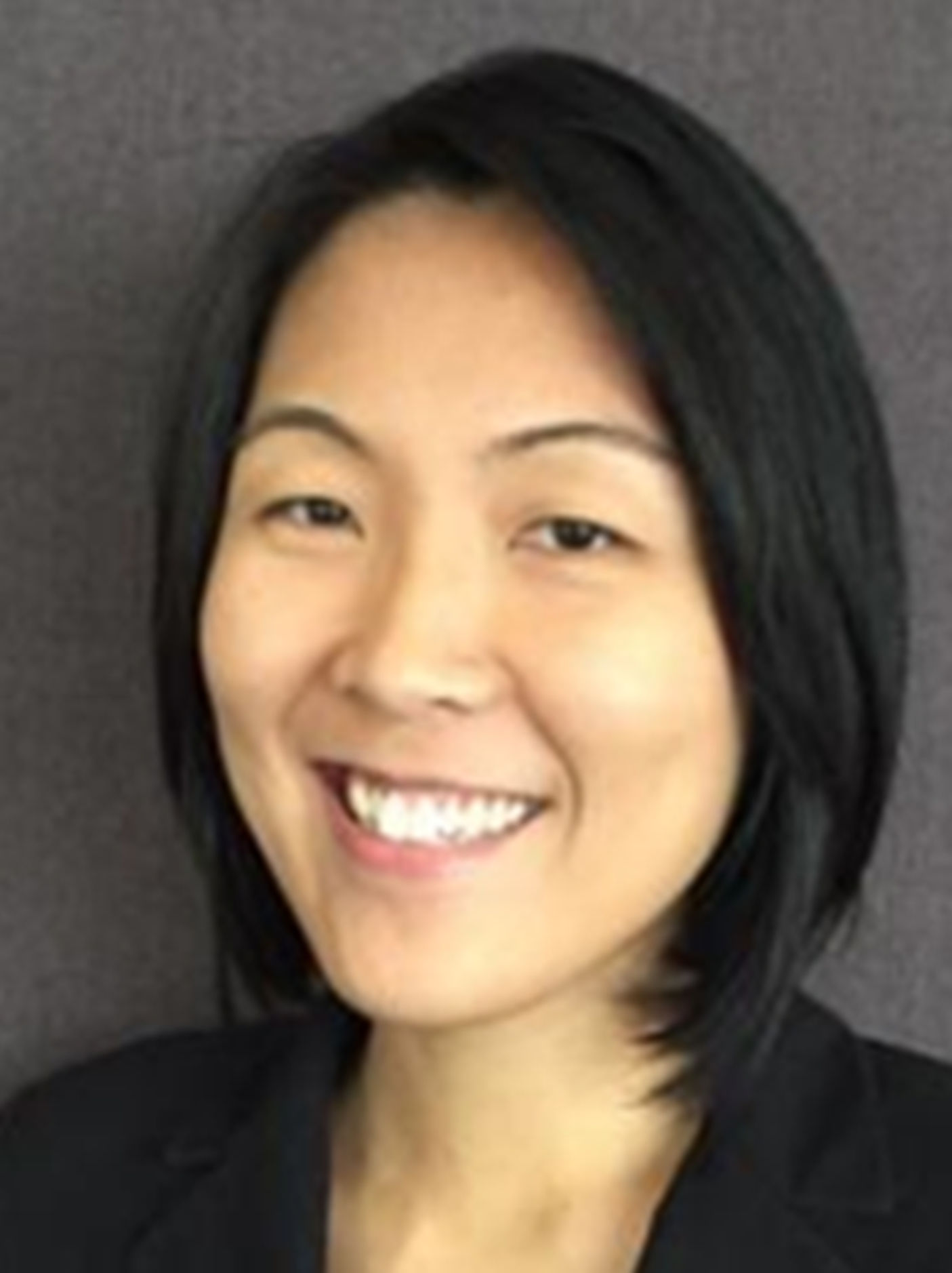
CSR is receiving significant attention from both academics and businesses. However, CSR and economic goals are still perceived as conflicting. To address this gap, this dissertation put together three essays that to inform about the instrumental value of CSR and how CSR could be compatible with economic objectives.
Chapter Two reveals CSR reporting has strategic value for firms by improving firms' relationships with stakeholders and facilitating the development of sustainable development capabilities. Firms are responsive to shareholders' and governments' demands for reporting. As society increasingly demands for CSR reporting, the overlooked issue of costs and benefits of reporting is costly and counterproductive to sustainable development goals. I propose a collaborative, parsimonious and fine-grained regulatory approach, focusing on issue saliency and issue-specific regulations to address the gap.
Chapter Three explores the interplay between financial performance and CSR in business practices. Drawing on behavioral economics, it investigates how relational rationality, emphasizing stakeholder relationship preservation, and economic efficient rationality, prioritizing resource conservation, influence CSR strategizing. The paper highlights the need for CSR strategizing to facilitate resource access for profitability-related projects and complements overall firms’ strategy. The essay conceptualizes resource conservation and relationship preservation mechanisms, providing insights into how firms allocate resources to economic and social objectives.
Chapter Four examines the contingencies of the CSR-performance relationship. Integrating trust research with signaling theory, this paper proposes that perceived trustworthiness influences the credibility of CSR signals. Specifically, this study examines how the propensity to trust and category-based trust moderate the association between CSR and firm performance. This paper address an overlooked gap that stakeholders may perceive firms' CSR differently across countries, therefore influencing the CSR-performance relationship and informing businesses to invest in trust-building strategies.
In this thesis, we develop novel nonparametric estimation techniques for two distinct classes of models: (1) Generalized Additive Models with Unknown Link Functions (GAMULF) and (2) Generalized Panel Data Transformation Models with Fixed Effects. Both models avoid parametric assumptions on their respective link or transformation functions, as well as the distribution of the idiosyncratic error terms.
The first chapter aims to provide an in-depth and systematic introduction to cross- sectional and panel-data nonparametric transformation models, encompassing practical applications, a diverse range of estimation techniques, and the study of asymptotic properties. We discuss the advantages and limitations of these models and estimation methods, delving into the latest advancements and innovations in the field. Furthermore, we propose a potential approach to mitigate the curse of dimensionality in the context of fully nonparametric transformation models with fixed effects in panel-data settings.
The second chapter proposes a three-stage nonparametric least squares (NPLS) estimation procedure for the additive functions in the GAMULF. In the first stage, we estimate conditional expectation by the local-linear kernel regression and then apply matching method to the splines series to obtain initial estimators. In the second stage, we use the local-polynomial kernel regression to estimate the link function. In the third stage, given the estimators in Stages 1 and 2, we apply the local-linear kernel regression to refine the initial estimator. The great advantage of such a procedure is that the estimators obtained at all stages have closed-form expressions, which overcomes the computational hurdle for existing estimators of the GAMULF model.
The third chapter proposes a multiple-stage Local Maximum Likelihood Estimator (LMLE)
for the structural functions in the generalized panel data transformation model with fixed effects. In the first stage, we apply the regularized logistic sieve method to estimate the sieve coefficients associated with the approximation of a composite function and then apply a matching method to obtain initial consistent estimators of the additive structural functions. In the second stage, we apply the local polynomial method to estimate certain composite function and its derivatives to be used later on. In the third stage, we apply the local linear method to obtain the refined estimator of the additive structural functions based on the estimators obtained in Steps 1 and 2. The greatest advantage is that all minimization problems are convex and thus overcome the computational hurdle for existing approaches to the generalized panel data transformation model.
The final estimates of the additive terms in two models achieve the optimal one-dimensional convergence rate, asymptotic normality and oracle efficiency. The Monte Carlo simulations demonstrate that our new estimator performs well in finite samples.
The thesis demonstrates the effectiveness of the proposed nonparametric estimation techniques in addressing the complexities of generalized additive models with unknown link functions and panel data transformation models with fixed effects.
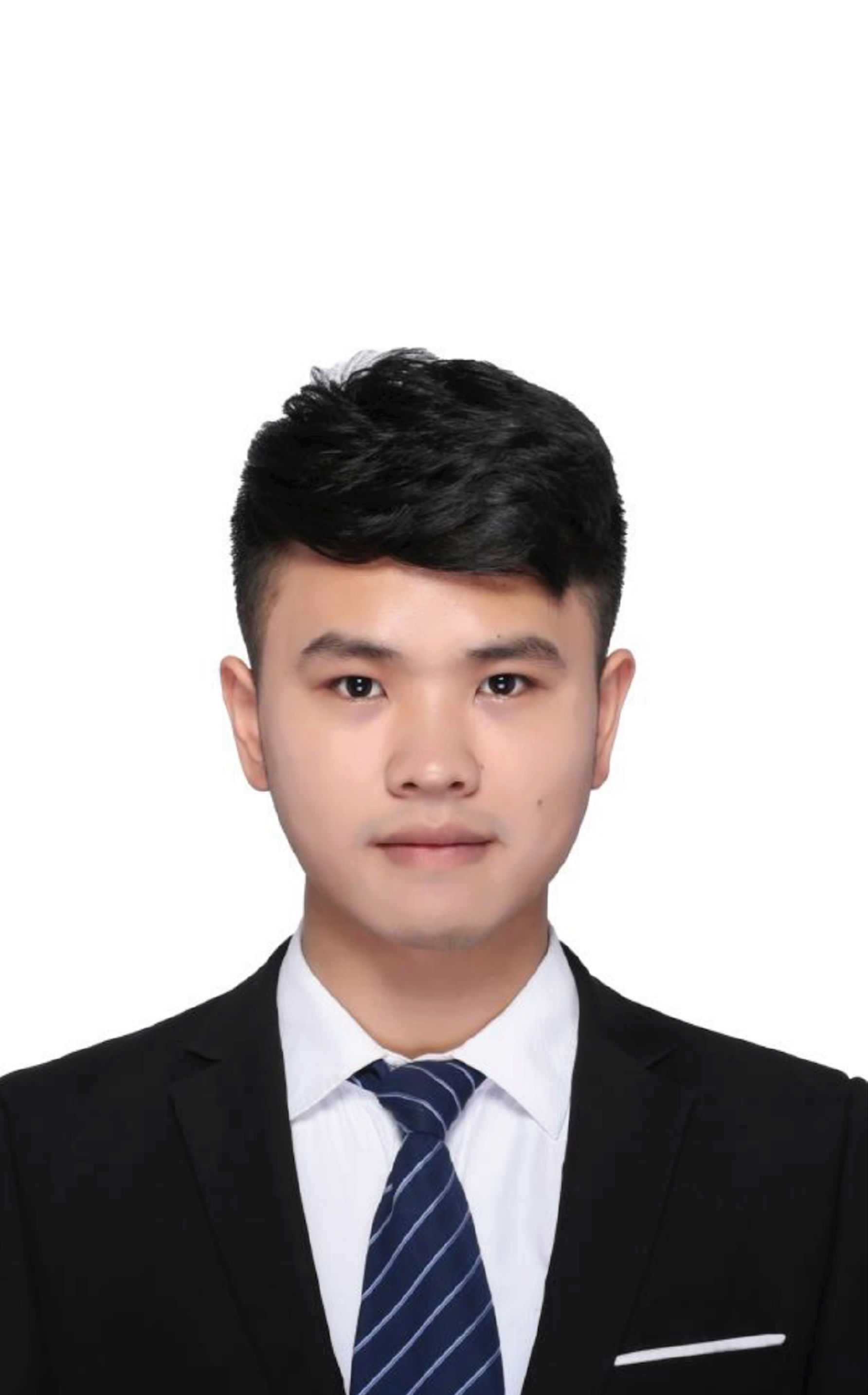
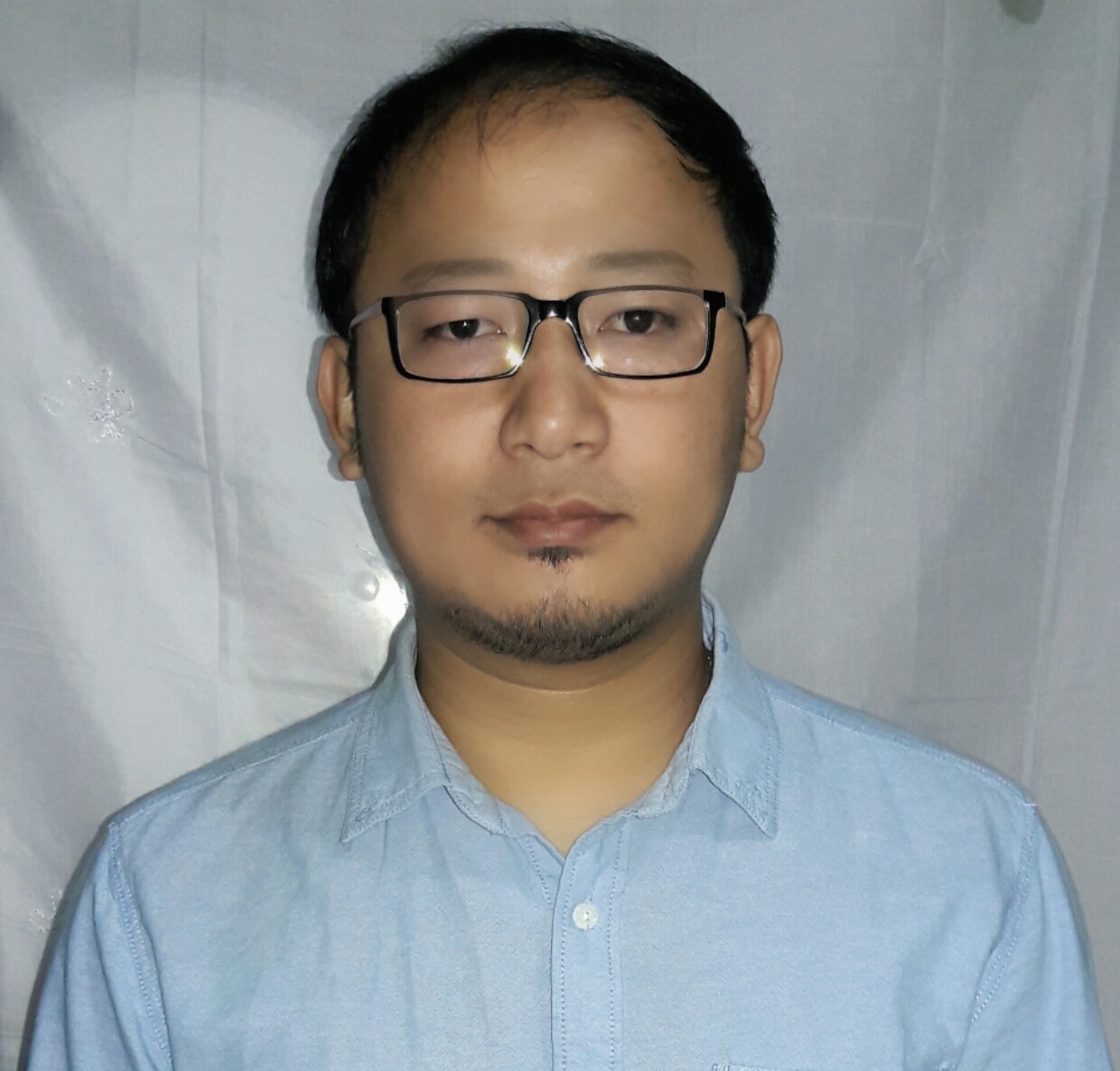
In the current age, rapid growth in sectors like finance, transportation etc., involve fast digitization of industrial processes. This creates a huge opportunity for next-generation artificial intelligence system with multiple agents operating at scale. Multiagent reinforcement learning (MARL) is the field of study that addresses problems in the multiagent systems. In this thesis, we develop and evaluate novel MARL methodologies that address the challenges in large scale multiagent system with cooperative setting. One of the key challenge in cooperative MARL is the problem of credit assignment. Many of the previous approaches to the problem relies on agent's individual trajectory which makes scalability limited to small number of agents. Our proposed methodologies are solely based on aggregate information which provides the benefit of high scalability. The dimension of key statistics does not change with increasing agent population size. In this thesis we also address other challenges that arise in MARL such as variable duration action, and also some preliminary work on credit assignment with sparse reward model.
The first part of this thesis investigates the challenges in a maritime traffic management (MTM) problem, one of the motivating domains for large scale cooperative multiagent systems. The key research question is how to coordinate vessels in a heavily trafficked maritime traffic environment to increase the safety of navigation by reducing traffic congestions. MTM problem is an instance of cooperative MARL with shared reward. Vessels share the same penalty cost for any congestions. Thus, it suffer from the credit assignment problem. We address it by developing a vessel-based value function using aggregate information, which performs effective credit assignment by computing the effectiveness of the agent’s policy by filtering out the contributions from other agents. Although this first approach achieved promising results, its ability to handle variable duration action is rather limited, which is a crucial feature of the problem domain. Thus, we address this challenge using hierarchical reinforcement learning, a framework for control with variable duration action. We develop a novel hierarchical learning based approach for the maritime traffic control problem. We introduce a notion of meta action a high level action that takes variable amount time to execute. We also propose an individual meta value function using aggregate information which effectively address the credit assignment problem.
We also develop a general approach to address the credit assignment problem for a large scale cooperative multiagent system for both discrete and continuous actions settings. We extended a shaped reward approach known as difference rewards (DR) to address the credit assignment problem. DRs are an effective tool to tackle this problem, but their computation is known to be challenging even for small number of agents. We propose a scalable method to compute difference rewards based on the aggregate information. One limitation of this DR based approach for credit assignment is that it relies on learning a good approximation of reward model. But, in a sparse reward setting agents do not receive any informative immediate reward signal until the episode ends, so this shaped reward based approach is not effective in sparse reward case. In this thesis, we also propose some preliminary work in this direction.
