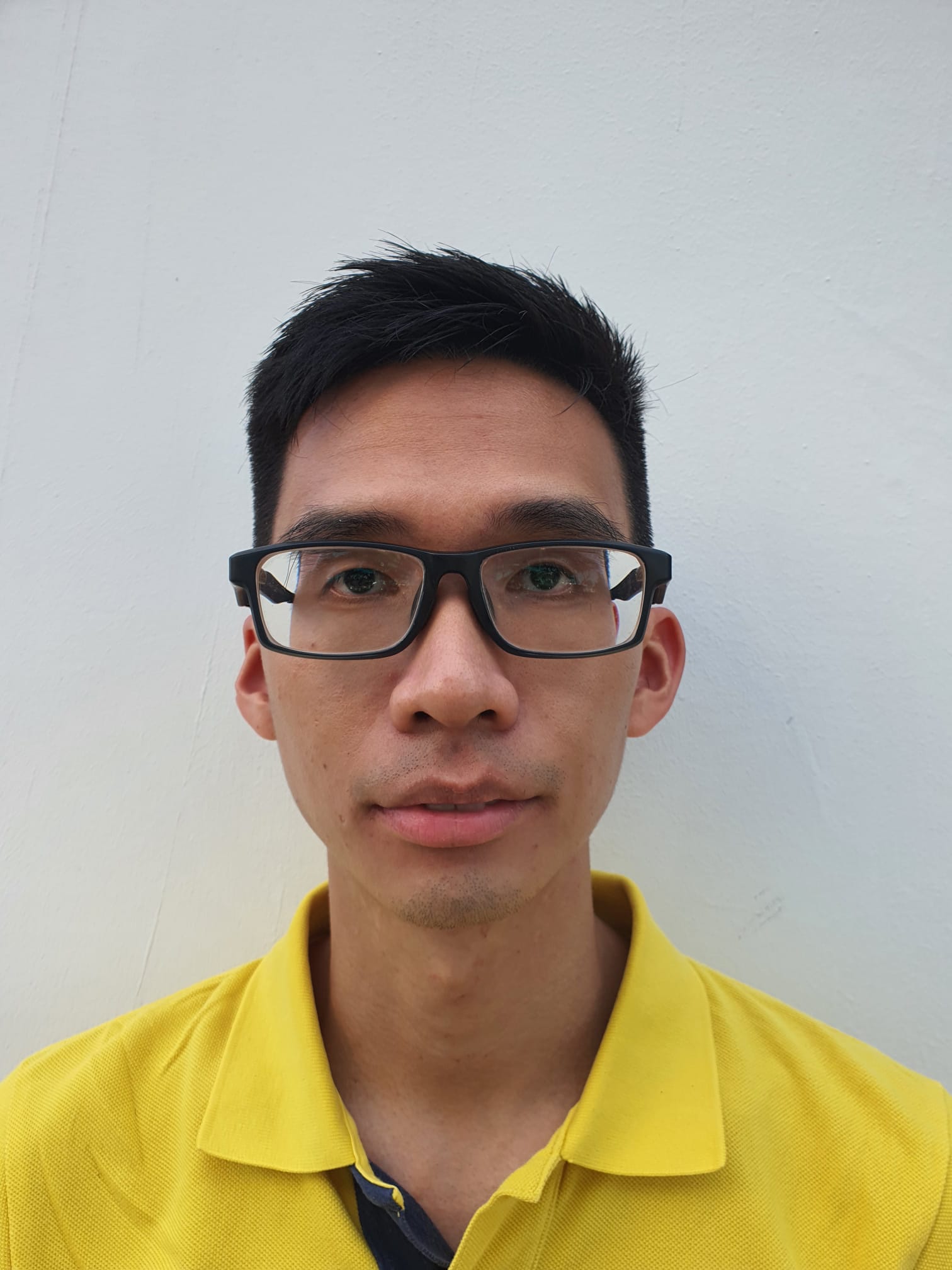
Reinforcement Learning Approach to Coordinate Real-World Multi-Agent Dynamic Routing and Scheduling
In this dissertation, we study new variants of routing and scheduling problems motivated by real-world problems from the urban logistics and law enforcement domains. In particular, we focus on two key aspects: dynamic and multi-agent. While routing problems such as the Vehicle Routing Problem (VRP) is well-studied in the Operations Research (OR) community, we know that in real-world route planning today, initially-planned route plans and schedules may be disrupted by dynamically-occurring events. In addition, routing and scheduling plans cannot be done in silos due to the presence of other agents which may be independent and self-interested.
