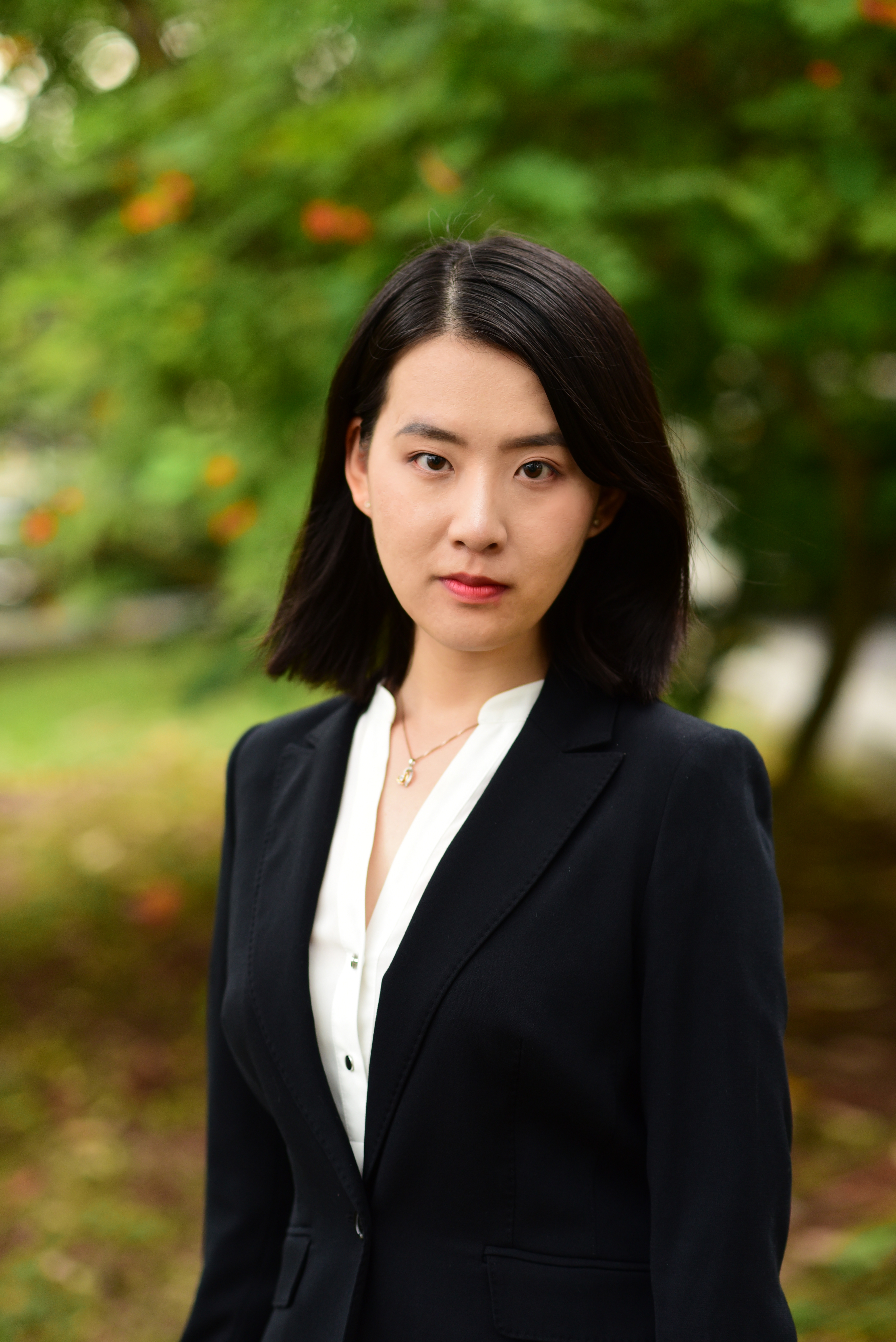
Three Essays on Quality of Tradable Products
This dissertation includes three essays on the quality of tradable products. The first chapter studies the supply-side determinants of quality specialization across Chinese cities. Specifically, we complement the quality specialization literature in international trade and study how larger cities within a country produce goods with higher quality. In our general equilibrium model, firms in larger cities specialize in higher-quality products because agglomeration benefits (arising from the treatment effect of agglomeration and firm sorting) accrue more to skilled workers, who are also more efficient in upgrading quality, although these effects are partially mitigated by higher skill premium in larger cities. Using firm-level data from China, we structurally estimate the model and find that agglomeration and firm sorting each accounts for about 50% of the spatial variation in the quality specialization. A counterfactual policy to relax land use regulation in housing production raises product quality in big cities by 5.5% and indirect welfare of residents by 6.2%. The second chapter examines how information frictions matter in the endogenous choice of product quality made by firms. We introduce quality choice into a trade-search model with information frictions Allen (2014). In our model, producers must search to learn about the quality-augmented price index elsewhere and decide whether to enter a specific destination market. Hence, a fall in information frictions such as the building of information and communications technologies infrastructure (i.e., faster mobile networks) will induce quality upgrading. We empirically test the predictions of our model using unit value data and variation in information and communications technologies infrastructure across Chinese cities. The third chapter provides empirical evidence on the effects of falling trade costs on product quality across cities within a country. We approach this question in the context of expanding the highway system in China in the past decades, which substantially reduces the trade costs across regions within the nation. Empirically, we combine two firm-level panels that provide unit-value information of products across Chinese cities with city-level data on transportation infrastructure for 2001-2007. We find that firms choose to upgrade product quality more in cities with a greater expansion of connecting highways. In addition, this effect is more pronounced in larger cities, which speaks to changes in the spatial concentration of higher-quality products. These results are also robust to the inclusion of an exhaustive battery of fixed effects and to changes in estimation specifications. Our findings shed important insights on the impact of falling intranational trade cost on quality specialization pattern across cities, which is difficult to model quantitatively due to the presence of agglomeration and sorting.
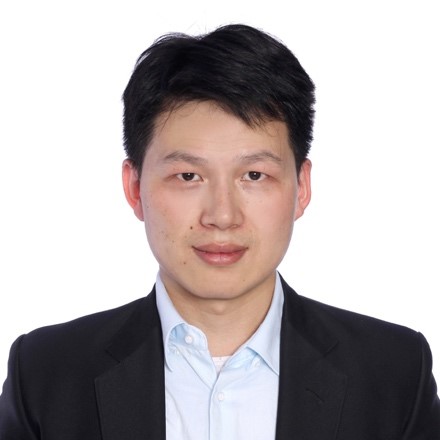
Essays on Agricultural Commodity Processing
This dissertation investigates two important issues in agricultural commodity processing: (i) biomass commercialization; that is, converting organic waste into a saleable product, from economic and environmental perspectives, and (ii) optimal procurement portfolio design using multiple suppliers and spot market, and the impact of by-product introduction on this optimal portfolio.
The first chapter examines the economic implications of biomass commercialization from the perspective of an agri-processor that uses a commodity input to produce both a commodity output and biomass. We characterize the value of biomass commercialization and perform sensitivity analysis to investigate how spot price uncertainty (input and output spot price variabilities and the correlation between the two spot prices) affects this value. We find that commercializing biomass makes the profits less sensitive to changes in spot price uncertainty. Using a model calibration in the context of palm industry, we show that the value of biomass (palm kernel shell) commercialization can be as high as 26.54% of the processor (palm oil mill)’s profits.
The second chapter examines the environmental implications of biomass commercialization. To this end, we characterize the expected carbon emissions considering the profit-maximizing operational decisions using the economic model of the first chapter. In comparison with the common perception in practice, which fails to consider the changes in operational decisions after commercialization, we identify two types of misconceptions (and characterize conditions under which they appear). In particular, the processor would mistakenly think that commercializing its biomass is environmentally beneficial when it is not, and vice versa. Using a model calibration, we show that the former misconception is likely to be observed in the palm industry. we perform sensitivity analyses to investigate how a higher biomass price or demand (which is always economically superior) affects the environmental assessment and characterize conditions under which these changes are environmentally superior or inferior. Based on our results, we put forward important practical implications that are of relevance to both agri-processors and policy makers.
The third chapter studies the procurement portfolio design of an agri-processor that sources a commodity input from two suppliers that use quantity flexibility contracts---characterized by reservation cost and exercise cost---to produce and sell a commodity output under input and output spot price uncertainties. We characterize the optimal procurement portfolio that is composed of three strategies---single sourcing from the supplier with lower reservation price, and single sourcing from the supplier with lower exercise price, and dual sourcing. We investigate how the spot price correlation shapes the optimal procurement strategy and the value of using suppliers. We then study the impact of introducing a non-commodity by-product on the optimal procurement portfolio. Based on our results, we put forward important managerial implications about the procurement strategy and by-product management in agricultural processing industries.
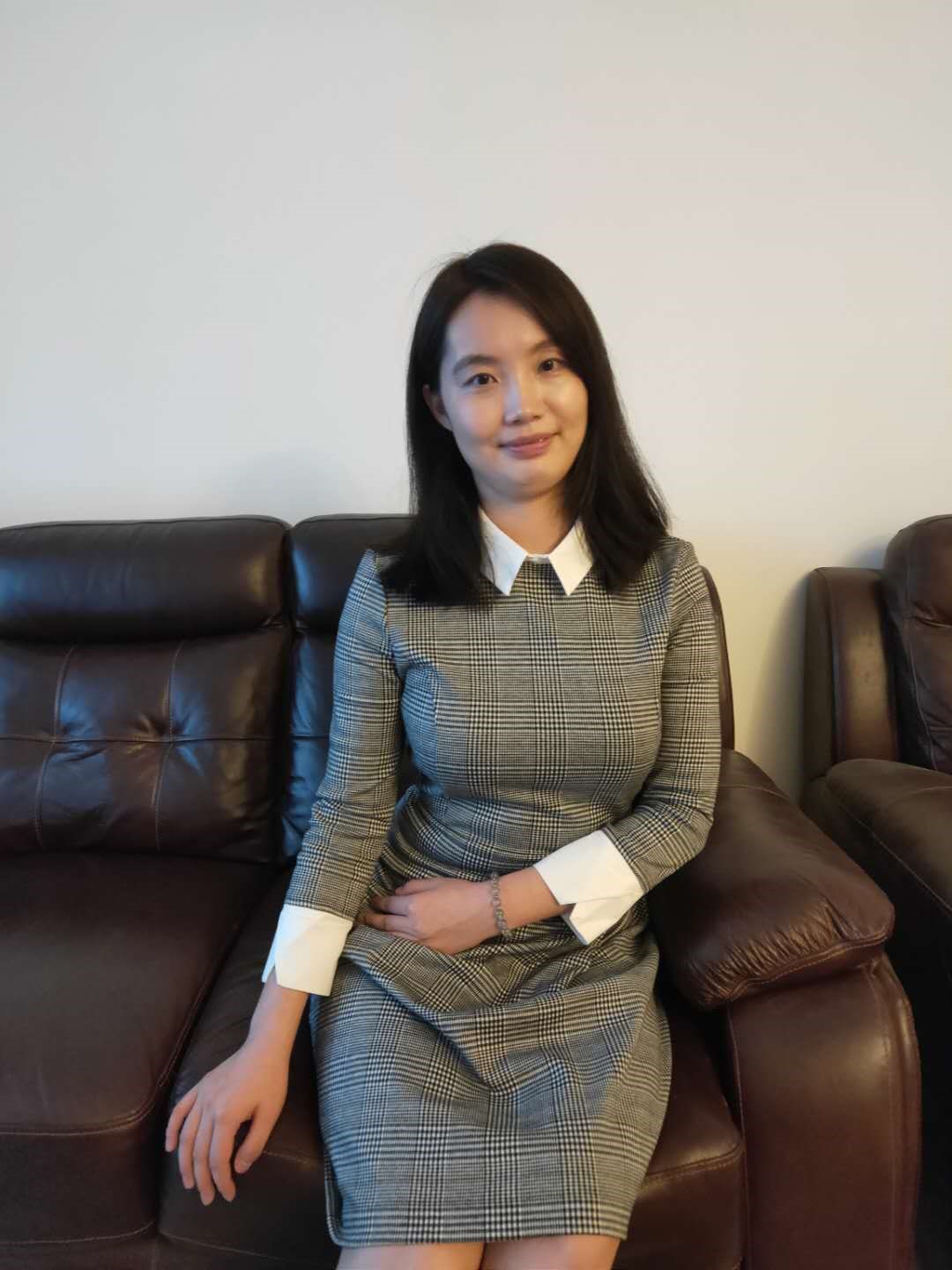
This dissertation studies the capacity investment decision of a manufacturing firm facing demand uncertainty in the presence of shortage possibility in production resources, as often ignored in the literature. These production resources can be physical resources (component / raw material) or financial resources (working capital / budget). The shortage in these resources can be caused by a variety of supply chain disruptions; examples include global disruptions like COVID-19 and financial crisis in 2008 and local disruptions like shortage of components/workforce. The dissertation analyses two important issues related to capacity management: (i) the effect of production resource disruption on the capacity investment strategy and the profitability of the firm (including the significance of profitability loss incurred when the resource shortage possibility is ignored, and (ii) the role of production resource disruption management strategies, i.e. using pre-shipment financing to mitigate the effect of financial resource disruption and using hedging to mitigate physical resource disruption.
The first part examines a two-stage capacity-production framework that capacity investment decision is in anticipation of demand and production resource uncertainties and production quantity is decided after the revelation of uncertainties. I characterize the optimal decisions and investigate how the uncertainties (demand and production resource variability and the correlation between the two) affect the optimal capacity investment level and profitability.
My results provide a rule of thumb for the managers in capacity management. I also study the significance of profitability loss incurred when the resource uncertainty is ignored in choosing a capacity level. Through both analytical and extensive numerical analysis, I show that the profitability loss is high when 1) correlation is high; 2) either production resource variability is sufficiently high or sufficiently low; and 3) either demand variability is sufficiently high or sufficiently low.
The second part examines the role of pre-shipment finance in managing financial production resource (working capital/budget) disruption. Pre-shipment finance allows the firm to transfer the purchase orders (which will be paid after production) to an external party that provides immediate cash flow (at a cost) that can be used for financing the production process. To this end, I characterize the optimal pre-shipment finance level (proportion of sales revenues transferred) and the production volume in the production stage and the optimal capacity investment level in the capacity stage. I make comparisons with the results in the first chapter to understand how pre-shipment financing alters the effects of demand and production resource uncertainties on the optimal capacity investment level, expected profit and profitability loss due to ignoring resource uncertainty. I identify that applying pre-shipment finance makes the capacity investment and profits more resilient to changes in spot price uncertainty.
The third part studies the role of procurement hedging contract in managing physical production resource (e.g., component/raw material) disruption. With the hedging contract, the firm can engineer the production resource uncertainty at the capacity investment stage—for example, with full hedging this uncertainty can be completely removed. I provide the joint characterization of the optimal hedging level and capacity investment decisions. I find that these decisions critically depend on the covariance between demand and production resource uncertainties and the unit capacity investment cost. For example, I find that fully hedging is always optimal when the correlation is non-positive. I highlight conditions under which the firm optimally does not hedge at all or use partial hedging strategy. I then investigate the significance of the profitability loss due to i) misspecification of capacity level by ignoring production resource uncertainty and ii) misspecification of hedging strategy (using full hedging which is easy to implement), and provide conditions under which these profitability losses are significant.
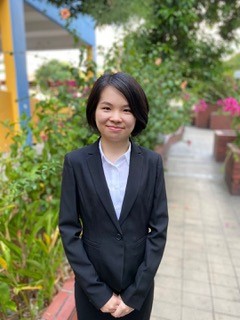
Essays on a Mechanism Design Approach to the Problem of Bilateral Trade and Public Good Provision
The dissertation consists of three chapters which studies a mechanism design approach to the problem of bilateral trade and public good provision.
Chapter 1 characterizes mechanisms satisfying Bayesian incentive compatibility (BIC) and interim individual rationality (IIR) in the classical public good provision problem. We propose a stress test for the results in the standard continuum type space by subject- ing them to a finite type space. The main contribution of this paper is to propose a set of techniques that allow us to characterize the efficient and optimal mechanisms in a discrete setup. Using these techniques, we conclude that many of the known results gained within the standard continuum type space also hold when it is replaced by a discrete type space.
Chapter 2 seeks for more positive results by employing two-stage mechanisms (Mezzetti (2004)), as efficient, voluntary bilateral trade is generally not incentive compatible in an interdependent-value environment (Fieseler, Kittsteiner, Moldovanu (2003) and Gresik (1991)). First, we show by means of a stylized example that the generalized two-stage Groves mechanism never guarantees voluntary trade, while it satisfies efficiency and in- centive compatibility. In a general environment, we next propose Condition α under which there exists a two-stage incentive compatible mechanism implementing an effi- cient, voluntary trade. Third, within the same example, we confirm that our Condition α is very weak because it holds as long as the buyer’s degree of interdependence of preferences is not too high relative to the seller’s counterpart. Finally, we show by the same example that if Condition α is violated, our proposed two-stage mechanism fails to achieve voluntary trade.
Chapter 3 clarifies how the interdependence in valuations and correlation of types across agents affect the possibility of efficient, voluntary bilateral trade in a model with discrete types, as efficient, voluntary bilateral trades are generally not incentive compat- ible in a private-value model with independently distributed continuous types (Myerson and Satterthwaite (1983)). First, we identify a necessary condition for the existence of in- centive compatible mechanisms inducing an efficient and voluntary trade in a finite type model. Second, we show that the identified necessary condition becomes sufficient for a two-type model. Using this characterization in a model with linear valuations and two types, we next conduct the comparative statics for how possibility results rely on the inter- dependence and correlation. Third, using the linear programming approach, we establish the general existence of an efficient, incentive compatible trade in a model with two types. This suggests that voluntary trade becomes a stringent requirement in an interdependent values model with correlated signals.
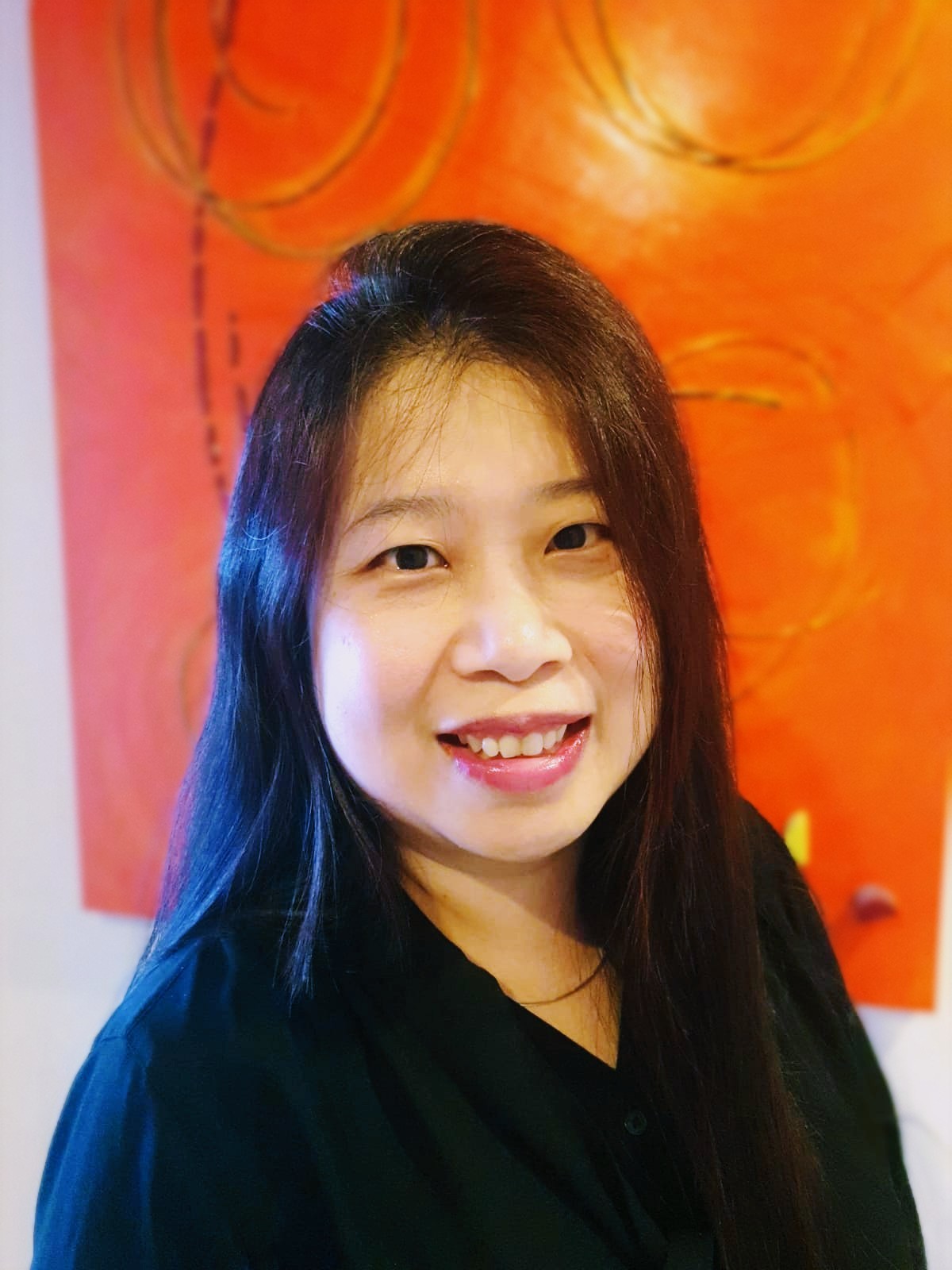
Raising Funds in the Era of Digital Economy
The rapid advancement in technology and internet penetration have substantially increased the number of economic transactions conducted online. Platforms that connect economic agents play an important role in this digital economy. The unbridled proliferation of digital platforms calls for a closer examination of the factors that could affect the welfare of the increasing number of economic agents who participate in them.