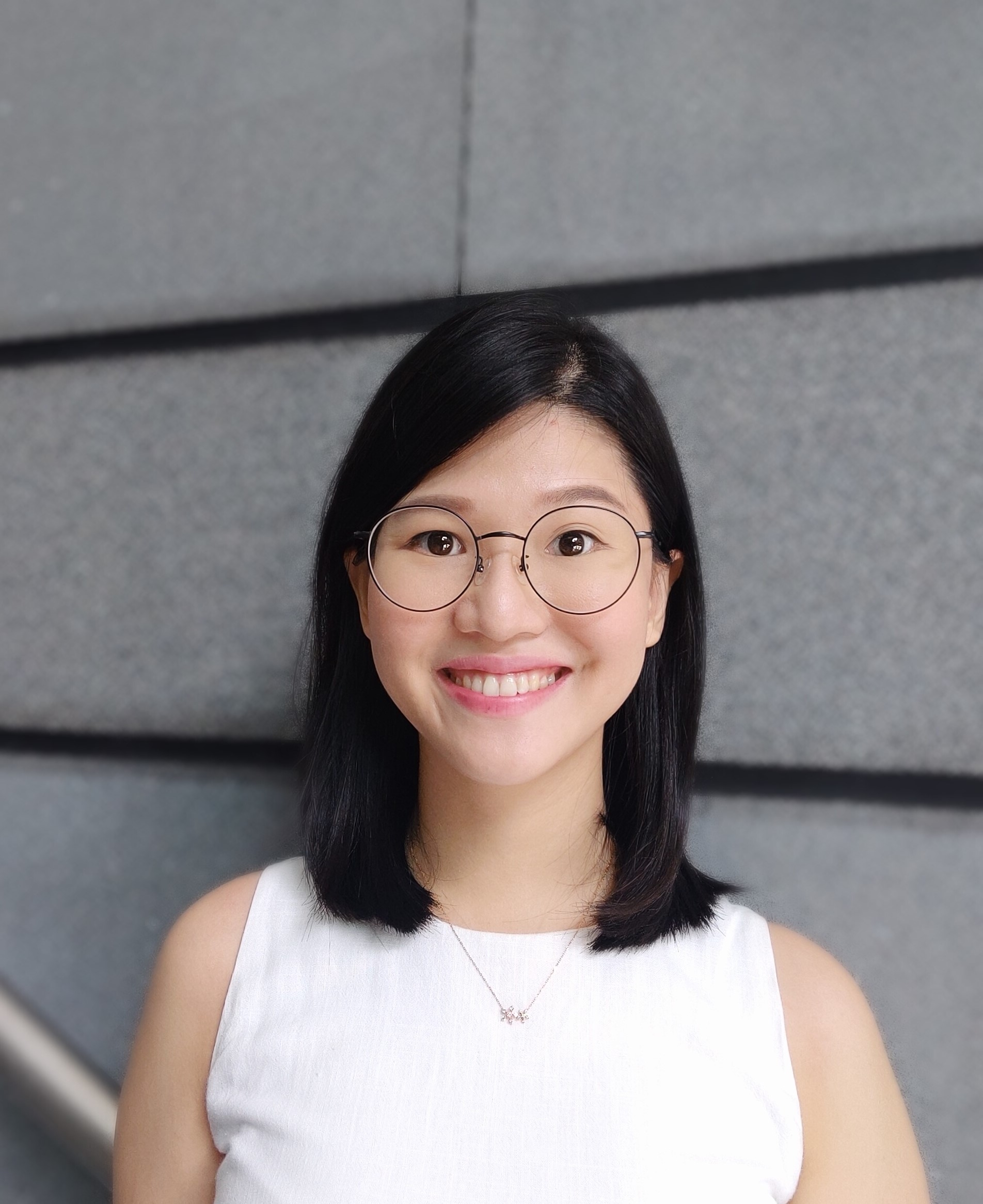
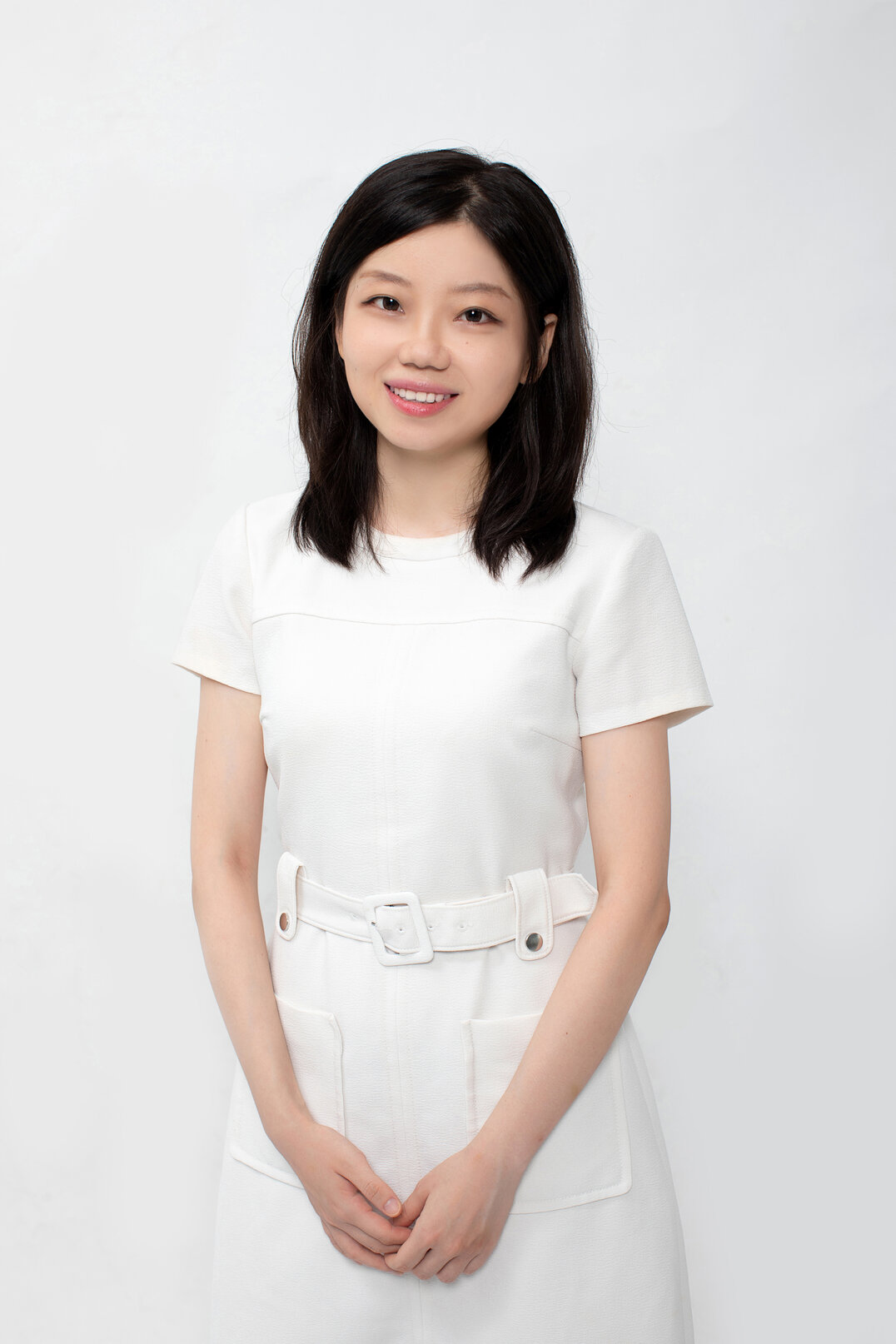
Information Acquisition and Market Friction
My dissertation consists of three papers related to information diversity, acquisition, and asymmetry. One part of the dissertation explores the implications of interactions among different market participants and subsequent price efficiency in the stock market. The empirical findings indicate the information diversity between individuals and institutional investors, as well as an important channel for retail investors to obtain useful information – through insider filings. The remaining part investigates the information asymmetry between issuers and naive investors in the cryptocurrency market. In Chapter 2, I aggregate trading signals from hedge funds and retail investors, in order to examine their information diversity and the combined informational role in the stock market. I show that incorporating signals from both groups is necessary to identify firm-level information. Stocks that reflect consistent trading between two groups exhibit strong return predictability without reversal. When trading in the opposite direction to retail investors, hedge funds cannot yield any significant return, even in a longer horizon. I also document that consistent trading between two groups significantly predicts firm fundamentals, informational events, market reactions, and helps alleviate stock-level mispricing. Overall, the findings suggest combining signals that solely from hedge funds is incomplete, as there remain signals from retail investors who are informed in different aspects of stock fundamentals. In Chapter 3, we examine the trading patterns of retail investors following insider trading and the corresponding price impact. Retail investors follow the opportunistic purchases by insiders, but not their routine purchases. The abnormal retail downloads of the Form 4 filings from the EDGAR database also increase for opportunistic insider purchases. Neither investor attention nor common information such as earnings announcements or analysts forecast revisions explains the results. Moreover, for stocks with opportunistic insider purchases, those that retail investors bought yield higher cumulative abnormal returns than those that retail investors sold. The effect is mostly driven by the information component of the retail trades, rather than liquidity provision or temporary price pressure. Variance ratio tests also suggest price efficiency improvements for stocks bought by retail investors following opportunistic insider purchases. The evidence is mostly consistent with retail investors learning from opportunistic insider purchases, and their trading helping expedite price discovery. In Chapter 4, we study the economics of financial scams by investigate the market for initial coin offerings (ICOs) using point-in-time data snapshots of 5,935 ICOs. Our evidence indicates that ICO issuers strategically screen for na¨ıve investors by misrepresenting the characteristics of their offerings across listing websites. Misrepresented ICOs have higher scam risk, and misrepresentations are unlikely to reflect unintentional mistakes. Using on-chain analysis of Ethereum wallets, we find that less sophisticated investors are more likely to invest in misrepresented ICOs. We estimate that 40% of ICOs (U.S. $12 billion) in our sample are scams. Overall, our findings uncover how screening strategies are used in financial scams and reinforce the importance of conducting due diligence.
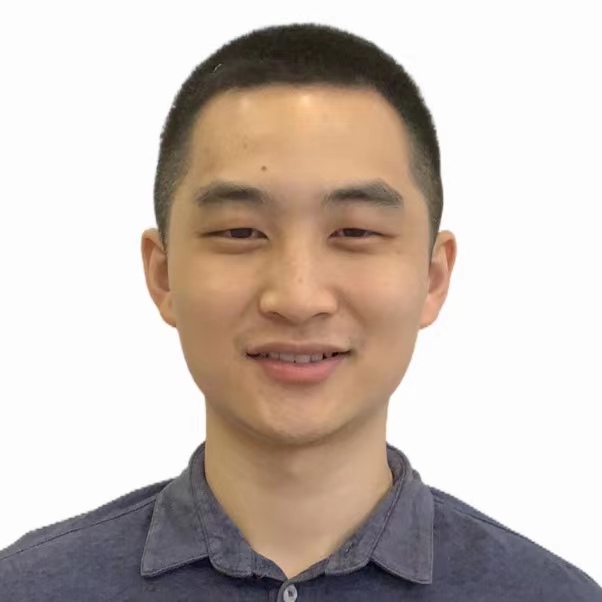
Nowadays, software question and answer (SQA) data has become a treasure for software engineering as it contains a huge volume of programming knowledge. That knowledge can be interpreted in many different ways to support various software activities, such as code recommendation, program repair, and so on. In this dissertation, we interpret SQA data by addressing three novel research problems.
The first research problem is about linkable knowledge unit prediction. In this problem, a question and its answers within a post in Stack Overflow are considered as a knowledge unit (KU). KUs often contain semantically relevant knowledge, and thus linkable for different purposes. Being able to classify different classes of linkable knowledge units would support more targeted information needs when users search or explore the linkable knowledge. Compare with the approaches proposed in prior works, we design a relatively simpler but more effective machine learning model to address the problem. Moreover, we discover the limitation of the dataset used in the previous works and construct a new one with a larger size and higher diversity. Our experimental result shows that our model outperforms the state-of-the-art approaches significantly.
The second research problem is about distributed representation for Stack Overflow posts. In this dissertation, we propose a specialized deep learning architecture Post2Vec which extracts distributed representations of Stack Overflow posts. To evaluate Post2Vec, we first investigate its end-to-end effectiveness in tag recommendation task. We observe that Post2Vec achieves significant improvement in terms of F1-score@5 at a lower computational cost. Moreover, to evaluate the value of representations learned by Post2Vec, we use them for three other tasks, i.e., relatedness prediction, post classification, and API recommendation. We demonstrate that the representations can be used to boost the effectiveness of state-of-the-art solutions for the three tasks by substantial margins.
The third research problem is about answer summary generation for technical questions. We formulate the task as a query-focused multi-answer-posts summarization task for a given technical question. We conduct user studies to evaluate the quality of the answer summaries generated by our approach. The user study results demonstrate those answer summaries generated by AnswerBot are relevant, useful, and diverse.
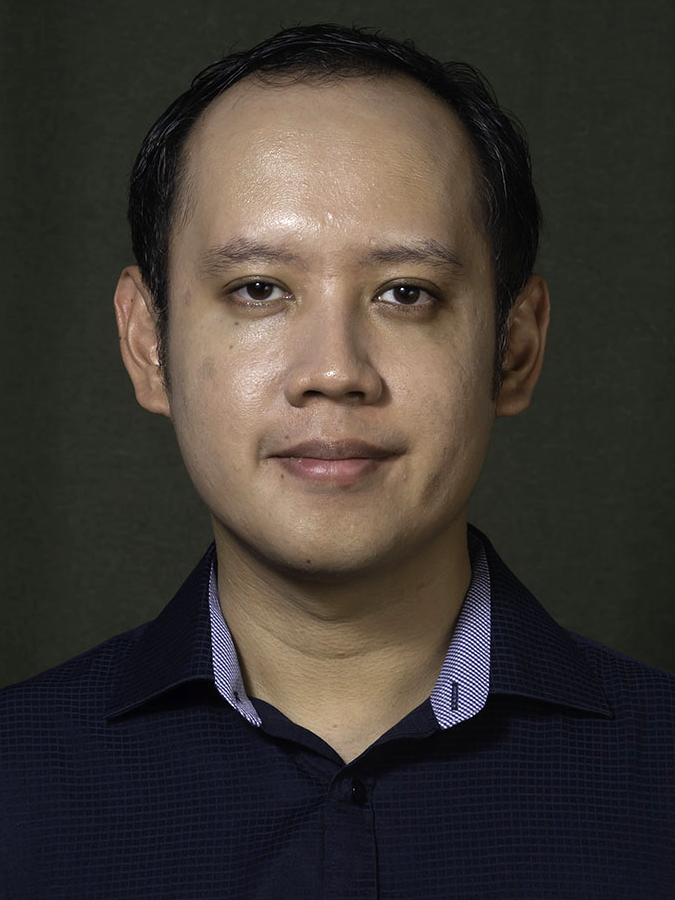
The code hosting platform GitHub has gained immense popularity worldwide in recent years, with over 200 million repositories hosted as of June 2021. Due to its popularity, it has great potential to facilitate widespread improvements across many software projects. Naturally, GitHub has attracted much research attention, and the source code in the various repositories it hosts also provide opportunity to apply techniques and tools developed by software engineering researchers over the years. However, much of existing body of research applicable to GitHub focuses on code quality of the software projects and ways to improve them. Fewer work focus on potential ways to improve quality of GitHub repositories through other aspects, although quality of a software project on GitHub is also affected by factors outside a project's source code, such as documentation, the project's dependencies, and pool of contributors.
The three works that form this dissertation focus on investigating aspects of GitHub repositories beyond the code quality, and identify specific potential improvements that can be applied to improve wide range of GitHub repositories. In the first work, we aim to systematically understand the content of README files in GitHub software projects, and develop a tool that can process them automatically. The work begins with a qualitative study involving 4,226 README file sections from 393 randomly-sampled GitHub repositories, which reveals that many README files contain the ``What'' and ``How'' of the software project, but often do not contain the purpose and status of the project. This is followed by a development and evaluation of a multi-label classifier that can predict eight different README content categories with F1 of 0.746. From our subsequent evaluation of the classifier, which involve twenty software professionals, we find that adding labels generated by the classifier to README files ease information discovery.
Our second work focuses on characteristics of vulnerabilities in open-source libraries used by 450 software projects on GitHub that are written in Java, Python, and Ruby. Using an industrial software composition analysis tool, we scanned every version of the projects after each commit made between November 1, 2017 and October 31, 2018. Our subsequent analyses on the discovered library names, versions, and associated vulnerabilities reveal, among others, that ``Denial of Service'' and ``Information Disclosure'' vulnerability types are common. In addition, we also find that most of the vulnerabilities persist throughout the observation period, and that attributes such as project size, project popularity, and experience level of commit authors do not translate to better or worse handling of vulnerabilities in dependent libraries. Based on the findings in the second work, we list a number of implications for library users, library developers, as well as researchers, and provide several concrete recommendations. This includes recommendations to simplify projects' dependency sets, as well as to encourage research into ways to automatically recommend libraries known to be secure to developers.
In our third work, we conduct a multi-region geographical analysis of gender inclusion on GitHub. We use a mixed-methods approach involving a quantitative analysis of commit authors of 21,456 project repositories, followed by a survey that is strategically targeted to developers in various regions worldwide and a qualitative analysis of the survey responses. Among other findings, we discover differences in diversity levels between regions, with Asia and Americas being highest. We also find no strong correlation between gender and geographic diversity of a repository's commit authors. Further, from our survey respondents worldwide, we also identify barriers and motivations to contribute to open-source software. The results of this work provides insights on the current state of gender diversity in open source software and potential ways to improve participation of developers from under-represented regions and gender, and subsequently improve the open-source software community in general. Such potential ways include creation of codes of conduct, proximity-based mentorship schemes, and highlighting of women / regional role models.
