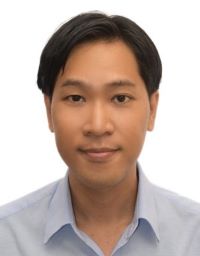
Flexible Moral Behavior in the Workplace
In my dissertation, I systematically examine what it means to be morally flexible. I develop a scale to capture an individual’s willingness to adapt their moral behavior and examine both positive and negative consequences of this type of moral flexibility in the workplace. My dissertation consists of three studies. In Chapter 2, I draw from the personality strength literature and research on within-person variability in moral behavior to introduce the construct of moral adaptability (MA) defined as the willing to adjust moral behavior depending on the situation. I argue MA functions in a similar manner to personality strength (but in the moral domain), such that when individuals are low in MA, they are most likely to express their moral values, while being high in MA makes individuals much more susceptible to situational influences and less likely to express their moral preferences. I develop and validate a scale assessing the construct and demonstrate in six independent samples (four samples of undergraduate students at a university in Singapore and two samples of working adults in the United States) that the scale shows good psychometric properties. I also develop initial empirical evidence for how the scale functions and in two independent samples illustrate how low MA strengthens the positive relationship between moral character (i.e., internalization dimension of moral identity, Honesty-Humility) and moral behavior (i.e., charitable behavior) and explains both positive and negative employee outcomes (i.e., constructive deviance, unethical pro-organizational behavior).
Building from these results, in Chapter 3, I draw from feelings-as-information theory and propose that individuals high in MA use situations to justify their past unethical behavior and therefore experience less guilt and shame. An experience-sample study with a sample of 55 undergraduate students in Singapore shows that respondents high in MA experienced lower guilt and shame in the aftermath of their unethical behavior as compared to those low in MA and that MA explained the felt emotions above and beyond a wide array of other traditional moral constructs.
In Chapter 4, I integrate the concept of ethical leadership to examine the implications of MA for workplace interpersonal relationships and leader influence on subordinates. Drawing from research on role modeling and moral self-threat, I hypothesize that subordinates who perceive ethics to be highly relevant to them and work under an ethical leader with low MA are more likely to experience higher self-threat due to the leader and become less likely to perceive their ethical leader as a role model. A two-wave sample of 486 subordinate-supervisor dyads in organizations in India provides partial support that subordinates who perceive ethics to be highly relevant and work under an ethical leader with low MA experience higher self-threat due to the leader.
In each chapter, I discuss the contributions of this dissertation to the organizational ethics literature, practical implications for managers in organizations, limitations, and directions for future research.
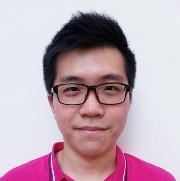
In this dissertation, we address the subject of modeling and simulation of agents and their movement decision in a network environment. We emphasize the development of high quality agent-based simulation models as a prerequisite before utilization of the model as an evaluation tool for various recommender systems and policies. To achieve this, we propose a methodological framework for development of agent-based models, combining approaches such as discrete choice models and data-driven modeling.
The discrete choice model is widely used in the field of transportation, with a distinct utility function (e.g., demand or revenue-driven). Through discrete choice models, the movement decision of agents are dependent on a utility function, where every agent chooses a travel option (e.g., travel to a link) out of a finite set. In our work, not only do we demonstrate the effectiveness of this model in the field of transportation with a multiagent simulation model and a tiered decision model, we demonstrate our approach in other domains (i.e., leisure and migration). where the utility function might not be as clear, or involve various qualitative variables.
The contribution of this dissertation is therefore two-fold. We first propose a methodological framework for development of agent-based models under the conditions of varying data observability and network model scale. Thereafter, we demonstrate the applicability of the proposed framework through the use of three case studies, each representing a different problem domain.
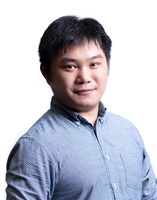
Information Diffusion and Market Friction
How markets impound information into asset prices is one of the most important concerns of financial economics. Due to behavioural bias and transaction friction, information could be mispriced in the real world, thus driving market anomalies and return predictability of behavioural factors. My dissertation contributes to the literature by investigating how information can be quantified, acquired, disseminated and priced in the financial market with the existence of market frictions.
In Chapter 2, we propose an efficient method based on machine learning and textual analysis to quantify cross industry news and shed light on how news travels across different industries. The results show that cross-industry news contains valuable information about firm fundamentals that is not fully captured by firms’ own news or within-industry peers’ news. Stock prices do not promptly incorporate cross-industry news, generating return predictability. Moreover, underreaction to cross-industry news is more pronounced among smaller stocks that are more illiquid, more volatile, and have fewer analysts following. A long–short strategy exploiting cross-industry news yields annual alphas of over 10%.
In Chapter 3, we construct a novel measure of market wide investor attention by applying a social network analysis to aggregate the attention spillover effects among stocks that are co-mentioned by media news. Empirically, we find that the News Network Triggered Attention index (NNTA), negatively predicts market returns with a monthly in-sample (out-of-sample) R-square of 5.97% (5.80%). In the cross-section, a long-short portfolio based on a news co-occurrence generates a significant monthly alpha of 68 basis points. We further validate the attention spillover effect by showing that news co-mentioning significantly increases Google and Bloomberg search volumes than that of unconditional news coverage. The results hence suggest that attention spillover in a news-based network can lead to significant stock market overvaluations, especially when arbitrage is limited.
Besides behavioural bias, security analysts seem to also contribute to the market friction by issuing biased recommendations. In Chapter 4, we find that the biased recommendations of analysts could be a source of market friction that impede the efficient correction of mispricing. In particular, analysts tend to make more favourable recommendations to overvalued stocks, which have particularly negative abnormal returns ex-post. While analysts whose recommendations are better aligned with anomaly signals are more skilled and elicit stronger recommendation announcement returns.
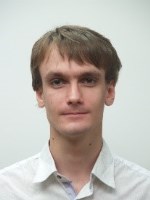
Comparison Mining from Text
Online product reviews are important factors of consumers' purchase decisions. They invade more and more spheres of our life, we have reviews on books, electronics, groceries, entertainments, restaurants, travel experiences, etc. More than 90 percent of consumers read online reviews before they purchase products as reported by various consumers surveys. This observation suggests that product review information enhances consumer experience and helps them to make better-informed purchase decisions. There is an enormous amount of online reviews posted on e-commerce platforms, such as Amazon, Apple, Yelp, TripAdvisor. They vary in information and may be written with different experiences and preferences.
If online opinions are indeed important in many spheres of our lives, then their systematic analysis is a real-life problem. Due to an enormous amount of opinions scattered across the Web, a handcrafted analysis seems to carry an inadmissible cost of time and efforts. An alternative to consider is an automated or, more appropriately, semi-automated analysis conducted by computers as an assistance to human analysts. Text processing applications have received much attention in the past three decades and have been shown successful for language understanding.
Comparison mining aims at understanding opinion mining problems when multiple entities are present simultaneously. This includes, but not limited to deriving similarities and differences between entities and discovering information about the entity relations. The entities may be products, individuals, issues, etc. The notion of comparison tangles in in a form of joint evaluative statements, such as "I think A is better than B", "I think A is a good alternative to B", and introduces new research questions, similar and yet different from traditional opinion mining. How do we find these statements in a review? How do we interpret these statements? How do we make sense of thousands of such comparisons? In this study, we seek to answer these questions and propose a set of related computational solutions.
First, we investigate a comparison identification problem and cast it as a relation extraction problem. Within the relation extraction setup, we develop a new approach for identifying comparative relations. The formal investigation of the syntactic structure of comparative statements leads us to a kernel-based approach, which relies on the dependency structure of sentences. The proposed method shows state-of-the-art results for the comparison identification problem.
Second, we explore intrinsic properties of a comparative corpus to derive a joint model for comparison interpretation and aggregation. At the level of comparisons, the model seeks to derive the comparison outcome of a statement, i.e., which entity is preferred by the writer. At the aggregated level, it seeks to understand the overall ranking of the entities in a corpus of comparisons. The proposed model is shown to be superior to the approaches that tackle each level separately. An empirical evaluation demonstrates its effectiveness on real-world datasets.
Third, we look at the phenomenon of comparison disagreement, i.e., different users may have different preferences over the same set of entities. To capture this diversity, we propose a model for preference clustering and demonstrate its effectiveness and utility.
Fourth, we propose a method for explaining entity comparisons, when entities are identified by their textual representations. CompareLDA, a supervised topic model, is employed to align topics, distributions of co-occurring words, with comparisons, so that the topics are indicative of the "better" and "worse" entities. Through an empirical evaluation, we show that the proposed model is more effective for capturing comparisons than alternative supervised topic models.
All the proposed methods form substantial contribution within the comparison mining research and facilitate a better understanding of the opinion language.
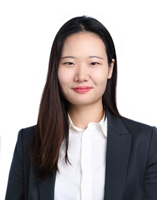
Innovation and creativity are the engines of social and economic progress. What roles do women play in innovation? Emerging evidence reveals that fewer women than men enter and succeed in innovation-related fields. Tackling gender inequality at work has always been one of the grand societal challenges, however little is known about gender issues specific to innovation achievements. This dissertation attempts to explain gender gaps in the innovation and creativity context. Innovation typically involves generating multiple novel and useful ideas, selecting the most promising one for implementation, and persistently championing the idea through implementation. I theorize and unpack the gender effect situated in different stages of innovation, specifically in idea selection and idea championing.
I propose that although women are equally capable as men in generating highly novel ideas, there is greater “novelty avoidance” in women than men - the extent to which individuals refrain from pursuing the most novel ideas they have generated. In a series of studies designed to feature the innovation process (Studies 1-3), I showed the differential influence of gender on idea generation and idea selection. Furthermore, I tested three alternative explanations to the gender difference in novelty avoidance tendency, namely, risk aversion, interdependent self-construal, and fear of social backlash associated with novelty (Study 2). Results suggest that fear of social backlash associated with novelty explains the gendered novelty avoiding/seeking tendencies. I also proposed and showed that the gender difference in novelty avoidance tendency was alleviated when women were told that their innovation will be judged by other women (Study 3).
For idea championing (Studies 4-6), I theorize that women employees are less likely than their men colleagues to engage in autonomous idea championing - bypassing norms, rules, and established procedures to promote creative ideas. Drawing on the “creative prototype model”, I further theorized and showed that the more men employees autonomously champion their creative ideas, the more their supervisors perceived them as creative. In contrast, when women employees engaged in autonomous championing behaviors, they faced backlashes, especially from their women supervisors.
I conclude by discussing the implications of these findings and future research to help advance current understanding of the challenges and opportunities that surround women innovators.