This dissertation examines the factors that could affect the welfare of agents using the setting of a crowdfunding platform where fundraisers develop campaigns to solicit funding from potential donors. These factors can be broadly categorized into three distinct groups: (1) campaign and its corresponding fundraiser characteristics, (2) other factors within the platform, and (3) other factors outside the platform. The first group of factors has been examined in a large number of studies. The second and third groups, which encompass factors external to the campaigns and fundraisers remain under-explored and therefore are the focus of this dissertation.
The first essay in this dissertation explores a factor within the platform; how displaying certain campaigns more prominently on the platform affects the performance of other campaigns. Such selective prominent practice is often viewed negatively because it is perceived to place less prominent sellers at a disadvantage (Kramer & Schnurr, 2018). The findings from the first essay provide a counterpoint to this popular view by documenting a positive spill-over effect from an increase in the performance of the prominent campaigns. In particular, when the prominent campaigns perform well, market expansion occurs with more donors entering the platform, benefiting the less prominent campaigns. These findings mitigate the concern that non-neutral practices on digital platforms naturally lead to the rich getting richer and the poor getting poorer.
The second essay explores a factor external to the platform; how public statements from a government official affect private donations to charitable crowdfunding campaigns. A clear pattern of ethnic homophily among fundraisers and donors, where Hispanic fundraisers receive disproportionately more donations from Hispanic donors, is observed in this setting. This pattern of homophily becomes stronger following statements from President Donald Trump. This essay documents how social media usage, particularly by a government official, can influence the dynamic within and across ethnic groups. In sum, the findings from the two essays help inform platform designers, policymakers, and government officials of the potential effects of their actions on the digital economy.
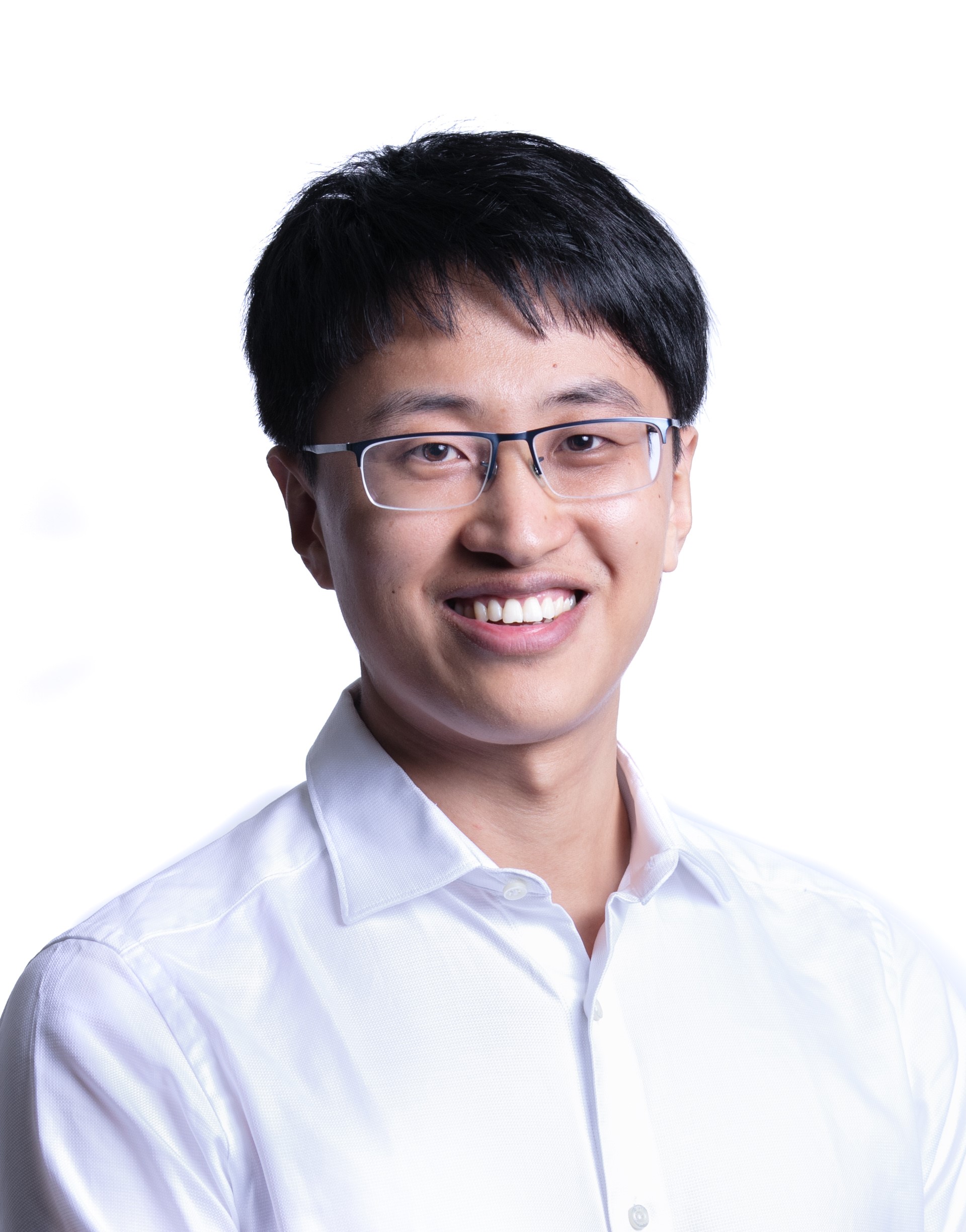
Stock Market Information and Security Prices
Chapter 1: Analyst report content and stock market anomalies A series of recent papers document that security analyst recommendations tend to contradict stock-mispricing signals. This seems at odds with the large prior literature on the investment value of analyst recommendations. What justifications do analysts make when they write reports on mispriced stocks? I use the latest techniques in machine learning and textual analysis to categorize the qualitative information in a large sample of analyst reports. I find that report content can be intuitively classified into five categories or topics: 1) Growth, 2) Earnings, 3) New developments, 4) Management transactions, and 5) Conviction. I then relate the frequency of each topic and the tone surrounding the topic to stock-anomaly mispricing signals. I find that although analysts are incorrectly optimistic about overvalued stocks in general, reports on new developments and management transactions have investment value after controlling for the predictive power of the mispricing signals. For undervalued stocks, while analysts are on average incorrectly pessimistic, reports on growth, new developments, and management transactions have investment value. Overall, this paper helps to understand how analysts provide value in their reports even when the report ratings appear to contradict well-known signals of mispricing.
Chapter 2: The information cycle and return seasonality (with Roger Loh) Heston and Sadka (2008) find that the monthly cross-sectional returns of stocks depend on their historical same calendar-month returns. We propose an information-cycle explanation for this seasonality anomaly—that firms’ seasonal release of information coincide with higher returns during months with such dissolution of information uncertainty, and lower returns during months with no information releases. Using earnings announcements and changes in implied volatility as proxies for scheduled information releases, we find that seasonal winners in information-release months and seasonal losers in non-information release months indeed drive the seasonality anomaly. Our evidence shows that scheduled firm-level information releases can give rise to the appearance of an anomalous seasonal pattern when stock returns are in fact responding to information uncertainty.
Chapter 3: Managerial and analyst horizons during conference calls It is alleged that public-firm managers face short-term pressures from investors. In this paper, I examine managers’ tendency to talk about the short versus the long term by analyzing the language in quarterly analyst conference calls. Using the word embedding model, I determine whether conference calls focus on the short or long term. I find that when firms fail to meet analyst expectations, both managers and analysts focus on the short term rather than the long term. However, in macro bad times, analysts question managers about the short term rather than the long term, while managers maintain the same long term-short term balance whether in good or bad macro conditions. Finally, I show that firms whose conference call participants focus more on the long term have negative initial market reactions, but stock prices recover in the subsequent months. subsequent months. The results are consistent with Wall Street exerting excessive short-term pressures on public firm managers.
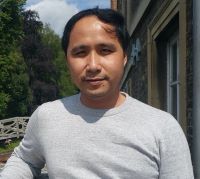
Wearable devices are gaining in popularity, but are presently used primarily for productivity-related functions (such as calling people or discreetly receiving notifications) or for physiological sensing. However, wearable devices are still not widely used for a wider set of sensing-based applications, even though their potential is enormous. Wearable devices can enable a variety of novel applications. For example, wrist-worn and/or finger-worn devices could be viable controllers for real-time AR/VR games and applications, and can be used for real-time gestural tracking to support rehabilitative patient therapy or training of sports personnel. There are, however, a key set of impediments towards realizing this vision. State-of-the-art gesture recognition algorithms typically recognize gestures, using an explicit initial segmentation step, only after the completion of the gesture, thereby being less appropriate for interactive applications requiring real-time tracking. Moreover, such gesture recognition & hand tracking is relatively energy-hungry and requires wearable devices with sufficient battery capacity. Such battery-driven operation further restricts widespread adoption, as (a) the device must be periodically recharged, thereby requiring human intervention, and (b) the battery also adds to the wearable device’s weight, which potentially affects the wearer’s motion dynamics.
In this thesis, I explore the development of new capabilities in wearable sensing along two different dimensions which we believe can help increase the diversity and sophistication of applications and use cases supported by wearable- based systems: (i) Low-latency, low-complexity gesture tracking, and (ii) Ultra-low-power or Battery-less operation. The thesis first proposes the development of a battery-less wearable device that permits tracking of gestural actions by harvesting power from appropriately beamformed WiFi signals. This work requires innovations in both wearable and WiFi AP operations, which work together to support adequate energy harvesting over distances of several meters. Through a combination of simulations and real-world studies, I show that (a) smart WiFi beamforming techniques can help support sufficient energy harvesting by up to 3-4 battery-less devices in a small room, and (b) the prototype battery-less wearable device can support uninterrupted tracking of significant gestural activities by an individual. The thesis then explores the ability of smartwatch to recognize hand gestures early and to track the hand trajectory with low latency, so that it can be used in realizing interactive applications. In particular, I show that our techniques allow a wrist-worn device to be used as a real-time hand tracker and gesture recognizer for an interactive application, such as Table Tennis. The dissertation also demonstrates that my proposed method provides a superior energy-vs-accuracy trade-off compared to more complex gesture tracking algorithms, thereby making it more conducive to operation on battery-less wearable devices. Finally, I evaluate whether my proposed techniques for low- latency gesture recognition can be supported by WiWear-based wearable devices, and establish the set of operating conditions under which such operation is feasible. Collectively, my work advances the state-of-the-art in low-energy wearable-based low-latency gesture recognition, thereby opening up the possible use of battery-less, WiFi-harvesting based devices for gesture-driven applications, especially for sports & rehabilitative training.
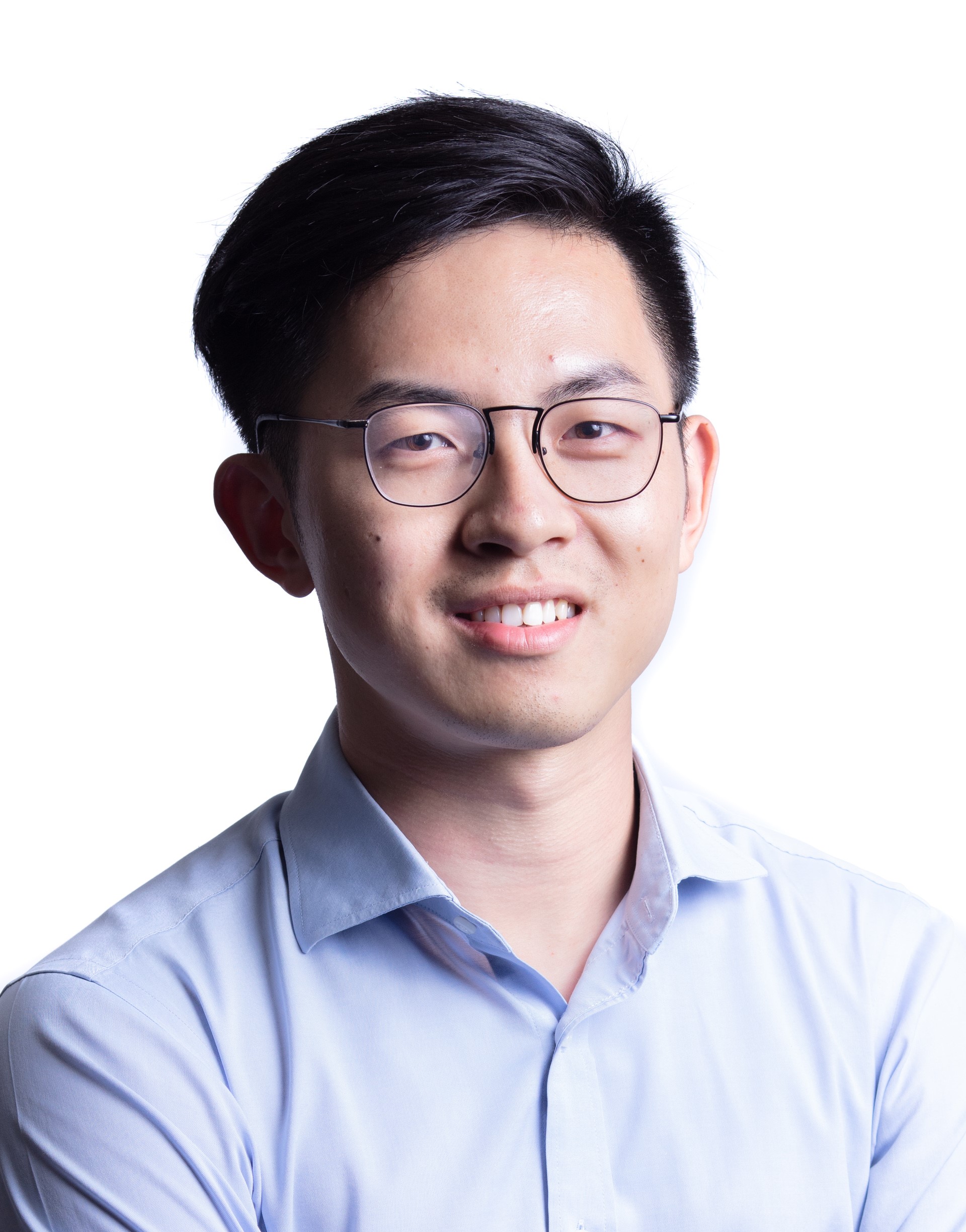
Three Essays on Financial Economics
Disagreement measures are known to predict cross-sectional stock returns but fail to predict market returns. This paper proposes a partial least squares disagreement index by aggregating information across individual disagreement measures and shows that this index significantly predicts market returns both in- and out-ofsample. Consistent with the theory in Atmaz and Basak (2018), the disagreement index asymmetrically predicts market returns with greater power in high sentiment periods, is positively associated with investor expectations of market returns, predicts market returns through a cash flow channel, and can explain the positive volume-volatility relationship.
Dynamic malware analysis schemes either run the target program as is in an isolated environment assisted by additional hardware facilities or modify it with instrumentation code statically or dynamically. The hardware-assisted schemes usually trap the target during its execution to a more privileged environment based on the available hardware events. The more privileged environment is not accessible by the untrusted kernel, thus this approach is often applied for transparent and secure kernel analysis. Nevertheless, the isolated environment induces a virtual address gap between the analyzer and the target, which hinders effective and efficient memory introspection and undermines the correctness of semantics extraction. Code instrumentation mixes the analyzer code together with the target, thus they share the same execution flow as well as the virtual address space at runtime. The instrumentation code has native access capabilities to the target’s virtual memory, which seamlessly introspects and controls the target. However, code instrumentation based schemes are inadequate to tackle malicious execution since the analysis can be detected, evaded, or even tampered with as noted in many recent works.
We securely bridge the virtual address gap by designing a system called the On-site Analysis Infrastructure(OASIS) based on hardware virtualization technology. OASIS features a one-way address space sharing: on the one hand, the analyzer, as an independent full-fledged application, runs in a fused virtual address space comprising both its own space and the target’s; on the other hand, the analyzer’s space is separated and isolated from the target which still runs within its unmodified address space. We also extend OASIS with a significant instrumentation technique which allows secure transitions between the analyzer and the target without precipitating any CPU mode/privilege switch. In total, OASIS offers three capabilities to the analyzer: to reference the target virtual memory in a native way with mapping consistency; to dynamically control and instrument the target execution; and to transparently receive unmodified host OS services. With these capabilities, the analyzer performs onsite analysis on a malicious user/kernel thread running in the guest VM.
We propose two new dynamic analysis models based on OASIS: Onsite Memory Analysis (OMA) and Execution Flow Instrumentation (EFI). In OMA, the analyzer examines the user/kernel thread’s live virtual memory without interposing on its execution. We developed four tools to demonstrate its capability. The first one is a virtual machine introspection tool which is up to 87 times faster than the state of the art. The second one delineates the target’s virtual memory layout without trusting any kernel objects. The third one captures the target’s system call events along with their parameters without intercepting its execution. The last one generates the control flow graph for Just-In-Time emitted code. In EFI, the analyzer is provisioned with two options to directly intercept the user/kernel thread execution and dynamically instrument it. Despite being securely and transparently isolated from the target, the analyzer introspects and controls it in the same native way as the instrumentation code. We have also conducted three case studies. The first one is a cross-space control flow tracer which shows OASIS based EFI has better performance than existing hardware trapping based schemes. The second one works in tandem with Google Syzkaller which demonstrates EFI’s agility in controlling and introspecting the target thread. The last one examines how a user-space program exploits the vulnerability in dynamically loaded kernel modules. EFI tools are well-suited for targeted and fine-grained analysis.
We have implemented a prototype of OASIS on an x86-64 platform and have rigorously evaluated it with various experiments including performance and security tests. OASIS and its tools remain transparent and effective against targets armed with anti-analysis techniques including packing.
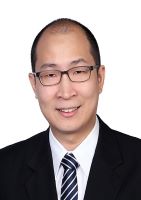
Policy Impact Evaluations on Labor and Health
This dissertation consists of three chapters that evaluate the impacts of public policies on labour and health.
The first chapter studies a wage supplement scheme in Singapore, called the Workfare Income Supplement, which targets older low-income workers. I exploit differences in maximum benefits across age and over time to find that increasing benefits generosity encourages labour market participation and selfemployment. I also find improved life satisfaction and happiness among those with low education, who are likely to be eligible for the scheme. These results suggest that wage supplements can ease some burdens of an ageing population.
The second chapter investigates the effects of raising a non-pension retirement age on labour market outcomes and subjective well-being in Singapore. Adopting a difference-in-differences identification strategy, I find an increase in employment and a decrease in retirement of older workers. Additional analyses suggest that mental anchors may be an important mechanism. I also find improved satisfaction with life as whole and with health, especially among those who are less educated, less prepared for retirement or dissatisfied with household income.
The third chapter examines heterogeneous health effects of medical marijuana legalization on young adults in the United States. Using a difference- in-differences approach accounting for spatial spill-over, I find that states with stricter regulations generate health gains, but not states with lax access to marijuana. Subsample analysis reveal that subgroups such as Blacks, individuals from lower-income households and the uninsured experience larger gains under strict regulations. However, the low-educated, individuals from lower-income households and the uninsured are more likely to suffer worse health under lax regulations.
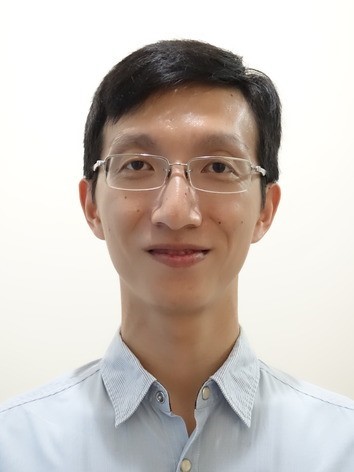
The first essay is about how high and moderate aspiration levels compare in terms of affecting the decision making and reinforcement learning in an uncertain environment. After developing a thought experiment and a computational model, I used lab experiments to test the model’s predictions: a high (moderate) aspiration level reduces (increases) feedback ambiguity about the relative attractiveness of different options, thus increases the exploitation (exploration) tendency of the decision maker. The behavioural difference suggests that high aspirations lead to better performance in stable environments, but worse performance after disruptive shocks. The second essay investigates whether organizations should commit more (or less) to exploration in response to an increased environmental dynamism. Using a computational model, I address the literature contradictions by disentangle exploration intensity and width. I demonstrate that the phenomenon of “chasing a moving target” (Posen & Levinthal, 2012) – the decreasing optimal exploration level under increased environmental dynamism – is caused by the entanglement of exploration intensity and width. The third essay addresses the question about how ambiguous performance feedback across organizational levels affects resource allocation. Attribution theory suggests organizations and organizational members will attribute success internally while attributing failures externally, resulting different learning and response patterns following organizational success and failure. Using professional basketball data, I demonstrate the resources (minutes) allocated to players are subject to the players prior performance. Team performance (game win) positively moderates the relationship between allocated resource and a player’s performance. The moderating effect is the weakest when the team experience a loss with large point-deficit.