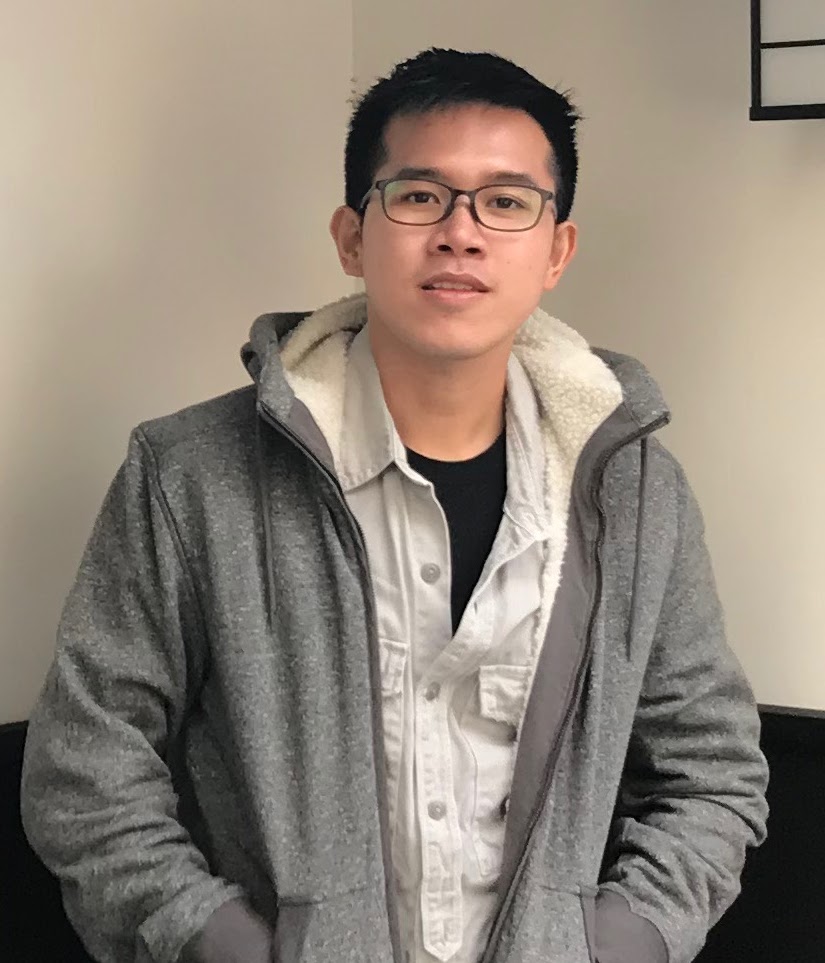
In recent years, we have witnessed significant progress in building systems with artificial intelligence. However, despite advancements in machine learning and deep learning, we are still far from achieving autonomous agents that can perceive multi-dimensional information from the surrounding world and converse with humans in natural language. Towards this goal, this thesis is dedicated to building intelligent systems in the task of video-grounded dialogues. Specifically, in a video-grounded dialogue, a system is required to hold a multi-turn conversation with humans about the content of a video. Given an input video, a dialogue history, and a question about the video, the system has to understand contextual information of dialogue, extract relevant information from the video, and construct a dialogue response that is both contextually relevant and video-grounded. Compared to related research domains in computer vision and natural language processing, the video-grounded dialogue task raises challenging requirements, including: (1) language reasoning in multiple turns: the ability to understand contextual information from dialogues, which often consist of linguistic dependencies from turn to turn; (2) visual reasoning in spatio-temporal space: the ability to extract information from videos, which contain both spatial and temporal variations that characterize object appearance and actions; and (3) language generation: the ability to acquire natural language and generate responses with both contextually relevant and video-grounded information. Towards building an intelligent system for the video-grounded dialogue task, we introduced a neural model, Multimodal Transformer Network (MTN), that can be trained in an end-to-end manner to reason over both dialogue and video inputs and decode a natural language response. The architecture was tested against the established benchmark Audio-Visual Scene-Aware Dialogue (AVSD) and achieved superior performance from other neural-based systems. Despite this success, we found that MTN is not specifically designed for scenarios that require sophisticated visual or language reasoning. To further improve the reasoning capability of models in visual aspects, we introduced BiST, a Bidirectional Spatio-Temporal Reasoning approach that can extract relevant visual cues in videos in both spatial and temporal dimensions. This approach achieved consistent performance in both quantitative and qualitative results. However, our findings show that in many scenarios, systems failed to learn the contextual information of dialogue, which may lead to incorrect or incoherent system responses. To address this limitation, we focused our attention on the language reasoning capability of models. We proposed PDC, a path-based reasoning approach for dialogue context. PDC requires systems to learn to extract a traversal path among dialogue turns in the dialogue context. Our findings demonstrate the performance gains of this approach as compared to sequential or graph-based learning approaches. To combine both visual and language reasoning, we adopted compositionality to encode questions as a sequential reasoning program. The program is parameterized by entities and actions which are used to extract more refined features from video inputs. We denoted this approach as Video-grounded Neural Module Network (VGNMN). From experiments with VGNMN, we found not only potential performance gains in automatic metrics but also improved interpretability through learned reasoning programs. In video-grounded dialogue research, we found a major obstacle that hindered our progress: limitation of data. While there are very limited video-grounded dialogue data available, developing a new benchmark involves costly and time-consuming manual annotation efforts. The data limitation essentially prevents a system from acquiring sufficient natural language understanding. We then proposed to make use of pretrained language models such as GPT, to leverage their linguistic dependencies learned from large-scale text data. In another work, we adopted causality to augment current data with counterfactual samples that support model training. Our findings show that both pretrained systems and data augmentation are effective strategies to alleviate the data limitation. To facilitate further research in this field, we developed DVD, a Diagnostic Video-grounded Dialogue benchmark. We built DVD as a diagnostic and synthetic benchmark to fairly evaluate systems by visual and textual complexity. We tested several baselines, from simple heuristic models to complex neural networks, and found that all models are inefficient in different aspects, from multi-turn textual references to visual object tracking. Our findings suggest that current approaches still perform poorly in DVD and future approaches should be integrated with multistep and multi-modal reasoning capabilities. In view of the above findings, we developed a new sub-task within video-grounded dialogue systems. We introduced Multimodal Dialogue State Tracking (MM-DST) task, which requires a system to maintain a recurring memory or state of all visual objects that are mentioned in dialogue context. At each dialogue turn, dialogue utterances may introduce new visual objects or new object attributes, and a dialogue system are required to update the states of these objects. We leveraged techniques from the research of task-oriented dialogues, introduced a new baseline, and discussed our findings. Finally, we concluded the dissertation with a summary of our contributions and a discussion of potential future directions in video-grounded dialogue research.
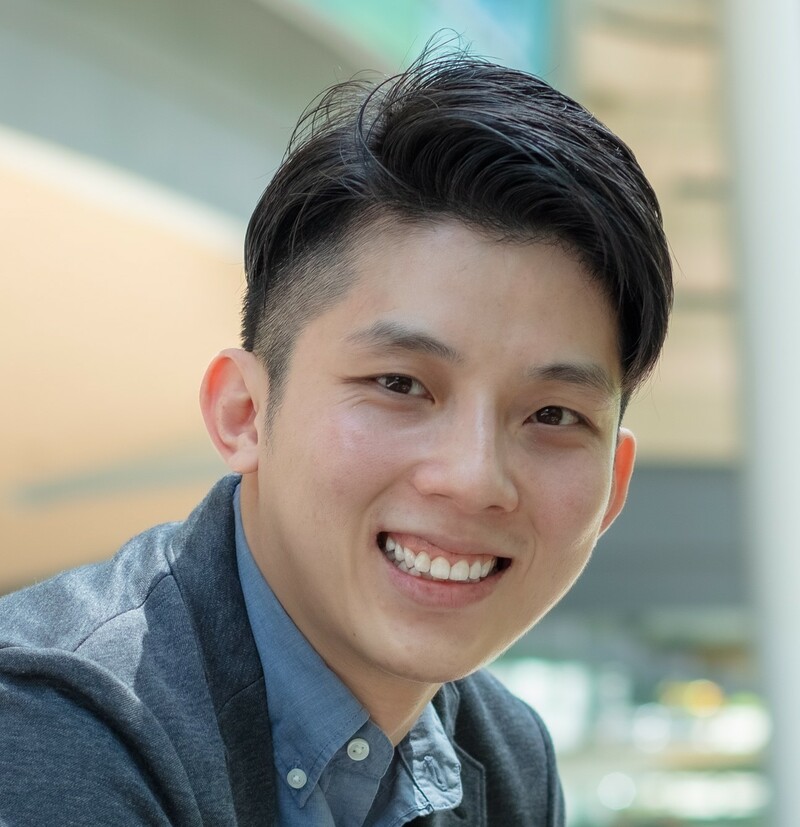
Extant research has demonstrated robust positive relations between positive affect (PA) and meaning, although the strength of this relationship has been found to vary as a function of both chronological age and time horizon (Hicks et al., 2012). This can be explained by the Socioemotional Selectivity Theory (SST), which posits that both older adults and those with a limited time horizon (i.e., perceive less remaining in life) tend to focus on emotional goals over knowledge goals. In the current paper, I sought to extend SST’s findings to the level of activities by examining how chronological age, time horizon (both existing and manipulated), and one’s focus on emotional/knowledge goals influenced the strength of the relationship between the enjoyableness and meaningfulness of specific activities. These hypotheses were tested using an older (Study 1) and a younger adult sample (Study 2). Although none of the hypothesized relations were fully supported, interesting relations were uncovered through exploratory analyses that examined specific activities in terms of their experiential qualities and the joint effects of both positive (PA) and negative affect (NA) on activity-related meaning perceptions. In older adults, I found that for those with a limited time horizon, high-PA activities were less meaningful when also accompanied by NA. In contrast, for those with an expansive time horizon, high-PA activities remained meaningful even when accompanied by NA. In younger adults, I found that those who prioritized emotional goals experienced less meaning from uniformly negative activities compared to those who prioritized knowledge goals. Theoretical and practical implications of the current study are discussed.
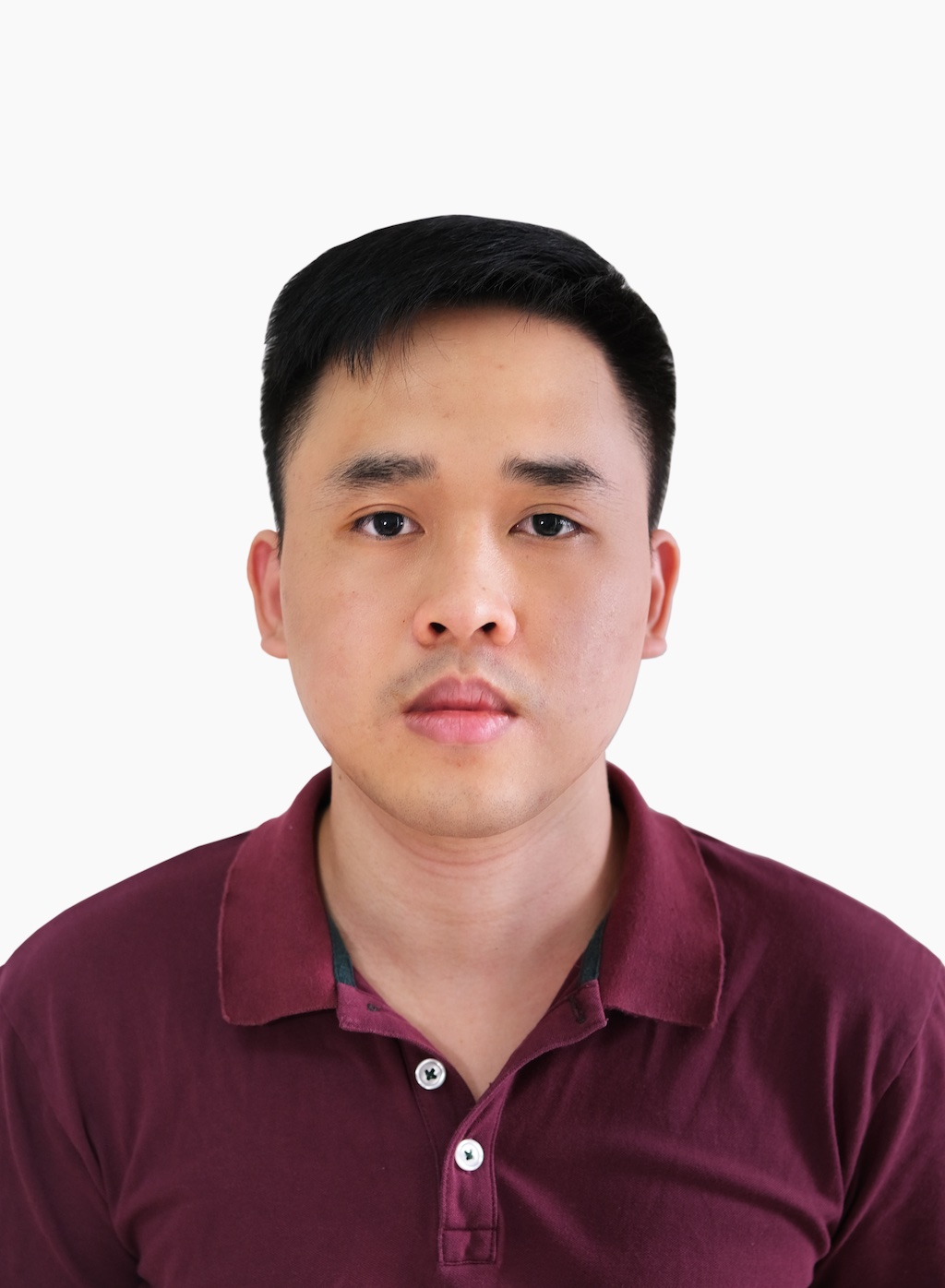
Online reviews are prevalent in many modern Web applications, such as e-commerce, crowd-sourced location and check-in platforms. Fueled by the rise of mobile phones that are often the only cameras on hand, reviews are increasingly multimodal, with photos in addition to textual content. In this thesis, we focus on modeling the subjectivity carried in this form of data, with two research objectives.
In the first part, we tackle the problem of detecting sentiment expressed by a review. This is a key unlocking many applications, e.g., analyzing opinions, monitoring consumer satisfaction, assessing product quality.
Traditionally, the task of sentiment analysis primarily relies on textual content. We focus on the visual sentiment of review images and develop models to systematically analyze the impact of three factors: image, user, and item. Further investigation leads to a notion of concept-orientation generalizing visual sentiment analysis for Web images. Then, we observe that in many cases, with respect to sentiment detection, images play a supporting role to text, highlighting the salient aspects of an entity, rather than expressing sentiments independently. Therefore, we develop a visual aspect attention mechanism that relies on visual information as alignment for pointing out the important sentences of a document.
The method is effective for a scenario of one document being associated with multiple images, such as online reviews, blog posts, social networks, and media articles. Furthermore, we study the utilization of sentiment as an independent modality in the context of cross-modal retrieval. We first formulate the problem of sentiment-oriented text-to-image retrieval and then propose two approaches for incorporating sentiment into text queries based on metric learning. Each approach emphasizes a hypothesis on how the sentiment vectors aligned in the metric space that also includes text and visual vectors.
In the second part, we focus on developing models for capturing user preferences from multimodal data. Preference modeling is crucial to recommender systems which are core to modern online user-based platforms. The need for recommendations is to guide users in browsing the myriad of options offered to them. In online reviews, for instance, preference manifests in numerical rating, textual content, as well as visual images. First, we hypothesize that modeling these modalities jointly would result in a more holistic representation of a review towards more accurate recommendations. Therefore, we propose an approach that captures user preferences via simultaneously modeling a rating prediction component and a review text generation component. Second, we introduce a new generative model of preferences, inspired by the dyadic nature of the preference signals. The model is bilateral making it more apt for bipartite interactions, as well as allowing easy incorporation of auxiliary data from both sides of user and item. Third, we develop a probabilistic framework for modeling preferences involving logged bandit feedback. It helps deal with the sparsity issue in learning from bandit feedback on publisher sites by leveraging relevant organic feedback from e-commerce sites. Through empirical evaluation, we demonstrate that the proposed framework is effective for recommendation and ads placement systems.
In general, we present multiple approaches to modeling various aspects of sentiment and preference signals from multimodal data. Our work contributes a set of techniques that could be broadly extensible for mining Web data. Additionally, this research facilitates the development of recommender systems, which play a significant role in many online user-based platforms.
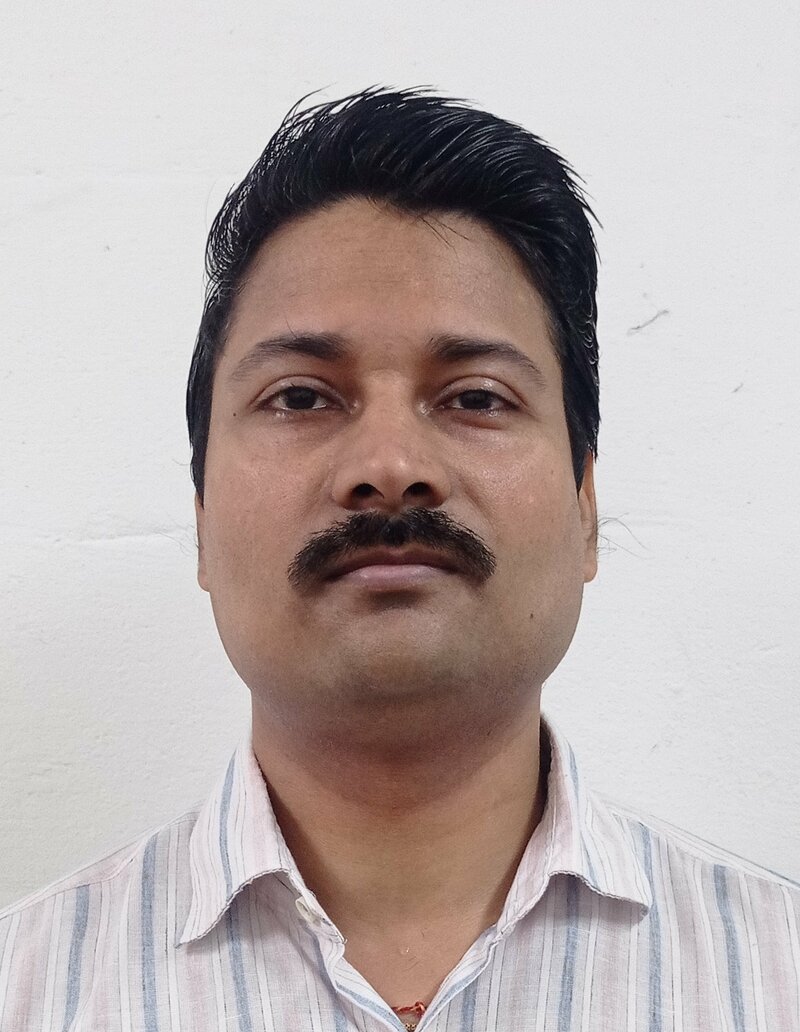
Towards Improving System Performance in Large Scale Multi-Agent Systems with Selfish Agents
Intelligent agents are becoming increasingly prevalent in a wide variety of domains including but not limited to transportation, safety and security. To better utilize the intelligence, there has been increasing focus on frameworks and methods for coordinating these intelligent agents. This thesis is specifically targeted at providing solution approaches for improving large scale multi-agent systems with selfish intelligent agents. In such systems, the performance of an agent depends on not just his/her own efforts, but also on other agent’s decisions. The complexity of interactions among multiple agents, coupled with the large scale nature of the problem domains and the uncertainties associated with the environment, make decision making very challenging. In this work, we specifically study the problem from the perspective of a centralized aggregator, that needs to maximize the revenue of the entire system.