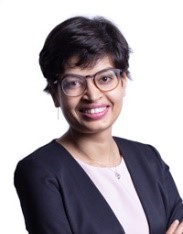
Being born into a poorer family is associated with lower socioeconomic attainment even when people are provided with identical educational and job opportunities, a pattern known as the “class ceiling.” The class ceiling is generated within organizations, but specific reasons causing this effect are not well understood. I propose that one important explanation why employees from poorer families do not fare as well as their more fortunate co-workers concerns differences in families themselves. I integrate research from sociology and psychology explaining challenges faced by families with scarce resources with organizational research on specific pathways through which families can interfere with work activities of employees. This theoretical integration suggests that higher family demands (in terms of time and values) and lower family resources (instrumental support and behavioral scripts) among workers from poorer backgrounds cause a negative influence on employee personal resources, and thus act as a mechanism of disadvantage reproduction after workers join the organization. A large field study of early-career employees who managed to obtain a higher education and secure high-potential jobs conducted in Singapore provides support for the model. I propose and test both institutional as well as individual solutions to the problem. I show that higher organizational support can compensate for lower family resources, but I also find that, at present, most organizations fail to provide such support. Second, I develop and test a psychological intervention that helps workers from poorer backgrounds cope more effectively with higher family demands. A two-week field experiment utilizing a dairy study design provides evidence of the effectiveness of the intervention. Taken together, this research uncovers a fundamental process through which the class ceiling is generated and offers solutions to resolve the identified issues, with implications for socioeconomic mobility, employee wellbeing, organizational effectiveness, and a positive role of organizations in the society.
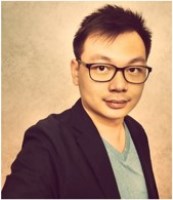
This dissertation addresses the empirical analysis on user-generated data from multiple online social platforms (OSPs) and modeling of latent user factors in multiple OSPs setting.
In the first part of this dissertation, we conducted cross-platform empirical studies to better understand user's social and work activities in multiple OSPs. In particular, we proposed new methodologies to analyze users' friendship maintenance and collaborative activities in multiple OSPs. We also apply the proposed methodologies on real-world OSP datasets, and the findings from our empirical studies have provided us with a better understanding on users' social and work activities which are previously not uncovered in single OSP studies.
In the second part of this dissertation, we developed user modeling techniques to learn latent user factors in multiple OSPs setting. In particular, we proposed generative models to learn the user topical interests, topic-specific platform preferences and influences in multiple OSPs setting. The proposed models are also applied to real-world OSPs datasets to profile user topical interests and identify influential users in multiple OSPs. The designed generative models are also generalizable and can be applied to different cross-OSP datasets.
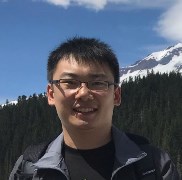
Question answering (QA) is one of the most important applications in natural language processing. With the explosive text data from the Internet, intelligently getting answers of questions will help humans more efficiently collect useful information. My research in this thesis mainly focuses on solving question answering problem with textual sequence matching model which is to build vectorized representations for pairs of text sequences to enable better reasoning. And our thesis consists of three major parts.
In Part I, we propose two general models for building vectorized representations over a pair of sentences, which can be directly used to solve the tasks of answer selection, natural language inference, etc.. In Chapter 3, we propose a model named ``match-LSTM", which performs word-by-word matching followed by a LSTM to place more emphasis on important word-level matching representations. On the Stanford Natural Language Inference (SNLI) corpus, our model achieved the state of the art. Next in Chapter 4, we present a general ``compare-aggregate'' framework that performs word-level matching followed by aggregation using Convolutional Neural Networks. We focus on exploring 6 different comparison functions we can use for word-level matching, and find that some simple comparison functions based on element-wise operations work better than standard neural network and neural tensor network based comparison.
In Part II, we make use of the sequence matching model to address the task of machine reading comprehension, where the models need to answer the question based on a specific passage. In Chapter 5, we explore the power of word-level matching for better locating the answer span from the given passage for each question in the task of machine reading comprehension. We propose an end-to-end neural architecture for the task. The architecture is based on match-LSTM and Pointer Net which constrains the output tokens coming from the given passage. We further propose two ways of using Pointer Net for our tasks. Our experiments show that both of our two models substantially outperform the best result using logistic regression and manually crafted features. Besides, our boundary model also achieved the best performance on the SQuAD and MSMARCO dataset. In Chapter 6, we will explore another challenging task, multi-choice reading comprehension, where several candidate answers are also given besides the question related passage. We propose a new co-matching approach to this problem, which jointly models whether a passage can match both a question and a candidate answer.
In Part III, we focus on solving the problem of open-domain question answering, where no specific passage is given any more comparing to the reading comprehension task. Our models for solving this problem still rely on the textual sequence matching model to build ranking and reading comprehension models. In Chapter 7, we present a novel open-domain QA system called Reinforced Ranker-Reader (R3), which jointly trains the Ranker along with an answer-extraction Readermodel, based on reinforcement learning. We report extensive experimental results showing that our method significantly improves on the state of the art for multiple open-domain QA datasets. As this work can only make use of a single retrieved passage to answer the question, in the next Chapter 8, we propose two models, strength-based re-ranking and coverage-based re-ranking, which make use of multiple passages to generate their answers. Our models have achieved state-of-the-art results on three public open-domain QA datasets: Quasar-T, SearchQA and the open-domain version of TriviaQA, with about 8 percentage points of improvement over the former two datasets.
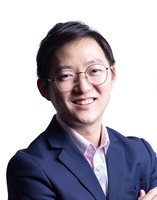
Financial Market Implications of Marketing Actions and the Disclosure of Marketing Information
This dissertation contributes to marketing literature by examining the effects of marketing actions and the disclosure of marketing information through the financial market perspective that has high managerial relevance and policy implications. Specifically, the first essay provides the first empirical examination of the effects of the disclosure of advertising spending on investors’ and analysts’ uncertainty. It is responsive to calls by Marketing Science Institute and Marketing Accountability Standard Board to examine the consequences of disclosure of marketing metrics. The second essay examines the effect of advertising spending on firm value and explores firm and market level contingency effects. By doing so, the second essay identifies relevant firm financial conditions and market environments, and provides managerial implications. Importantly, for the identification of the proposed effects in both essays, I apply the instrumentation strategy to address the potential endogeneity concerns related to disclosure of advertising spending and the level of advertising spending. Specifically, I develop and propose instruments alternative to those frequently used in extant literature in marketing. Accordingly, this dissertation contributes to marketing literature by proposing alternative instruments that can mitigate the critiques by Rossi (2014) and Angrist (2014) and potentially be used in other contexts.
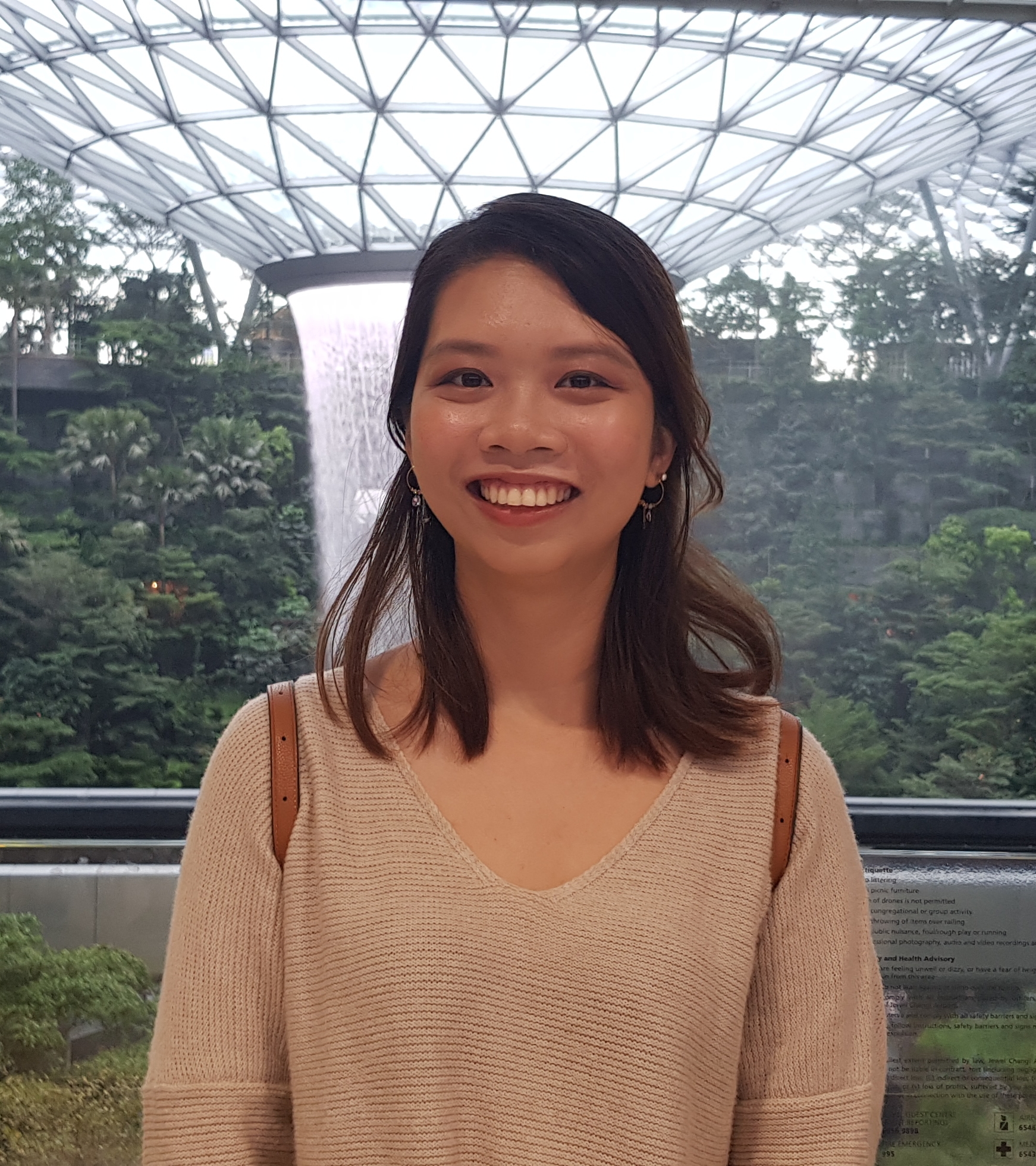
This dissertation discusses two questions in international economics. The first two chapters focus on the matter of global value chains. We first explore the participation of Singapore in the global value chains by characterizing the position of Singapore in the global network and identifying Singapore’s key upstream and downstream trade partners. This is done at both the country aggregate and at the sector level. We trace how the country’s position in global value chains has changed in the past two decades: whether it has moved upstream or downstream, how involved it is in global value chains, how its trend compares with other major Asian exporters (including Japan, Korea, China, Taiwan, and Hong Kong). In addition, the paper identifies the key sectors of Singapore which play a major role in the global trade networks.