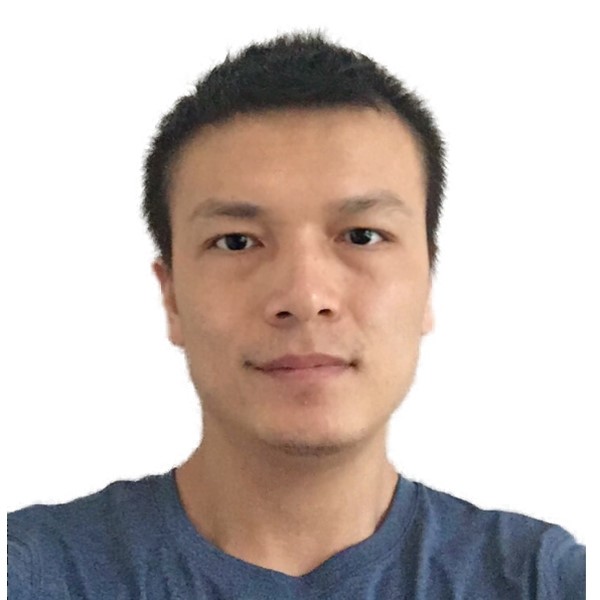
This dissertation two issues related to business ethics: how corporate social responsibility (CSR) affects the value creation in an acquisition and how corporate decoupling behaviors are driven by the CEO narcissism, consisting of two essays. The first essay examines how target corporate social responsibility affects the economic gains for acquirers, as reflected in market reaction to acquisition announcement, from two distinct perspectives: stakeholder preservation versus stakeholder appropriation. The stakeholder preservation perspective suggests that positive market reaction to an acquisition stems from potential new value creation by honoring implicit contracts and maintaining good relationships with target stakeholders. By contrast, the stakeholder appropriation perspective posits that positive market reaction is primarily derived through wealth transfer to acquirers by defaulting on implicit contracts with target stakeholders. Findings from this essay indicate that target CSR is positively associated with acquirer abnormal returns upon acquisition announcement. Moreover, stakeholder value congruence between the merging firms strengthens this positive relationship, whereas business similarity between them weakens it. These findings align with the stakeholder preservation perspective and challenge the stakeholder appropriation perspective. The second essay investigates antecedents of corporate decoupling behaviors from the perspective of CEO attributes. This essay is conducted in the context of corporate buyback program. Corporate decoupling happens when a firm announces a buyback policy but does not implement the buyback program. Findings from this essay suggest that there is a positive relationship between CEO narcissism and buyback policy adoption whereas, following a buyback policy adoption, there is a negative relationship between CEO narcissism and buyback program implementation. Also, this essay examines the peer influence on a focal firm’s buyback practice and finds that peer buyback policy adoption will weaken the relationship between CEO narcissism and firm buyback policy adoption. In addition, the buyback policy adoption initiated by more narcissistic CEOs receives less favorable stock market reactions.
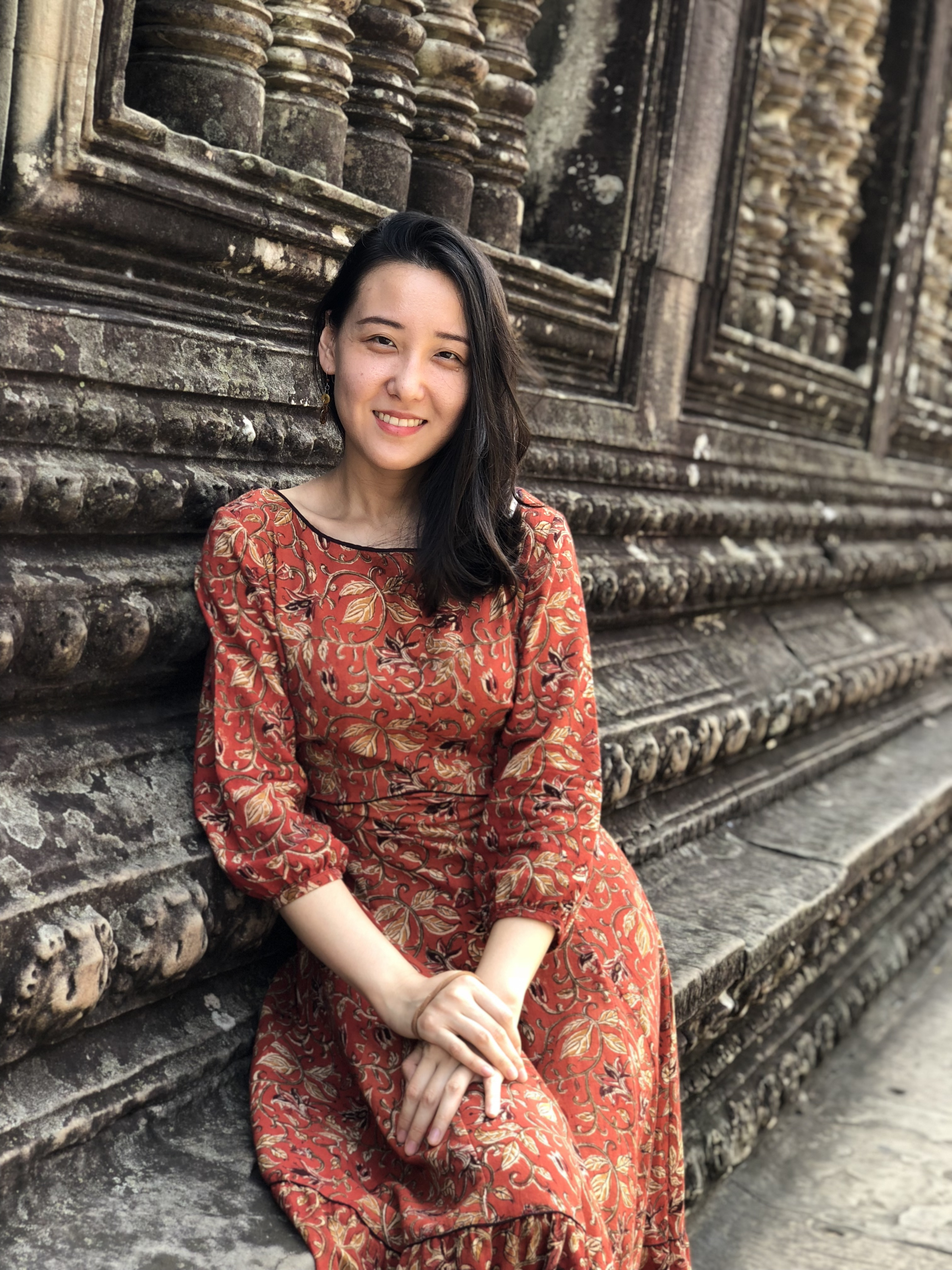
In this paper, M-estimation and inference methods are developed for spatial dynamic panel data models with correlated random effects, based on short panels. The unobserved individual-specific effects are assumed to be correlated with the observed time-varying regressors linearly or in a linearizable way, giving the so-called correlated random effects model, which allows the estimation of effects of time-invariant regressors. The unbiased estimating functions are obtained by adjusting the conditional quasi-scores given the initial observations, leading to M-estimators that are consistent, asymptotically normal, and free from the initial conditions except the process starting time. By decomposing the estimating functions into sums of terms uncorrelated given idiosyncratic errors, a hybrid method is developed for consistently estimating the variance-covariance matrix of the M-estimators, which again depends only on the process starting time. Monte Carlo results demonstrate that the proposed methods perform well in finite sample.
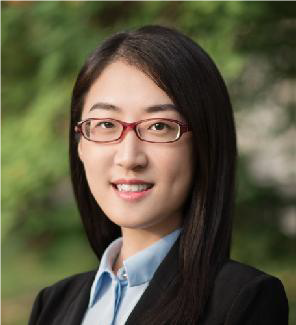
Essays on Empirical Asset Pricing
The dissertation consists of four chapters on empirical asset pricing. The first chapter reexamines the existence of time-series momentum. Time-series momentum (TSM) refers to the predictability of the past 12-month return on the next one month return. Using the same data set as Moskowitz, Ooi, and Pedersen (2012) (MOP, henceforth), we show that asset-by-asset time-series regressions reveal little evidence of TSM, both in- and out-of-sample. While the t -statistic in a pooled regression appears large, it is not statistically reliable as it is less than the critical values of parametric and nonparametric bootstraps. From an investment perspective, the performance of the TSM strategy is virtually the same as that of a similar strategy that is based on the historical sample mean and does not require predictability. Overall, the evidence on TSM is weak, particularly for the large cross-section of assets.
The second chapter focuses on disagreement, which is regarded as the best horse for behavioral finance to obtain as many insights as classic asset pricing theories. Existing disagreement measures are known to predict cross-sectional stock returns but fail to predict market returns. We propose a disagreement index by aggregating information across individual measures using the partial least squares (PLS) method. This index significantly predicts market returns both in- and out-of-sample. Consistent with the theory in Atmaz and Basak (2018), the disagreement index asymmetrically predicts market returns with greater power in high sentiment periods, is positively associated with investor expectations of market returns, predicts market returns through a cash flow channel, and can explain the positive volume-volatility relationship.
The third and fourth chapters investigate the impacts of political uncertainty. We focus on one type of political uncertainty, partisan conflict, which is caused by the dispute or disagreement among party members or policymakers. Chapter 3 finds that partisan conflict positively predicts stock market returns, controlling for economic predictors and proxies for uncertainty, disagreement, geopolitical risk, and political sentiment. A one-standard-deviation increase in partisan conflict is associated with a 0.54% increase in next month's market return. The forecasting power is symmetric across political cycles and operates via a discount rate channel. Increased partisan conflict is associated with increased fiscal policy and healthcare policy uncertainties, and leads investors to switch their investments from equities to bonds.
Chapter 4 shows that intensified partisan conflict widens corporate credit spreads. A one standard deviation increase in partisan conflict is associated with a 0.91% increase in the next one-month corporate credit spreads after controlling for bond-issue information, firm characteristics, macroeconomic variables, uncertainty measures, and sentiment measures. The result holds when using the instrumental variables to resolve endogeneity concerns. I further find that partisan conflict has a greater impact on corporate credit spreads for firms with higher exposure to government policies, including government spending policy and tax policy, and for firms with higher dependence on external finance. Firms that are actively involved in political activities are also more sensitive to changes in political polarization.
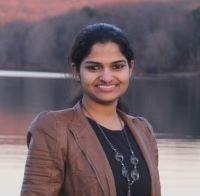
The widespread availability of sensors on personal devices (e.g., smartphones, smartwatches) and other cheap, commoditized IoT devices in the environment has opened up the opportunity for developing applications that capture and enhance various lifestyle-driven daily activities of individuals. Moreover, there is a growing trend of leveraging ubiquitous computing technologies to improve physical health and wellbeing. Several of the lifestyle monitoring applications rely primarily on the capability of recognizing contextually relevant human movements, actions and gestures. As such, gesture recognition techniques, and gesture-based analytics have emerged as a fundamental component for realizing personalized lifestyle applications.
This thesis explores how such wealth of data sensed from ubiquitously available devices can be utilized for inferring fine-grained gestures. Subsequently, it explores how gestures can be used to profile user behavior during daily activities and outlines mechanisms to tackle various real-world challenges. With two daily activities (shopping and exercising) as examples, it then demonstrates that unobtrusive, accurate and robust monitoring of various aspects of these activities is indeed possible with minimal overhead. Such monitoring can then, in future, enable useful applications (e.g., smart reminder in a retail store or digital personal coach in a gym).
First, this thesis presents the IRIS platform, which explores how appropriate mining of sensors available in personal devices such as a smartphone and a smartwatch can be used to infer micro-gestural activities, and how such activities help reveal latent behavioral attributes of individual consumers inside a retail store. It first investigates how inertial sensor data (e.g., accelerometer, gyroscope) from a smartphone can be used to appropriately decompose an entire store visit into a series of modular and hierarchical individual interactions, modeled as a sequence of in-aisle interactions, interspersed with non-aisle movement. Further, by combining such sensor data from a wrist-worn smartwatch and by deriving discriminative features, the IRIS platform demonstrates that different facets of a shopper’s interaction with individual items (e.g., picking an item, putting an item in trolley), as well as attributes of the overall shopping episode or the store, can be inferred.
This thesis next investigates the possibility of using a wearable-free sensing modality for fine-grained and unobtrusive monitoring of multiple aspects of individuals’ gym exercises. It describes the W8-Scope approach that requires no on-body instrumentation and leverages only simple accelerometer and magnetometer sensors (on a cheap IoT device) attached to the weight stack of an exercise machine to infer various exercise gestures, and thereby identify related novel attributes such as the amount of weight lifted, the correctness of exercise execution and identify the user who is performing the exercise. It then also experimentally demonstrates the feasibility of evolving W8-Scope’s machine learning-based classifiers to accommodate the medium-time scale (e.g., across weeks or months) changes in an individual’s exercise behavior (an issue that has received insufficient attention to date).
Finally, this thesis explores the possibility of accurately inferring complex activities and gestures performed concurrently by multiple individuals in an indoor gym environment. It introduces a system that utilizes a hybrid architecture, combining sensor data from ‘earables’ with non-personal IoT sensors attached to gym equipment, for individual-specific fine-grained monitoring of weight-based exercises in a gym. Using real-world studies conducted with multiple concurrent gym-goers, this thesis validates that accurate association of “user-equipment” pairings is indeed possible, for a majority of common exercises, in spite of the significant signal dampening on the earable. Moreover, it demonstrates how features from the earable and IoT sensors can be combined to significantly increase the accuracy and robustness of exercise recognition. In future, the real-time exercise analytics capabilities developed in this thesis can be used to enable targeted and individualized real-time feedback on user dynamics and increase user engagement.
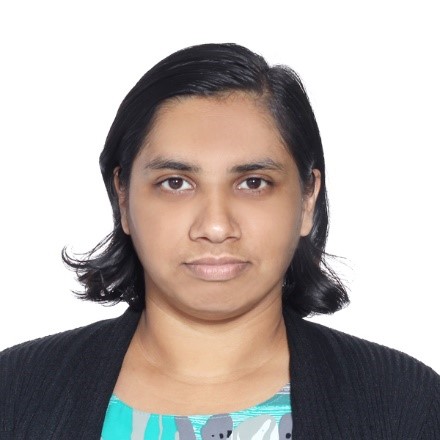
Online Spatio Temporal Demand Supply Matching
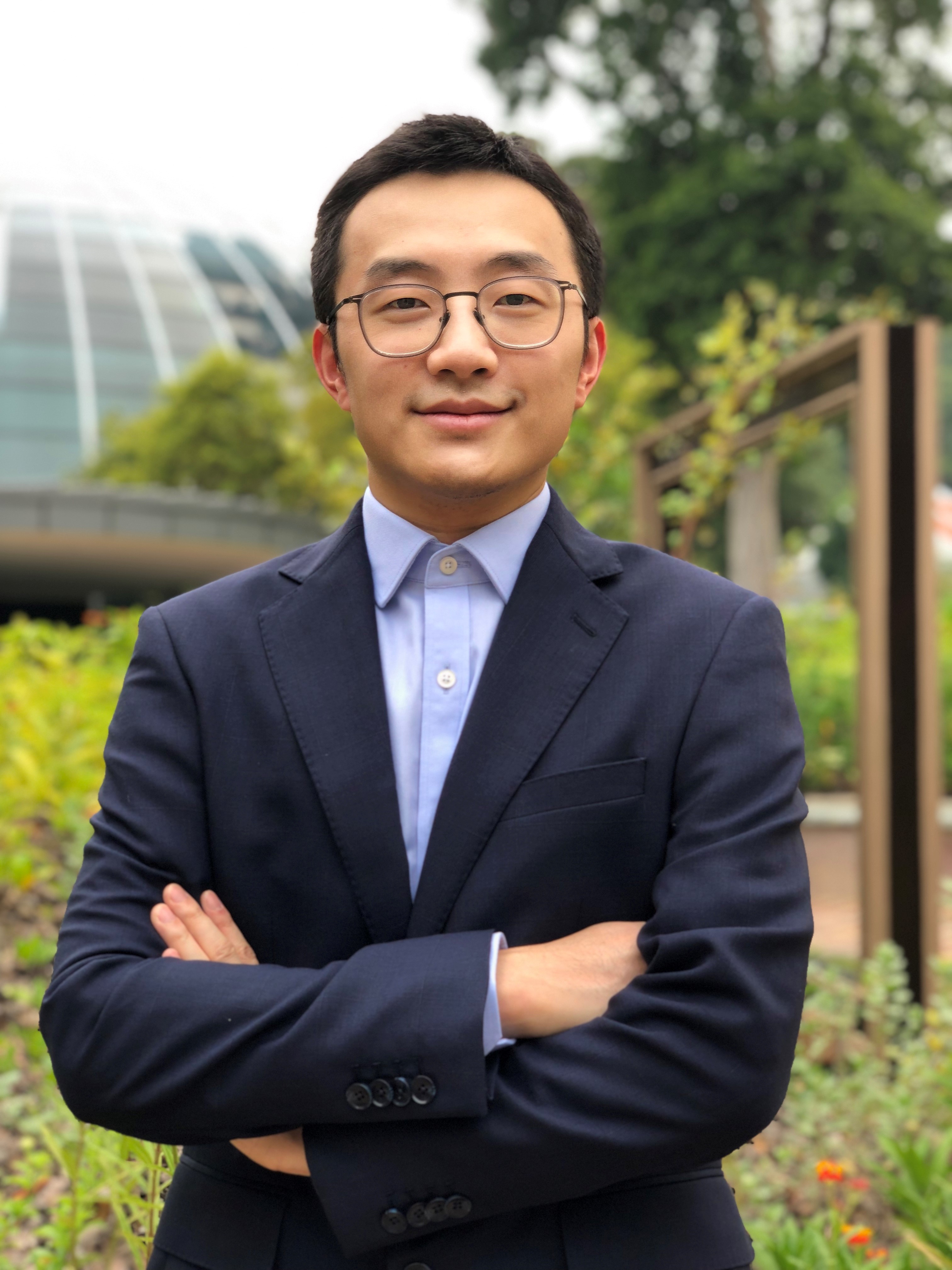
This dissertation consists of three papers which contribute to the estimation and inference theory of the heterogeneous large panel data models. The first chapter studies a panel threshold model with interactive fixed effects. The least-squares estimators in the shrinking-threshold-effect framework are explored. The inference theory on both slope coefficients and the threshold parameter is derived, and a test for the presence of the threshold effect is proposed. The second chapter considers the least-squares estimation of a panel structure threshold regression (PSTR) model, where parameters may exhibit latent group structures. Under some regularity conditions, the latent group structure can be correctly estimated with probability approaching one. A likelihood-ratio-based test on the group-specific threshold parameters is studied. Two specification tests are proposed: one tests whether the threshold parameters are homogeneous across groups, and the other tests whether the threshold effects are present. The third chapter studies high-dimensional vector autoregressions (VARs) augmented with common factors. An L1-nuclear-norm regularized estimator is considered. A singular value thresholding procedure is used to determine the correct number of factors with probability approaching one. Both a LASSO estimator and a conservative LASSO estimator are employed to improve estimation. The conservative LASSO estimates of the non-zero coefficients are shown to be asymptotically equivalent to the oracle least squares estimates. Monte Carlo studies are conducted to check the finite sample performance of the proposed test and estimators. Empirical applications are conducted in each chapter to illustrate the usefulness of the proposed methods.
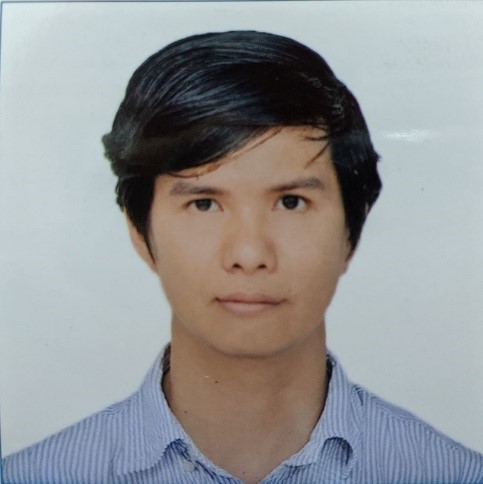
Exploting Approximation, Caching and Specialization to Accelerate Vision Sensing Applications
Over the past few years, deep learning has emerged as state-of-the-art solutions for many challenging computer vision tasks such as face recognition, object detection, etc. Despite of its outstanding performance, deep neural networks (DNNs) are computational intensive, which prevent them to be widely adopted on billions of mobile and embedded devices with scarce resources. To address that limitation, we
focus on building systems and optimization algorithms to accelerate those models, making them more computational-efficient.
First, this thesis explores the computational capabilities of different existing processors (or co-processors) on modern mobile devices. It recognizes that by leveraging the mobile Graphics Processing Units (mGPUs), we can reduce the time consumed in the deep learning inference pipeline by an order of magnitude. We further investigated variety of optimizations that work on the mGPUs for more accelerations and built the DeepSense framework to demonstrate their uses.
Second, we also discovered that video streams often contain invariant regions (e.g., background, static objects) across multiple video frames. Processing those regions from frame to frame would waste a lot of computational power. We proposed a convolutional caching technique and built a DeepMon framework that quickly determines the static regions and intelligently skips the computations on those regions during the deep neural network processing pipeline.