To that end, we study this problem from strategic and operational point of view. With regards to strategic decision making, we propose planning and deep reinforcement learning based solution algorithms to improve the system performance by optimizing the adaptive operating hours of selfish agents and by providing flexible work schedules to them. From operational point of view, we propose novel mechanism to incentivise selfish agents, so that performance of all the agents and the overall system improve . Basically, through strategic and operational decision making, we assist selfish agents in making intelligent decisions that results in improved system performance.
In the first part of this thesis, we focus on making strategic decisions for the workers in the digital gig economy. To provide a concrete context, we focus on taxi drivers in the transport gig economy. Taxi fleets and car aggregation systems are an important component of the urban public transportation system. Taxis and cars in taxi fleets and car aggregation systems (e.g., Uber) are dependent on a large number of self-controlled and profitdriven taxi drivers, which introduces inefficiencies in the system. There are two ways in which taxi fleet performance can be optimized: (i) Operational decision making: improve assignment of taxis/cars to customers, while accounting for future demand; (ii) strategic decision making: optimize operating hours of (taxi and car) drivers. Existing research has primarily focused on the operational decisions in (i) and we focus on the strategic decisions in (ii).
We first model this complex real world decision making problem (with thousands of taxi drivers) as a multi-stage stochastic congestion game with a non dedicated set of agents (i.e., agents start operation at a random stage and exit the game after a fixed time), where there is a dynamic population of agents (constrained by the maximum number of drivers). We provide planning and learning methods for computing the ideal operating hours in such a game, so as to improve efficiency of the overall fleet. In our experimental results, we demonstrate that our planning based approach provides up to 16% improvement in revenue over existing method on a real world taxi dataset. The learning based approach further improves the performance and achieves up to 10% more revenue than the planning approach.
In second part of this thesis, We focus on: a) addressing the problem of handling schedule constraints of individual agents (e.g., breaks during work hours) to provide a flexible work schedule for them; and b) provide a scalable solution approach in such large scale problem settings. We introduced a simulation based (faster) equilibrium computation method that relies on policy imputation. We studied and analyzed different imputation methods and show that a good imputation method coupled with a well designed simulation based best response computation can help in achieving better symmetric equilibrium for large scale systems, in a time efficient manner. We demonstrate that our methods provide significantly better policies than the previous approach in terms of improving individual agent revenue and overall agent availability.
In the third/final part of the thesis, we focus of operational decision making, where we improve system performance by inducing cooperation among selfish agents. Here we focus on principal-agent problem setting. Principalagent relationships, where a principal employs several agents to accomplish tasks on its behalf, are prevalent in many domains (e.g., Manufacturer distributors for product distribution, Uber-taxi drivers for transportation, FoodPanda-delivery personnel for food delivery). Principal has a global observation on all the tasks, while agents only have local observations with regards to local tasks. This limited observability coupled with selfish interest of agents results in a misalignment between Principal and agents objectives. We provide Multi-Agent Reinforcement Learning (MARL) approaches for sequentially designing incentives that improves objectives for principal and agents. We demonstrate that our approaches are able to outperform the state of art approaches for sequential incentive design on Escape-Room and adapted StarCraft-2 environments.
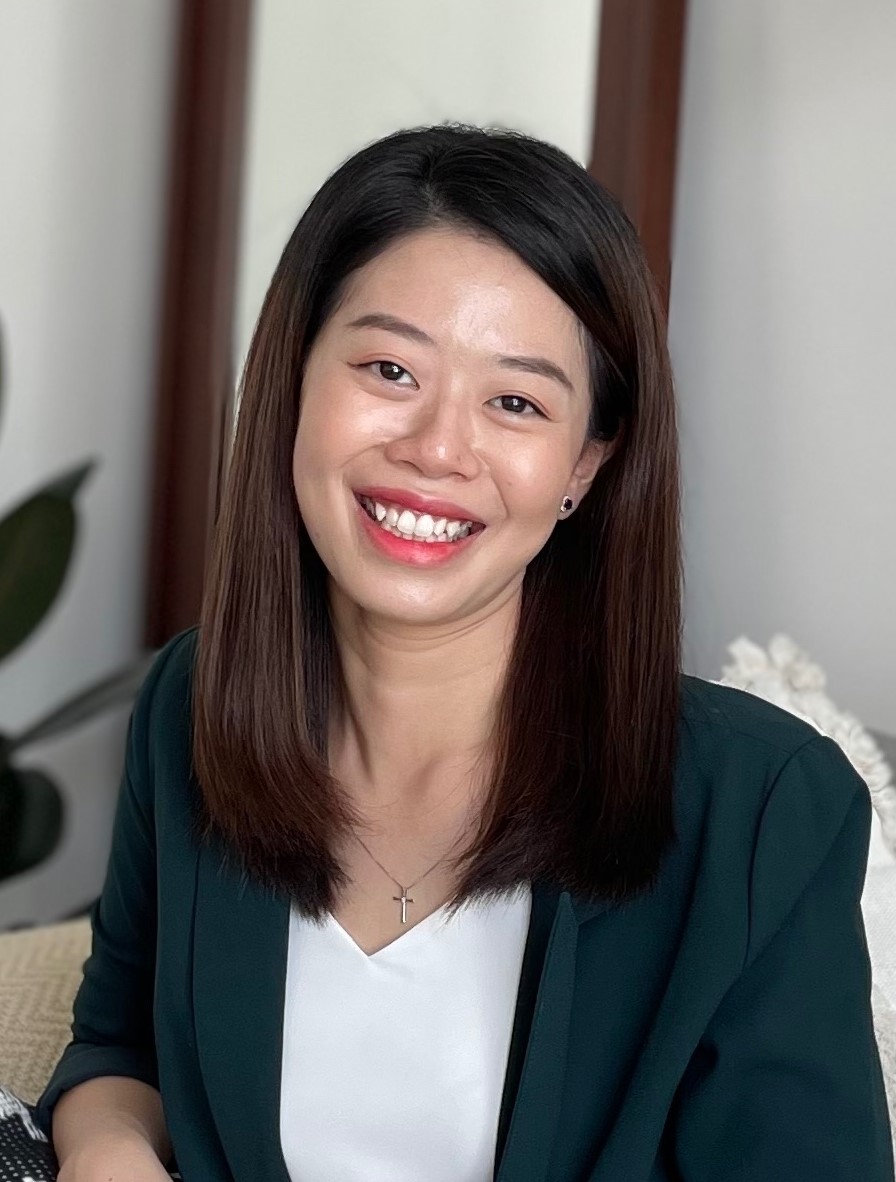
Battling Self-Esteem Issues During SNS Use: A Multilevel Latent Variable Path Analysis Approach
Although studies have consistently indicated that heavier social networking sites (SNS) use perpetuates poorer self‑esteem outcomes, no study has examined potential intervention methods that can counteract the ill-effects of SNS use. We sought to examine whether SNS use in a self-affirmative manner could mitigate threats to self that are often experienced during its use. Specifically, we hypothesized that the viewing of one’s SNS profile (i.e., Instagram profile) would have self-affirmative effects on individuals and improve their self-perception, and these effects are mediated by self‑concept clarity. We tested these hypotheses through cross-sectional (Study 1) and intensive longitudinal (Study 2) studies. Across two studies, we found that participants who spent time on their own Instagram profile felt more positive about themselves. In Study 2, using multilevel latent variable path analyses, we found that SNS-influenced self‑concept clarity mediated the relations between self-affirmative SNS use and SNS-influenced self-esteem. Our findings provide preliminary evidence for our hypothesis that guided SNS use can have beneficial effects on one’s self-perception.
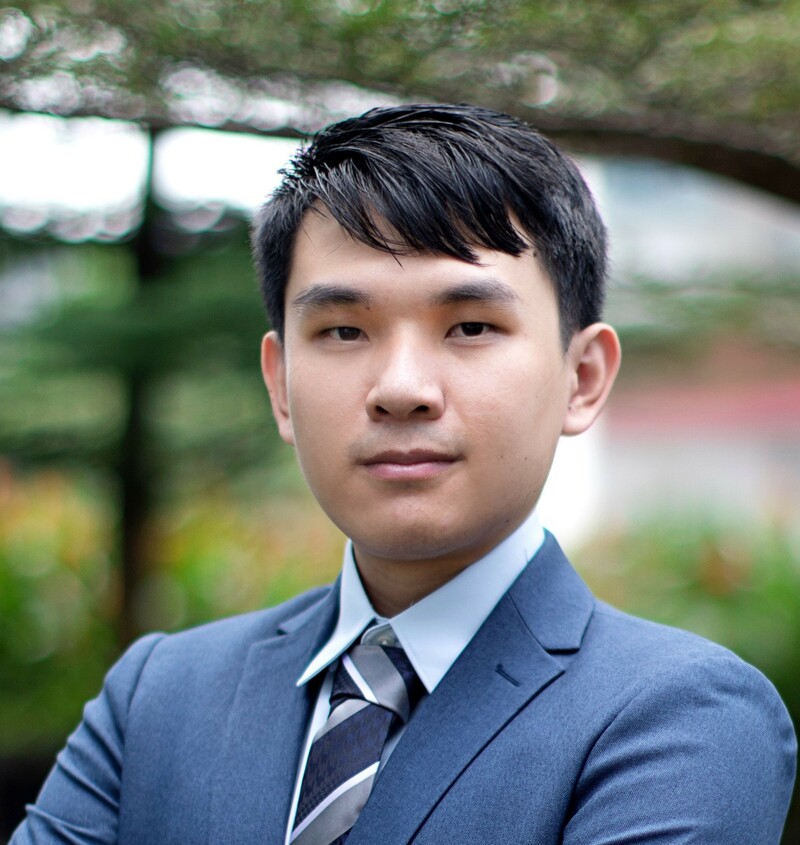
This dissertation studies different long memory models. The first chapter considers a time series regression model where both the regressors and error term are locally stationary long memory processes with time-varying memory parameters, and the regression coefficients are also allowed to be time-varying. We consider a frequency-domain least squares estimator with kernelized discrete Fourier transform and derive its pointwise asymptotic normality and uniform consistency. A specification test on the constancy of coefficients is provided. The second chapter studies a linear regression panel data model with interactive fixed effects where the regressors, factors and idiosyncratic error terms are all stationary but with potential long memory. The setup involves a new factor model formulation for which weakly dependent regressors, factors and innovations are embedded as a special case. Standard methods based on principal component decomposition and least squares estimation, as in Bai (2009), are found to suffer bias correction failure because the order of magnitude of the bias is determined in a complex manner by the memory parameters. To cope with this failure and to provide a simple implementable estimation procedure, frequency domain least squares estimation is proposed. The limit distribution of this frequency domain approach is established and a hybrid selection method is developed to determine the number of factors. The third chapter estimates the memory parameters and test them against spurious long memory of the latent factors in a linear regression model with interactive fixed effects, based on the estimated discrete Fourier transform of the factors. The same asymptotic properties hold as if we use the infeasible true factors for both the memory estimator and the test. This result illustrates how the frequency domain least squares estimator can be applied to further inference other than the regression coefficients.
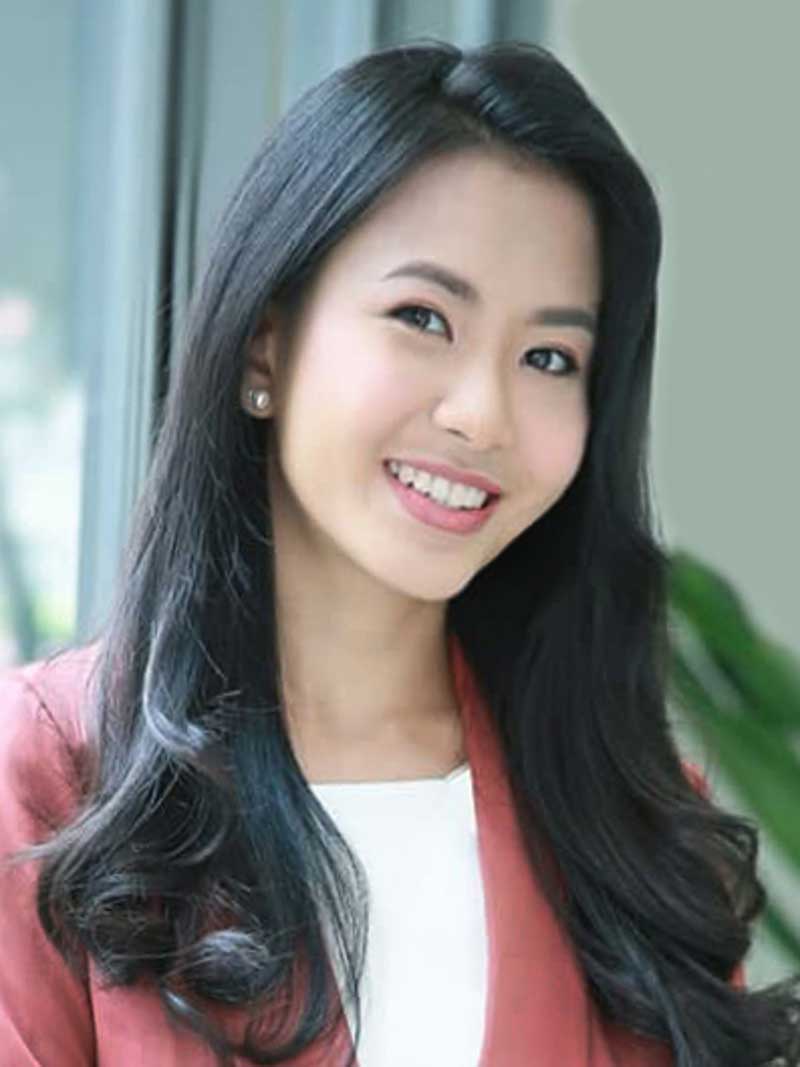
Behavioral spillover occurs when performing an initial behavior increases the likelihood of performing a subsequent behavior (positive spillover) or decreases this likelihood (negative spillover). The current research focuses on negative spillovers of pro-environmental behaviors (PEB), which has the implication of limiting individuals’ environmental conservation efforts. To offer insights, three studies sought to explicate how and for whom negative spillovers would occur. I theorized that prior behaviors would negatively predict subsequent behaviors via greater perceived goal progress and that this negative association between perceived goal progress and subsequent engagement would be more pronounced for people with a strong (vs. weak) promotion focus. This is because promotion-focused individuals are more sensitive to gains (e.g., goal progress) and may discontinue their pursuits when they perceive a positive state has been attained (Zou et al., 2014). Across two studies, self-reported (Study 1, N = 161) and experimentally induced recall (Study 2, N = 481) of prior PEB led to greater perceived goal progress. However, its effect varied with a stronger promotion focus accentuating a negative spillover for PEB intentions in Study 1 but a positive spillover for environmental donation in Study 2. As Study 1 referenced a general collective goal of addressing climate change and Study 2 referenced a personal goal of addressing climate change, Study 3 (N = 501) sought to examine whether the observed differing spillover effects would be moderated by goal framing (i.e., collective vs. personal goal). Negative spillovers may be more pronounced for collective (vs. personal) goals as people feel that they can be relieved of the responsibility for expending further effort toward the collective goals if they have previously contributed. However, Study 3 could not reconcile the inconsistent spillover patterns found in Studies 1 and 2. The implications of these findings and future directions are discussed.
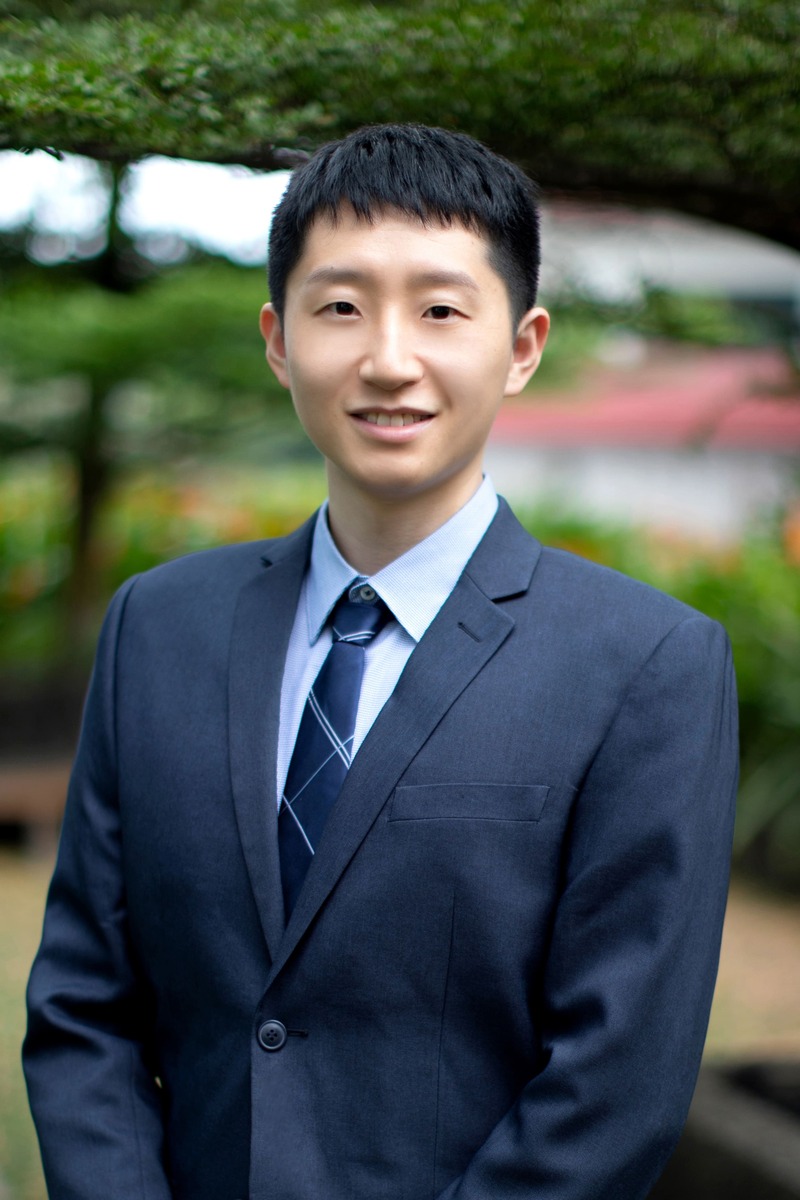
This thesis studies the estimation and inference problems for spatial panel data models when the panels are unbalanced, when the panels contain threshold effects, or when the panels contain time-varying network structures. These three scenarios divide the thesis naturally into three chapters.