The second chapter expands the analysis to a larger trading block – the Comprehensive and Progressive Agreement for Trans-Pacific Partnership (CPTPP). This is an example of a “mega-regional” free trade agreement, whose provisions on the rules of origin and trade facilitation can have potentially large impacts on the CPTPP-wide supply chains. We investigate whether the CPTPP members are key upstream and downstream trade partners to one another in the global value chains. In doing this, we hope to evaluate how closely connected the CPTPP members were with one another in the global value chains before the formation of CPTPP. Would alternative groupings with the addition of some third country enhance the tightness of the network? We develop formula of bilateral upstreamness and downstreamness, based on the gross-export decomposition framework of Koopman, Wang, and Wei (2014) and Borin and Mancini (2014). The chapter demonstrates how the decomposition of gross exports can be used to construct informative measures of the position of countries in the global value chains.
The final chapter explores a question on the effects of fluctuations in the crude oil market on various external accounts. We employ a structural Vector Autoregression model to investigate the impact of oil price changes on the external balances of oil-exporting and oil-importing countries. We look deeper into the non-oil trade balance of each country to determine the dynamics of the durable and non-durable trades in response to both demand and supply oil price shocks. We find that the source of crude oil price fluctuations lead to diverse effects on both the macroeconomic aggregates as well as the exports and the imports of goods. The paper reaffirms the importance of distinguishing shocks in the energy market when studying their effects and formulating appropriate policies.
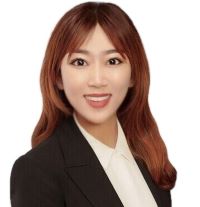
Essays in Asset Pricing
My dissertation centers on two areas related to market microstructure. First, the role of retail traders, or the information they have, for financial markets. I use retail short selling as an example to narrow down the topic and study their trading patterns and trading strategies. Second, the role of passive indexers such as index funds or ETFs, for financial markets. I am trying to analyze the channels through which the nominally uninformed traders have influences on the return and market quality of the underlying stocks.
In Chapter 2, I study retail short sellers. It is interesting to paint retail short sellers in a positive light, because most of the literature assume that retail investors are noise traders and less likely to take short selling positions. First, by using a novel short sell transaction data from 2010 to 2016, this paper is the first to provide a comprehensive sample of short selling initiated by retail investors. I find that retail short selling is not limited, which takes up around 11% of retail trading. Second, using this sample, I find that retail short selling can predict negative stock returns. A trading strategy that mimics weekly retail shorting earns an annualized riskadjusted value-(equal-) weighted return of 6% (12.25%). Their predictive ability is beyond that coming from overall retail investors as a group or from off-exchange institutional short sellers. Third, my results suggest that retail short sellers can profitably exploit public information, especially when it is negative. Retail short sellers also tend to be contrarians who provide liquidity when the market is one-sided due to (institutional) buying pressures. Therefore, this paper broadens our understanding on the heterogeneity of short sellers, sheds new light on the strategies of informed traders, and complements a growing literature about the informativeness of retail investors.
In Chapter 3, I am mainly working on passive investing. Since the decision to buy or sell stocks is often directed by broader fund flows and rebalancing and not typically by stock fundamentals, we construct proxies for the two sources of trading in passive investments: one is proportionally flow-induced trading; the other is disproportionally index rebalancing. Next, we consider systematic information and provide three measures of price efficiency: price delay, variance ratio, and return synchronicity. We find that indexing significantly increases price efficiency, especially the market- (industry-) wide information. There are two related channels that drive this positive effect. First is through arbitrage: passive investing causes price discrepancies and decreases arbitrage costs, which in turn increases the speed with which systematic information is incorporated into stock prices. The second driver of the positive effect is through short selling: stocks that are added to indexes increase the available lendable shares, which reduces the cost of short selling and thus makes the incorporation of (negative) information into price faster. Overall, this paper establishes and explains the link between indexing and market quality.
In Chapter 4, I am instead starting from passive holding. Especially, index funds right now are the largest stake holders of most SP500 stocks, and they are regarded as long-term holders. It is therefore important to see how the fund managers are motived to monitor, vote, and engage with firm-level governance and long-term performance. We find that the stocks with the longest passive holding indeed outperform. We stress that the active monitoring role of passive funds contributes to long-term value creation.
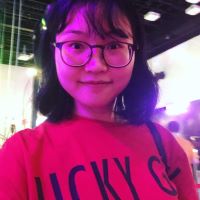
Dynamic analysis is widely used in malware detection, taint analysis, vulnerability detection, and other areas for enhancing the security of Android. Compared to static analysis, dynamic analysis is immune to common code obfuscation techniques and dynamic code loading. Existing dynamic analysis techniques rely on in-lab running environment (e.g., modified systems, rooted devices, or emulators) and require automatic input generators to execute the target app. However, these techniques could be bypassed by anti-analysis techniques that allow apps to hide sensitive behavior when an in-lab environment is detected through predefined heuristics (e.g., IMEI number of the device is invalid). Meanwhile, current input generators are still not intelligent enough to invoke adequate app behavior and provide sufficient code coverage. Therefore, it is an important research direction to investigate dynamic analysis techniques which enable a more complete execution under real running environments. This thesis focuses on dynamically analyzing app behavior by using public APIs and side-channel information, such that the techniques can be deployed on unrooted devices used by public users.
We first propose an advanced code obfuscation technique to hide small pieces of sensitive code with a code-reuse technique. This technique can hinder existing static analysis as well as dynamic analysis based on code-level events, such as API calls or Dalvik instructions. We implement a semi-automatic tool named AndroidCubo and show that it protects both Java and native code at a small runtime overhead.
Since relying on code-level event monitoring for revealing underlying app behavior can be bypassed by obfuscation and anti-analysis techniques, we propose a novel technique to dynamically monitoring apps by observing changes to public resources on the device. We propose to observe the resources with public APIs and virtual file interfaces to monitor sensitive behavior, and then use machine learning techniques to identify the initiating app of the behavior. We implement a system named UpDroid which contains a monitor published on Google Play and a server-side analyzer. UpDroid can be easily deployed on devices used by the public and successfully monitor sensitive behavior of the app that is being analyzed. This work is a successful investigation of dynamic analysis on unrooted devices.
To conduct more fine-grained analysis on apps, we propose to use GPU interrupt timing information to infer the launched app and concrete behavior within a running app, such as layout switching. We obtain GPU interrupt timing information from a side channel - /proc/interrupts. We sample the number of the raised GPU interrupt and get the timing series while an activity occurs on the device to generate a feature vector for that activity. Then, we use machine learning techniques to train classification models for the activities. With the models, we are able to identify different types of app activities, e.g., identify the launched app or disdinguish the activities within an app. This work further demonstrates the effectiveness of dynamic analysis on unrooted devices.
Finally, we conduct a simulation study for dynamically analyzing the factors that would affect the malware spreading on unrooted devices. In this work, we recruit participants to spread out messages, which simulates the malware spreading messages sent from infected mobile devices, to their friends. Each message contains a malicious-look link to simulate the malware downloading links. When the participants spread out the messages, we use dynamic analysis to monitor the status of their devices and record the infection rate. The results show that spreading method, relationship, contact frequency would significantly affect the spreading of malware by analyzing the infection rates of different statuses of the device and the differences of the spreading messages.
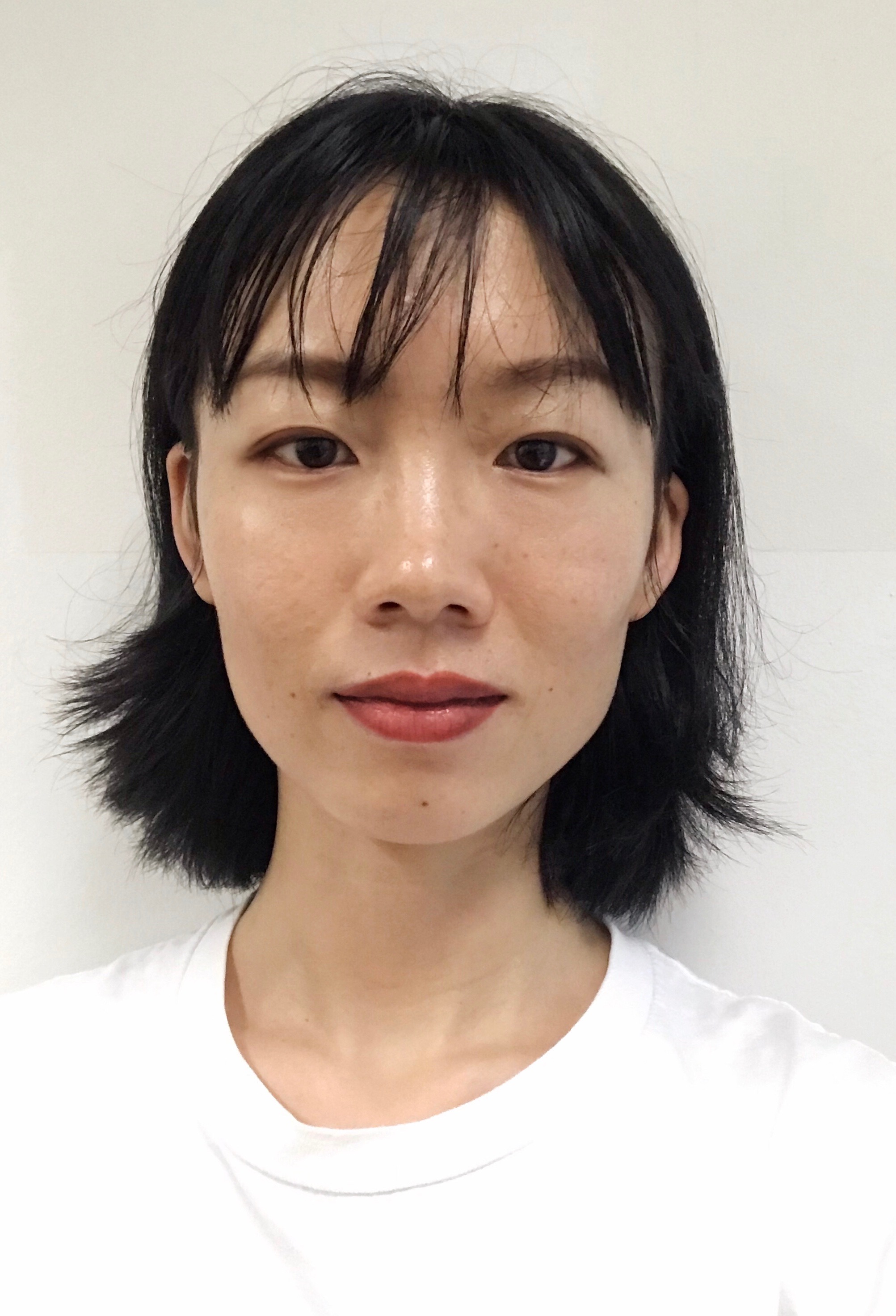
Land Policy and Welfare
This dissertation quantitatively studies the impact of land policy on welfare in China. There are two specific nationwide land policies of importance on which I focus: 1) The red-line policy that imposes a minimum 1.2 million square kilometers for agricultural use only 2) The zoning policy for urban land that strictly regulates the amount of industrial and residential land usages respectively; In the first chapter, I give an introduction about the land policy and institutional background in China and the related literature to my dissertation. Second chapter builds a two-sector(two-region) model to examine how the red-line policy interacts with land misallocation within agricultural-sector. Third chapter gives a spatial equilibrium with internal urban structure to quantify to what extent the zoning policy for urban land accounts for the empirically observed high price ratio of residential over industrial land and how it affects welfare.