The thesis also explores how to make deep learning models more computational-efficient by pruning unnecessary parameters. Many studies have shown that most of the computations occurred within convolutional layers, which are widely used in convolutional neural networks (CNNs) for many computer vision tasks. We designed a novel D-Pruner algorithm that allows us to score the parameters based on how important they are to the final performance. Parameters with little impacts will be removed for smaller, faster and more computational-efficient models.
Finally, we investigated the feasibility of using multi-exit models (MXNs), which consist many neural networks with shared-layers, as an efficient implementation to accelerate many existing computer vision tasks. We show that applying techniques such as aggregating results cross exits, threshold-based early exiting with MXNs can significantly speed up the inference latency in indexed video querying and face
recognition systems.
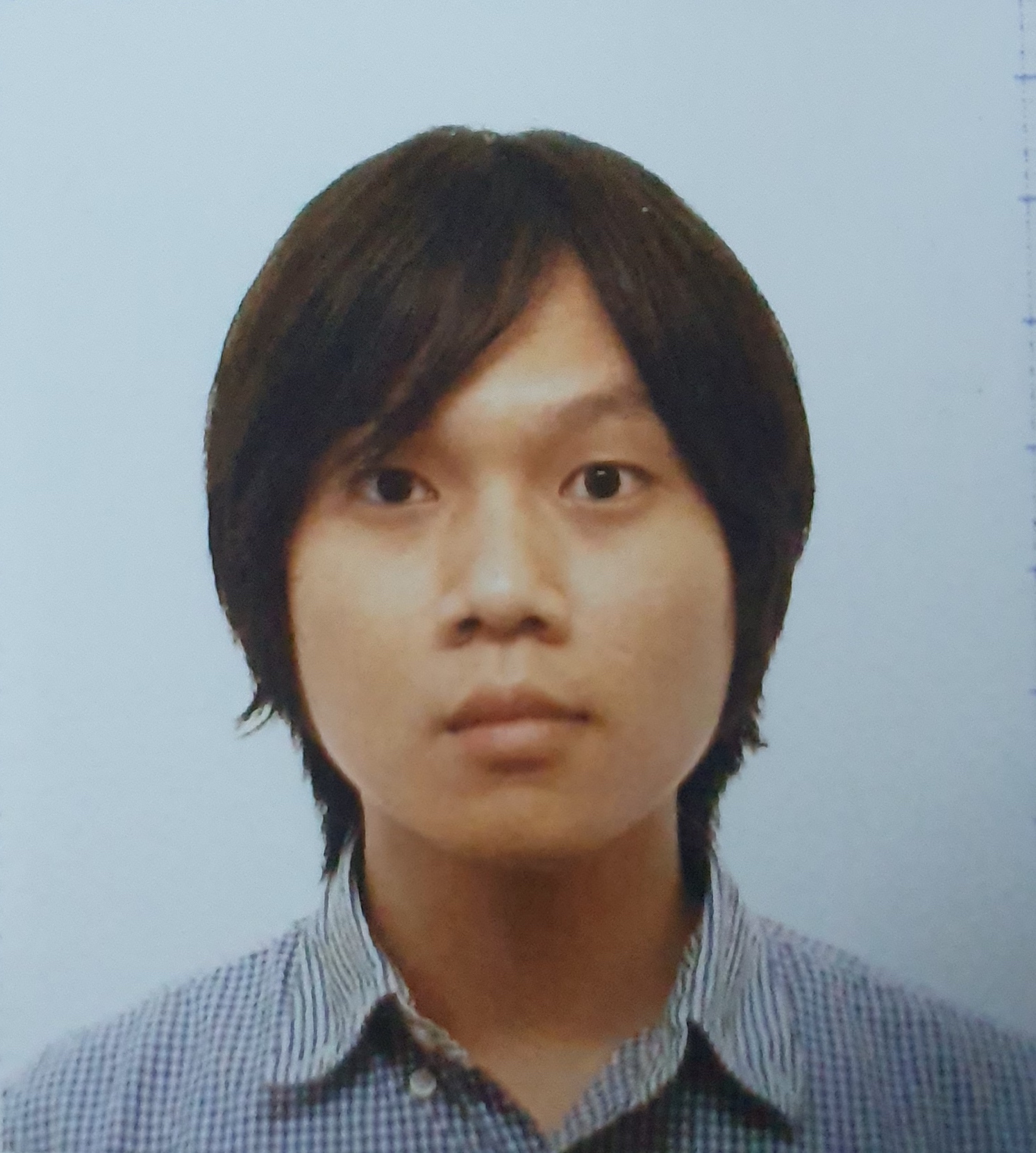
Sensing systems for monitoring physiological and psychological states have been studied extensively in both academic and industry research for different applications across various domains. However, most of the studies have been done in the lab environment with controlled and complicated sensor setup, which is only suitable for serious healthcare applications in which the obtrusiveness and immobility can be compromised in a trade-off for accurate clinical screening or diagnosing. The recent substantial development of mobile devices with embedded miniaturized sensors are now allowing new opportunities to adapt and develop such sensing systems in the mobile context. The ability to sense physiological and psychological state using mobile (and wearable) sensors would make its applications much more feasible and accessible for daily use in different domains such as healthcare, education, security, media and entertainment. Still, there are several research challenges remain in order to develop mobile sensing systems that can monitor users’ physiological signals and psychological conditions accurately and effectively.
This thesis will address three key aspects related to realizing the multimodal mobile sensing systems for physiological and psychological state assessment. First, as the mobile embedded sensors are not designed exclusively for physiological sensing purpose, we attempt to improve the sensing capabilities of mobile devices to acquire the vital physiological signals. Specifically, we study the feasibility of using mobile sensors to measure a set of vital physiological signals, in particular, the cardiovascular metrics including blood volume, heartbeat-to-heart beat interval, heart rate, and heart rate variability. The changes in those physiological signals are essential in detecting many psychological states. Second, we validate the importance of assessing the physiological and psychological states in mobile context across various domains. Lastly, we develop and evaluate a multimodal sensing system to measure engagement level of mobile gamers. While the focus of our study was on mobile gaming scenario, we believe the concept of such sensing system is applicable to improve user experience in other mobile activities, including playing games, watching advertisements, or studying using their mobile devices.
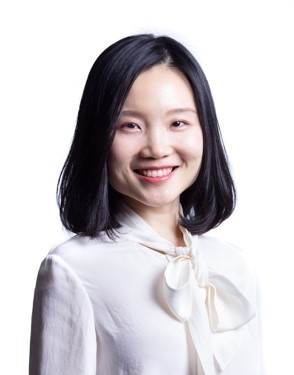
Innovative Business Models in Online Retailing
Internet has opened the door for e-commerce and created a business avenue, online retailing. E-commerce presently shapes the manner in which consumers shop for products. The online retailing markets have grown by 56% during the past five years, while traditional retailing markets are only grown by 2% during the same time. The noticeable growth of online retailing creates numerous opportunities as well as challenges for the context of operations management.
Extensive literature in this domain focus on the conventional inventory management and pricing problems as in traditional retailing. However, the rapid development of information technology threatens the established business models and creates opportunities for new business models. Companies may find it increasingly difficult to make strategic decisions, such as how to deal with the challenge associated with online retailing and how to adapt to the new retailing environment. This thesis aims to investigate innovative business models involved in online retailing, to capture trendy phenomena that are under-studied, and provide managerial insights.
The first chapter focuses on dealing with the logistics challenge caused by the booming e-commerce activities. An urban consolidation center (UCC) or a peer-to-peer platform may alleviate the economic, social and environmental pressure on well-being. We compare the performance of these two business models to guide a consolidator to make efficient operational decisions. The second chapter focuses on the channel management decisions of a retailer who operates an offline (brick-and-mortar) channel and an online channel. The two channels are either operated separately or integrated. We explore how the retailer can profitably integrate her offline and online channels, from a perspective of product descriptions and consumer reviews. The last chapter focuses on a seller's decisions in the process of entering the online market through online marketplaces. In addition to pure-play marketplaces, some marketplaces also sell their own products directly competing with sellers, which creates a new form of channel conflict. We analyze the optimal decisions for both the seller and the marketplaces to characterize the system equilibrium.
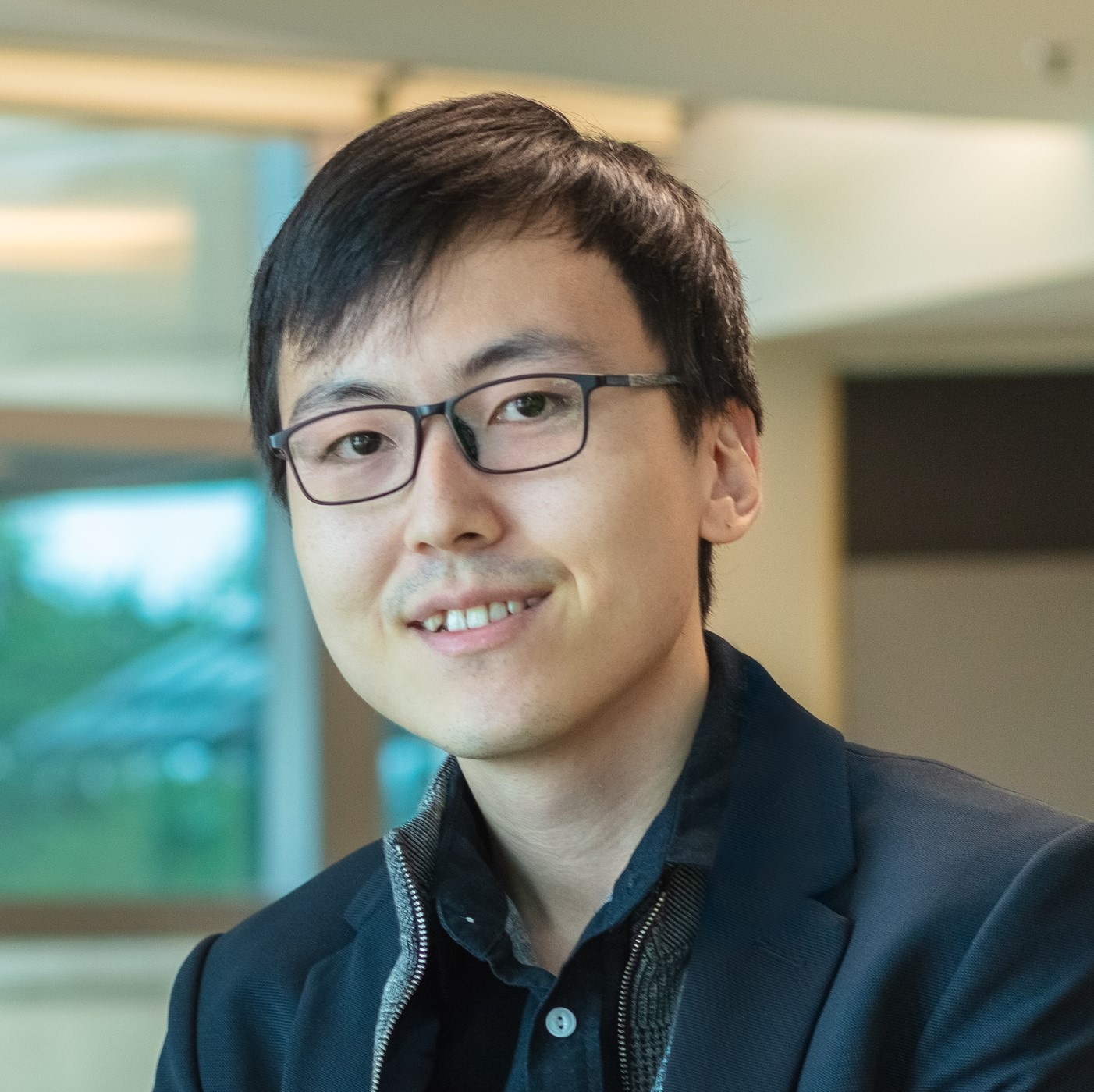
Although creative ideation requires deviating sufficiently from conventional thoughts, people tend to fixate on highly salient and accessible concepts when responding to idea generation tasks. Surmounting such a default tendency then, is crucial to generating creative ideas. Bridging creative cognition with self-regulation research, I hypothesized that inhibitory control over such a default response may require self-regulatory resources. This would suggest that interventions that increase people’s self-regulatory resources may also boost their creativity. However, results from Study 1 did not support this hypothesis. Specifically, there was no significant difference between ego-depleted versus non-depleted participants in terms of inhibitory control over salient concepts (assessed by the newly developed Concept Inhibition Task; CIT) or creative performance. Interestingly, post-hoc findings suggest a moderating relationship between ego-depletion status and inhibitory control, such that higher inhibitory control was associated with increased creativity only for non-depleted participants; the association was otherwise null for depleted participants. Study 2 replicated the null findings of Study 1 and did not support the utility of glucose consumption – an established ego-replenishing intervention – in increasing the creative performance of ego-depleted individuals. Study 3 examined the effectiveness of mindfulness meditation – an established self-regulation boosting intervention – in elevating people’s creativity. Results revealed no significant difference in inhibitory control and creativity between participants who meditated versus those who listened to music (a comparable control group) after a ten-day intervention period. Although improvements to both inhibitory control and creativity were found when comparing baseline to post-intervention levels, such improvements were not unique to those who meditated. Interestingly, Study 3 showed that inhibitory control was positively associated with creativity at both pre- and post-intervention assessments, whereas the association was null for Study 2 where most participants were subjected to ego-depletion. Together, these three studies suggest that self-regulatory resources may not exert a direct impact on inhibitory control over salient concepts and generating creative ideas. Instead, self-regulatory resource levels may modulate the relationship between inhibitory control and creativity, such that only non-depleted individuals may reap creative benefits from inhibiting salient concepts. For ego-depleted individuals, inhibitory control over salient concepts appear to be inconsequential towards their creative performance. This post-hoc finding is explained by considering the dual pathway theory of creative idea generation (Nijstad et al., 2010). Implications and future directions are discussed.
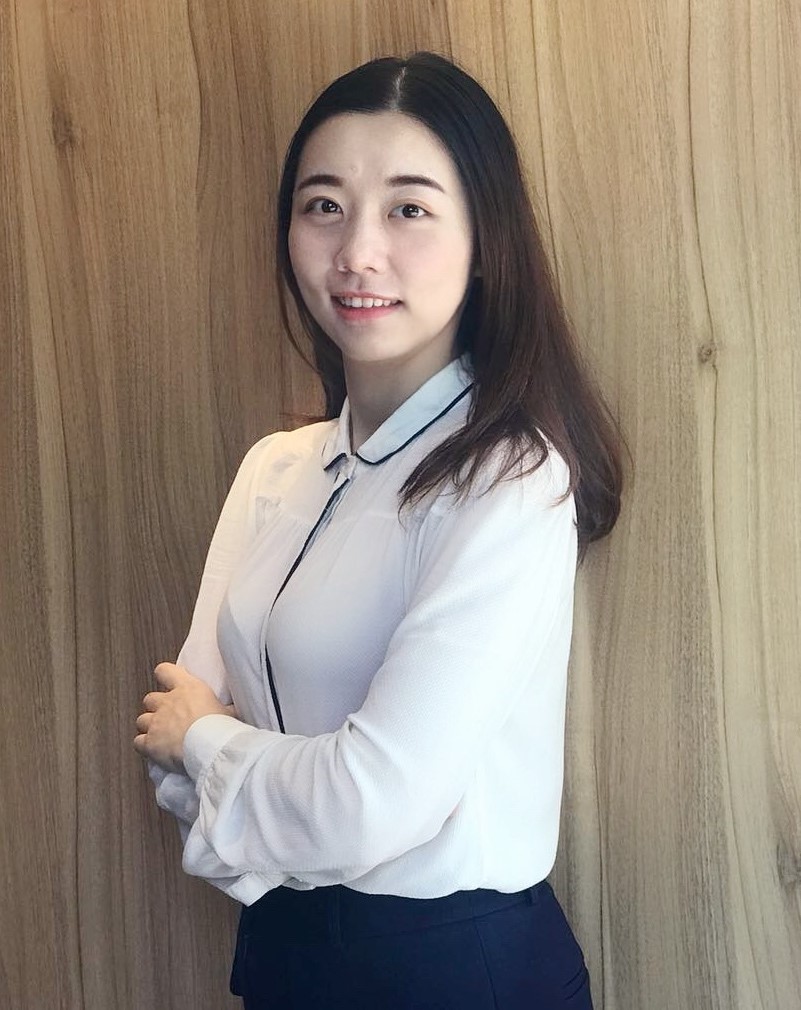
Essays on Corporate Finance
This dissertation has two essays on corporate finance. In the first chapter, I investigate the dual-class structure. The dual-class structure is often regarded as poor corporate governance and the source of agency problems. However, I find that, for companies with high information asymmetry and long investment horizon, dual-class structure delivers higher operating performance and valuation ratios. These performing dual-class companies tend to have a higher investment in intangibles, more innovations, less pay-out, and less CEO compensation. The findings suggest that dual-class structure could be optimal in empowering information-advantageous inside shareholders and ensuring corporate long-term goals.
In the second chapter of my dissertation, we study how air pollution influences firm performance. Air pollution is a growing hazard to human health. This study examines whether air pollution affects the formation of corporate human capital and thereby performance. We find that people exhibit an intention to look for jobs in less polluted areas on days when air pollution occurs in the area where they are located, suggesting that an individual’s sort in response to air pollution. Consistent with this sorting prediction, we find that the level of firms’ skilled executives and employees significantly drops when pollution information becomes real-time accessible and when the pollution level increases in their locations, especially in places where concerns for health is more sensitive to air pollution. Moreover, parallel reductions in firm productivity and value are found and become more salient when firms have a greater dependence on human capital.
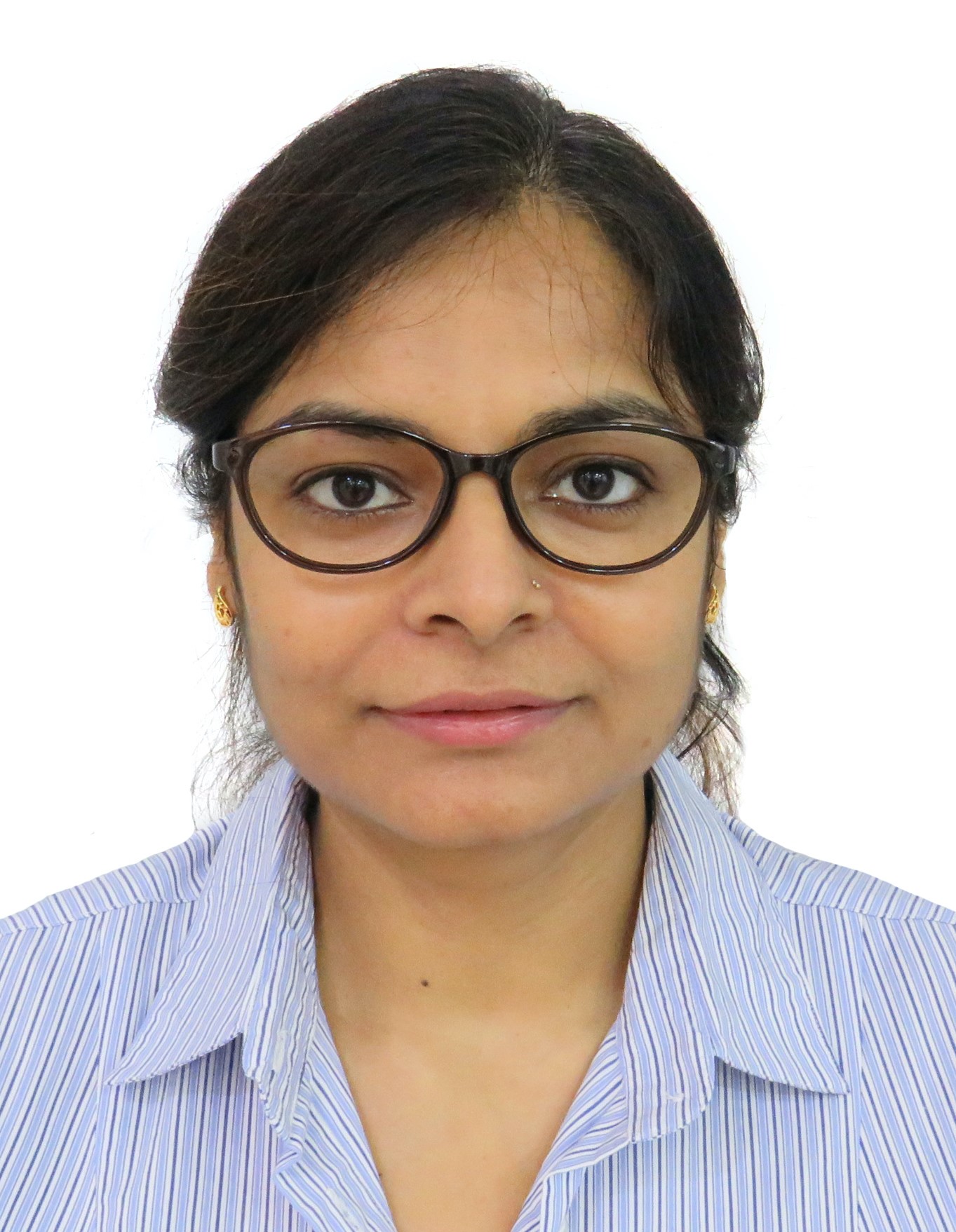
Efficient sequential matching of supply and demand is a problem of interest in many online to offline services. For instance, Uber, Lyft, Grab for matching taxis to customers; Ubereats, Deliveroo, FoodPanda etc. for matching restaurants to customers. In these systems, a centralized entity (e.g., Uber) aggregates supply and assigns them to demand so as to optimize a central metric such as profit, number of requests, delay etc. However, individuals (e.g., drivers, delivery boys) in the system are self interested and they try to maximize their own long term profit. The central entity has the full view of the system and it can learn policies to maximize the overall payoff and suggest it to the individuals. However, due to the selfish nature of the individuals, they might not be interested in following the suggestion. Hence, in my thesis, I develop approaches that learn to guide these individuals such that their long term revenue is maximized. There are three key characteristics of the aggregation systems which make them unique from other multi-agent systems. First, there are thousands or tens of thousands of individuals present in the system. Second, the outcome of an interaction is anonymous, i.e., the outcome is dependent only on the number and not on the identities of the agents. And third, there is a centralized entity present which has the full view of the system, but its objective does not align with the objectives of the individuals. These characteristics of the aggregation systems make the use of the existing Multi-Agent Reinforcement Learning (MARL) methods challenging as they are either meant for just a few agents or assume some prior belief about others. A natural question to ask is whether individuals can utilize these features and learn efficient policies to maximize their own long term payoffs. My thesis research focuses on answering this question and provide scalable reinforcement learning methods in aggregation systems. Utilizing the presence of a centralized entity for decentralized learning in a non-cooperative setting is not new and existing MARL methods can be classified based on how much extra information related to the environment state and joint action is provided to the individual learners. However, presence of a self-interested centralized entity adds a new dimension to the learning problem. In the setting of an aggregation system, the centralized entity can learn from the overall experiences of the individuals and might want to reveal only those information which helps in achieving its own objective. Therefore, in my work I propose approaches by considering multiple combinations of levels of information sharing and levels of learning done by the centralized entity. My first contribution assumes that the individuals do not receive any extra information and learn from their local observation. It is a fully decentralized learning method where independent agents learn from the offline trajectories by considering that others are following stationary policies. In my next work, the individuals utilize the anonymity feature of the domain and consider the number of other agents present in their local observation to improve their learning. By increasing the level of learning done by the centralized entity, in my next contribution I provide an equilibrium learning method where the centralized entity suggests a variance minimization policy which is learned based on the values of actions estimated by the individuals. By further increasing the level of information shared and the level of learning done by the centralized entity, I next provide a learning method where the centralized entity acts as an correlation agent. In this method the centralized entity learns social welfare maximization policy directly from the experiences of the individuals and suggests it to the individual agents. The individuals in turn learn a best response policy to the suggested social welfare maximization policy. In my last contribution I propose an incentive based learning approach where the central agent provides incentives to the individuals such that their learning converges to a policy which maximizes overall system performance. Experimental results on real-world data sets and multiple synthetic data sets demonstrate that these approaches outperform other state-of-the-art approaches both in the terms of individual payoffs and overall social welfare payoff of the system.