The first chapter considers estimation and inferences for fixed effects spatial panel data models based on unbalanced panels that result from randomly missing spatial units. The unbalanced nature of the panel data renders the standard method of estimation inapplicable. In this chapter, we proposed an M-estimation method where the estimating functions are obtained by adjusting the concentrated quasi scores to account for the estimation of fixed effects and/or the presence of unknown spatiotemporal heteroscedasticity. The method allows for general time-varying spatial weight matrices without row-normalization, and is able to give full control of the individual and time specific effects for all the spatial units involved in the data. Consistency and asymptotic normality of the proposed estimators are established. Inference methods are introduced and their consistency is proved. Monte Carlo results show excellent finite sample performance of the proposed methods. An empirical application is presented on commodity tax competition among US states.
The second chapter introduces general estimation and inference methods for threshold spatial panel data models with two-way fixed effects (2FE) in a diminishing-threshold-effects framework. A valid objective function is first obtained by a simple adjustment on the concentrated quasi loglikelihood with 2FE being concentrated out, which leads to a consistent estimation of all common parameters including the threshold parameter. We then show that the estimation of threshold parameter has an asymptotically negligible effect on the asymptotic distribution of the other estimators, and thereby lead to valid inference methods for other common parameters after a bias correction. A likelihood ratio test is proposed for statistical inference on the threshold parameter. We also propose a sup-Wald test for the presence of threshold effects, based on an M-estimation method with the estimating functions being obtained by simply adjusting the concentrated quasi-score functions. Monte Carlo results show that the proposed methods perform well in finite samples. An empirical application is presented on age-of-leader effects on political competitions across Chinese cities.
The third chapter considers the specification and estimation of a three-dimensional (3-D) spatial panel data model with time-varying network structures. The model allows for endogenous and exogenous interaction effects, correlation of unobservables, and most importantly group-specific effects that are allowed to interact with the individual and time specific effects. The time-varying network structures provide information on the identification of various interaction effects but also yield time-varying sociomatrices whose row sums may not be constant, which renders the transformation-based quasi maximum likelihood inapplicable. In this chapter, we propose an adjusted quasi score method where the estimating functions are obtained by adjusting the concentrated quasi scores (with fixed effects being concentrated out) to account for the effects of concentration. The method is able to give full control of general specifications of three-way fixed effects. Consistency and asymptotic normality of the proposed estimators are established. Monte Carlo results show excellent finite sample performance of the proposed methods.
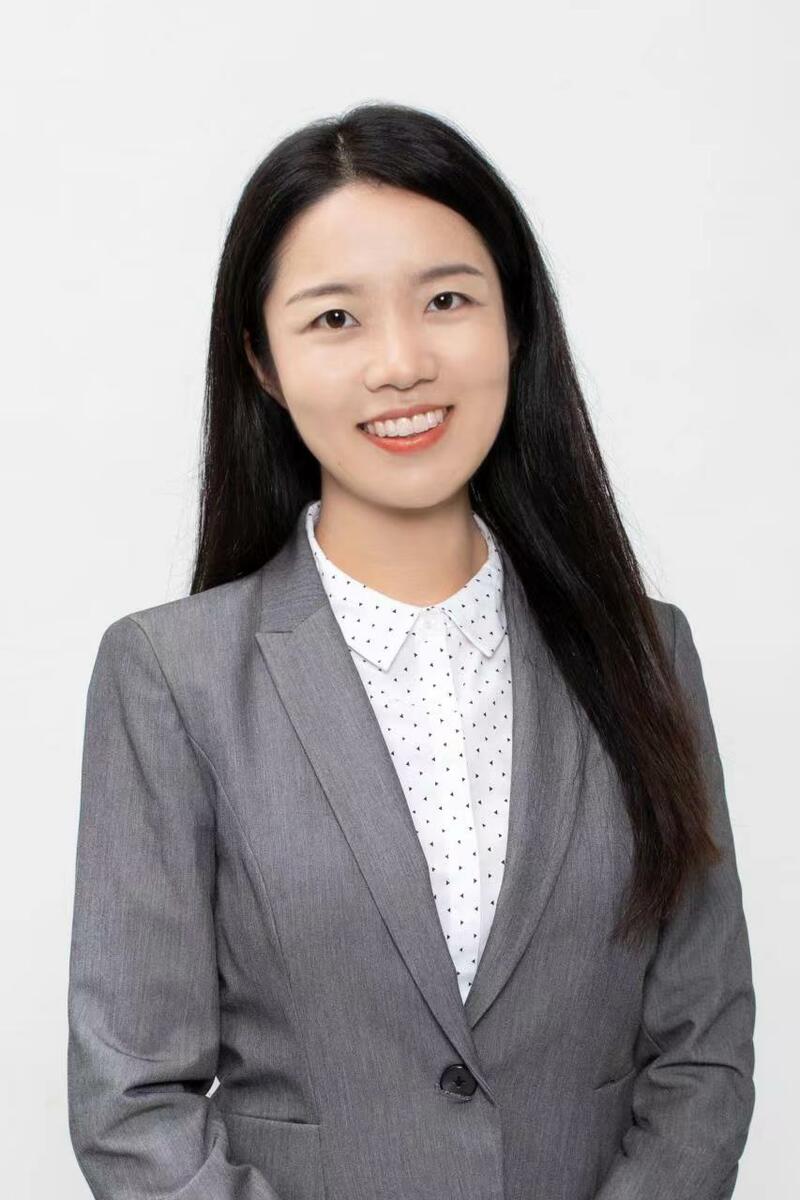
Essays on Financial Materiality of Corporate Social Responsibility and Corporate Strategies
This dissertation investigates how the endorsement of certain social activities by CSR standards impacts stakeholders’ interpretation on firms’ motivation of doing CSR and how managers make decisions on which specific CSR activities they would like to participate in. The first essay examines how the standards release of CSR by Sustainability and Accounting Standards Board (SASB) affects the relationship between material CSR and firm performance outcomes in terms of stock returns (for investors) and sales growth (for customers), through shaping investor and customer perceptions on the motivation underlying a firm’s material CSR activities. I further argue that a sharp increase in material CSR after the SASB standards release, as a strong indicator of a firm’s opportunistic response to the endorsement, is more likely to be penalized by prosocial shareholders and customers. The second essay explores what drives a firm to select different CSR investment strategies, in terms of the financial materiality of CSR. I posit that firms with stronger financial orientation, which is reflected by more analyst coverage and higher institutional ownership, are more likely to engage in financial material CSR investment, but firms with stronger social orientation, which is reflected by higher female board proportion and more liberal CEOs, are more likely to engage in financial immaterial CSR investment. In addition, these effects are moderated by firm’s financial distress. The empirical results support most of arguments.
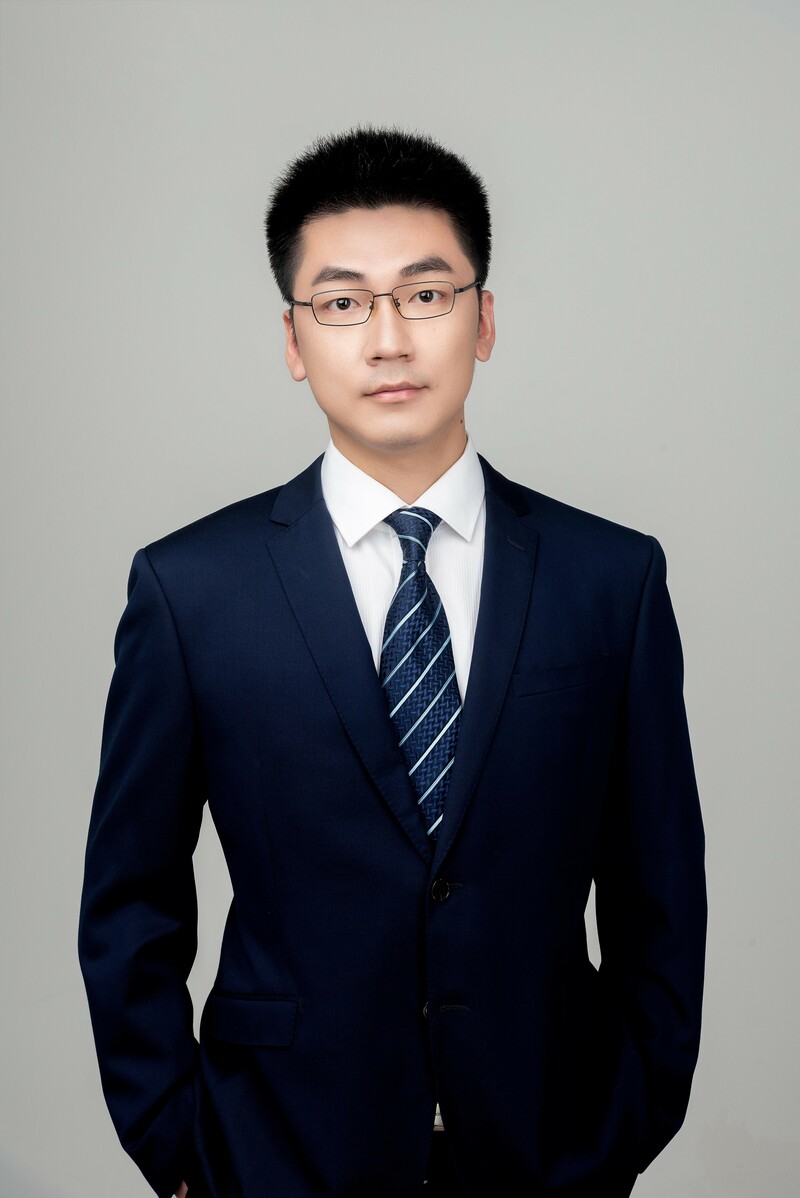
The dissertation consists of three essays on asset pricing by constructing new data set and developing new methodologies. In the first chapter, we conduct empirical studies on the volatility-managed portfolios in the Chinese stock market. Using data from the Chinese stock market, we have found that the main empirical findings in Moreira and Muir (2017) break down. Based on the empirical findings, we exploit a comprehensive set of $99$ equity strategies in the Chinese stock market to analyze the value of managed portfolios. Based on these $99$ equity trading strategies, we find that there exists no systematic gain from scaling the original portfolios using volatility. Our empirical results suggest that one should be careful to use volatility-managed portfolios in practice as the expected performance gains are rather limited.
In the second chapter, we review a Bayesian interpretable machine-learning method proposed by Kozak, Nagel, and Santosh (2020). We show how the method can link two strands of literature, namely the literature on empirical asset pricing and the literature on statistical learning. Based on a recently developed data-cleaning technique, we obtain 123 financial and accounting cross-sectional equity characteristics in the Chinese stock market. When applying the method of Kozak, Nagel, and Santosh (2020) to the Chinese stock market, we find that it is futile to summarize the stochastic discount factor (SDF) in the Chinese stock market as the exposure of several dominant cross-sectional equity characteristics in-sample. A cross-validated out-of-sample analysis further supports this finding.
In the third chapter, we propose several alternative parametric models for spot volatility in high frequency, depending on whether or not jumps, seasonality, and announcement effects are included. Together with these alternative parametric models, nonlinear non-Gaussian state-space models are introduced based on the fixed-k theory of Bollerslev, Li, and Liao (2021). According to Bollerslev, Li, and Liao (2021), the log fixed-k estimator of spot volatility equals the true log spot volatility plus a non-Gaussian random variable. Bayesian methods are introduced to estimate and compare these alternative models and to extract volatility from the estimated models. Simulation studies suggest that the Bayesian methods can in general work well. Empirical studies using high-frequency market indexes and individual stock prices reveal several important results. As an application of extracting volatility, we quantify the strategic value of information.
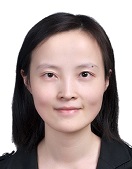
This dissertation seeks to gain insight into the critical roles of consumers and marketers in a retail context using a variety of unique and rich data sources (e.g., tracking data, retail scanner data, ad intel data and publicly available data). The main aim of the two essays is to focus on unique aspects of retail analytics. The first essay examines how consumers conduct haptic search to make purchase decisions using a unique dataset collected by the state-of-the-art sensing technology. This research contributes to the literature by defining key attributes of the shoppers’ speed, consideration set, and shopping path at the shelf space and investigating the effects of consumer haptic search on price paid across food and non-food categories. This paper further provides managerial implications regarding in-store category management and shelf layout. The second essay investigates the spillover effects of recreational cannabis legalization (RCL) on related categories (i.e., alcohol, tobacco, candy, and salty snacks) using secondary data (Nielsen retailer scanner data and ad intel data). This study employs synthetic control method to show that RCL resulted in an increase in per capita dollar sales and per capita unit sales of alcohol, salty snacks, and candy, while this was not observed for tobacco sales. To rule out alternative explanations, this work identifies a "null category" (i.e., batteries) and demonstrates that RCL did not lead to changes in pricing or advertising. The findings are likely to help policymakers in understanding unintended consequences and potential problems associated with RCL such as excessive drinking and junk food consumption resulting in increasing health care expenses.
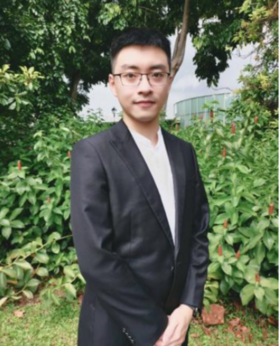
This thesis studies the externalities in the housing market and agglomeration economies. While knowledge-based externalities, or knowledge spillovers are one of the most important micro-foundations of agglomeration economies, the first chapter studies how knowledge spillovers from universities affect local innovation activities. In the second chapter, we propose a high-order spatiotemporal autoregression approach to study the externalities in the housing market. The third chapter studies another important but under explored aspect of the agglomeration economies – the role that marriage market plays in providing incentives to promote urbanization, along with the unique feminization phenomenon during this process.
The first chapter studies the impact of universities on local innovation activity by exploiting a unique university expansion policy in China as a quasi-experiment. In this chapter, we take a geographic approach, empowered by geocoded data on patents and new products at the address level, to identify knowledge spillovers as an important channel. We obtain three main findings. First, university expansion significantly increases universities’ own innovation capacity, which results in a dramatic boom of local industry patents. Second, the impact of university expansion on local innovation activities attenuates sharply within 2 kilometers of the universities. Third, university expansion boosts nearby firms’ new products and the number of nearby industrial patents that cite university patents but not industry patents that cite patents far away from universities.
In the second chapter, we propose a high-order spatiotemporal autoregression approach for analyzing large real estate prices data. Real estate prices arrive sequentially on different housing units over time in a large volume. In this paper, we propose a high-order spatiotemporal autoregressive model with unobserved cluster and time heterogeneity. When the numbers of clusters (C) and time segments (T) are finite and the errors are iid, quasi maximum likelihood method is used for model estimation and inference. In the presence of unknown heteroskedasticity, or C and/or T is large, an adjusted quasi score method is proposed for model estimation and inference. Methods for constructing the space-time connectivity matrices are proposed. Monte Carlo experiments are performed for assessing the finite sample properties of the proposed methods. An empirical application is presented using the housing transaction data in Beijing. We find that the estimation of the spatiotemporal interaction effects are largely affected after controlling for cluster heterogeneity at the community level.
The third chapter studies the relationship between urbanization and feminization, where the marriage market plays an important role in connecting the two. Previous literature studying urbanization and migration has mainly considered incentives arising from cross-city variation in productivity and the subsequent labour market outcomes. In this paper, we study an important but under explored migration incentives arising from the matching outcomes in the marriage market and the gender differences in responding to such incentives. To achieve identification, we exploit the setup of special economic zones (SEZs) as a pull force and China’s accession to the World Trade Organization (WTO) as a push force that exogenously trigger urbanization across locations, which leads to a unique feminization phenomenon during this process. The paper highlights important distributional implications on gender inequality and spatial disparity during the rapid urbanization process.
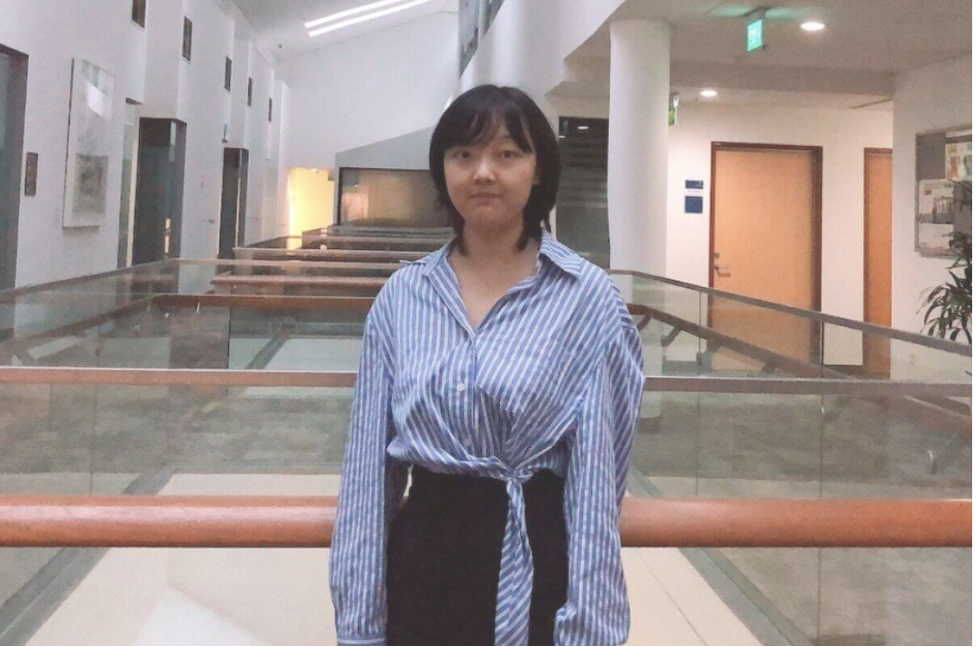
The dissertation consists of three chapters on information diffusion and stock market efficiency and analyst style. The first chapter examines the asset pricing implications of investors’ inattention to non-obvious firm relatedness hidden in earnings calls. This chapter documents that the overlap in attention allocation over various business aspects serves as a time-sensitive proxy for firm relatedness. By employing the unsupervised topic modelling methodology, I characterize the attention allocation of earnings conference call participants (executives, investors and analysts) over topics discussed. I construct a novel cross-firm topic similarity measure that captures difficultto-observe and time-varying firm relatedness compared with existing peer-firm classification systems. I verify that topic peers are fundamentally comoved. However, it is beyond human capacity to process information from a large number of earnings calls in a timely manner. A long-short strategy based on returns of topic peers yields a monthly alpha of approximately 69 basis points. The return predictability mainly stems from topic peers with similar business models, customer management and influential macroeconomic situations. The lead-lag return pattern is more pronounced among focal firms with less firm visibility, higher information complexity and less common information processors. The second chapter investigates whether investors incorporate the value-relevant information from peers with similar geographic locations. Using detailed information on establishments owned by U.S. public firms, we construct a novel measure of geographic linkage between firms. We show that the returns of geography-linked firms have strong predictive power for focal firm returns and fundamentals. A long-short strategy based on this effect yields monthly value-weighted alpha of approximately 60 basis points. This effect is distinct from other cross-firm return predictability and is not easily attributable to risk-based explanations. It is more pronounced for focal firms that receive lower investor attention, are more costly to arbitrage, and during high sentiment periods. Sell-side analysts similarly underreact, as their forecast revisions of geography-linked firms predict their future revisions of focal firms. Further tests suggest that the lead-lag relation we document results from innovation spillover among geographic peers in addition to their common exposure to the local economy. The third chapter examines whether abstract thinking facilitates generating investment insights. Exploiting the questions raised by analysts during earnings conference calls, we construct a (timevarying) Abstract Thinking Index (ATI) to quantify an individual analyst’s propensity to think in an abstract way. Analysts with a higher level of ATI are more likely to ask questions using abstract words and focus on logical reasoning, broader categories of topics and a firm’s future prospects. Abstract thinking analysts issue more accurate and informative earnings forecasts and recommendations. Such effects are stronger when analysts cover firms with more uncertain fundamentals and a poorer information environment. Abstract thinking analysts survive and improve the information environment of firms they cover, and oppositely concrete thinking analysts are less likely to be promoted. Overall, this chapter suggests that abstract thinking is valueenhancing for analysts and facilitates information discovery in financial markets.