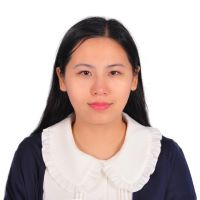
Essays on Anomalies in Asset Pricing
In Chapter 1, we examine whether various anomalies can be driven by two common behavioral forces, namely, "subjective" sentiment (representing investors' subjective biased beliefs) and "objective" limited attention (representing investors' objective cognitive constraints). While sentiment explains well many anomalies that are more speculative on the short-leg, it fails to explain anomalies that are equally speculative on the long and short-leg, including momentum and post-earnings announcement drift. Market-wide attention shifts, proxied by number of news averaged across stocks, significantly attenuates underreaction-driven anomalies, beyond the effect of sentiment. Our findings suggest that increase in market-wide attention can temporarily reduce the cost of attending to market and improve price efficiency.
In Chapter 2, we use systematic methods to solve factor timing problem and to improve the performance of factor investing. Past factor returns predict the cross section of factor returns, and this predictability is at its strongest at the one-month horizon (Arnott et al. 2019). We find that factor momentum is pervasive in international stock markets. We show that factor momentum can be captured by trading almost any set of factors. Industry momentum and size-B/M momentum stem from factor momentum. We further find that stock factor momentum, stock factor IVOL, and cross-assets factor momentum can generate alphas. These alphas cannot be explained by current asset pricing models.
In Chapter 3, We take an optimal portfolio approach on investing in multiple anomalies. We find that a variety of estimated optimal rules outperform substantially investing in any single anomaly. In addition, although it has been documented that the publication of a given anomaly may significantly reduce its standalone economic value, we show that these anomalies are still valuable collectively in the optimal portfolio even after they are published.
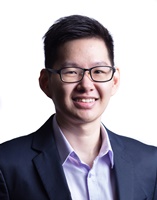
This dissertation examines digital strategies used by online retailers to engage customers and increase sales. The first essay investigates the impact of offering incentives to recapture lost sales from abandoned online shopping carts (i.e., customers adding items to their carts, but leaving without purchasing). Collaborating with an online fashion retailer, I conducted a randomized field experiment by manipulating the presence of incentives in recovery interventions. Findings reveal that incentives facilitate purchase conversion, but responsiveness to the incentives differs across various customer and cart characteristics.
In the second essay, I explore how retailers can leverage Augmented Reality (AR), a technology that helps users visualize how virtual objects fit into their physical reality, to enable customers to try products virtually. Using data from an international cosmetics retailer who incorporated AR in their mobile app, I find that customers who use AR display higher levels of in-app engagement, and are more inclined to explore products and brands they have never purchased before. Furthermore, AR benefits lower-priced products and less-popular brands and could potentially level the playing field for brands or products at the long tail of the product sales distribution.
The findings uncovered in these two essays contribute to the literature on digital marketing and retailing, and would benefit retailers who are planning to implement these strategies.
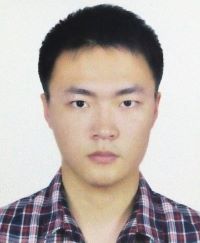
My dissertation consists of three essays which contribute new theoretical results to nonstationary time-series analysis and network dynamics.
Chapter 2 examines the limit properties of information criteria (such as AIC, BIC, HQIC) for distinguishing between the unit root model and the various kinds of explosive models. The explosive models include the local-to-unit-root model, the mildly explosive model and the regular explosive model. Initial conditions with different orders of magnitude are considered. Both the OLS estimator and the indirect inference estimator are studied. It is found that BIC and HQIC, but not AIC, consistently select the unit root model when data come from the unit root model. When data come from the local-to-unit-root model, both BIC and HQIC select the wrong model with probability approaching 1 while AIC has a positive probability of selecting the right model in the limit. When data come from the regular explosive model or from the mildly explosive model in the form of 1 + n^a/n with a in the range of (0,1), all three information criteria consistently select the true model. Indirect inference estimation can increase or decrease the probability for information criteria to select the right model asymptotically relative to OLS, depending on the information criteria and the true model. Simulation results confirm our asymptotic results in finite sample.
Chapter 3 studies a continuous time dynamic system with a random persistence parameter. The exact discrete time representation is obtained and related to several discrete time random coefficient models currently in the literature. The model distinguishes various forms of unstable and explosive behavior according to specific regions of the parameter space that open up the potential for testing these forms of extreme behavior. A two-stage approach that employs realized volatility is proposed for the continuous system estimation, asymptotic theory is developed, and test statistics to identify the different forms of extreme sample path behavior are proposed. Simulations show that the proposed estimators work well in empirically realistic settings and that the tests have good size and power properties in discriminating characteristics in the data that differ from typical unit root behavior. The theory is extended to cover models where the random persistence parameter is endogenously determined. An empirical application based on daily real S&P 500 index data over 1928-2018 reveals strong evidence against parameter constancy over the whole sample period leading to a long duration of what the model characterizes as extreme behavior in real stock prices.
Chapter 4 develops a dynamic covariate-assisted spectral clustering method to uniformly estimate the latent group membership of cryptocurrencies consistently. We show that return inter-predictability and crypto characteristics, including hashing algorithms and proof types, jointly determine the crypto market segmentation. Based on this classification result, it is natural to employ eigenvector centrality to identify a cryptocurrency’s idiosyncratic risk. An asset pricing analysis finds that a cross-sectional portfolio with a higher centrality earns a higher risk premium. Further tests confirm that centrality serves as a risk factor well and delivers valuable information content on cryptocurrency markets.
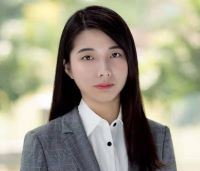
International trade, FDI and Agency Problems
This dissertation comprises three papers that separately study product quality in international trade, the governance’ effect on FDI and the agency problems in firms’ exporting decisions.
The first chapter quantifies the contribution of differences in quality preferences to the differences in gains from trade across countries. The quantification demonstrates that variations in the strength of quality preferences across countries add to heterogeneities across countries in market competitiveness. If the quality channel is shut down, countries with stronger preferences for quality have larger degrees of underestimations in their losses from the trade barrier. Finally, gains from a universal rise in quality preference are unequal among countries, with larger economies generally gaining more than smaller economies.
The second chapter proposes a theoretical model to micro-found firms’ optimal choice of FDI location, and sourcing and production, allowing for many countries, industries, and heterogeneous firms. We arrive at the main hypothesis that predicts an institutional complementarity pattern across countries in bilateral FDI flows at both the firm and country levels. We conduct an extensive test of the theory using worldwide bilateral FDI data at the firm level and at the country level. The results indicate a statistically significant assortative matching pattern in the institutional qualities of FDI origins and destinations.
The third chapter incorporates financial constraints into agency problems of firms. We show that under the same conditions, managers of potential exporting firms around the export threshold exert more efforts in financially under-developed countries to induce their owners to export. This finding has very positive policy implications, as firms in financially under-developed countries can compete with their peers in financially developed countries by exerting more managerial efforts. We find clear empirical evidence for this theoretical prediction using World Management Survey data for more than ten thousands firms from around 20 countries during 1999 – 2010.
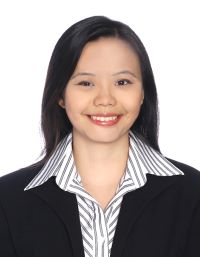
Objective: This study aimed to distinguish between daily experiences of gratitude and indebtedness through three stages - emotional appraisals, motivations to reciprocate and behavioural tendencies. Through these three stages, I aimed to gain a better insight into the emotional process involved before and after receiving favours. Method: 196 participants were recruited from Singapore Management University to take part in a 14-day diary study. Every two days, participants were asked to report a favour they received over the past two days and evaluate the favour based on their appraisals of the experience. They were also asked to report their motivation to reciprocate each favour received. Upon completing the diary study, participants attended a follow-up session where were asked to report their behavioural tendencies over the past week. Results: Gratitude and indebtedness were associated with varying extents with different benefit appraisals. For instance, gratitude was positively associated with perceived benevolence and indebtedness with perceived expectations of repayment. Gratitude was also found to motivated reciprocity via the desire to affiliate, while indebtedness motivated reciprocity through the desire to adhere to the norm of reciprocity. Finally, gratitude was associated with increased reports of affiliative behaviours while indebtedness was associated with the likelihood of repaying the favour. The theoretical implications, practical implications, and future directions of these findings were discussed.
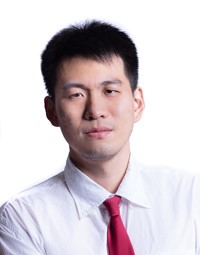
New Perspectives on M&A Research
Corporate acquisition is among the most important strategic tools wielded by managers to achieve competitive advantage. Acquisition may create strategic values for the acquirer by gaining market power through industry consolidation, diversifying into rapidly growing industries, entering into emerging markets, and most importantly by combining unique valuable resources from acquirer and target. Despite the many appealing aspects of the motive for acquisition, however, meta-analytical studies suggest that though highly beneficial for the target firm’s shareholders, acquisition on average destroys acquirer shareholder values. to understand the determinants of acquisition performance success, it becomes imperative to study the antecedents as well as consequences of acquisition. Existing literature has found scores of potential culprits including agency behavior, position in merger wave, managerial hubris, poor acquisition capability in post-acquisition integration, and loss of valuable human capital resources among others. Beyond acquisition performance, acquisition also results in many other intended and unintended consequences such as employee turnover and CEO departures. What may affect various acquisition outcomes? How do these outcomes affect future acquisition decisions?