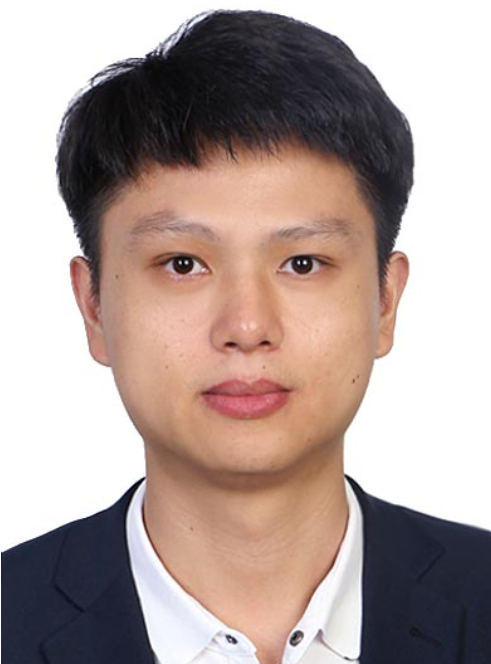
How Does Status Affect Peformance and Learning from Failure? Evidence from Online Communities
This dissertation is composed of two essays. In the first essay, I investigate the factors that can alleviate the detrimental effect of hierarchy on team performance. I first show that hierarchy negatively impacts team performance, which is consistent with recent meta-analytic evidence. One mechanism that drives this negative effect is that hierarchy prevents low-ranking members from voicing their potentially valuable insights. Then I propose that team familiarity is one factor that can encourage low-ranking team members to speak up. I contend that team familiarity can be established either by team members’ prior experience in working with one another or can be built by team members’ prior experience in working in hierarchical teams, such that they are familiar with hierarchical working relationships. Using data collected from an online crowdsourcing contest community, I find that team members’ familiarity with each other and their familiarity with hierarchical working relationships can alleviate the detrimental effect of hierarchy on team performance. By illuminating the moderating effect of team familiarity on the hierarchy-performance relationship, this study advances current understandings of how to reduce the detrimental effect of hierarchy on performance, and offers insights about how teams should be organized to improve performance.
In the second essay, I examine what factors drive learning from failure. In answering this question, I bring status theory into the literature on learning from failure and propose that status can drive people’s learning from their failures. I propose that failure feedback given by a higher-status source is more likely to drive a focal individual to learn from her failures than failure feedback given by a lower-status source. This is because people pay more attention to and are more engaged with failure feedback given by a higher-status source than failure feedback given by a lower-status source. Data collected from an online programming contest community provides support to my prediction that failure feedback given by higher-status peers has stronger effect in driving learning from failure than failure feedback given by lower-status peers. By demonstrating that status is a driver of learning from failure, I expand experiential learning theories by incorporating status theory.
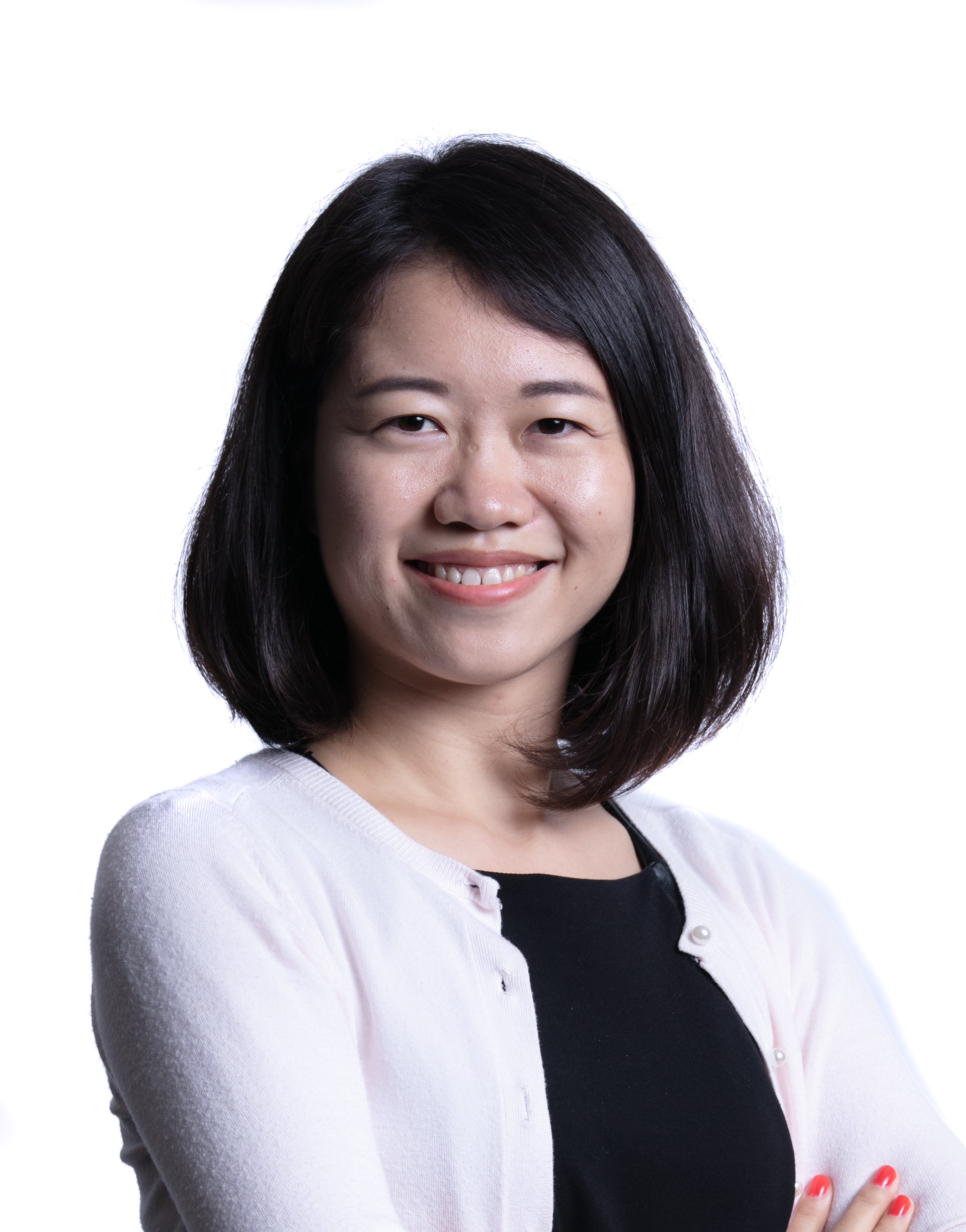
Chapter 1: How institutions enhance mindfulness: interactions between external regulators and front-line operators around safety rules (with Ravi S. Kudesia and Jochen Reb) How is it that some organizations can maintain nearly error-free performance, despite trying conditions? Within research on such high-reliability organizations, mindful organizing has been offered as a key explanation. It entails interaction patterns among front-line operators that keep them attentive to potential failures—and relies on them having the expertise and autonomy to address any such failures. In this study, we extend the mindful organizing literature, which emphasizes local interactions among operators, by considering the broader institutional context in which it occurs. Through interview, observational, and archival data of a high-reliability explosive demolitions firm in China, we find that external regulators can crucially enhance the mindful organizing of front-line operators as regulators and operators interact around safety rules. Regulators go beyond the interactions emphasized in institutional theory, whereby regulators help operators internalize the content of rules and follow the rules in practice. Rather, regulator interactions also help ensure the salience of rules, which enriches and distributes operator attention throughout the firm. We also find evidence of regulator learning, as interactions with operators help regulators improve rule content and the techniques by which rules remain salient. These findings expand our understanding of mindful organizing and the interactional dynamics of institutions. They also particularly speak to the debate over whether and how rules can enhance safety. Namely, through distinct practices that impact the content and salience of rules, regulators can increase standardization without diminishing operator autonomy.
Chapter 2: Entrainment and the temporal structuring of attention: insights from a high-reliability explosive demolitions firm (with Ravi S. Kudesia and Jochen Reb) Attention has always been central to organization theory. What has remained implicit is that attention is a temporal phenomenon. Attention accumulates and dissipates at multiple timescales: it oscillates wavelike within a performance episode, decays gradually over the course of a performance episode, and withdraws in a step-like manner across multiple performance episodes. Organizations attempt to regulate the attention of front-line employees. But to the extent that attention has been examined as a stable phenomenon, rather than a temporal one, metacognitive practices that stabilize attention remain unexamined in organization theory. And to the extent that fluctuations in attention on the front lines generate systemic risks, these unexamined stabilizing practices constitute a core part of organizational reliability. In this case study, we examine a high-reliability explosive demolitions firm. Going beyond past work that identifies best practices shared across organizations, we instead uncover the logic of how several practices are bundled together in a single organization to stabilize front-line attention across these timescales. We uncover distinct bundles of attention regulation practices designed to proactively encourage attention and discourage inattention and to reactively learn from problems, including problems resulting from inattention. We theorize that these practices are bundled according to a logic of entrainment. Practices that proactively regulate the fluctuations of attention over time are mapped onto existing work routines that repeat cyclically across concentrically nested timescales—and reactive practices enhance learning by extracting lessons from mindless behaviors and feeding them back into entrained practice.
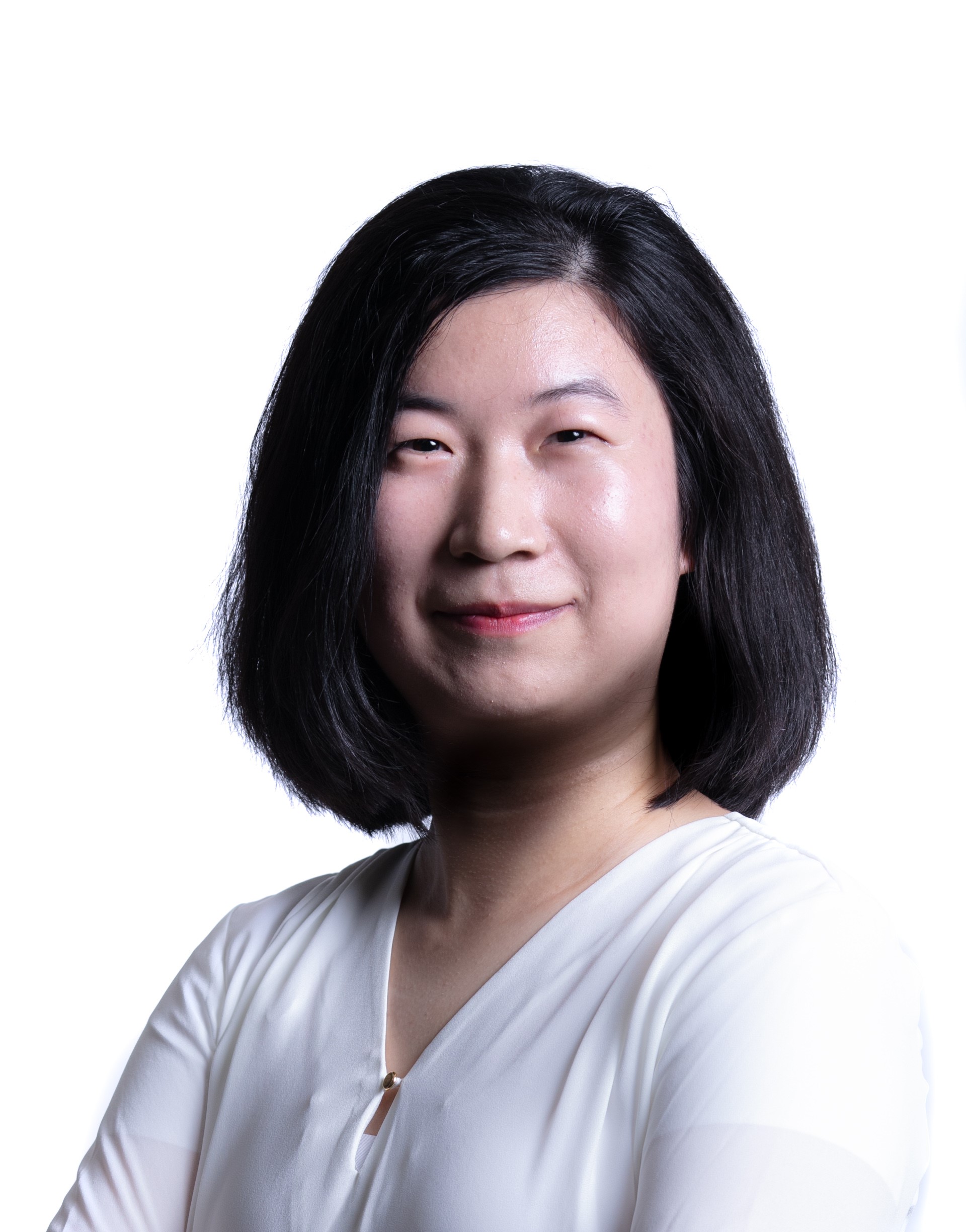
Research and Development (R&D) is time consuming, expensive and risky; yet product life cycles are shortening and competition is fierce. Therefore, R&D often requires the collaboration and input of multiple stakeholders. This dissertation studies how collaborations involving multiple stakeholders can effectively make R&D project portfolio selection decisions to create the optimal social welfare. The two essays in the dissertation build stylized analytical models to examine R&D project portfolio selection in two different settings, academia and industry respectively. The models explicitly acknowledge the different information, goals and operational decisions of the stakeholders. In the first essay, we study a two-stage funding process for university research project selection, with bridge funding by the university first followed by government funding after. We consider different project selection mechanisms by the university corresponding to different strategic missions. We focus on the impact of the university-level selection on government funding and project success and provide recommendations for university funding in terms of policies, objectives and coverage. In the second essay, we look at strategic R&D alliances between two profit-maximizing firms. Specifically, we study how the payment structure and the contract timing affects the project selection decisions of the stakeholders in a strategic alliance, in the presence of an R&D budget constraint, market interactions, and varying levels of bargaining power. We provide recommendations for the effective formation of strategic alliances.
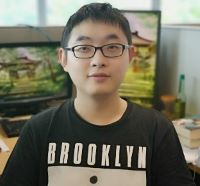
Password is a prevalent means used for user authentication in pervasive computing environments since it is simple to be deployed and convenient to use. However, the use of password has intrinsic problems due to the involvement of keystroke. Keystroke behaviors may emit various side-channel information, including timing, acoustic, and visual information, which can be easily collected by an adversary and leveraged for the keystroke inference. On the other hand, those keystroke-related information can also be used to protect a user's credentials via two-factor authentication and biometrics authentication schemes. This dissertation focuses on investigating the PIN inference due to the side-channel information disclosure and exploring the design of a new two-factor authentication system.
The first work in this dissertation proposes a user-independent inter-keystroke timing attack on PINs. Our attack method is based on an inter-keystroke timing dictionary built from a human cognitive model whose parameters can be determined by a small amount of training data on any users. Our attacks can thus be potentially launched in a large scale in real-world settings. We investigate inter-keystroke timing attacks in different online attack settings and evaluate their performance on PINs at different strength levels. Our experimental results show that the proposed attack performs significantly better than random guessing attacks. We further demonstrate that our attacks pose a serious threat to real-world applications and propose various ways to mitigate the threat.
We then propose a more accurate and practical PIN attack based on ultrasound, named UltraPIN, in the second work. It can be launched from commodity smartphones. As a target user enters a PIN on a PIN-based user authentication system, an attacker may use UltraPIN to infer the PIN from a short distance without a line of sight. In this process, UltraPIN leverages on smartphone speakers to issue human-inaudible ultrasound signals and uses smartphone microphones to keep recording acoustic signals. It applies a series of signal processing techniques to extract high-quality feature vectors from low-energy and high-noise signals. Taking the extracted feature vectors as input, UltraPIN applies a combination of machine learning models to classify finger movement patterns during PIN entry, and generates a ranked list of highly possible PINs as result. Rigorous experiments show that UltraPIN is highly effective in PIN inference and robust to different attacking settings.
Keystroke timing information and keystroke typing sounds can also be used to protect users' accounts. In the third work, we propose Typing-Proof, a usable, secure and low-cost two-factor authentication mechanism. Typing-Proof is similar to software token based 2FA in a sense that it uses password as the first factor and uses a registered phone to prove the second factor. During the second-factor authentication procedure, it requires a user to type any random code on a login computer and authenticates the user by comparing the keystroke timing sequence of the random code recorded by the login computer with the sounds of typing random code recorded by the user's registered phone. Typing-Proof achieves good performance in most settings and requires zero user-phone interaction in most cases. It is secure and immune to the existing attacks to recent 2FA mechanisms. In addition, Typing-Proof enables significant cost savings for both service providers and users.
This dissertation makes contributions to understanding the potential risk of side-channel information leaked by keystroke behaviors and designing a secure, usable and low-cost two-factor authentication systems. On the one hand, our proposed side-channel attacks make use of human cognitive model and ultrasound, which provides useful insights into the field of combining cognitive psychology and Doppler effect with human behavior related insecurity. On the other hand, our proposed two-factor authentication system eliminates the user-phone interaction in most cases and can effectively defend against the existing attacks to recent 2FA mechanisms.
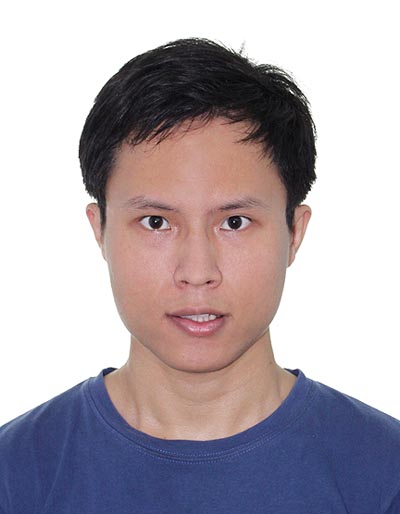
Three Essays on International Trade Policies
This dissertation studies the empirical and quantitative implications of trade policies. The first chapter examines the effects of trade policies on quality specialization across cities within a country. Specifically, we complement the quality specialization literature in international trade and study how larger cities within a country produce goods with higher quality. We first establish three stylized facts on how product quality is related to agglomeration, firm productivity, and worker skills. We then rationalize these facts in a spatial equilibrium model where all the elements mentioned above are present and firms are free to choose their locations. Using firm-level data from China, we structurally estimate the model and find that agglomeration and spatial sorting of firms each accounts for about 50% of the spatial variation in quality specialization. A counterfactual to relax land use regulation in housing production raises product quality in big cities by 5.5% and indirect welfare of individuals by 6.2%. The second chapter zooms into distributional issues and studies the implication of rising income inequality on product price dispersion. Using big data on a broad set of goods sold in the US (Nielsen Retail Scanner Data) from 2006 to 2017, we find that in general there is a missing middle phenomenon, where the product price distribution loses its mass in the middle price support. In addition, we find that this pattern is more pronounced in the densely populated metropolitan areas. We further link this observation to changes in income inequality, which are measured from a panel of US households from 2006 to 2017 (IPUMS ACS). The results support our conjecture that demand-side demographics has a significant influence on the missing middle phenomenon. The third chapter examines the transition dynamics of trade liberalization. In particular, we develop a multi-country, multi-sector quantitative trade model with dynamic Roy elements such as occupational choice and occupation-specific human capital accumulation. Given an abrupt trade liberalization, a country that is relatively more productive in some sectors may not have comparative advantage initially, as it takes time to accumulate occupation-specific human capital which increases occupational skill supply endogenously. We quantify this transition dynamics and its distributional consequences by calibrating the model to a North-South setup.