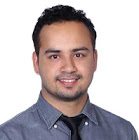
Proliferation of Internet of Things (IoT) sensor systems, primarily driven by cheaper embedded hardware platforms and wide availability of light-weight software platforms, has opened up doors for large-scale data collection opportunities. The availability of massive amount of data has in-turn given way to rapidly growing machine learning models e.g. You Only Look Once (YOLO), Single-Shot-Detectors (SSD) and so on. There has been a growing trend of applying machine learning techniques, e.g., object detection, image classification, face detection etc., on data collected from camera sensors and therefore enabling plethora of vision-sensing applications namely self-driving cars, automatic crowd monitoring, traffic-flow analysis, occupancy detection and so on. While these vision-sensing applications are quite useful, their real-world deployments can be challenging for various reasons namely DNN performance drop on data collected in-the-wild, high energy consumption by vision sensors, privacy concerns raised by the captured audio/video data and so on. This dissertation explores how a combination of IoT sensors and machine-learning models can help resolve some of these challenges. It proposes novel vision-analytics techniques, aimed at improving the large-scale adoption of vision-sensing techniques, with their potential performance improvements demonstrated by using two different vision-sensing systems namely SmrtFridge and CollabCam.
First, this dissertation describes SmrtFridge system, which uses a combination of embedded RGB & Infrared (IR) camera sensors and a machine-learning model for automatic food item identification and residual quantity sensing. SmrtFridge adopts a user interaction-driven sensing approach which is triggered as and when a user is interacting (adding/removing items) with any food item. Using two different processing pipelines, i.e., motion-vector based and IR based, SmrtFridge isolates the food item from the other background objects that might be present in the captured images. The segmented items are then assigned a food label by an image classifier. SmrtFridge shows that using these segmentation techniques can help convert the item identification problem from a complex object-detection problem to a relatively simpler object-classification problem. Also, SmrtFridge proposes a novel IR based residual quantity estimation technique which can quantify the residual content inside food item containers (transparent/opaque) of various shapes, sizes and material types.
Secondly, this dissertation presents CollabCam, a novel and distinct multi-camera collaboration framework for energy efficient visual (RGB) sensing in a large-scale camera deployment. CollabCam exploits the partially overlapping FoVs of cameras to selectively reduce imaging resolution in their mutually common regions. This resolution reduction can enable overall energy savings of a camera sensor by reducing the energy consumption in image capture, optional storage and network transmission. CollabCam proposes novel techniques for (a) autonomous and accurate estimation of overlapping regions between a pair of cameras (b) mixed resolution sensing where selected regions of an image are captured at lower resolution, whereas the remaining regions are captured at default (higher) resolution and (c) collaborative object inference where a modified DNN model, called CollabDNN, utilizes the perspective of other collaborating cameras to enhance performance of object detection on low-resolution images. Application of CollabCam techniques on two publicly available datasets demonstrates the potential high energy savings for a multi-camera system and takes a step towards making energy efficient large-scale vision-sensing systems a reality
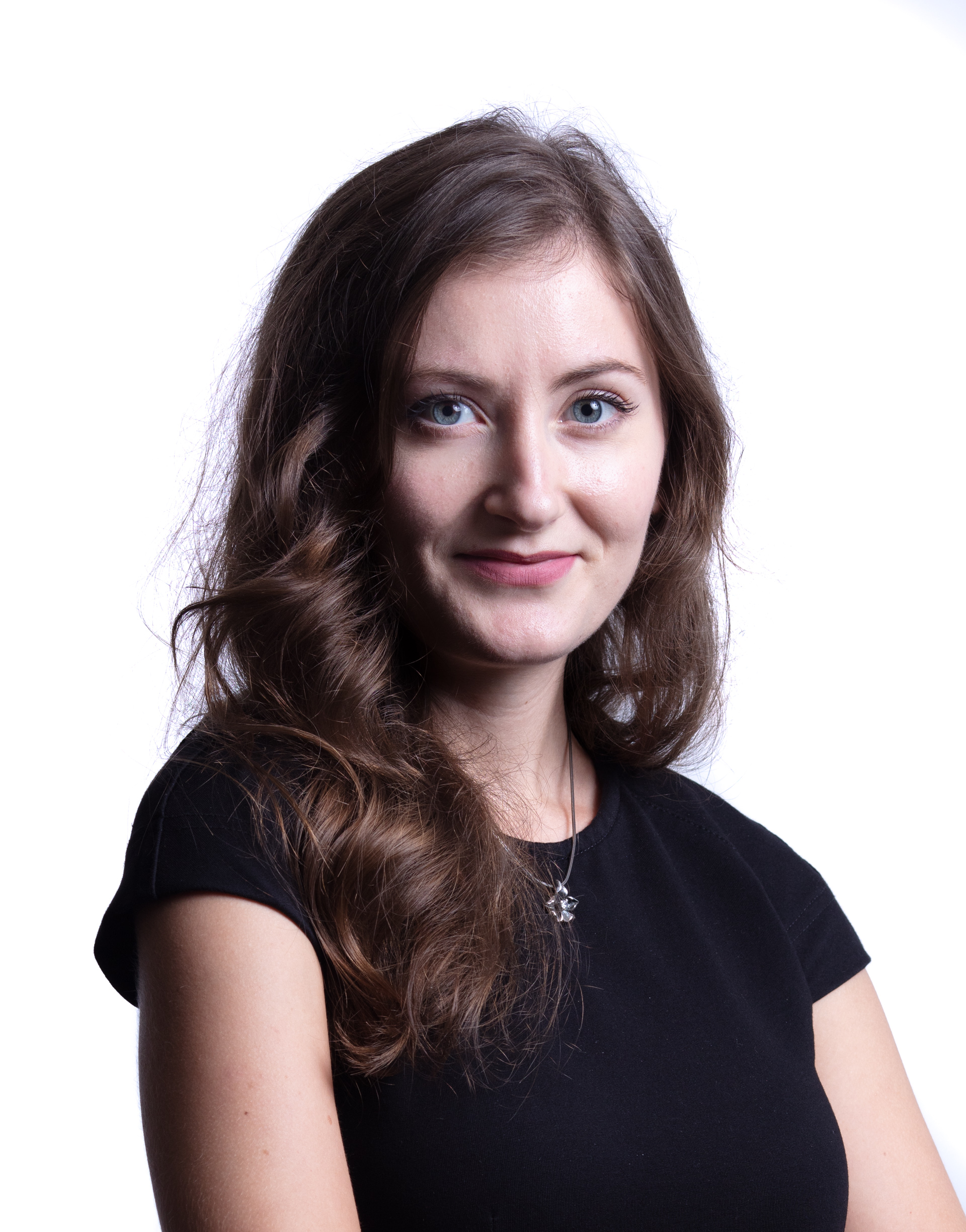
This dissertation consists of three studies in the areas of empirical asset pricing, market microstructure, and behavioural finance. I study the trading behavior and portfolio choices of institutions and retail investors in the equity and derivatives markets. Examining the ways in which different market participants make investment decisions allows us to understand their role in shaping financial market dynamics. This is important in order to know how to structure markets for enhanced market efficiency, and to protect less sophisticated investors through better policies and regulations. Although there is a considerable amount of literature disputing the ability of retail investors and different types of institutions to make informed investment decisions, their trading patterns and the various effects they have on the markets are not fully understood. My dissertation aims to explore this broad issue from several different angles.
Chapter I examines retail investor activity in the extreme portfolios of well-known cross-sectional anomalies. This study is co-authored with Prof. Ekkehart Boehmer. We show that retail investors tend to trade in the opposite direction of anomalies (buying stocks in the short portfolios and selling stocks in the long portfolios), both before and after the anomaly variables become public information. However, we do not find evidence that retail trading is the cause of mispricing and subsequent return predictability. Stocks with high retail participation do not appear to be more mispriced after controlling for confounding factors. Instead of pushing prices away from fundamentals, contrarian retail trades are likely to provide liquidity to arbitrageurs after firm announcements. In addition, we show that retail short sellers exploit anomaly information and help to correct mispricing of overvalued stocks in the short portfolios of value-versus-growth anomalies. Overall, the goal of this study is to show that retail participation in equity markets is not detrimental to market efficiency and in certain settings can even be helpful in correcting anomalies.
Chapter II investigates trading styles and profitability of institutional and retail investors in a leading derivatives market. This study is co-authored with Prof. Jianfeng Hu, Prof. Seongkyu Gilbert Park, and Prof. Doojin Ryu. Using comprehensive account-level transaction data, we provide a detailed description of the options market and the different types of investors. We find that retail investors tend to stick to one trading style. About 70% of retail investors predominantly hold simple positions such as long calls or long puts. Institutional investors are more likely to use multiple strategies with various levels of complexity. We use trading style complexity as an ex-ante measure of trading skills and show that it significantly affects investment performance. Specifically, retail investors using simple strategies lose to the rest of the market. For both retail and institutional investors, volatility trading is the most profitable strategy, although subject to large downside risk. After adjusting for risk, Greek neutral strategies outperform. These style effects are persistent and cannot be explained by systematic risk exposure or known behavioral biases.
Chapter III is about rational regulation and irrational investors. It is co-authored with Prof. Jianfeng Hu. We show that irrational response to regulatory reforms aimed at investor protection can lead to these reforms having the opposite effect and hurting investors. After the August 2011 crisis in the Korean equity market, regulators increase the contract size of equity index options fivefold, hoping to limit retail participation and excessive speculation in the market. Contradicting the purpose of the reform, we find that investors’ propensity to exit the market decreases after the reform. The dollar risk exposure of remaining investors significantly increases after the reform, consistent with investor inattention to the reform. Our estimation shows that it takes six months for risk taking activity to return to the pre-reform level but there is no significant decrease afterward. Heightened risk taking also leads to worse performance in the post-reform period. Although these effects are always stronger on retail investors, institutional investors are not spared either. In addition, we find that investors who are adversely affected by the reform exhibit self-attribution bias which causes them to extrapolate their performance into the future. They tend to outperform their peers before the crisis and their trading activity becomes more responsive to past performance after the crisis. However, limited attention to the market reform exacerbates their losses when their performance reverts to the mean. These results highlight the importance of considering behavioural biases in policy research and setting to avoid unintended consequences.
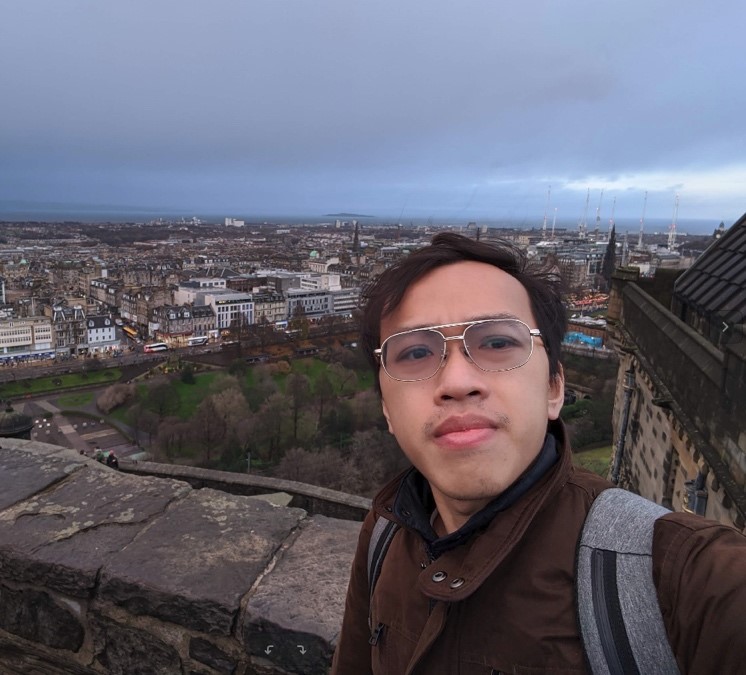
It is desirable to combine machine learning and program analysis so that one can leverage the best of both to increase the performance of software analytics. On one side, machine learning can analyze the source code of thousands of well-written software projects that can uncover patterns that partially characterize software that is reliable, easy to read, and easy to maintain. On the other side, the program analysis can be used to define rigorous and unique rules that are only available in programming languages, which enrich the representation of source code and help the machine learning to capture the patterns better. In this dissertation, we aim to present novel code modeling approaches to learn the source code better and demonstrate the usefulness of such approaches in various software engineering tasks. The methods developed for the aims to utilise the advantages of both deep learning techniques and static code analysis techniques.
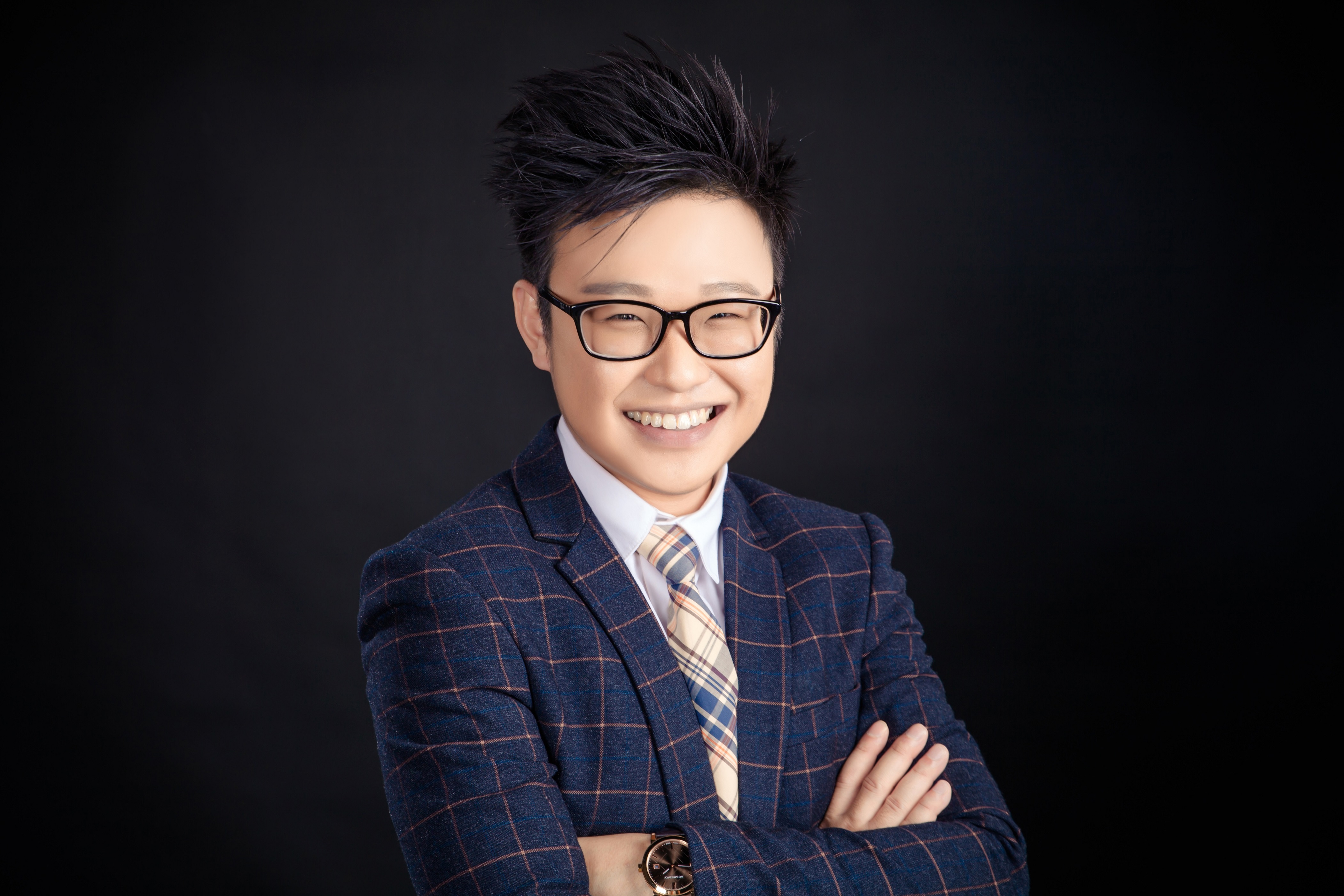
Triads of Interorganizational Conflict: Investigating Asymmetries, Disputes and Tensions
Interorganisational relations are critical resources that are enablers for organisations to achieve competitive advantage. Collaborative ties provide organisations access to new markets, distribution channels, information, and present opportunities to develop or enhance capabilities and competencies. However, interorganisational ties are dynamic and susceptible to relational tensions among collaborative, coordinative, and competitive elements. As such, primarily focusing on collaborative elements between organisations presents an incomplete representation. Social relations involve elements of collaboration and conflict that are not antithetical but dialectical determinants of one another. Despite these conjectures of dialectical tensions, the nature of interorganisational conflict remains elusive. Hence, this dissertation is devoted to: (i) explicating the conceptual underpinnings of interorganisational conflict, (ii) exploring conflict as an experience that develops organisations’ abilities to address interorganisational ties and brokerage and, (iii) examining the asymmetric role of conflict as opportunities for learning and strategic actions.