This dissertation discusses and proposes new methodologies that incorporate relevant techniques from the field of AI (Reinforcement Learning (RL) and Multi-Agent System (MAS) more precisely) to supplement and complement classical OR techniques to solve dynamic and multi-agent variants of routing and scheduling problems. This dissertation makes three main contributions. Firstly, to address dynamic aspect of routing and scheduling problem, we propose an RL-based approach that combines Value Function Approximation (VFA) and planning heuristic to learn assignment/dispatch and rerouting/rescheduling policies jointly without the need to decompose the problem or action into multiple stages. Secondly, to address multi-agent aspect of routing and scheduling problem, we formulate the problem as strategic game and propose a scalable, decentralized, coordinated planning approach based on iterative best response. Lastly, to address both dynamic and multi-agent aspects of the problem, we present a pioneering effort on a cooperative Multi-Agent RL (MARL) approach to solve multi-agent dynamic routing and scheduling problem directly without any decomposition step. This contribution builds upon our two earlier contributions by extending the proposed VFA method to address multi-agent setting and incorporating the iterative best response procedure as a decentralized optimization heuristic and an explicit coordination mechanism.
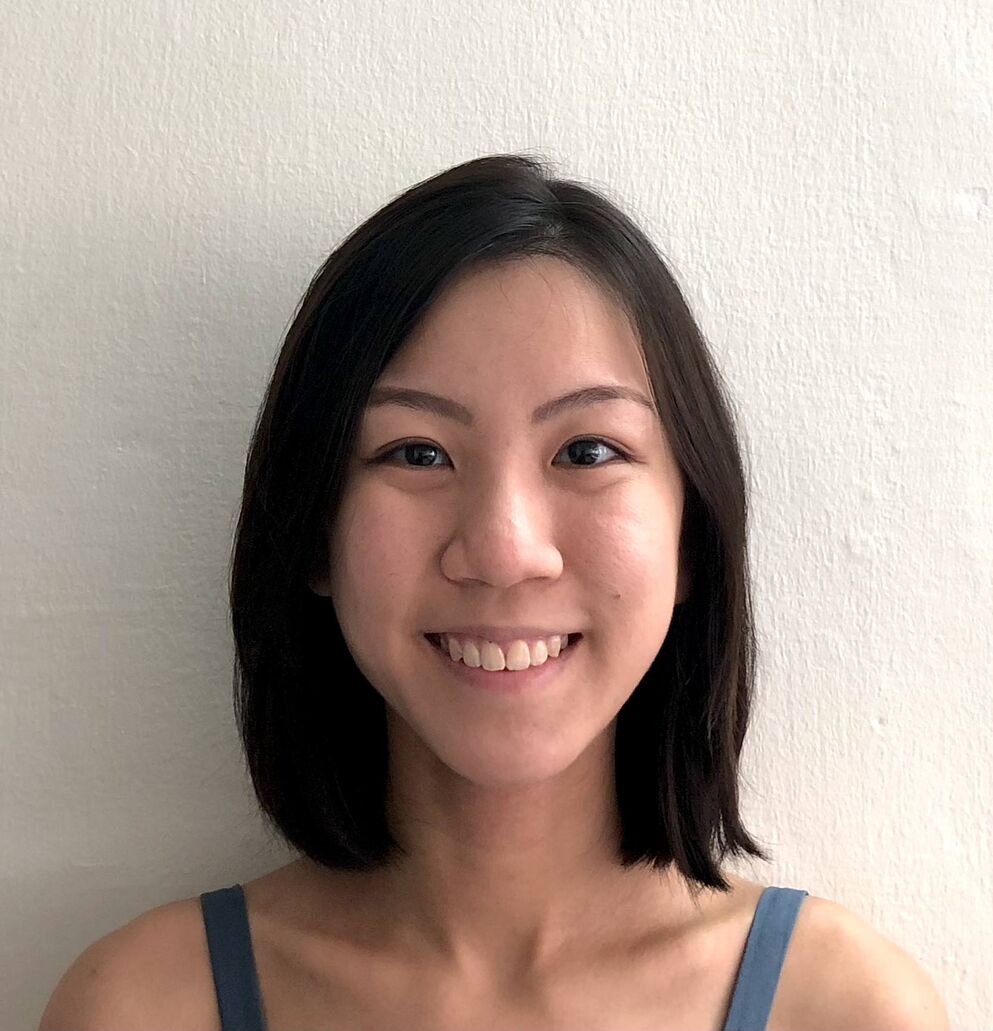
Despite previous research demonstrating the negative effects of IADL limitations on depressive symptoms in older adults, there is a dearth of research examining the underlying mechanism of this relationship. Drawing on the stress process model, the present research