In my dissertation, I look at two additional factors that may affect the success of acquisition by conducting two empirical studies on the antecedents as well as consequences of acquisition using behavioral and resource based theories. I further explore the connections between the antecedents and outcomes of acquisitions. In the second chapter, incorporating risk taking, I focus on the influences of alliance and acquisition performance feedback on the rate of future acquisitions. The Behavioral Theory of the Firm (BTF) and performance feedback theory have been used to explain corporate acquisition behaviors both at the organizational and deal levels. However, extant literature does not agree on the relationship between acquisition performance feedback and
likelihood of subsequent acquisition. Firms may search in different directions in response to acquisitions failures. Moreover, we still lack a clear understanding of the behavioral influences on the various sourcing mechanisms. To address these questions, we built on both the BTF and prospect theory to propose a search hierarchy among corporate sourcing methods. We posit that the general search direction in corporate development follows from simple to complex and from economical to costly. We hypothesize that, under the duo influences of problemistic search and risk taking, deal performance feedback is negatively related to future acquisitions. We further investigate the interaction between alliance and acquisition performance feedback on future acquisitions.
In the third chapter, given the importance of human capital retention and turnover in the success of acquisition, I examine how target firm’s knowledge base may affect acquirer’s decision to retain the target’s CEO. Drawing upon the firm-specific resources and strategic human capital literatures, we develop the argument that the level of firm-specific knowledge in an acquisition target may affect the likelihood of the target’s CEO being retained after an acquisition. Specifically, due to the important role of target CEOs in preserving the value of and integrating firm-specific knowledge, we expect a positive relationship between a target’s pre-acquisition firm-specific knowledge level and the likelihood that its CEO is retained. Furthermore, we argue that this relationship is strengthened by the target’s pre-acquisition performance, which signals a higher value of firm-specific knowledge, and the target CEO’s tenure, which is positively associated with both the benefit of retaining and the cost of replacing the CEO. Using a sample of acquisitions involving US target firms acquired between 1995 and 2006, we find support for our hypotheses.
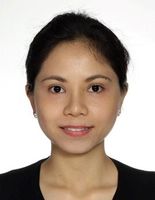
IT innovations disrupt traditional business models and challenge conventional thinking. Thus, industry incumbents face fierce competition from start-ups with new business models and new ways of engaging customers. Digital entertainment goods and personalized services have become a lucrative market, which has undergone a transformation enabled by seamless Internet connections. Meanwhile, social networks and other online platforms have brought people and business even closer.
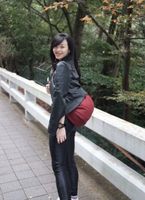
Negative stereotypes concerning females’ inferior quantitative abilities continue to hinder females’ preference and success in science, technology, engineering, and mathematics (STEM) fields. Studies on multiple identities show that priming females with a favorable identity, a social identity they possess that is associated with superior quantitative abilities, can reduce the aversive effects of stereotype threat. However, this line of research overlooked the fact that females manage their multiple identities in different ways and therefore respond to identity cues differently. This paper examined the role of gender-professional identity integration (G-PII), an individual difference on perceived compatibility of gender and professional identities, in influencing how women cope with stereotype threat when a favorable identity is primed. Study 1 examined how female professionals with varying levels of G-PII react to identity cues differently. Results show that only Low G-PIIs were sensitive to the identity cues and behaved in accordance to the primed identity. In contrast, High G-PIIs were not significantly influenced by the identity cues. Moreover, performance differences were only observed in a domain where females are stereotyped against (i.e., in a math test). Study 2 investigated how G-PII influences the effects of stereotype threat when a favorable identity is made salient during stereotype threat and the underlying mechanism that accounts for the performance difference observed amongst females with different levels of identity integration. The findings of Study 2 were not significant but were consistent with the prediction that Low G-PIIs spend more cognitive effort in processing identity cues, depleting those that could have been use for subsequent performance task. The theoretical implications, practical implications, and future directions of this paper will then be discussed.
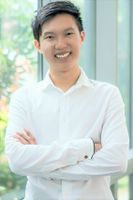
Despite a huge number of studies examining bilingual advantages in executive functions (EFs), the research findings with regards to the relations between bilingualism and EFs are mostly inconsistent and mixed. In order to shed light on these inconsistent findings, the current research aimed to tackle on both conceptual and methodological limitations that are prevalent in previous studies, namely: (a) failure to consider bilingual experiences in assessing bilingual advantages, and (b) task impurity due to substantial influence of non-EFs processes on EFs task performance. Based on Adaptive Control Hypothesis and Control Process Model of Code-switching, a theory-driven multisession study coupled with a latent variable approach was conducted to systematically examine the relations between bilingual interactional contexts and EFs, measured by nine different EFs tasks. The study found that dual-language context significantly predicted latent variable of task-switching, while dense code-switching context significantly predicted latent variable of inhibitory control and goal maintenance. The findings remained robust even taking into account potential confounds of demographics, socioeconomic status, intelligence, and unintended language-switching tendency. The current study identified bilingual interactional contexts as the key language experiences that could modulate the manifestation of bilingual advantages in EFs.
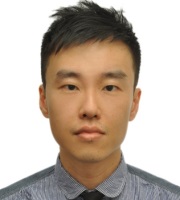
Research has shown that gratitude towards a benefactor positively predicts subjective well-being and other outcomes such as reciprocity and helping behaviours. However, previous research has not examined whether this effect is consistent or will differ across benefactor type (i.e., individual versus group). Research has also not examined the potential effects of accompanying thoughts related to the benefit assessment.
Through two experimental studies, the hypotheses that gratitude towards benefactor is lower for group benefactor as compared to individual benefactor, that self-entitlement thoughts and downward counterfactual thoughts will have main effects on gratitude as well as moderate the effect of benefactor type on gratitude, were tested. Results showed that the hypothesised main effect of benefactor type on gratitude was supported in one of the two studies (Study 2) but the other hypotheses were not supported.
Contrary to the hypothesised stronger positive effect, Study 2 found that there was no difference in effect between downward counterfactual thoughts and neutral thoughts that focused on recalling about benefiting experiences. Study 2 found that participants in the individual benefactor condition reported higher intent to help than participants in the group benefactor condition, and this effect of benefactor type on intent to help was partially mediated by gratitude.
In addition, trait gratitude was a moderator. When trait gratitude was high, those who reflected upon the benefits brought about by group benefactor experienced lower gratitude than those who reflected upon the benefits brought about by individual benefactor. However, when trait gratitude was low, the difference in the level of gratitude across benefactor type was not significant. The findings also showed that gratitude and indebtedness, as measured in both studies, were distinct constructs. Limitations of the current research, as well as future research directions and potential contributions were discussed.
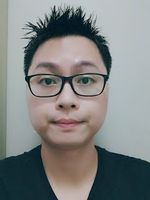
This chapter discusses the main problem and motivation of this dissertation. It also discusses a quantification of various research issues directly related to the dissertation. A summary of works done will also be presented along with the structure of the dissertation.
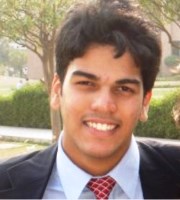
Online Learning with Nonlinear Models
This thesis proposes a general solution framework that integrates methods in machine learning in creative ways to solve a diverse set of problems arising in urban environments. It particularly focuses on modeling spatiotemporal data for the purpose of predicting urban phenomena. Concretely, the framework is applied to solve three specific real-world problems: human mobility prediction, trac speed prediction and incident prediction. For human mobility prediction, I use visitor trajectories collected a large theme park in Singapore as a simplified microcosm of an urban area. A trajectory is an ordered sequence of attraction visits and corresponding timestamps produced by a visitor. This problem has two related subproblems: (spatial) bundle prediction and trajectory prediction. In the first problem, I apply the framework to predict a bundle (i.e., an unordered set) of attractions that a given visitor would visit given a time budget. In the second problem, the framework is applied to predict the visitor's actual trajectory given the current partial trajectory and time budget. In both problems, I apply the methods of trajectory clustering, hidden Markov model, revealed preference learning and (inverse) reinforcement learning in the integrated framework. In trac speed prediction, I wish to predict the spatiotemporal distribution of trac speed over urban road networks. To this end, I propose local Gaussian processes which combine non-negative matrix (NMF) factorization with Gaussian process (GP) in order to enhance the efficiency of model training such that the solution could be deployed in real-time use cases. NMF is essentially a spatiotemporal clustering technique. The solution is extensively evaluated using real-world trac data collected in two U.S. cities. The incident prediction problem is about predicting the distribution of the number of crime incidents over urban areas in future time periods. Because of its similarity to the trac prediction problem above, its solution greatly benefits from the GP model developed earlier. Particularly, the GP kernel function is inherited and extended to model the distribution of incidents in urban areas and their features. The proposed solution is evaluated using real-world incident data collected in a large Asian city. Conceptually, this thesis uses machine learning techniques to solve three separate urban problems, whose contribution belongs to the large category of urban computing. At the core, its technical contribution lies in the unification of separate solutions tailored to those problems into an integrated framework that reasons with spatiotemporal data and, thus, is highly generalizable to other problems of similar nature.
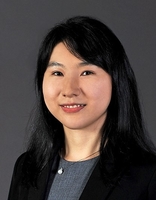
Essay on Asset Pricing
We uncover a novel stock return predictor from the options market, the volume-weighted strike-spot price ratio (VWKS) across all traded option contracts. High (low) VWKS indicates that the mass of options volume on an underlying stock centers at the out-of-the-money region of call (put) options. Empirically, VWKS has positive and robust predictive ability for underlying returns after controlling for a long list of variables including known return predictors from the options market, stock illiquidity, and past stock returns, and has more persistent and stronger predictive power for stocks with higher information asymmetry and arbitrage costs. We also find that VWKS exhibits abnormal run-ups and becomes more informative before permanent but not transitory price jumps, suggesting that options traders exploit only fundamental information. Finally, VWKS significantly predicts the merger premium in an event study.
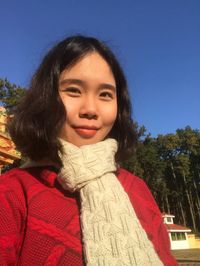
The rapid increase in online social networking services over the last decade has pre- sented an unprecedented opportunity to observe users’ behaviour both on a societal and individual level. The insight gained from analysing such data can help foster a deeper understanding of social media users and the flow of information, while also offering valuable business applications. User relationships are among the most studied aspects of online behaviour. These relationships are not homogeneous. Past research has shown that people use social networks to both socialize and source in- formation. Hence, different types of links – used to socialize, gain information, or both – are formed among users. While much research has focused on how users are connected online in general, it is crucial to explore how users interact with those present in offline social networks on the online social networks. Questions such as, ”What would speed up the diffusion of a piece of information?” can be better answered from an integrated offline-online perspective. My thesis explores the be- haviour of offline friends on the social information network in three main areas. I especially focus on social information networks, and use Twitter as a case study. In the first study, I explore and compare network characteristics on Twitter among of- fline friends and online friends. In the second study, I explore information diffusion in the same setting. In the last study, I investigate whether we can use the measure- ment of tie strength among friends on Twitter as a substitute for, or a complement to the measurement of tie strength among friends in the offline world.