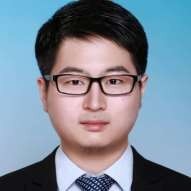
Three Essays on Econometrics
The dissertation includes three chapters on econometrics. The first chapter is about treatment effects and its application in randomized control trial. The second chapter is about specification test. The third chapter is about panel data model with fixed effects.
In the first chapter, we study the estimation and inference of the quantile treatment effect under covariate-adaptive randomization. We propose two estimation methods: (1) the simple quantile regression and (2) the inverse propensity score weighted quantile regression. For the two estimators, we derive their asymptotic distributions uniformly over a compact set of quantile indexes, and show that, when the treatment assignment rule does not achieve strong balance, the inverse propensity score weighted estimator has a smaller asymptotic variance than the simple quantile regression estimator. For the inference of method (1), we show that the Wald test using a weighted bootstrap standard error under-rejects. But for method (2), its asymptotic size equals the nominal level. We also show that, for both methods, the asymptotic size of the Wald test using a covariate-adaptive bootstrap standard error equals the nominal level. We illustrate the finite sample performance of the new estimation and inference methods using both simulated and real datasets.
In the second chapter, we propose a novel consistent model specification test based on the martingale difference divergence (MDD) of the error term given the covariates. The MDD equals zero if and only if error term is conditionally mean independent of the covariates. Our MDD test does not require any nonparametric estimation under the null or alternative and it is applicable even if we have many covariates in the regression model. We have established the asymptotic distributions of our test statistic under the null and under a sequence of Pitman local alternatives converging to the null at the usual parametric rate. We have conducted simulations to evaluate the finite sample performance of our test and compare it with its competitors. We find that our MDD test has superb performance in terms of both size and power and it generally dominates its competitors. In particular, it’s the only test that has well controlled size in the presence of many covariates and reasonable power against high frequent alternatives as well. We apply our test to test for the correct specification of functional forms in gravity equations for four datasets. For all the datasets, we reject the log and level model coherently at 10% significance level. However, its competitors show mixed testing results for different datasets. The findings reveal the advantages of our test.
In the third chapter, we consider the Nickell bias problem in dynamic fixed effects multilevel panel data models with various kinds of multi-way error components. For some specifications of error components, there exist many different forms of within estimators which are shown to be of possibly different asymptotic properties. The forms of the estimators in our framework are given explicitly. We apply the split-sample jackknife approach to eliminate the bias. In practice, our results can be easily extended to multilevel panel data models with higher dimensions.
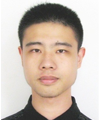
Deep Learning for Real-world Object Detection
Despite achieving significant progresses, most existing detectors are designed to detect objects in academic contexts but consider little in real-world scenarios. In real-world applications, the scale variance of objects can be significantly higher than objects in academic contexts; In addition, existing methods are designed for achieving localization with relatively low precision, however more precise localization is demanded in real-world scenarios; Existing methods are optimized with huge amount of annotated data, but in certain real-world scenarios, only a few samples are available. In this dissertation, we aim to explore novel techniques to address these research challenges to make object detection algorithms practical for real-world applications.
The first problem is scale-invariant detection. Detecting objects with multiple scales is covered in existing detection benchmarks. However, in real-world applications the scale variance of objects is extremely high and thus it requires more discriminative features. Face detection is a suitable benchmark to evaluate scale-invariant detection due to the vastly different scales of faces. In this dissertation, we propose a novel framework of ``Feature Agglomeration Networks" (FAN) to build a new single stage face detector. A novel feature agglomeration block is proposed to enhance low-level feature representation and the model is optimized in a hierarchical manner. FAN achieved state-of-the-art results in real world face detection benchmarks with real-time inference speed.
The second problem is high-quality detection. This challenge requires detectors to predict more precise localization. In this dissertation, we propose two novel detection frameworks for high-quality detection: ``Bidirectional Pyramid Networks'' (BPN) and ``KPNet''. In BPN, a Bidirectional Feature Pyramid structure is proposed for robust feature representations, and a Cascade Anchor Refinement is proposed to gradually refine the quality of pre-designed anchors. To eliminate the initial anchor design step in BPN, KPNet is proposed which automatically learns to optimize a dynamic set of high-quality keypoints without heuristic anchor design. Both BPN and KPNet show significant improvement over existing on MSCOCO dataset, especially in high quality detection settings.
The third problem is few-shot detection, where only a few training samples are available.
Inspired by the principle of meta-learning methods, we propose two novel meta-learning based few-shot detectors: ``Meta-RCNN" and ``Meta Constrastive Detector'' (MCD). Meta-RCNN learns an binary object detector in an episodic learning paradigm on the training data with a class-aware attention module, and it can be end-to-end meta-optimized. Based on Meta-RCNN, MCD follows the principle of contrastive learning to enhance the feature representation for few-shot detection, and a new hard negative sampling strategy is proposed to address imbalance of training samples. We demonstrate the effectiveness of Meta-RCNN and MCD in few-shot detection on Pascal VOC dataset and obtain promising results.
The proposed techniques address the problems discussed and show significant improvement on real-world utility.
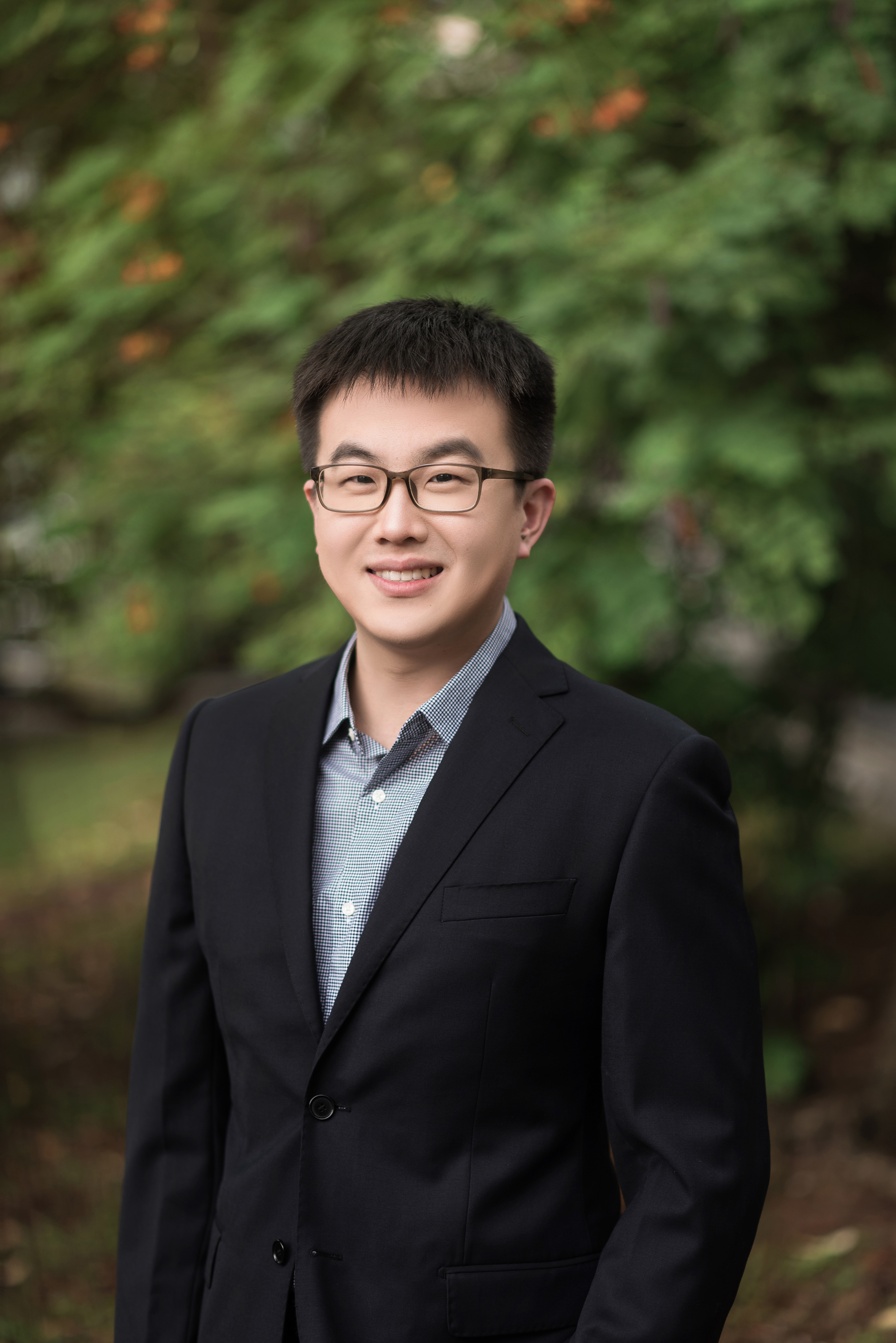
Essays on Nonstationary Econometrics
My dissertation consists of three essays that contribute new theoretical results to robust inference procedures and machine learning algorithms in nonstationary models.
Chapter 2 compares OLS and GLS in autoregressions with integrated noise terms. Grenander and Rosenblatt (2008) gave sufficient conditions for the asymptotic equivalence of GLS and OLS in deterministic trend extraction. However when extending to univariate autoregression model yt = ρnyt−1 + ut , ρn = 1 + c nα , ut = ut−1 + t , and t is one iid disturbance term with zero expectation and σ 2 variance, the asymptotic equivalence no longer holds. Under the mildly explosive (c > 0, α ∈ (0, 1)) and pure explosive (c > 0, α = 0) cases, the limiting distributions of OLS and GLS estimates are identical as standard Cauchy distribution, and the OLS estimate has a slower convergence rate. Under the mildly stationary (c < 0, α ∈ (0, 1)) case, the limiting distribution of OLS is degenerate centered at −c, while the GLS estimate is Gaussian distributed. Under the local to unity (α = 1) case, when c ≥ c ∗ , the mean and variance of the asymptotic distribution of the OLS estimate are smaller than the GLS estimate, showing the efficiency gains in OLS.
Chapter 3 proposes novel mechanisms for identifying explosive bubbles in panel autoregressions with a latent group structure. Two post-classification panel data approaches are employed to test the explosiveness in time-series data. The first approach applies a recursive k-means clustering algorithm to explosive panel autoregressions. The second approach uses a modified k-means clustering algorithm for mixed-root panel autoregressions. We establish the uniform consistency of both clustering algorithms. The abovementioned k-means procedures achieve the oracle properties so that the post-classification estimators are asymptotically equivalent to the infeasible estimators that use the true group identities. Two right-tailed t-statistics, based on post-classification estimators, are introduced to detect explosiveness. A panel recursive procedure is proposed to estimate the origination date of explosiveness. The asymptotic theory is available for concentration inequalities, clustering algorithms, and right-tailed t-tests based on mixed-root panels. Extensive Monte Carlo simulations provide strong evidence that the proposed panel approaches lead to substantial power gains compared with the time-series approach.
Chapter 4 explores predictive regression models with stochastic unit root (STUR) components and robust inference procedures that encompass a wide class of persistent and time-varying stochastically nonstationary regressors. The paper extends the mechanism of endogenously generated instrumentation known as IVX, showing that these methods remain valid for short- and long-horizon predictive regressions in which the predictors have STUR and local STUR (LSTUR) generating mechanisms. Both mean regression and quantile regression methods are considered. The asymptotic distributions of the IVX estimators are new compared to previous work but again lead to pivotal limit distributions for Wald testing procedures that remain robust for both single and multiple regressors with various degrees of persistence and stochastic and fixed local departures from unity. Numerical experiments corroborate the asymptotic theory, and IVX testing shows good power and size control. The new methods are illustrated in an empirical application to evaluate the predictive capability of economic fundamentals in forecasting excess returns in the Dow Jones industrial average index.
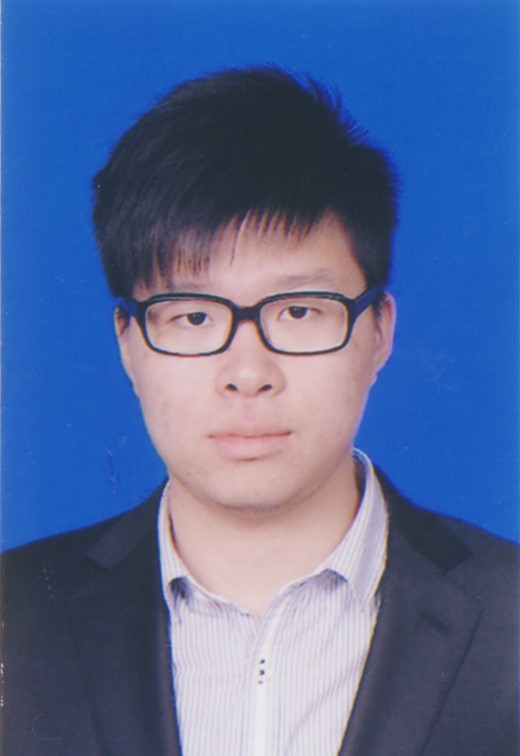
Essays on Time Series and Financial Econometrics
This dissertation contains four essays in financial econometrics. In the first essay, some asymptotic results are derived for first-order autoregression with a root moderately deviating from unity and a nonzero drift. It is shown that the drift changes drastically the large sample properties of the least-squares (LS) estimator. The second essay is concerned with the joint test of predictability and stability in the context of predictive regression. The null hypothesis under investigation is that the potential predictors exhibit no predictability and incur no structural break during the sample period. We first show that the IVX estimator provides better finite sample performance than LS when they are used to test for a structural break in the slope coefficient. We then consider a new test by combining the IVX and sup-Wald statistics. The third essay considers the impact of level-shifts in the predicted variable on the performance of the conventional test for predictability when highly persistent predictors are used. It is shown that the limiting distribution of conventional t-statistic depends on the magnitude of break size. When the breaks are ignored, the t-statistic generates a too large type-I error. To alleviate this problem, we propose to base the inference on a sample-splitting procedure. Applications to the prediction of stock return volatility and housing price index are conducted. In the last essay, we consider a new multivariate stochastic volatility (MSV) model, applying a fully flexible parameterization of the correlation matrix, which generalizes Fisher’s z-transformation to the high-dimensional case. In the new model, we can separately model the dynamics in volatilities and correlations. To conduct statistical inference of the proposed model, we propose the Particle Gibbs Ancestor Sampling (PGAS) method. Extensive simulation studies are conducted to show the proposed method works well.
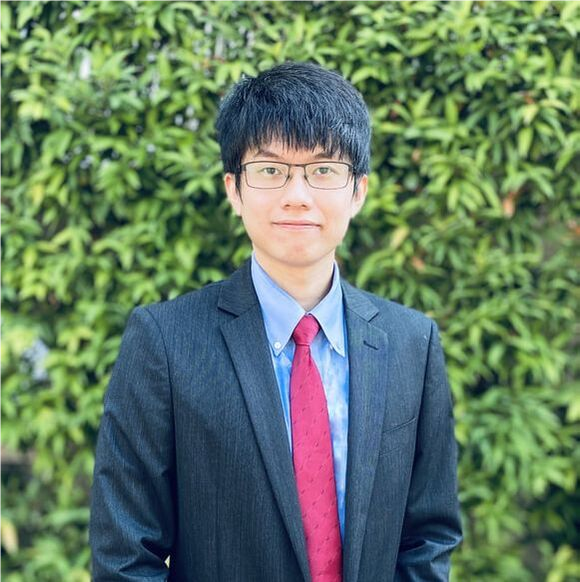
This dissertation comprises three papers that separately study different nonstationary time series models.
The first paper, titled as "The Grid Bootstrap for Continuous Time Models", is a joint work with Professor Jun Yu and Professor Weilin Xiao. It considers the grid bootstrap for constructing confidence intervals for the persistence parameter in a class of continuous-time models driven by a Lévy process. Its asymptotic validity is discussed under the assumption that the sampling interval (h) shrinks to zero, the time span (N) goes to infinity or both. Its improvement over the in-fill asymptotic theory is achieved by expanding the coefficient-based statistic around its in-fill asymptotic distribution which is non-pivotal and depends on the initial condition. Monte Carlo studies show that the grid bootstrap method performs better than the in-fill asymptotic theory and much better than the long-span asymptotic theory. Empirical applications to U.S. interest rate data and volatility data suggest significant differences between the bootstrap confidence intervals and the confidence intervals obtained from the in-fill and long-span asymptotic distributions.
The second paper, "Mildly Explosive Autoregression with Anti-persistent Errors" is another joint work with Professor Yu and Professor Xiao. It studies a mildly explosive autoregression model with Anti-persistent Errors. An asymptotic distribution is derived for the least squares (LS) estimate of a first-order autoregression with a mildly explosive root and anti-persistent errors. While the sample moments depend on the Hurst parameter asymptotically, the Cauchy limiting distribution theory remains valid for the LS estimates in the model without intercept and a model with an asymptotically negligible intercept. Monte Carlo studies are designed to check the precision of the Cauchy distribution in finite samples. An empirical study based on the monthly NASDAQ index highlights the usefulness of the model and the new limiting distribution.
The third paper "Testing for Rational Bubbles under Strongly Dependent Errors" considers testing procedures for rational bubbles under strongly dependent errors. A heteroskedasticity and autocorrelation robust (HAR) test statistic is proposed to detect the presence of rational bubbles in financial assets when errors are strongly dependent. The asymptotic theory of the test statistic is developed. Unlike conventional test statistics that lead to a too large type I error under strongly dependent errors, the new test does not suffer from the same size problem. In addition, it can consistently timestamp the origination and termination dates of a rational bubble. Monte Carlo studies are conducted to check the finite sample performance of the proposed test and estimators. An empirical application to the S&P 500 index highlights the usefulness of the proposed test statistic and estimators.
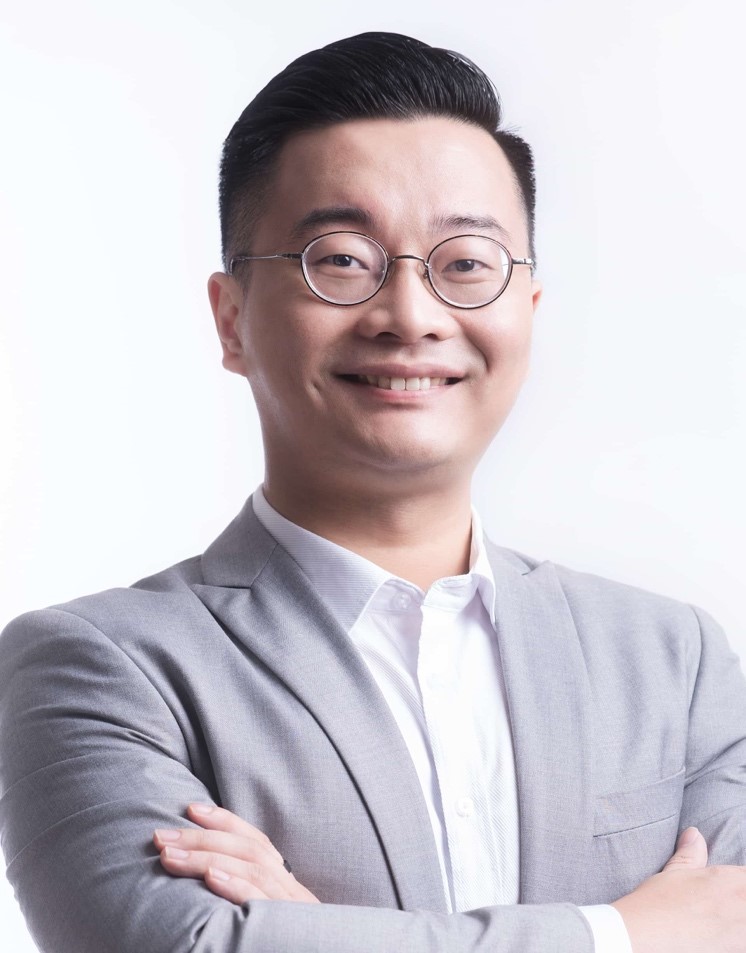
Followers’ Reactions to Leader Differentiation
Leaders generally differentiate their relationships with followers, for example, by providing some with more respect, trust, support, or information than others (Liden & Graen, 1980). However, the effects of such leader differentiation on followers remain inconclusive such that research suggests that leader differentiation may have negative, positive, or null effects on favorable employee work-related outcomes (for a recent review, see Martin et al., 2018). To better understand the effects of leader differentiation, utilizing leader-member exchange (LMX) theory, I considered three inherently connected properties in the leader differentiation process – LMX differentiation, LMX quality and LMX social comparison (Martin et al., 2018). I theorized that the three properties interact to influence followers’ supervisory interactional justice perceptions and subsequently their discretionary behaviors toward their leaders. Results from three studies with different research designs and conducted in different cultures, largely supported my hypothesized conditional moderated mediation model. When LMX quality and LMX social comparison were both high, the negative impact of LMX differentiation on followers’ supervisory interactional justice perceptions was the weakest. In addition, when LMX quality and LMX social comparison were both high, LMX differentiation’s positive indirect effect on followers’ supervisor-directed deviance and its negative indirect effect on followers’ supervisor-directed organizational citizenship behaviors via followers’ supervisory interactional justice perceptions were the weakest.