In chapter two, the dissertation discusses various conceptualisations of interorganisational conflict and highlights conflict as a distinct construct involving relational tensions between interacting organisations. I present an exploratory framework to capture prior perspectives of interorganisational conflict and claim that redefining conceptualisations of interorganisational conflict will present new opportunities for management research. The following chapters in the dissertation highlight that our theoretical understanding of interorganisational relations and beyond can be extended by inculcating the antecedents, processes, and outcomes of interorganisational conflict as part of future research considerations.
Chapter three focuses on firm-level triadic ego-network structures by examining firms’ ability to reside in brokerage positions based on their prior experiences with collaboration and conflict. The chapter develops on the basis that the dualistic experience of collaboration and conflict has implications for a firm’s ability to span structural holes. Empirical results indicate that ambidextrous experiences related to collaborative and conflictual experiences positively and significantly affect firms’ ability to reside in brokerage positions. However, such effects were found to be contingent on the levels of environmental volatility. It was found that environmental volatility reduced the learning effects of prior experiences on brokerage. This suggests that firm learning and the development of capabilities based on prior experiences are contingent on the magnitude of environmental shifts.
Chapter four focuses specifically on the role of conflictual ties on a firm’s ability and strategic positioning to bridge structural holes with the goal of explicating a firm’s role as an initiator or target of conflictual ties. The paper posits that the directionality of conflict impacts a firm’s strategic choices to reside in brokerage positions. The results highlight a significant increase in the likelihood that both targets and initiators of conflict span structural holes. However, when firm performance was considered as a trigger for firm motivation and risk predilection to broker, the effects of directed conflictual ties on brokerage formation were diminished. The results indicate that firm learning is contingent on event-specific determinants as well as firm-related aspirations of motivation and risk partiality.
Empirical chapters three and four are anchored by a 10-year longitudinal sample of contract litigation on breach of contracts supplemented by alliance and joint venture activities of publicly traded firms in the United States of America between 2009 to 2018.
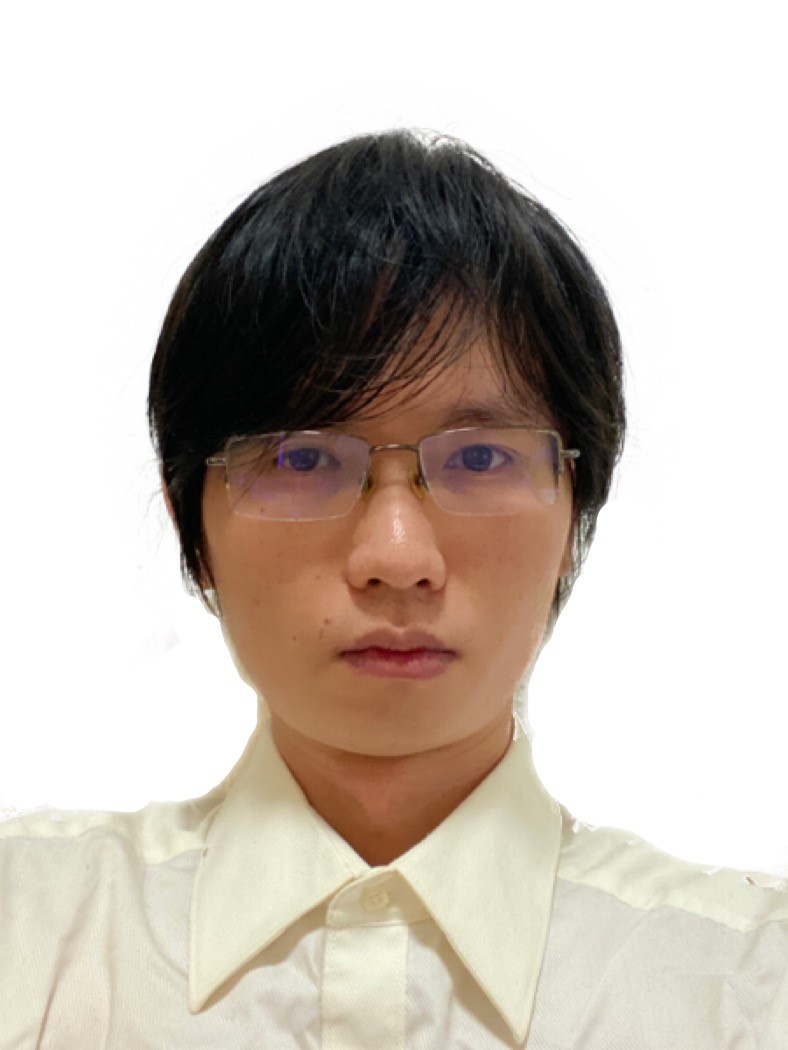
In this dissertation, I have made several contributions to the literature on the multivariate stochastic volatility model. First, I have considered a new multivariate stochastic volatility (MSV) model based on a recently proposed novel parameterisation of the correlation matrix. This modeling design is a generalisation of Fisher's z-transformation to the high-dimensional case. It is fully flexible as the validity of the resulting correlation matrix is guaranteed automatically. It allows me to completely separate the driving factors of volatilities and correlations. To conduct an econometric analysis of the proposed model, I develop a new Bayesian method that relies on the Markov Chain Monte Carlo (MCMC) tool. For the latent variables, the traditional single-move or multi-move sampler is replaced by a novel technique called Particle Gibbs Ancestor Sampling (PGAS), which is built upon the Sequential Monte Carlo (SMC) method. Simulation results indicate that our algorithm performs well when a small number of particles are used. Empirical studies based on the exchange rate returns and equity returns are considered and reveal some interesting empirical results. Second, I further develop a multivariate stochastic volatility model with intra-day realised measures. A simple and consistent estimation technique is developed. The problem of under-identification is discussed. A two-stage approach is introduced to address the problem. A simulation study shows that the proposed method works well in finite samples. The new model is then implemented using two financial datasets. A comparison with some existing models is made. Third, I also incorporate the leverage effect and the heavy-tailed error distribution into the MSV model. A Particle Gibbs Sampling Algorithm is developed for the extended MSV model. Simulation results indicate that our algorithm performs well when a small number of particles are used. Empirical studies of the stock indices are considered. I have found strong evidence of the leverage effect and, more, importantly, heavy-tails in the errors.
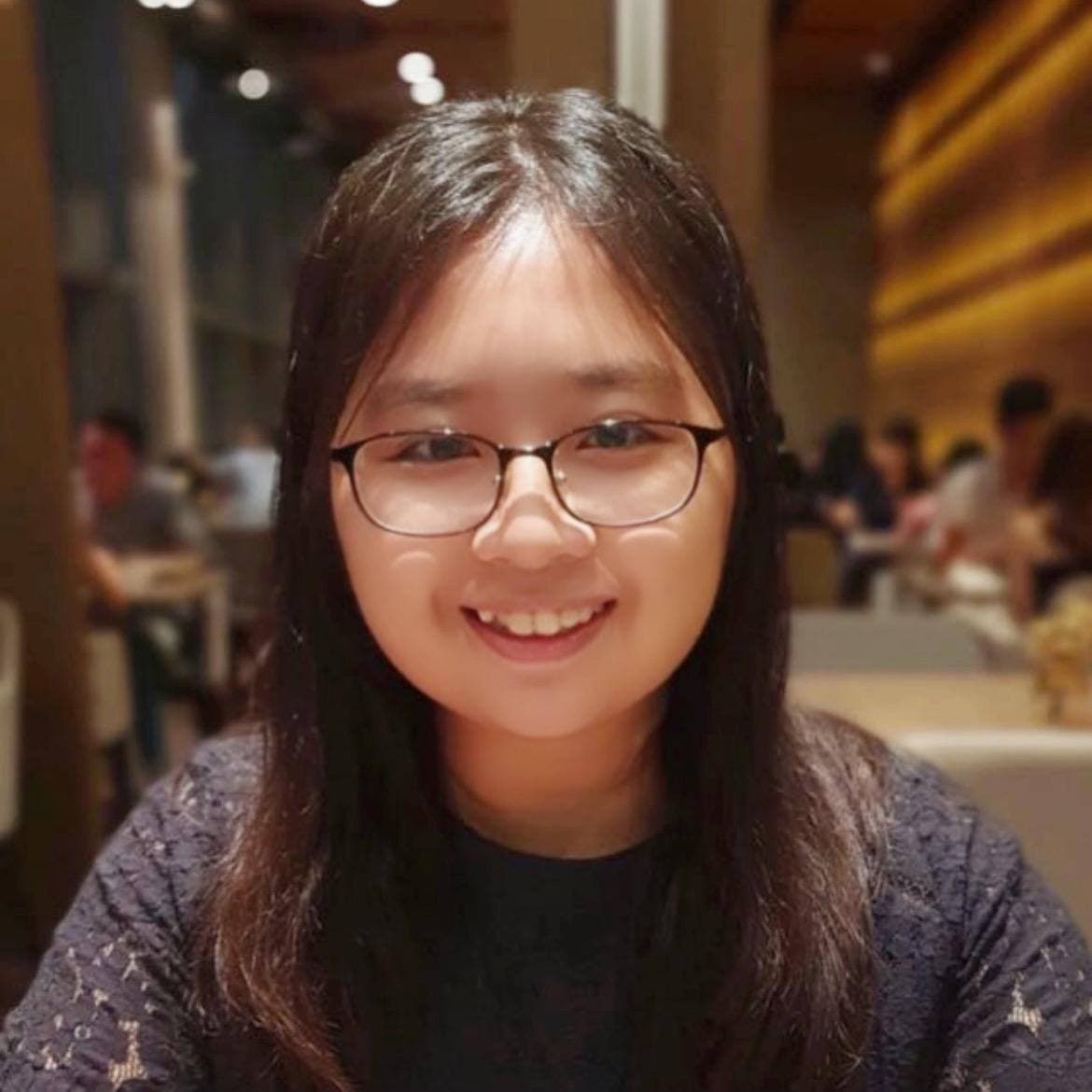
Building on past studies that have found positive influence of minority member on team creativity, this research examined an underexplored yet crucial topic of a unique opinion holder’s happy and anger emotions on team creativity. Using a collective information processing perspective, this study examined whether the expression of anger and happiness would be beneficial for team creativity by spurring team members to respond qualitatively differently to each other’s ideas during the discussion. Additionally, this study examined whether the influence of a unique opinion holder’s emotions on team creativity through information-processing pathways would depend on individual members’ working memory capacities. Three hundred and ninety-six undergraduate students (M = 22.07 years, SD= 1.84) were randomly assigned to work with three to five members, including a confederate who expressed anger, happy or neutral emotions. They were asked to brainstorm ideas that could improve online learning for future semesters in Singapore. As compared with teams with a neutral unique opinion holder, teams with a happy unique opinion holder showed an improvement in their creativity by expanding the active associations within the semantic network of ideas across members (i.e., generative pathway). On the other hand, teams with an angry unique opinion holder elicited improved team creativity by deliberating on expressed ideas (i.e., elaborative pathway). These mediational pathways, however, did not depend on teams’ levels of working memory capacity. Future applications with technological tools and implications of this research for organisations would be discussed.
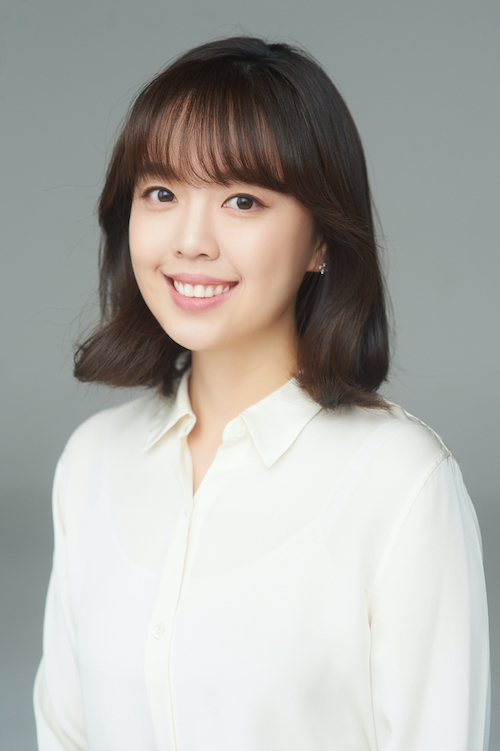
Creativity and innovation are vital for organizational growth and success, driving many organizations to increase pressure for employee creativity. Yet, researchers have neglected investigating how employees respond to creativity pressure at the workplace. This dissertation introduces and develops a new scale for the concept of organizational creativity pressure – the pressure on employees to continually develop novel and useful ideas and solutions. The scale is further validated through extensive assessment of content and construct validity, empirically differentiating the construct from similar others such as performance pressure and support for creativity.
Drawing on the transactional theory of stress (Lazarus & Folkman, 1984) and the need-based theory of work motivation (Green, Finkel, Fitzsimons, & Gino, 2017), I theorize that organizational creativity pressure is appraised more strongly as a challenge stressor than a hindrance stressor, in turn promoting work engagement in employees. Building on the emerging research on gender and creativity, I further theorize that the positive effects of organizational creativity pressure on challenge appraisal and work engagement are stronger for men than for women. Four studies provide evidence consistent with the model. Interestingly, the pattern of interaction is such that men are significantly less motivated and engaged than women at low organizational creativity pressure. At high organizational creativity pressure, there is no significant gender difference in work engagement. Women are also not more likely to see organizational creativity pressure as a hindrance stressor compared to men. This essay has important theoretical contributions to research in creativity, gender, and workplace stress. In a separate chapter, I investigate whether organizational creativity pressure induces feeling of task uncertainty among employees, which in turn leads to negative perception of fairness in the workplace. In sum, this dissertation draws attention to the new construct and the related workplace phenomenon, develops a scale to provide a foundation for empirically rigorous research and investigates both positive and negative effects of organizational creativity pressure in the workplace.
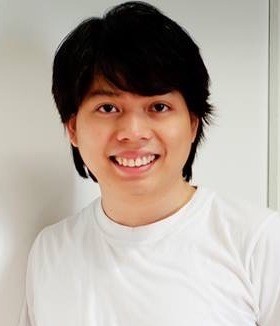
This dissertation focuses on proposing statistical and deep learning models for software engineering corpora to detect bugs in software system. The dissertation aims to solve three main software engineering problems, i.e., bug localization (locating the potential buggy source files in a software project given a bug report or failing test cases), just-in-time defect prediction (identifying the potential defective commits as they are introduced into a version control system), and bug fixing patch identification (identifying commits repairing bugs for their propagation to parallelly maintained versions) to save developers’ time and effort in improving software system quality. Moreover, I also propose a neural network model learning a vector representation of code changes based on their commit messages. The vector representation of code changes contains its semantic intent and can be used to improve the performance of just-in-time defect prediction and bug fixing patch identification. This vector can also be applicable for potentially many other software engineering problems related to code changes, such as tangled change prediction, the recommendation of a code reviewer for a patch, etc.
My dissertation develops one statistical model and three deep learning models for various software engineering tasks. The first one introduces a statistical model which is a novel multi-modal approach for bug localization problem. The multi-modal approach is built by utilizing information from both bug reports and program spectra (or program elements) to effectively localize bugs in programs. Different from other multi-modal approaches for bug localization that treat bug reports (or program elements) as independent, my approach considers similarities between bug reports (or program elements). Hence, similar bugs should have model parameters that are close together. My novel multi-modal approach employs network Lasso regularization to incentivize the model parameters of similar bug reports (or program elements) to be close together.
The second one presents a novel deep learning framework to find likely defective code early; the problem is commonly referred to as Just-In-Time (JIT) defect prediction. While most existing JIT defect prediction approaches involve a manual feature engineering step, where researchers propose a number of features extracted from commits (e.g., the number of deleted and added lines, number of files, information of authors and code reviewers, etc.), I introduce an end-to-end deep learning framework, namely DeepJIT, which automatically extracts features from commit messages and code changes in the commits, and then uses them to identify defects.
The third one introduces a hierarchical deep learning-based approach, namely PatchNet, to find bug fixing patches in the Linux kernel. Bug fixing patch identification and JIT defect prediction are pretty similar as they take as input the same type of data (i.e., commits to version control systems). While DeepJIT simply merges the removed and added code in the code changes together, PatchNet separates the removed and added code and takes into account the hierarchical structure of the removed and added code.
Finally, the last one presents a neural network model, namely CC2Vec, that learns a representation of code changes based on the semantic information in commit messages. Unlike DeepJIT or PatchNet which only solve a specific software engineering task (i.e., just-in-time defect prediction or bug fixing patch identification), the vector representation represents the semantic meaning of the code changes and can be used to solve a number of software engineering problems related to commits (i.e., just-in-time defect prediction, identification of bug fixing patches, and tangled change prediction, etc.).