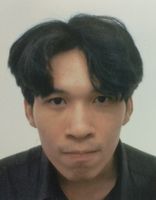
Recommending APIs for Software Evolution
Softwares are constantly evolving. This evolution has been made easier through the use of Application Programming Interfaces (APIs). By leveraging APIs, developers reuse previously implemented functionalities and concentrate on writing new codes. These APIs may originate from either third parties or internally from other compo- nents of the software that are currently developed. In the first case, developers need to know how to find and use third party APIs. In the second case, developers need to be aware of internal APIs in their own software. In either case, there is often too much information to digest. For instance, finding the right APIs may require sifting through many different APIs and learning them one by one, which can easily cost a large amount of time. Also, as the software becomes bigger and more complex, developers may not be aware of all functionalities available in their software.
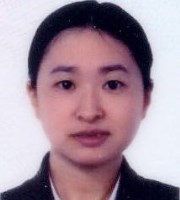
Scalable Online Kernel Learning
One critical deficiency of traditional online kernel learning methods is their increasing and unbounded number of support vectors (SV’s), making them inefficient and non-scalable for large-scale applications. Recent studies on budget online learning have attempted to overcome this shortcoming by bounding the number of SV’s. Despite being extensively studied, budget algorithms usually suffer from several drawbacks. First of all, although existing algorithms attempt to bound the number of SV’s at each iteration, most of them fail to bound the number of SV’s for the final averaged classifier, which is commonly used for online-to-batch conversion. To solve this problem, we propose a novel bounded online kernel learning method, Sparse Passive Aggressive learning (SPA), which is able to yield a final output kernel-based hypothesis with a bounded number of support vectors. The idea is to attain the bounded number of SV’s using an efficient stochastic sampling strategy which samples an incoming training example as a new SV with a probability proportional to its loss suffered. Since the SV’s are added wisely and no SV’s are removed during the learning, the proposed SPA algorithm achieves a bounded final averaged classifier. We theoretically prove that the proposed SPA algorithm achieves an optimal regret bound in expectation, and empirically show that the new algorithm outperforms various bounded kernel-based online learning algorithms. Secondly, existing budget learning algorithms are either too simple to achieve satisfactory approximation accuracy, or too computationally intensive to scale for large datasets. To overcome this limitation and make online kernel learning efficient and scalable, we explore two functional approximation based online kernel machine learning algorithms, Fourier Online Gradient Descent (FOGD) and Nystr¨om Online Gradient Descent (NOGD). The main idea is to adopt the two methods to approximate the kernel model with a linear classifier, so that the efficiency is highly improved. The encouraging results of our experiments on large-scale datasets validate the effectiveness and efficiency of the proposed algorithms, making them potentially more practical than the family of existing budget online kernel learning approaches Thirdly, we also extend the proposed algorithms to solve the online multiple kernel learning (OMKL) problem, in which the goal is to significantly reduce the learning complexity of Online Multiple Kernel Classification (OMKC) while achieving satisfactory accuracy as compared with existing unbounded OMKC algorithms. We theoretically prove that the proposed algorithms achieve an optimal regret bound, and empirically show that the new algorithms outperform various bounded kernel-based online learning algorithms. In conclusion, this work presents several novel solutions for scaling up online kernel learning algorithms for large scale applications. To facilitate other researchers, we also provide an open source software tool-box that includes the implementation of all proposed algorithms in this paper, as well as many state-of-the-art online kernel learning algorithms.
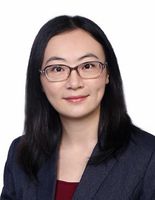
Music Popularity, Diffusion and Recommendation in Social Networks: A Fusion Analytics Approach
Streaming music and social networks offer an easy way for people to gain access to a massive amount of music, but there are also challenges for the music industry to design for promotion strategies via the new channels. My dissertation employs a fusion of machine-based methods and explanatory empiricism to explore music popularity, diffusion, and promotion in the social network context.
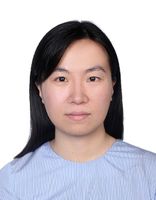
Advanced Malware Detection for Android Platform
In the first quarter of 2018, 75.66% of smartphones sales were devices running An- droid. Due to its popularity, cyber-criminals have increasingly targeted this ecosys- tem. Malware running on Android severely violates end users security and privacy, allowing many attacks such as defeating two factor authentication of mobile bank- ing applications, capturing real-time voice calls and leaking sensitive information. In this dissertation, I describe the pieces of work that I have done to effectively de- tect malware on Android platform, i.e., ICC-based malware detection system (IC- CDetector), multi-layer malware detection system (DeepRefiner), and self-evolving and scalable malware detection system (DroidEvolver) for Android platform.
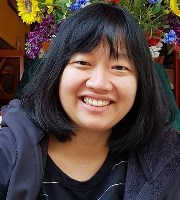
Policy analytics are essential in supporting more informed policy-making in environmental management. This dissertation employs a fusion of machine methods and explanatory empiricism that involves data analytics, math programming, optimization, econometrics, geospatial and spatiotemporal analysis, and other approaches for assessing and evaluating current and future environmental policies.
Essay 1 discusses household informedness and its impact on the collection and recycling of household hazardous waste (HHW). Household informedness is the degree to which households have the necessary information to make utility-maximizing decisions about the handling of their waste. Such informedness seems to be influenced by HHW public education and environmental quality information. This essay assesses the effects of household informedness on HHW collection and recycling using public agency data, community surveys, drinking water compliance re-ports, and census data for California from 2004 to 2012. The results enable the calculation of the elasticity of the output quantities of HHW collected and recycled for differences in household informedness at the county level.
Essay 2 considers the pro-environmental spatial spillovers, based on agency actions and waste collection behavior that is occurring in other counties, that represent the influence of HHW-related practices in close-by regions. Using county-level spatio-temporal datasets that consist of economic, demographic, and HHW data in California from 2004 to 2015, I evaluate the impact of grants on the HHW collection activities using a research design that emphasizes spatial variations and controls for confounding factors. A random effects panel data model with instrumental variables is then developed to measure the effects of HHW grant on HHW collection activities while considering the spatial effects from the influence of the waste collection activities among close-by counties or regions.
Essay 3 assesses transition pathways in electricity generation and their future water impacts using an electricity generation capacity expansion model. Scenarios that do or do not comply with the U.S. Environmental Protection Agency's proposed carbon pollution standards – the New Source Performance Standards and Clean Power Plan – are considered. Using the Electric Reliability Council of Texas region as an illustration, the scenarios with the carbon regulations are shown to have lower water use from the power sector than the continuation of the status quo with more electricity generation from coal than natural gas. This is due to an increase in electricity generation from renewable sources and natural gas combined cycle plants that is influenced by the CO2 allowance price. Water withdrawal limits affect electricity generation, decreasing it from power plants with once-through cooling, but this will increase water consumption.
These essays demonstrate the use of a variety of data analytics and management science methods that represent advances in policy analytics to overcome the research challenges, such as the data limitations, the uncertainties associated with the analysis of energy futures, and best practices establishing causal estimates in empirical research designs. This dissertation contributes to the growing body of research on policy analytics for environmental sustainability and improves our understanding of how to craft policies that enhance sustainability for the future.
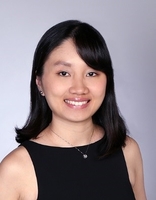
Examining Outcomes of Marketing Actions from Consumers, Investor and Operational Perspectives
This dissertation examines the impact of three distinct marketing actions from three different perspectives, i.e., customer, investor, and operational. Specifically, the first essay examines investors’ evaluation of firms’ price-increase preannouncements, thereby responding to recent calls for exploring investors’ evaluation of a firm’s pricing actions which have been predominantly examined from consumers’ perspective. The second essay adopts an operations lens to present the first empirical examination about the impact of customer satisfaction on the future costs of selling and producing for a firm. The essay, therefore, is of direct importance to CEOs as they consider costs as their top priority. In addition, it is responsive to recent calls for more research on the cost implications of marketing actions. Finally, the third essay integrates the customer, investor and operational perspectives, to explore the consequences of mergers and acquisitions using a stakeholder-specific approach. Specifically, using a longitudinal dataset, this essay examines how mergers and acquisitions in the airline industry have an impact on key stakeholders – consumers, employees, senior managers, and investors. Taken together, this dissertation seeks to contribute to existing literature by exploring, for different stakeholders, the outcomes of marketing actions that have high managerial relevance, but have received little attention in current literature.
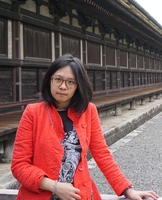
Essays in Corporate Finance
This dissertation studies the impact of credit rating on firms’ financing behavior and investigates insider trading activities.
The first essay documents how firms’ concerns about credit rating change affect their choice between the use of debt and lease. Firms approaching a credit rating change tend to use less debt relative to operating leases to finance their new projects. In this paper, I propose a new method of measuring the potential of a credit rating change. Using the new measures, I find that not only the concerns about being downgraded but also the at- tempts to get upgraded have significant impacts. The result is surprising because rating agencies are fully aware of firms’ use of off-balance-sheet finance and make correspond- ing adjustments when they assess firms’ creditworthiness. There are two possible reasons for the result. First, the operating lease obligations are usually underestimated. Second, auditors tolerate more misstatement for disclosed off-balance-sheet items than they do for recognized balance sheet items.
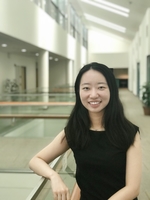
Three Essays on Corporate Finance
This dissertation has three essays in corporate finance. In the first chapter, We investigate whether a CEO’s experience with mergers matter when her firm becomes a takeover target? We find that shareholders receive higher premiums when their CEO has experience. The evidence suggests this is due to learning rather than innate skills or selection. Consistent with superior negotiation of salient features of takeover offers, experienced target CEOs obtain either safer cash payments or higher premiums as the fraction of cash in the offer decreases. These benefits do not come at the cost of other contractual concessions or inefficiencies in takeover negotiations. Overall, the results suggest that M&A experience is valuable when the CEO’s firm becomes a takeover target.