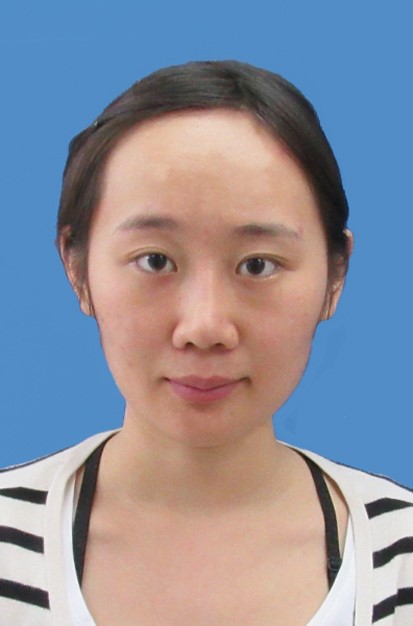
This dissertation studies the fixed effects (FE) spatial panel data (SPD) models with temporal heterogeneity (TH), where the regression coefficients and spatial coefficients are allowed to change with time. The FE-SPD model with time-varying coefficients renders the usual transformation method in dealing with the fixed effects inapplicable, and an adjusted quasi score (AQS) method is proposed, which adjusts the concentrated quasi score function with the fixed effects being concentrated out. AQS tests for the lack of temporal heterogeneity (TH) in slope and spatial parameters are first proposed. Then, a set of AQS estimation and inference methods for the FE-SPD model with temporal heterogeneity is developed, when the AQS tests reject the hypothesis of temporal homogeneity. Finally, an attempt is made to extend these methodologies to allow the idiosyncratic errors of the model to be heteroskedastic along the cross-section dimension, where a method called outer-product-of-martingale-differences is proposed to estimate the variance of the AQS functions which in turn gives a robust estimator of the variance-covariance matrix of the AQS estimators.
Asymptotic properties of the AQS tests are examined. Consistency and asymptotic normality of the AQS estimators are examined under both homoscedastic and heteroskedastic errors. Extensive Monte Carlo experiments are conducted and the results show excellent finite sample performance of the proposed AQS tests, the proposed AQS estimators of the full model, and the corresponding estimates of the standard errors. Empirical illustrations are provided.
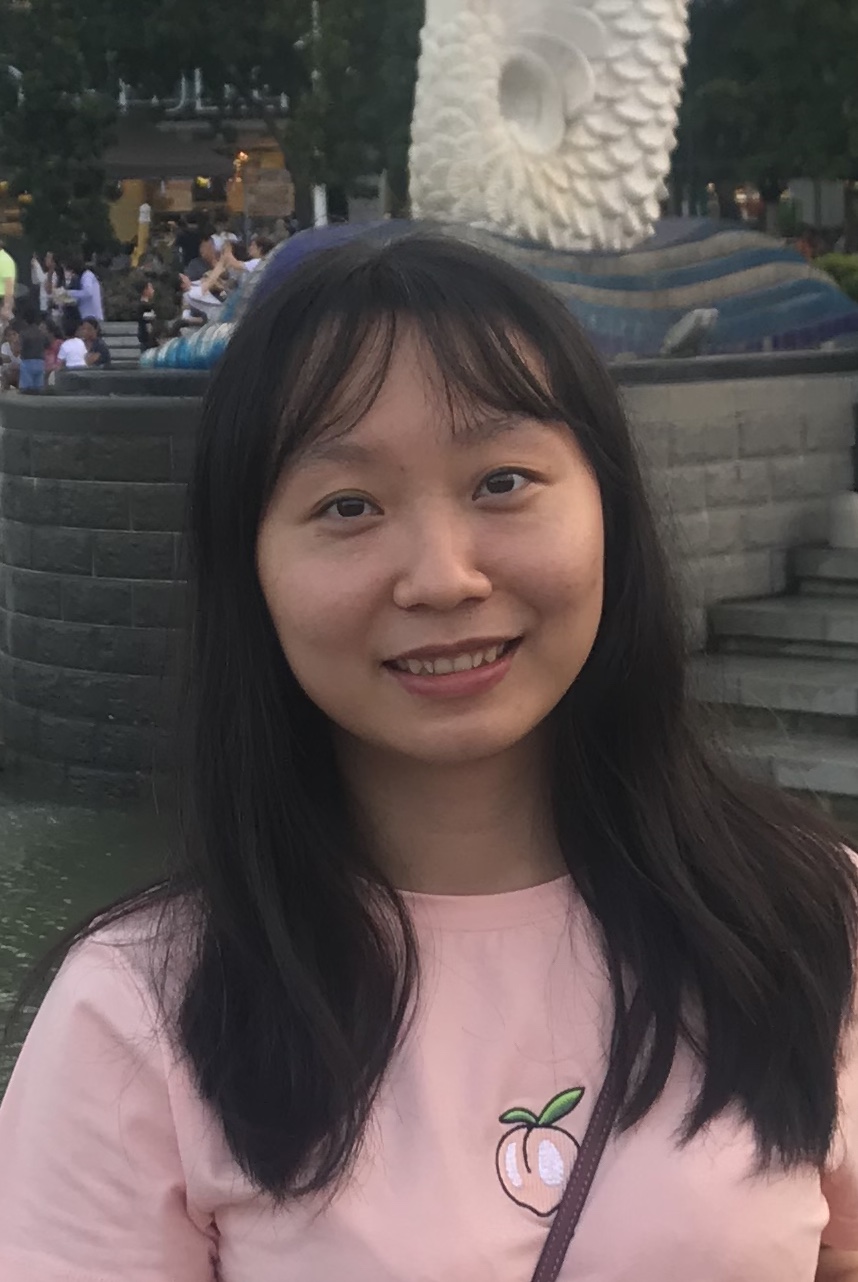
Using Knowledge Bases for Question Answering
A knowledge base (KB) is a well-structured database, which contains many of entities and their relations. With the fast development of large-scale knowledge bases such as Freebase, DBpedia and YAGO, knowledge bases have become an important resource, which can serve many applications, such as dialogue system, textual entailment, question answering and so on. These applications play significant roles in real-world industry.
In this dissertation, we try to explore the entailment information and more general entity-relation information from the KBs. Recognizing textual entailment (RTE) is a task to infer the entailment relations between sentences. We need to decide whether a hypothesis can be inferred from a premise based on the text of two sentences. Such entailment relations could be potentially useful in applications like information retrieval and commonsense reasoning. It's necessary to develop automatic techniques to solve this problem. Another task is knowledge base question answering (KBQA). This task aims to automatically find answers to factoid questions from a knowledge base, where answers are usually entities in the KB. KBQA task has gained much attention in recent years and shown promising contribution to real-world problems. In this dissertation, we try to study the applications of knowledge bases in textual entailment and question answering:
We propose a general neural network based framework which can inject lexical entailment relations to RTE, and a novel model is developed to embed lexical entailment relations. The experiment results show that our method can benefit general textual entailment model. We design a KBQA method based on an existing reading comprehension model. This model achieves competitive results on several popular KBQA datasets. In addition, we make full use of contextual relations of entities in the KB. Such enriched information helps our model to attain state-of-art. We propose to perform topic unit linking where topic units cover a wider range of units of a KB. We use a generation-and-scoring approach to gradually refine the set of topic units. Furthermore, we use reinforcement learning to jointly learn the parameters for topic unit linking and answer candidate ranking in an end-to-end manner. Experiments on three commonly used benchmark datasets show that our method consistently works well and outperforms the previous state of the art on two datasets. We further investigate multi-hop KBQA task, i.e., question answering from KB where questions involve multiple hops of relations, and develop a novel model to solve such questions in an iterative and efficient way. The results demonstrate that our method consistently outperforms several multi-hop KBQA baselines.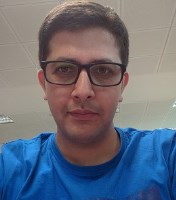
Over last few decades, the way software is developed has changed drastically. From being an activity performed by developers working individually to develop standalone programs, it has transformed into a highly collaborative and cooperative activity. Software development today can be considered as a participatory culture, where developers coordinate and engage together to develop software while continuously learning from one another and creating knowledge.
In order to support their communication and collaboration needs, software developers often use a variety of social media channels. These channels help software developers to connect with like-minded developers and explore collaborations on software projects of interest. However, developers face a lot of challenges while trying to make use of various social media channels. As the volume of content produced on social media is huge developers often face the problem of information overload while using these channels. Also creating and maintaining a relevant network among a huge number of possible connections is challenging for developers. The works performed in this dissertation focus on addressing the above challenges with respect to Twitter, a social media popular among developers to get the latest technology updates, as well as connect with other developers. The first three works performed as a part of this dissertation deal with understanding the software engineering content produced on Twitter and how it can be harnessed for automatic mining of software engineering related knowledge. The last work aims at understanding what kind of accounts software developers follow on Twitter, and then proposes an approach which can help developers to find software experts on Twitter. The following paragraphs briefly describe the works that have been completed as part of this dissertation and how they address the aforementioned challenges.
In the first work performed as part of the dissertation, an exploratory study was conducted to understand what kind of software engineering content is popular among developers in Twitter. The insights found in this work help to understand the content that is preferred by developers on Twitter and can guide future techniques or tools which aim to extract information or knowledge from software engineering content produced on Twitter. In the second work, a technique was developed which can automatically differentiate content related to software development on Twitter from other non-software content. This technique can help in creating a repository of software related content extracted from Twitter, that can be used to create downstream tools which can do tasks such as mining opinions about APIs, best practices, recommending relevant links to read, etc. In the third work, Twitter was leveraged to automatically find URLs related to a particular domain, as Twitter makes it possible to infer the network and popularity information of users who tweet a particular URL. 14 features were proposed to characterize each URL by considering webpage contents pointed by it, popularity and content of tweets mentioning it, and the popularity of users who shared the URL on Twitter.
In the final work of this dissertation, an approach has been proposed to address the challenge developers face in finding relevant developers to follow on Twitter. A survey was done with developers, and based on its analysis, an approach was proposed to identify software experts on Twitter, provided a given software engineering domain. The approach works by extracting 32 features related to Twitter users, with features belonging to the categories such as Content, Network, Profile, and GitHub. These features are then used to build a classifier which can identify a Twitter user as a software expert of a given domain or otherwise. The results show that our approach is able to achieve F-Measure scores of 0.522-0.820 on the task of identifying software experts, achieving an improvement of at-least 7.63% over the baselines.
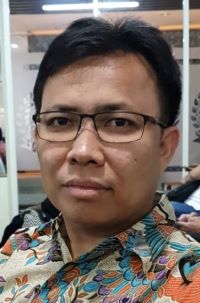
The rapid advances of the Web have changed the ways information is distributed and exchanged among individuals and organizations. Various content from different domains are generated daily and contributed by users' daily activities, such as posting messages in a microblog platform, or collaborating in a question and answer site. To deal with such tremendous volume of user generated content, there is a need for approaches that are able to handle the mass amount of available data and to extract knowledge hidden in the user generated content. This dissertation attempts to make sense of the generated content to help in three concrete tasks.
In the first work performed as part of the dissertation, a machine learning approach was proposed to predict a customer's feedback behavior based on her first feedback tweet. First, a few categories of customers were observed based on their feedback frequency and the sentiment of the feedback. Three main categories were identified: spiteful, one-off, and kind. By using the Twitter API, user profile and content features were extracted. Next, a model was built to predict the category of a customer given his or her first feedback. The experiment results show that the prediction model performs better than a baseline approach in terms of precision, recall, and F-measure. In the second work, a method was proposed to predict readers' emotion distribution affected by a news article. The approach analyzed affective annotations provided by readers of news articles taken from a non-English online news site. A new corpus was created from the annotated articles. A domain-specific emotion lexicon was constructed along with word embedding features. Finally, a multi-target regression model was built from a set of features extracted from online news articles. By combining lexicon and word embedding features, the regression model is able to predict the emotion distribution with RMSE scores between 0.067 to 0.232. For the final work of this dissertation, an approach was proposed to improve the effectiveness of knowledge extraction tasks by performing cross-platform analysis. This approach is based on transfer representation learning and word embedding to leverage information extracted from a source platform which contains rich domain-related content to solve tasks in another platform (considered as target platform) with less domain-related content. We first build a word embedding model as a representation learned from the source platform, and use the model to improve the performance of knowledge extraction tasks in the target platform. We experiment with Software Engineering Stack Exchange and Stack Overflow as source platforms, and two different target platforms, i.e., Twitter and YouTube. Our experiments show that our approach improves performance of existing work for the tasks of finding software-related tweets and filtering informative YouTube comments.
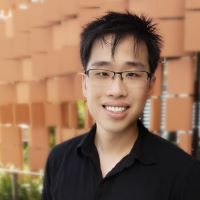
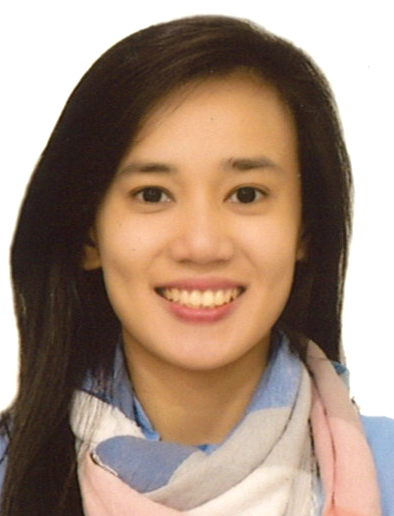
The rising mental health illnesses of severe stress and depression is of increasing concern worldwide. Often associated by similarities in symptoms, severe stress can take a toll on a person’s productivity and result in depression if the stress is left unmanaged. Unfortunately, depression can occur without any feelings of stress. With depression growing as a leading cause of disability in economic productivity, there has been a sharp rise in mental health initiatives to improve stress and depression management. To offer such services conveniently and discreetly, recent efforts have focused on using mobile technologies. However, these initiatives usually require users to install dedicated apps or use a variety of sensors, making such solutions hard to scale. Moreover, they emphasise sensing individual factors and overlook ‘physical social interaction’ that plays a significant role in influencing stress and depression. This thesis presents StressMon, a monitoring system that can easily scale across entire campuses by passively sensing location information directly from the WiFi infrastructure.
This dissertation explores how, by using only single-attribute location information, mobility features can be comprehensively extracted to represent individual behaviours to detect stress and depression accurately; it is important to note that this is without requiring explicit user actions or software installation on client devices. To overcome the low-dimensional data, StressMon additionally infers physical group interaction patterns from a group detector system. First, I investigate how mobility features can be exploited to better capture the dynamism of natural human behaviours indicative of stress and depression. Then, I present the framework to detect stress and depression accurately, albeit separately. In a supplementary effort, I demonstrate how optimising StressMon with group-based mobility features greatly enhances the performance of stress detection, and conversely, individual-based features improve depression detection. To extensively validate the system, I conducted three different semester-long longitudinal studies with different groups of undergraduate students at separate times, totalling up to 108 participants. Finally, this dissertation documents the differences learned in understanding stress and depression from a qualitative perspective.
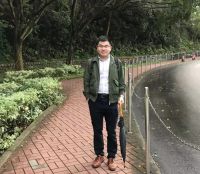
Static analysis is a common program analysis technique extensively used in the software security field. Widely-used static analysis tools for Android, e.g., Amandroid and FlowDroid, perform the whole-app analysis which is comprehensive yet at the cost of huge overheads. In this dissertation, we make a first attempt to explore a novel on-demand analysis that creatively leverages bytecode search to guide inter-procedural analysis on the fly or just in time, and develop such on-the-fly analysis into a tool, called BackDroid, for Android apps. We further explore how the core technique of on-the-fly static analysis in BackDroid can enable different vulnerability studies on Android and their corresponding new findings. To this end, we select three vulnerability analysis problems on Android as three representatives, since they require different extents of BackDroid customization in their methodology.
First, we explore how BackDroid can be applied to detect crypto and SSL/TLS misconfigurations in modern Android apps, and compare it with the state-of-the-art Amandroid tool. Second, we explore how an enhanced version of BackDroid and on-device crowdsourcing can facilitate a systematic security study of open ports in Android apps. Third, we explore how a lightweight version of BackDroid with SDK conditional statement checking can benefit a SDK-API inconsistency study that involves the control-flow analysis of multiple sink APIs. With all these works, this dissertation shows that on-the-fly Android static analysis guided by bytecode search can efficiently and effectively analyze the security of modern apps.
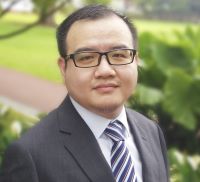
The dissertation explores the role of human capital, education, and political institutions in the process of economic and political development. The first chapter shows that economic development such as secondary school enrollment rates during the democratization period exerts long-lasting effects on growth, possibly by giving permanent birthmarks to newly minted democratic institutions. Specifically, democracies born in weak development tend to have weak institutions and slow growth, while in contrast, those with adequate development at the political transition time establish strong institutions and achieve faster growth. The second chapter explores the effect of curriculum control in schooling on national innovation and individual creativity. The evidence suggests that a more centralized curriculum control, as indicated by more centralized official curriculum design together with more frequent high-stakes achievement exams, tends to reduce individual creativity and weaken national innovation. The third chapter studies how state capacity affects the investment in human capital, economic growth and democratization. It shows that autocracy may not necessarily inhibit economic growth when a country is poor but the state capacity is strong, while democracy facilitates growth more when a country is rich. In particular, the relationship between state development and democratization follows an inverted U-shape.
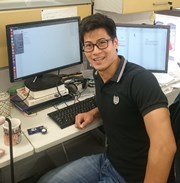
In this thesis, we study reinforcement learning algorithms to collectively optimize decentralized policy in a large population of autonomous agents. We notice one of the main bottlenecks in large multi-agent system is the size of the joint trajectory of agents which quickly increases with the number of participating agents. Furthermore, the noiseof actions concurrently executed by different agents in a large system makes it difficult for each agent to estimate the value of its own actions, which is well-known as the multi-agent credit assignment problem. We propose a compact representation for multi-agent systems using the aggregate counts to address the high complexity of joint state-action and novel reinforcement learning algorithms based on value function decomposition to address the multi-agent credit assignment problem as follows: 1. Collective Representation: In many real-world systems such as urban traffic networks, the joint-reward and environment dynamics depend on only the number of agents (the count) involved in interactions rather than agent identity. We formulate this sub-class of multi-agent systems as a Collective Decentralized Partially Observable Markov Decision Process (CDEC-POMDP). We show that in CDEC-POMDP, the transition counts, which summarize the numbers of agents taking different local actions and transiting from their current local states to new local states, are sufficient-statistics for learning/optimizing the decentralized policy. Furthermore, the dimensions of the count variables are not affected by the population size. This allows us to transform the original planning problems to optimize the complex joint agent trajectory into optimizing compact count variables. In addition, samples of the counts can be efficiently obtained with multinomial distributions, which provide a faster way to simulate the multi-agent systems and evaluate the planning policy. 2. Collective Multi-agent Reinforcement Learning (MRL): Firstly, to address multi-agent credit assignment problem in {\cmdp}, we propose the collective decomposition principle in designing value function approximation and decentralized policy update. Under this principle, the decentralized policy of each agent is updated using an individualized value instead of a joint global value. We formulate a joint update for policies of all agents using the counts, which is much more scalable than independent policy update with joint trajectory. Secondly, based on the collective decomposition principle, we design 2 classes of MRL algorithms for domains with local rewards and for domains with global rewards respectively. i) When the reward is decomposable into local rewards among agents, by exploiting exchangeability in CDEC-POMDPs we propose a mechanism to estimate the individual value function by using the sampled values of the counts and average individual rewards. We use this count-based individual value function to derive a new actor critic algorithm called fAfC to learn effective individual policy for agents. ii) When the reward is non-decomposable, the system performance is evaluated by a single global value function instead of individual value functions. To follow the decomposition principle, we show how to estimate individual contribution value of agents using partial differentials of the joint value function with respect to the state-action counts. This is the basis for us to develop two algorithms called MCAC and CCAC to optimize individual policy under non-decomposable reward domains. Experimentally, we show the superiority of our proposed collective MRL algorithms in various testing domains: a real-world taxi supply-demand matching domain, a police patrolling game and a synthetic robot navigation domain, with population size up to 8000. They converge faster convergence and provide better solutions than other algorithms in the literature, i.e. average-flow based algorithms and standard actor critic algorithm.
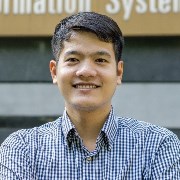
Top-K recommendation is a typical task in Recommender Systems. In traditional approaches, it mainly relies on the modeling of user-item associations, which emphasizes the user-specific factor or personalization. Here, we investigate another direction that models item-item associations, especially with the notions of sequence-aware and basket-level adoptions . Sequences are created by sorting item adoptions chronologically. The associations between items along sequences, referred to as “sequential associations”, indicate the influence of the preceding adoptions on the following adoptions. Considering a basket of items consumed at the same time step (e.g., a session, a day), “basket-oriented associations” imply correlative dependencies among these items. In this dissertation, we present research works on modeling “sequential & basket-oriented associations” independently and jointly for the Top-K recommendation task.