investigated whether purpose in life and resilience, as psychological resources, would mediate the relation between IADL limitations and depressive symptoms. We recruited 111 community-dwelling older adults (ages 54-85) and examined a parallel mediation model using the PROCESS macro. Our results revealed that purpose in life and resilience fully mediated the relation between IADL limitations and depressive symptoms. These mediation effects held true when we adjusted for notable covariates. Our findings underscore the crucial roles of purpose in life and resilience in explaining the relation between IADL limitations and depressive symptoms in older adults. Implications and opportunities for intervention programs to bolster purpose in life and resilience are discussed.
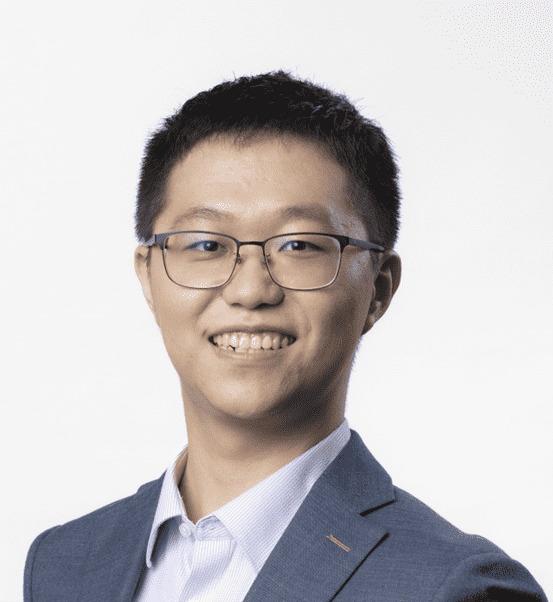
M&A advisor banks are privy to valuable and sensitive information through their service. I examine whether M&A advisor banks exploit such private information to trade in peers of M&A firms. I provide evidence that M&A advisor banks gain higher profits through their trading in peers of M&A firms, compared with non-advisor banks. Such informed trading is more intensive for M&A deals with larger impacts on peer firms (i.e., when the deal value is more significant for peer firms; when the M&A firms have larger market share in the industry; and when the stock price reactions of peer firms are stronger). Further analysis reveals that prior business relationships with peer firms enable M&A advisor banks to engage in such informed trading. In addition, M&A advisor banks’ performance pressure incentivizes them to utilize private M&A information for trading, while reputation concern deters such informed trading in peer firms.