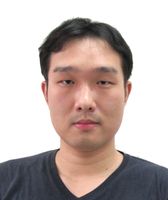
Three Essays on Empirical Asset Pricing
This thesis consists of three chapters. In Chapter 1, I show that returns to currency carry and momentum strategies are compensations for the risk of US monetary policy uncertainty (MPU), with risk exposures explaining 96% of their cross-sectional return variations. The findings are consistent with an intermediary-based exchange rate model. Higher MPU triggers position unwinding by the intermediary, which decreases there turns of currency with high-interest rate or appreciation, while that with low-interest rate or depreciation earns positive returns. Different responses stem from the long and short behavior of the intermediary. The explanatory power of US MPU risk is robust and unrelated to commonly used risk factors.
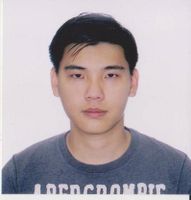
Essays in Commodities and Freight Markets
Chapter 1: Structural Changes in Functional Curves: Estimation and Testing
Abstract: This paper considers the estimation and testing of structural changes in functional curves that occurs at an unknown date. The functional principal component analysis is applied to the random functional curves, decomposing them into interpretable simple latent functions and random scalars. We model the random scalars using a simple autoregressive model and test for a change in parameters that occur at an unknown date. This method is applied to the crude oil futures market to estimate and date possible structural breaks during OPEC announcement periods from 1984 to 2017.
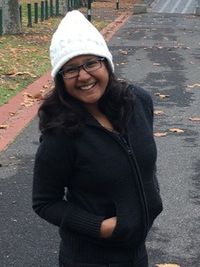
Proactive and Reactive Resource/Task Allocation for Agent Teams in Uncertain Environments
Synergistic interactions between task/resource allocation and multi-agent coordinated planning/assignment exist in many problem domains such as trans- portation and logistics, disaster rescue, security patrolling, sensor networks, power distribution networks, etc. These domains often feature dynamic environments where allocations of tasks/resources may have complex dependencies and agents may leave the team due to unforeseen conditions (e.g., emergency, accident or violation, damage to agent, reconfiguration of environment).
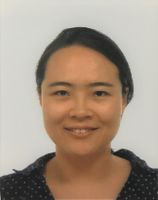
On Human Capital Development in Bangladesh
This dissertation consists of three chapters on human capital development in Bangladesh. The first chapter provides microeconometric evidence that access to electricity has a positive impact on the nutritional status of children under five in rural Bangladesh.
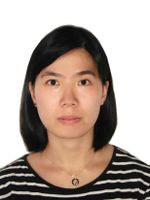
Automatic Vulnerability Detection and Repair
Vulnerability becomes a major threat to the security of many systems, including computer systems (e.g., Windows and Linux) and mobile systems (e.g., Android and iOS). Attackers can steal private information and perform harmful actions by exploiting unpatched vulnerabilities. Vulnerabilities often remain undetected for a long time as they may not affect the typical functionalities of systems. Thus, it is important to detect and repair a vulnerability in time. However, it is often difficult for a developer to detect and repair a vulnerability correctly and timely if he/she is not a security expert. Fortunately, automatic repair approaches significantly assist developers to deal with different types of vulnerabilities. There are lots of work to detect different vulnerabilities, and only few vulnerability repair approaches are proposed to repair certain types of vulnerabilities.
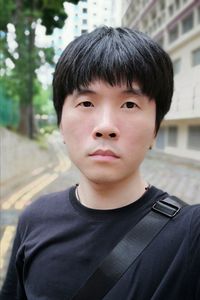
Secure Enforcement Of Isolation Policy On Multicore Platforms With Virtualization Techniques
A number of virtualization based systems have been proposed in the literature as an effective measure against the adversaries with the kernel privilege. However, under a systematic analysis, such systems exhibit vulnerabilities that can still be exploited by such an attacker with the kernel privilege. The fundamental reason is that there is an inherent incompatibility between the tamper-proof requirement and the complete mediation requirement of the reference monitor model. The incompatibility manifests in the virtualization based systems in the form of a discrepancy between the enforcement capability demanded by the high-level policy and the one achievable through the system design approach mandated by the low-level hardware enforcement mechanism.
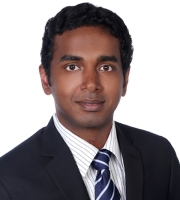
Due to the increasing population and lack of coordination, there is a mismatch in supply and demand of common resources (e.g., shared bikes, ambulances, taxis) in urban environments, which has deteriorated a wide variety of quality of life metrics such as success rate in issuing shared bikes, response times for emergency needs, waiting times in queues etc. Thus, in my thesis, I propose efficient algorithms that optimise the quality of life metrics by proactively redistributing the resources using intelligent operational (day-to-day) and strategic (long-term) decisions in the context of urban transportation and health & safety. For urban transportation, Bike Sharing System (BSS) is adopted as the motivating domain. Operational decisions are crucial for BSS, because the stations of BSS are often not balanced due to uncoordinated movements of resources (i.e., bikes) by customers. The imbalanced stations lead to significant loss in demand and increase the usage of private transportation and therefore, defeat the primary objective of BSS which is to reduce carbon footprint. In order to reduce the carbon footprint, I contribute three operational decision making approaches for sequential redistribution of bikes: (i) Optimising lost demand through dynamic redistribution; (ii) Optimising lost demand through robust redistribution; and (iii) Optimising lost demand through incentives. In the first approach, I consider the expected demand for multiple time steps to find a redistribution solution and provide novel decomposition and abstraction mechanisms to speed up the solution process. This approach is useful for BSS with consistent demand patterns. Therefore, the second approach proposes a robust redistribution solution using the notion of two-player adversarial game to address the scenarios where the demand has high variance. For the third approach, within the central budget constraints of the operators, a mechanism is designed to incentivise the customers for executing the bike redistribution tasks by themselves. The experimental results on two real-world data sets of Capital Bikeshare (Washington, DC) and Hubway (Boston, MA) BSS demonstrate that our approaches significantly reduce the average and worse case lost demand over the current practices. For health & safety, Emergency Medical System (EMS) is adopted as the motivating domain. EMS is an extremely sensitive and critical domain for public healthcare services, because reducing the response times for emergency incidents by a few seconds can save a human life. In order to reduce the response times, I propose strategic decision making approach for EMS so as to place base stations at “right” location and allocate “right” number of ambulances on those bases. An accelerated version of greedy algorithm on top of an existing data-driven optimisation formulation is proposed to jointly consider the placement of bases and allocation of ambulances. Subsequently, I provide insights to improve the operational decisions of EMS for dynamic redistribution of ambulances by incorporating the exact real-world dynamics of EMS into the existing data-driven optimisation formulation. Experimental results on real-world data sets demonstrate that both our strategic and operational decisions improve the efficacy of EMS over the existing approaches.
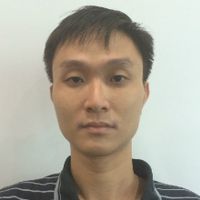
This dissertation addresses the modeling of latent characteristics of locations to describe the mobility of users of location-based social networking platforms. With many users signing up location-based social networking platforms to share their daily activities, these platforms become a gold mine for researchers to study human visitation behavior and location characteristics. Modeling such visitation behavior and location characteristics can benefit many use- ful applications such as urban planning and location-aware recommender sys- tems. In this dissertation, we focus on modeling two latent characteristics of locations, namely area attraction and neighborhood competition effects using location-based social network data. Our literature survey reveals that previous researchers did not pay enough attention to area attraction and neighborhood competition effects. Area attraction refers to the ability of an area with mul- tiple venues to collectively attract check-ins from users, while neighborhood competition represents the need for a venue to compete with its neighbors in the same area for getting check-ins from users.
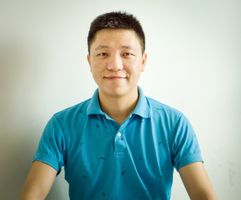
Entity Summarization of Review and Micro-Reviews
Along with the regular review content, there is a new type of user-generated content arising from the prevalence of mobile devices and social media, that is micro-review. Micro-reviews are bite-size reviews (usually under 200 char- acters), commonly posted on social media or check-in services, using a mobile device. They capture the immediate reaction of users, and they are rich in information, concise, and to the point. Both reviews and micro-reviews are useful for users to get to know the entity of interest, thus facilitating users in making their decision of purchasing or dining. However, the abundant number of both reviews and micro-reviews makes it increasingly difficult to go through them and extract the useful information. In this dissertation, we propose to summarize reviews and micro-reviews to ease users in understanding entity (or a set of entities).
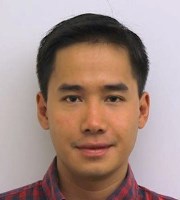
An Integrated Framework for Modeling and Predicting Spatiotemporal Phenomena in Urban Environments
This thesis proposes a general solution framework that integrates methods in machine learning in creative ways to solve a diverse set of problems arising in urban environments. It particularly focuses on modeling spatiotemporal data for the purpose of predicting urban phenomena. Concretely, the framework is applied to solve three specific real-world problems: human mobility prediction, trac speed prediction and incident prediction. For human mobility prediction, I use visitor trajectories collected a large theme park in Singapore as a simplified microcosm of an urban area. A trajectory is an ordered sequence of attraction visits and corresponding timestamps produced by a visitor. This problem has two related subproblems: (spatial) bundle prediction and trajectory prediction. In the first problem, I apply the framework to predict a bundle (i.e., an unordered set) of attractions that a given visitor would visit given a time budget. In the second problem, the framework is applied to predict the visitor's actual trajectory given the current partial trajectory and time budget. In both problems, I apply the methods of trajectory clustering, hidden Markov model, revealed preference learning and (inverse) reinforcement learning in the integrated framework. In trac speed prediction, I wish to predict the spatiotemporal distribution of trac speed over urban road networks. To this end, I propose local Gaussian processes which combine non-negative matrix (NMF) factorization with Gaussian process (GP) in order to enhance the efficiency of model training such that the solution could be deployed in real-time use cases. NMF is essentially a spatiotemporal clustering technique. The solution is extensively evaluated using real-world trac data collected in two U.S. cities. The incident prediction problem is about predicting the distribution of the number of crime incidents over urban areas in future time periods. Because of its similarity to the trac prediction problem above, its solution greatly benefits from the GP model developed earlier. Particularly, the GP kernel function is inherited and extended to model the distribution of incidents in urban areas and their features. The proposed solution is evaluated using real-world incident data collected in a large Asian city. Conceptually, this thesis uses machine learning techniques to solve three separate urban problems, whose contribution belongs to the large category of urban computing. At the core, its technical contribution lies in the unification of separate solutions tailored to those problems into an integrated framework that reasons with spatiotemporal data and, thus, is highly generalizable to other problems of similar nature.