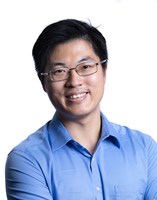
Three Essays on Credit Default Swaps
Chapter 1: Credit Default Swaps Pricing Errors and Related Stock Returns
This article investigates the impacts of Credit Default Swaps (CDS) pricing errors on related stock returns. Using a parsimonious CDS valuation model, which produces an above average adjusted R2 of 90%, I find that its pricing errors significantly predict cross-section stock returns. Further investigation reveals that the cross-market return predictability channels via Merton (1974)'s structural prediction and primary dealers' capital risk. This paper provides a novel view of the complex interactions of capital markets and offers insights on the relative market efficiencies.
Chapter 2: CDS Markets Informativeness and Related Hard-to-Value Stock Returns
This research investigates the conundrum whether the Credit Default Swaps (CDS) market is informed relative to the equity market. To do this, we examine the impact of CDS price changes on stock returns calculated by transaction prices in various trading intervals within daily close-to-close. We find that stock returns overreact to credit news during trading hours and partially reverse after the market closes. The predictive effect of CDS news concentrates on ``hard-to-value stocks'' with high credit spreads. The reversal happens mainly because overconfident investors over-bet on credit news. Limit-to-arbitrage such as stock illiquidity and short-sale constraint cannot fully explain the predictive results. Overall, our empirical evidence suggests that CDS informed traders step into hard-to-value stocks with high credit spread levels.
Chapter 3: The Effect of CDS on Earnings Quality: The Role of CDS Information
This paper investigates whether the initiation of trading in credit default swaps (CDSs) on a borrowing firm's outstanding debt is associated with the decline in that firm's earnings quality. Using a differences-in-differences approach, we find that after CDS trade initiation, there is a significant reduction in intentional earnings manipulation of the underlying borrowing firms. The reduction of earnings management activities is channeled through trade credit exposures and corporate cash holdings. Further, we show that CDS prices convey distress risk information of firms with poor earnings quality and help to improve their risk fundamentals through conservative liquidity management strategy such as holding more cash, enhancing future operating cash flow, and increasing net working capital. Overall, our evidence suggests that an external monitoring role provided by CDS markets can reduce earnings management activities and mitigate the information asymmetry between corporate insiders and outsiders.
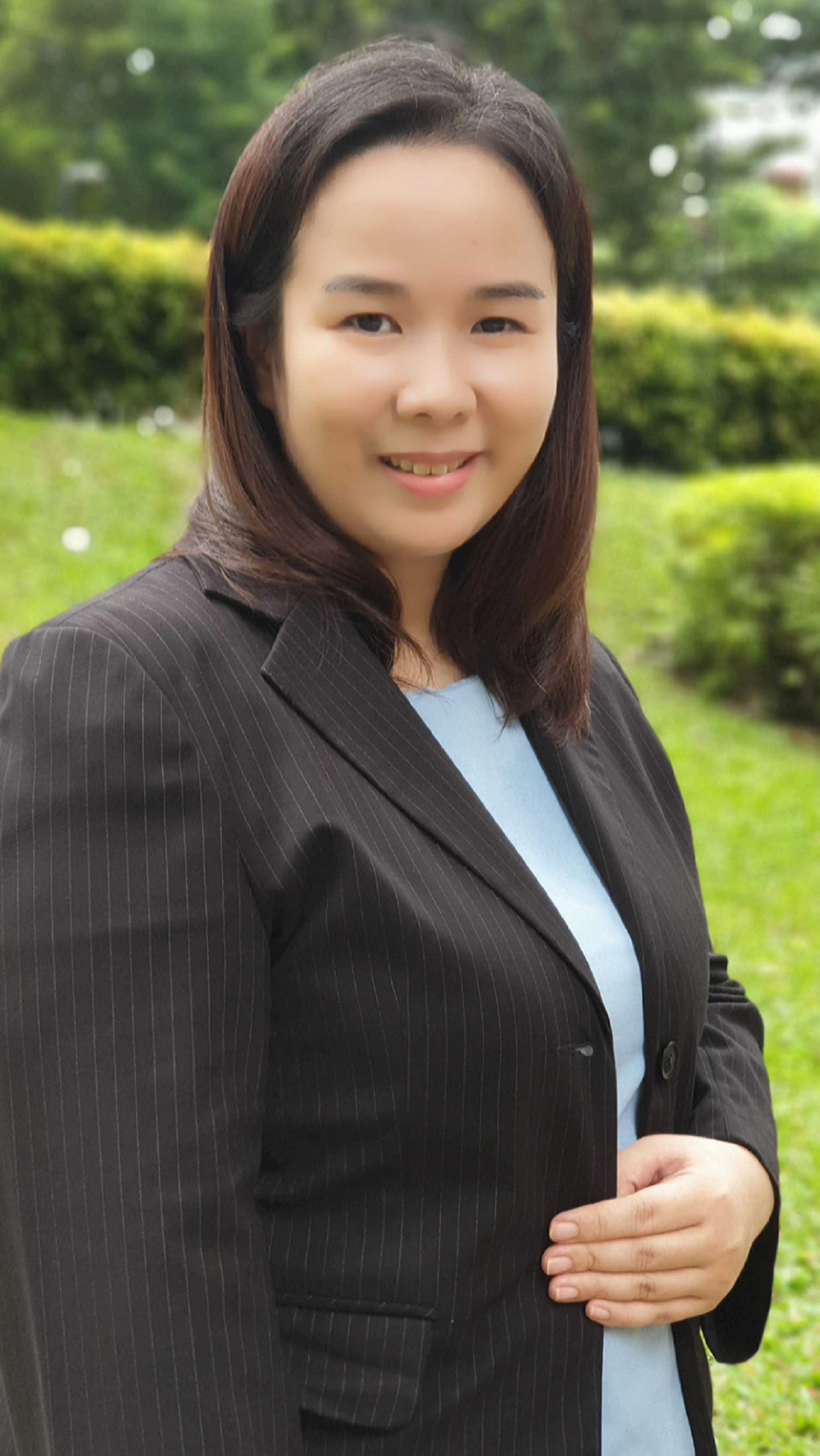
Advances in artificial intelligence are leading to many revolutions in robotics. How will the arrival of robots impact the growth of the economy, the workers' wage, consumption, and lifetime welfare? This dissertation attempts to answer this question by presenting a standard neoclassical growth model with two different kinds of robots, reflecting two ways that robots can transform the labor market. The first chapter introduces additive robots- a perfect substitution for human labor, while the second chapter employs multiplicative robots- a type of robots that augments human labor. The prevailing main result is that even in the case with no population growth and technical progress, the application of robots is enough to create a long term economic growth. Nevertheless, there is a difference in the behavior of real wage. The presence of additive robot solely makes wage jumps down and then stays constant throughout while utilization of multiplicative robots alone can increase productivity thus real wage increases fast over time.
In the last chapter, both types of robots are applied in the economy with a shrinking population, motivated by Japan. Under the perfect homogeneous labor market, there will be a shift of workers from jobs that can be substituted by additive robots to jobs that can be supported by multiplicative robots. This enables Japan to continue to enjoy the perpetual growth in real wage, consumption and wealth even after the labor market has finished its adjustment. However, as the interest rate would slowly decrease, proportionate to the decline of the population, there would be a point where it is no longer profitable to adopt robots although it would take a long time for the economy to face that issue.
.
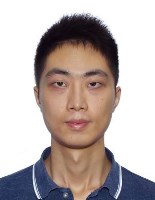
In the past few decades, supervised machine learning approach is one of the most important methodologies in the Natural Language Processing (NLP) community. Although various kinds of supervised learning methods have been proposed to obtain the state-of-the-art performance across most NLP tasks, the bottleneck of them lies in the heavy reliance on the large amount of manually annotated data, which is not always available in our desired target domain/task. To alleviate the data sparsity issue in the target domain/task, an attractive solution is to find sufficient labeled data from a related source domain/task. However, for most NLP applications, due to the discrepancy between the distributions of the two domains/tasks, directly training any supervised models only based on labeled data in the source domain/task usually results in poor performance in the target domain/task. Therefore, it is necessary to develop effective transfer learning techniques to leverage rich annotations in the source domain/task to improve the model performance in the target domain/task.
There are generally two settings of transfer learning. We use supervised transfer learning to refer to the setting when a small amount of labeled target data is available during training, and when no such data is available we call it unsupervised transfer learning. In this thesis, we focus on proposing novel transfer learning methods for different NLP tasks in both settings, with the goal of inducing an invariant latent feature space across domains or tasks, where the knowledge gained from the source domain/task can be easily adapted to the target domain/task.
In the unsupervised transfer learning setting, we first propose a simple yet effective domain adaptation method by deriving shared representations with instance similarity features, which can be generally applied for different NLP tasks, and empirical evaluation on several NLP tasks shows that our method has indistinguishable or even better performance than a widely used domain adaptation method. Furthermore, we target at a specific NLP task, i.e., sentiment classification, and propose a neural domain adaptation framework, which performs joint learning of the actual sentiment classification task and several manually designed domain-independent auxiliary tasks to produce shared representations across domains. Extensive experiments on both sentence-level and document-level sentiment classification demonstrate that our proposed domain adaptation framework can achieve promising results.
In the supervised transfer learning setting, we first propose a neural domain adaptation approach for retrieval-based question answering systems by simultaneously learning shared feature representations and modelling inter-domain and intra-domain relationships in a unified model, followed by conducting both intrinsic and extrinsic evaluation to demonstrate the efficiency and effectiveness of our method. Moreover, we attempt to improve multi-label emotion classification with the help of sentiment classification by proposing a dual attention transfer network, where a shared feature space is employed to capture the general sentiment words, and another task-specific space is employed to capture the specific emotion words. Experimental results show that our method is able to outperform several highly competitive transfer learning methods.
Although the transfer learning methods proposed in this thesis are originally designed for natural language processing tasks, most of them can be potentially applied to classification tasks in the other research communities such as computer vision and speech processing.
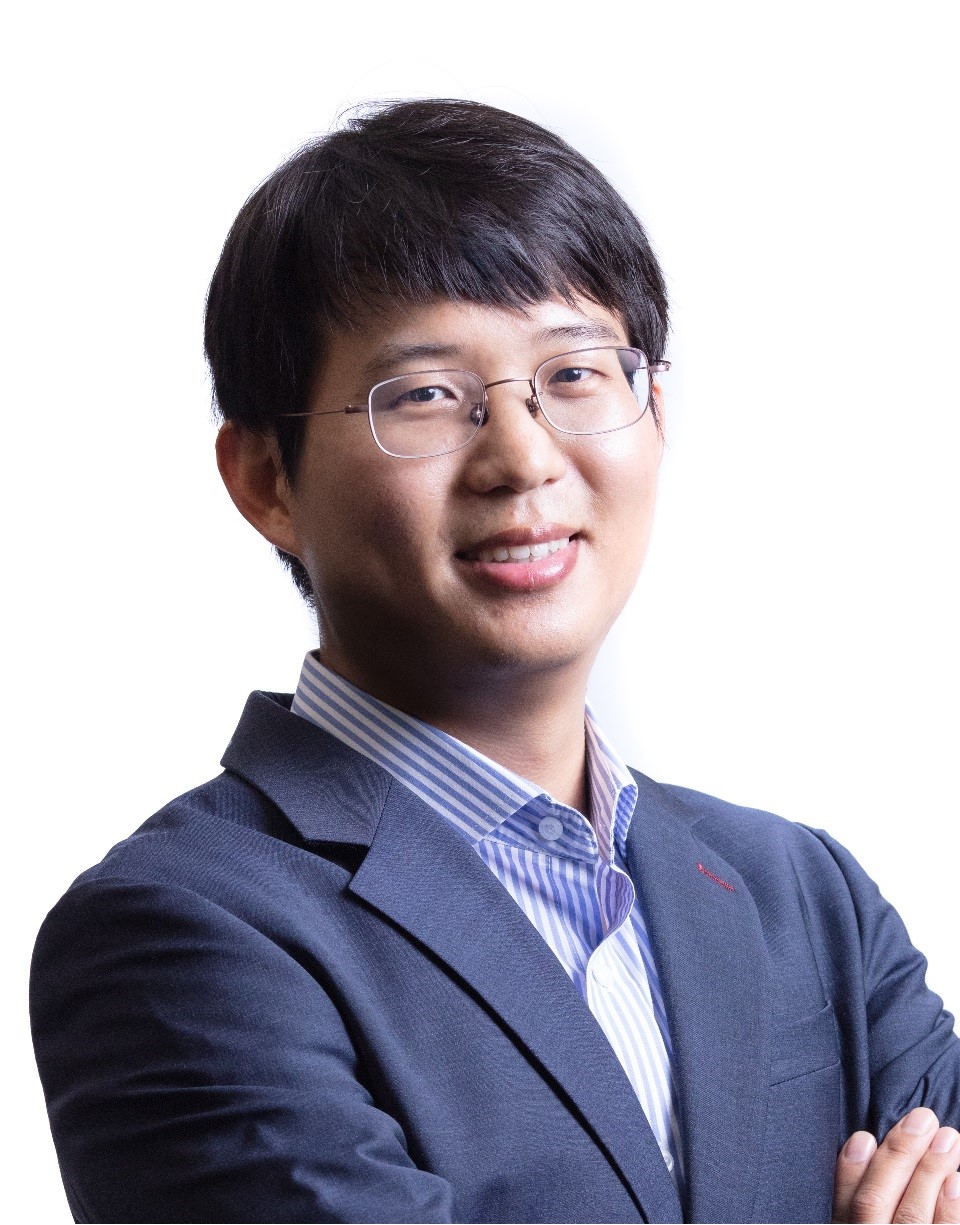
This dissertation consists of three papers in mutual fund governance or market microstructure that analyze the causal effect of board independence on mutual fund performance or the trading behavior of institutional trading and informed trading.
Chapter I studies how board independence affects fund performance, in relation to investment experience of independent directors. Using the SEC amendment in 2001 as an exogenous shock, I find that board independence does not improve or damage fund performance on average. When a fund board has independent directors with investment experience, however, it boosts fund performance. I also find that a fund manager is less constrained and the management fee on a contract is more aligned with fund performance under such a fund board. My findings suggest that board independence is not always beneficial to mutual fund shareholders, but its effectiveness varies depending on independent directors' investment experience.
Chapter II estimates daily aggregate order flow of individual stocks from all institutional investors as well as for hedge funds and other institutions separately. This study is coauthored with my advisor, Prof. Jianfeng Hu. We achieve this by extrapolating the relation between quarterly institutional ownership in 13F filings, aggregate market order imbalance in TAQ, and a representative group of institutional investors' transaction data. We find that the estimated institutional order imbalance positively predicts stock return on the next day and outperforms other institutional order flow estimates. The institutional order flow from hedge funds creates smaller contemporaneous price pressure and generates greater and more persistent price impact than the order flow from all other institutions. We also find that hedge funds trade on well-known anomalies while the other institutions do not. Our findings suggest that the superior trading skills of institutional investors can be largely attributed to hedge funds.
Lastly, I propose a simple measure of informed trading based on the Kyle (1985) model in Chapter III. This study is also coauthored with my advisor, Prof. Jianfeng Hu. We first calculate implied order imbalance (IOI) as contemporaneous stock returns divided by low-frequency illiquidity measures. The implied informed trading (IIT) is the residual of IOI regressed on its components (returns and illiquidity). We find that IIT positively predicts short-term future stock returns without subsequent reversals in the cross-section between 1927 and 2016. This predictability is robust in subperiods, and strengthens in stocks with high information asymmetry and before corporate events. The predictability survives existing measures of informed trading including short selling activities, order imbalance, and institutional trading in recent periods. Finally, IIT has the same predictive ability in G10 equity markets.
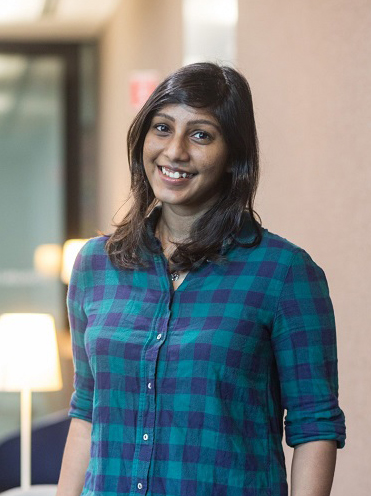
As cities worldwide invest heavily in smart city infrastructure, it invites opportunities for a next wave of urban analytics. Unlike its predecessors, urban analytics applications and services can now be real-time and proactive -- they can (a) leverage situational data from large deployments of connected sensors, (b) capture attributes of a variety of entities that make up the urban fabric (e.g., people and their social relationships, transport nodes, utilities, etc.), and (c) use predictive insights to both proactively optimize urban operations (e.g., HVAC systems in smart buildings, buses in the transportation network, crowd-workers, etc.) and promote smarter policy decisions (e.g., land use decisions pertaining to the positioning of retail establishments, incentives and rebates for businesses).
Individual and collective mobility has been long-touted as a key enabler of urban planning studies. With everyday artefacts that a city's population interacts with being increasingly embedded with hardware (e.g., contact-less smart fare cards that people tap-in and out of buses and metro), and due to the sheer uptake of location-based social media platforms in recent years, a wealth of mobility information is made available for both online and offline processing. This thesis makes two principal contributions -- it explores how such abundantly available mobility information can be (a) integrated with other urban data to provide aggregated insights into demand for urban resources, and (b) used to understand relationships among people and predict their movement behavior (including deviations from normal patterns). Additionally, this thesis introduces opportunities and offers preliminary evidence of how mobility information can be used to support a more efficient urban sensing infrastructure.
First, the thesis explores how mobility can be combined with other urban data for better policy decisions and resource utilization prediction. It first investigates how aggregate mobility data from heterogeneous sources such as public transportation and social media, can aid in quantifying urban constructs (e.g., customer visitation patterns, mobility dynamics of neighborhoods) and then demonstrate their use, as an example, in predicting the survival chances of individual retailers, a key performance measure of land use decisions of a city.
In the past, studies have relied on the predictability of mobility to generate various urban insights. In a complementary effort, by demonstrating the ability to predict instances of unpredictability, sufficiently in advance, this thesis explores opportunities to proactively optimize urban operations by harnessing such unpredictability. First it looks at individual mobility at campus-scale, to discover and quantify social ties. It then describes a framework to detect episodes of future anomalous mobility using social tie-aware mobility information, and then use such early warnings to demonstrate its use in an exemplar smart campus application; task assignments of workers of a mobility-aware crowd-sourcing platform.
In a final exposition of emerging possibilities of using mobility for real-time, operational optimization, I introduce a paradigm for collaboration between co-located sensors in dense deployments that exploits human mobility, at short spatio-temporal scales. As preliminary work, this thesis investigates how associations between densely co-located cameras with partially overlapping views can reinforce inferences for better accuracy, and offers evidence of the feasibility to run adaptive, light-weight operations of deep learning networks that drastically cut down on processing latencies.
This thesis provides additional examples of real--time, in-situ, mobility-driven urban applications, and concludes with key future directions.
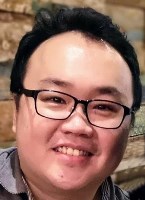
The explosive growth of the ecosystem of personal and ambient computing de- vices coupled with the proliferation of high-speed connectivity has enabled ex- tremely powerful and varied mobile computing applications that are used every- where. While such applications have tremendous potential to improve the lives of impaired users, most mobile applications have impoverished designs to be inclusive– lacking support for users with specific disabilities. Mobile app designers today haveinadequate support to design existing classes of apps to support users with specific disabilities, and more so, lack the support to design apps that specifically target these users. One way to resolve this is to use an empathetic computing system to let designer-developers step into the shoes of impaired users and experience the impairment while evaluating the designs of mobile apps.
A key challenge to enable this is in supporting real-time naturalistic interactions in an interaction environment that maintains consistency between the user’s tactile, visual and proprioceptive perceptions with no perceivable discontinuity. This has to be performed within the context of an immersive virtual environment, which allows control of any visual or auditory artefacts to simulate impairments. To achieve this, substantial considerations of the interaction experience and coordination between the various system components are required.
We designed Empath-D, an augmented virtuality system that addresses this chal- lenge. I show in this dissertation that through the use of naturalistic interaction in augmented virtuality, the immersive simulation of impairments can better support identifying and fixing impairment specific problems in the design of mobile appli- cations.
The dissertation was validated in the following way. I first demonstrate that the concept of immersive evaluation results in lower mental demands for designers in a design study. I then show that Empath-D despite the latencies introduced through creating the augmented virtuality, is usable, and has interaction performance closely matching physical interaction that is sufficient for most application uses, except where rapid interaction is required, such as in games. Next, I show that Empath-D is capable of simulating impairments such as to produce similar interaction perfor- mance. Finally, in an extensive user study, I demonstrate that Empath-D is able to identify more usability problems for specific impairments than with state of the art tools.
This thesis, to the best of my knowledge, is the first of its kind work to i) design and examine an augmented virtuality interface that supports naturalistic interaction with a mobile device, and ii) examine the impact of immersive simulations of im- pairments in evaluating the designs of mobile applications for accessibility.