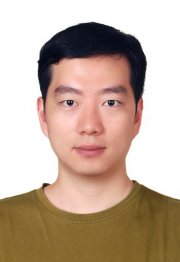
International Trade, Trade Policy, and the WTO
This dissertation comprises three papers that study the welfare impact of GATT/WTO, the effects of preference bias on trade flows and welfare, and the optimal trade policy with strategic interactions under a Ricardian model. The first chapter provides a comprehensive evaluation of the welfare impact of GATT/WTO in its entire history of 1950-2015 for 180 countries. The analysis embeds non-parametric matching methods in structural quantitative simulations. The results indicate substantial (but highly heterogeneous) welfare gains created by GATT/WTO at the global level and across more than six decades of its history. An extensive set of robustness checks with respect to model specifications, parameter values, and matching estimations are provided. We also characterize the effects of GATT/WTO on global income disparity, its interaction with preferential trade agreements, and the effects of China‘s WTO entry.
The second chapter estimates the effects of bilateral and time-varying preference bias on trade flows and welfare. We use a unique dataset from the BBC World Opinion Poll that surveys (annually during 2005-2017 with some gaps) the populations from a wide array of countries on their views of whether an evaluated country is having a mainly positive or negative influence in the world. We identify the effects on bilateral preference parameters due to shifts in these country image perceptions, and quantify their general equilibrium effects on bilateral exports and welfare (each time for an evaluated exporting country, holding the exporting country's own preference parameters constant). We consider five important shifts in country image: the George W. Bush effect, the Donald Trump effect, the Senkaku Islands Dispute effect, the Brexit effect, and the Good-Boy Canadian effect. We find that such changes in bilateral country image perceptions have quantitatively important trade and welfare effects. The negative impact of Donald Trump’s “America First" campaign rhetorics on the US' country image might have cost the US as much as 3% of its total exports and gains from trade. In contrast, the consistent improvement of Canadian country image between 2010 and 2017 has amounted to more than 8% of its total welfare gains from trade.
The third chapter incorporates strategic interactions into a canonical Ricardian model where two countries choose their optimal trade taxes. We show that in a Nash equilibrium, a country's optimal import tariffs are zero, whereas the optimal export taxes are weakly increasing with respect to its comparative advantage. Compared with Costinot, Donaldson, Vogel, and Werning (2015) where Foreign is passive, the structure of optimal trade policy with strategic interactions remains the same, but the welfare gain from trade policy for each country is lower. When Foreign is active, the welfare gain for Home from its trade policy is smaller because Foreign also exerts its market power on its exported goods over Home's consumers.
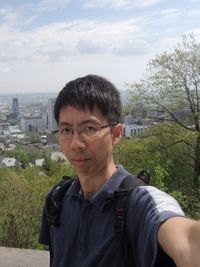
This dissertation addresses context recovery in Location-Based Social Networks (LBSN), which are platforms where users post content from various locations. With this general LBSN definition, many existing social media platforms that support user-generated location relevant content using mobile devices could also qualify as LBSNs. Context recovery for such user posts refers to recovering the venue and the semantic contexts of these user posts. Such information is useful for user profiling and to support various applications such as venue recommendation and location- based advertising.
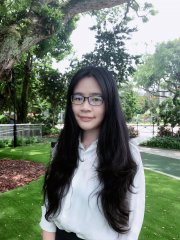
This dissertation develops several econometric techniques to address the unobserved heterogeneity in nonstationary panels, namely identifying latent group structures in cointegrated panels, studying nonstationary panels with both cross-sectional dependence and latent group structures, and estimating panel error-correction model with unobserved dynamic common factors.
Chapter 1 considers a panel cointegration model with latent group structures that allows for heterogeneous long-run relations across groups. We extend Su et al. (2013) classifier-Lasso (C-Lasso) method to the nonstationary panels and allow for the presence of endogeneity in both the stationary and nonstationary regressors in the model. In addition, we allow the dimension of the stationary regressors to diverge with the sample size. We show that we can identify the individuals’ group membership and estimate the group-specific long-run cointegrated relationships simultaneously. We demonstrate the desirable property of uniform classification consistency and the oracle properties of both the C-Lasso estimators and their post-Lasso versions. The special case of dynamic penalized least squares is also studied. Simulations show superb finite sample performance in both classification and estimation. In an empirical application, we study the potential heterogeneous behavior in testing the validity of long-run PPP hypothesis in the post-Bretton Woods period from 1975-2014 covering 99 countries. We identify two groups in the period 1975-1998 and three ones in the period 1999-2014. The results confirm that at least some countries favor the long-run PPP hypothesis in the post-Bretton Woods period.
Chapter 2 proposes a novel approach, based on Lasso, to handle unobserved parameter heterogeneity and cross-sectional dependence in nonstationary panel models. We propose a penalized principal component method to jointly estimate group-specific long-run relationships, unobserved common factors and to identify unknown group membership. Our Lasso-type estimators are consistent and efficient. We provide a bias-correction procedure under which our estimators are centered around zero as both dimensions of the panel tend to infinity. We establish a mixed normal asymptotic distribution for our estimators, which permit inference using standard test statistics. Finally, we apply our approach to study the international R&D spillovers model with unobserved group patterns. The results shed new light on growth convergence puzzle though the channel of technology diffusions.
Chapter 3 proposes a novel econometric model that accounts for both long-run and short-run co-movements in panel error correction models. By imposing latent group structures, we achieve efficient estimation for long-run cointegration vectors in the presence of unobserved heterogeneity. The short-run co-movements are driven by unobserved dynamic common factors, which can be consistently estimated by principal components. We propose a penalized generalized least squares method that jointly estimates long-run cointegration vectors and infers unobserved group structures. We establish asymptotic properties for two Lasso-type estimators. In an empirical application, we estimate longrun cointegration relationships between bid and ask quotes in stock market. We introduce a new measure for efficient price, which is weighted average of bid and ask quotes.
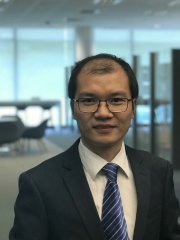
Three Essays on Panel Structure Models
In panel structure models, individuals can be classified into different groups with the slope parameters being homogeneous within the same group but heterogeneous across groups, both the number of groups and each individual’s group membership are unknown. This dissertation proposes some methods to identify the panel structure models under different specifications, namely, developing a Lasso-type Panel-CARDS method in the linear panel, constructing two sequential binary segmentation algorithms in the nonlinear panel, and using K-means algorithm in the spatial panel.
Chapter 2 studies the estimation of a linear panel data model with latent structures. To identify the unknown group structure of vector parameters, we design an algorithm called Panel-CARDS which is a systematic extension of the CARDS procedure proposed by Ke, Fan, and Wu (2015) in a cross-sectional framework. We show that it can identify the true group structure asymptotically and estimate the model parameters consistently at the same time. Simulations evaluate performance and corroborate the asymptotic theory in several practical design settings. The empirical economic application considers the effect of income on democracy by using cross-country data over the period 1961-2000. It reveals the presence of latent groupings in this panel data.
Chapter 3 proposes a procedure to identify latent group structures in nonlinear panel data models. To identify the group structures, we consider the order statistics for the preliminary unconstrained consistent estimators of the regression coefficients and translate the problem of classification into the problem of break detection. Then we extend the sequential binary segmentation algorithm of Bai (1997) for break detection from the time series setup to the panel data framework. We demonstrate that our method can identify the true latent group structures with probability approaching one and the post-classification estimators are oracle-efficient. The method has the advantage of more convenient implementation compared with some alternative methods, which is a desirable feature in nonlinear panel applications. To improve the finite sample performance, we also consider an alternative version based on the spectral decomposition of certain estimated matrix and link the group identification issue to the community detection problem in the network literature. Simulations show that our method has good finite sample performance. We apply this method to explore how individuals’ portfolio choices respond to their financial status and other characteristics using the Netherlands household panel data from year 1993 to 2015, and find three latent groups.
Chapter 4 considers the identification of latent group structures in dynamic spatial models with interactive fixed effects. The model treats three kinds of heterogeneity at the same time, namely, the spatial heterogeneity, individuals’ heterogeneous responses to the same time factors, and heterogeneous slope coefficients. To identify the latent group structures, we adopt quasi-maximum likelihood estimation to get preliminary unconstrained estimators of the slope coefficients. Then we use the K-means algorithm on slope coefficients’ consistent preliminary estimators to get the clusters. The asymptotic analysis shows that this method can identify the true group structure consistently. Therefore, the post-classification estimators have the oracle property. Monte Carlo simulations demonstrate that it has good finite sample performance.
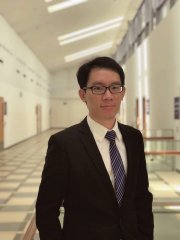
Three Essays on Bayesian Econometrics
My dissertation consists of three essays which contribute new theoretical results to Bayesian econometrics.
Chapter 2 proposes a new Bayesian test statistic to test a point null hypothesis based on a quadratic loss. The proposed test statistic may be regarded as the Bayesian version of the Lagrange multiplier test. Its asymptotic distribution is obtained based on a set of regular conditions and follows a chi-squared distribution when the null hypothesis is correct. The new statistic has several important advantages that make it appealing in practical applications. First, it is well-defined under improper prior distributions. Second, it avoids Jeffrey-Lindley’s paradox. Third, it always takes a non-negative value and is relatively easy to compute, even for models with latent variables. Fourth, its numerical standard error is relatively easy to obtain. Finally, it is asymptotically pivotal and its threshold values can be obtained from the chi-squared distribution.
Chapter 3 proposes a new Wald-type statistic for hypothesis testing based on Bayesian posterior distributions. The new statistic can be explained as a posterior version of Wald test and have several nice properties. First, it is well-defined under improper prior distributions. Second, it avoids Jeffreys-Lindley’s paradox. Third, under the null hypothesis and repeated sampling, it follows a c2 distribution asymptotically, offering an asymptotically pivotal test. Fourth, it only requires inverting the posterior covariance for the parameters of interest. Fifth and perhaps most importantly, when a random sample from the posterior distribution (such as an MCMC output) is available, the proposed statistic can be easily obtained as a by-product of posterior simulation. In addition, the numerical standard error of the estimated proposed statistic can be computed based on the random sample. The finite-sample performance of the statistic is examined in Monte Carlo studies.
Chapter 4 proposes a quasi-Bayesian approach for structural parameters in finitehorizon life-cycle models. This approach circumvents the numerical evaluation of the gradient of the objective function and alleviates the local optimum problem. The asymptotic normality of the estimators with and without approximation errors is derived. The proposed estimators reach the efficiency bound in the general methods of moment (GMM) framework. Both the estimators and the corresponding asymptotic covariance are readily computable. The estimation procedure is easy to parallel so that the graphic processing unit (GPU) can be used to enhance the computational speed. The estimation procedure is illustrated using a variant of the model in Gourinchas and Parker (2002).