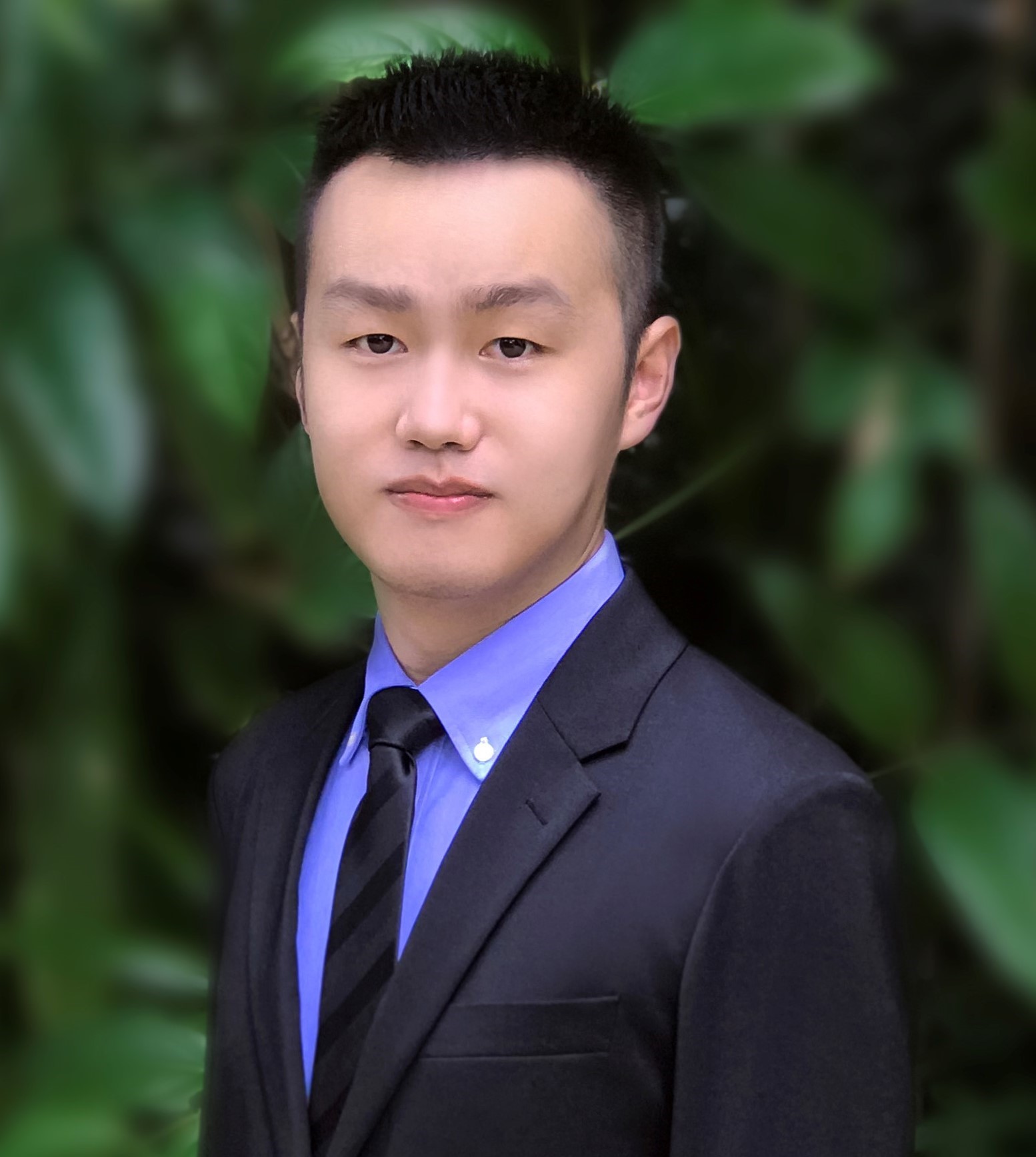
Three Essays on Panel and Factor Models
The dissertation includes three chapters on panel and factor models.
In the first chapter, we introduce a two-way linear random coefficient panel data models with fixed effects and the cross-sectional dependence. We follow the idea of the within-group fixed effects estimator to estimate parameters of interests. We establish the limiting distributions of the estimates and also propose the two-way heterogeneity bias test to check the desirability of the estimation strategy. The specification tests then are constructed to examine the existence of the slope heterogeneity and time-varyingness. We study the asymptotic properties of the specification tests and employ two bootstrap schemes to rectify the downward size distortion of the specification tests. We apply the specification tests to reveal the heterogenous relationship between the unemployment rate and youth labor rate in the working-age population.
In the second chapter, we devise a simple but effective procedure to test bubbles in the idiosyncratic components in the presence of nonstationary or mildly explosive factors in common components in panel factor models. We study the asymptotic properties of our test. We also propose a wild bootstrap procedure to improve the finite sample performance of our test. As an illustrative example, we consider testing the bubbles in the idiosyncratic components of cryptocurrency prices.
In the third chapter, we propose the tests constructed from estimated common factors for detecting bubbles in unobserved common factors when the idiosyncratic components follow a unit-root or local-to-unity process. We study the asymptotic properties of our proposed tests. We show that our proposed tests have non-trivial power to detect those bubbles in unobserved common factors under the alternative of local-to-unity. To implement our proposed tests, we propose to use the dependent wild bootstrap method to simulate the critical values in practice.
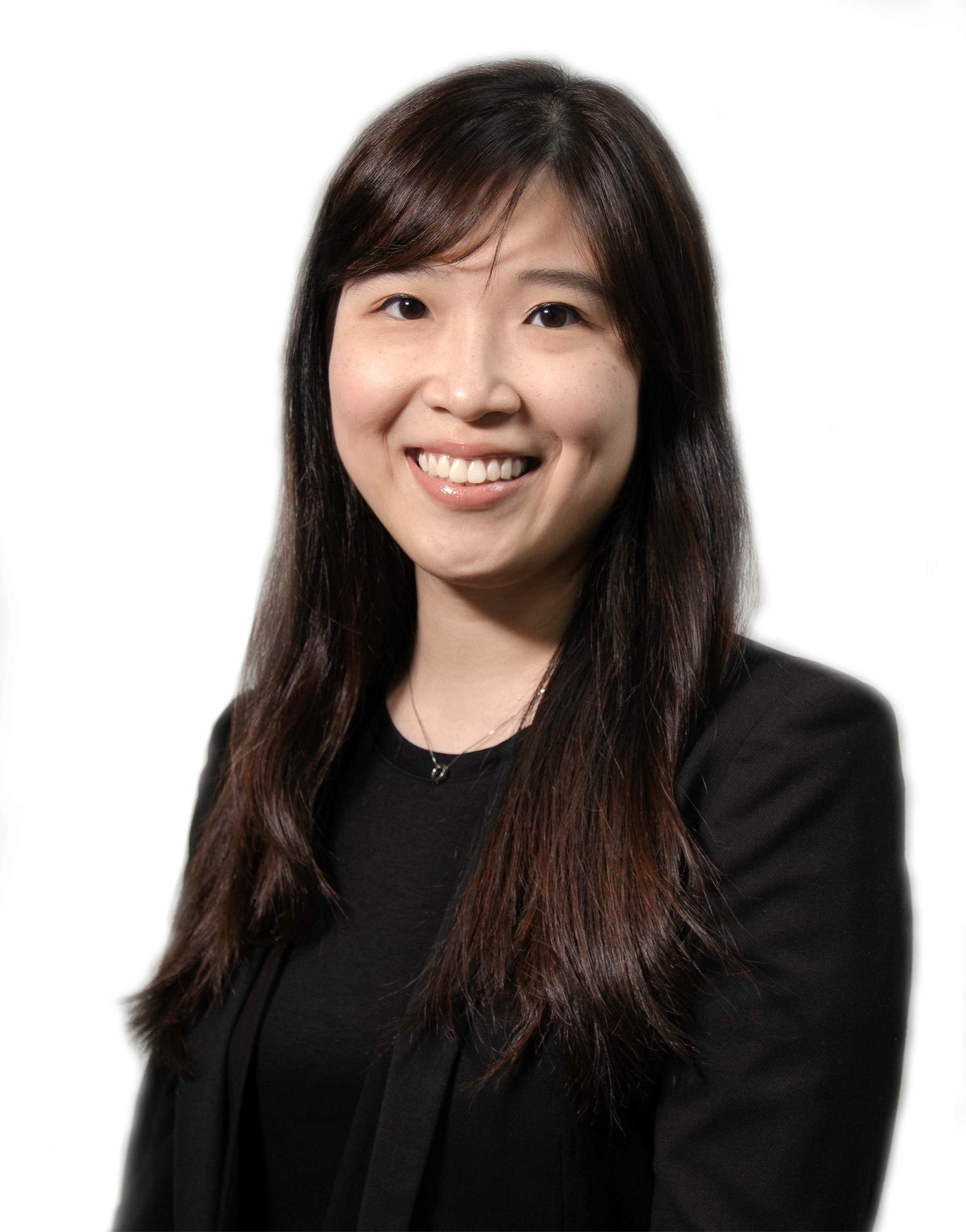
The Many Faces of Class Ceiling: Its Manifestation at Different Career Stages and Ways to Overcome It
Even with comparable education and level of competence, workers with lower socioeconomic status (SES) origins are disadvantaged in terms of earnings and occupational attainment. This class gap, or the “class ceiling,” is as large as the gender gap, but poorly understood. In my dissertation, I designed a series of related projects to explain and potentially mitigate the class ceiling problem. Across three projects, I mainly focused on where the problem starts—labor market and newcomer adjustment in organizations. I find that, beyond discrimination and bias that has been the focus of past work, many challenges stem from workers’ own psychology and behaviors, which can be effectively addressed with a psychological intervention.
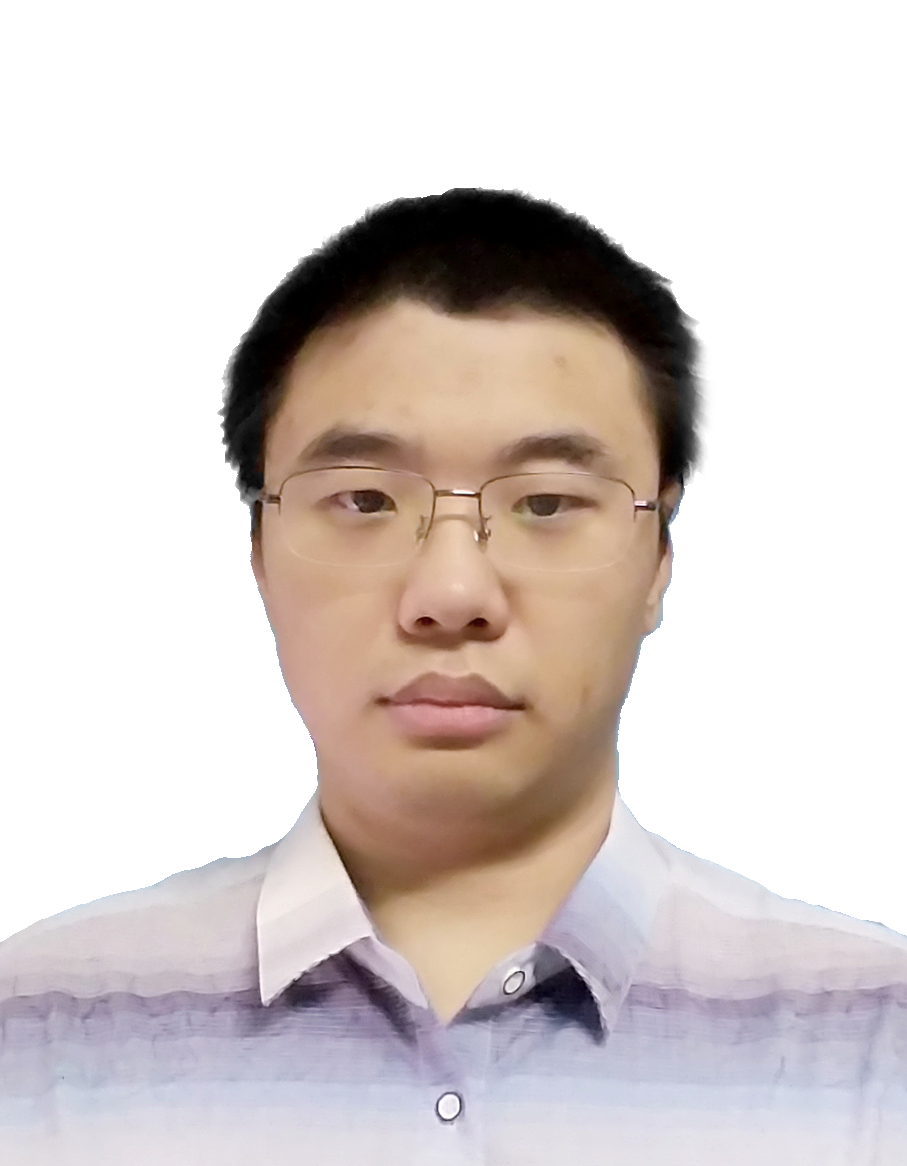
The Android platform is becoming increasingly popular and numerous applications (apps) have been developed by organisations to meet the ever increasing market demand over years. Naturally, security and privacy concerns on Android apps have grabbed considerable attention from both academic and industrial communities. Many approaches have been proposed to detect Android malware in different ways so far, and most of them produce satisfactory performance under the given Android environment settings and labelled samples. However, existing approaches suffer the following robustness problems:
In many Android malware detection approaches, specific API calls are used to build the feature sets, and their feature sets are fixed once the model has been trained. However, such feature sets lack of robustness against the change of available APIs. Since there are always new APIs released with old ones deprecated during the evolvement of Android specifications. If developers switch from old APIs to new ones in app development, older Android malware detection models which are trained before the release of new APIs may not be effective then, because these new APIs are not included in the previously fixed feature sets.
Besides, existing approaches are also lack of robustness towards the label noises. Recent research discovered that sample labels provided by malware detection websites may not be always reliable, and we also figure out that 10% of sample labels provided by VirusTotal change during a period of 2 years in our experiments. This indicated label noises cannot be ignored in the training of Android malware detection models, while existing approaches which directly use the provided labels will suffer from the label noise problem.
Furthermore, even if the sample labels are correct, there may still exist inconsistencies between the sample labels and the generated feature vectors in dynamic-based Android malware detection approaches. Since no triggering modules can perfectly trigger all potential malicious behaviors, and anti-analysis techniques are common in the apps. In this case, the triggered behaviour traces collected from samples labelled as “malware” may not contain “malicious” behaviors, thus feature vectors built from such traces may become noises in the model training.
Towards the above problems, three different works are presented in this dissertation to provide robustness to Android malware detection in different ways:
The first work in this dissertation proposes a slow-aging Android malware detection solution named SDAC. Towards solving the model aging problem, SDAC evolves its feature set effectively by evaluating new APIs’ contributions to malware detection using existing APIs’ contributions. In detail, SDAC evaluates the contributions of APIs using their contexts in the API call sequences. These sequences are extracted from Android apps demonstrating how the APIs are used in real world cases. Based on these sequences, an embedding algorithm named API2Vec is deployed to map APIs into a vector space in which the differences among API vectors are regarded as the semantic distances. Then SDAC clusters all these APIs based on the semantic distances among them to create a feature set in the training phase, and extends the feature set to include all new APIs in the detecting phase. By the feature extension, SDAC can adapt to the changes in Android specifications and thus produces a robust approach against changes in Android OS specifications.
The second work in this dissertation is named Differential Training, which is a general framework designed to reduce the noise level of training data for any machine learning-based Android malware detection approach. We discover that labels of samples provided by Anti-Virus organisations change over time. The changes imply certain labels are erroneous, and thus distort the performance when such labels are used in training Android malware detection models. Differential Training, which functions as a general framework, can detect label noises with different Android malware detection approaches. For the input sample apps, Differential Training firstly generates the noise detection feature vectors from all the intermediate states of two identical deep learning classification models. Then it applies outlier detection algorithms on these noise detection feature vectors, and the outliers detected are regarded as coming from noises. With the label noises being detected and reduced, Differential Training can thus help improve the detection accuracy of Android malware detection approaches.
The third work in the dissertation is a noise-tolerant dynamic-based Android malware detection approach named Dynamic Attention. In dynamic-based Android malware detection approaches, the triggered behaviour traces collected from samples with “malware” labels may not contain “malicious” behaviors due to the imperfect trigger procedure or anti-analysis methods, so they are in fact mislabelled when used in training Android malware detection models. Dynamic Attention is thus designed to solve this mislabelling problem: it identifies the label noises based on the variances of the attention weights associated within the behavior traces derived from malicious apps, and assigns correctly labelled behavior traces with high weights and wrongly-labelled ones with low weights during the model training. By doing so, Dynamic Attention makes the classification model learn less from wrongly-labelled feature vectors and gains resistances against the noises. This approach also enjoys high practicality, since it relies on neither domain knowledge nor manual inspection in the model training.
This dissertation contributes to the robustness of Android malware detection approaches in various ways. In particular, SDAC is robust towards changes in Android specifications, Differential Training provides robustness against label noises for Android malware detection in static analysis, and Dynamic Attention achieves the same goal for Android malware detection in dynamic analysis.
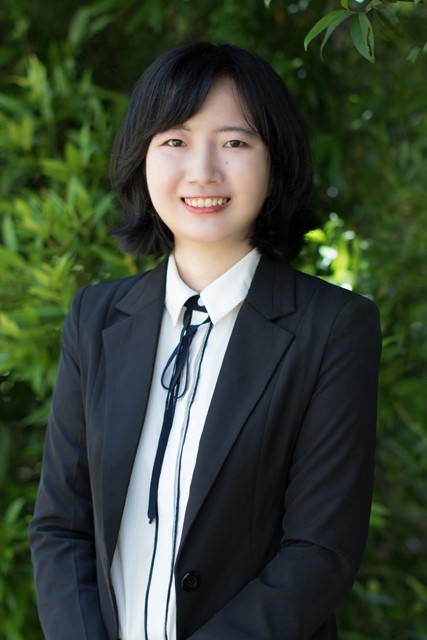
This dissertation consists of three chapters on Preferential Trade Agreements (PTAs) and trade policies. Increasing in numbers rapidly since 1990s, PTAs have extended their traditional focus on tariff reduction to deeper policy integration in areas such as competition policy, intellectual property rights, investment, and movement of capital. The first chapter of the dissertation uses a recently released dataset of PTA contents to quantify impacts of the horizontal depth of trade agreements on bilateral trade flows and national welfare for the period of 1980-2015. The results indicate that agreements that are deeper (covering a wider range of policy areas) contribute to larger trade growth and welfare gain. The second chapter of the dissertation expands the above analysis by using synthetic control matching (SCM) methods to obtain time-varying trade effects of PTAs, and isolates from the estimated total PTA effect the part contributed by different horizontal depths (coverages) of trade agreements. Built on the Anderson and van Wincoop (2003)’s set-up, we decompose and quantify the welfare effects of PTA deep integration for the different horizontal depths (coverages) of trade agreements for the period of 1988-2015, while controlling for the effect of tariff barriers. The third chapter of the dissertation analyses the short-run impact of 2018- 2019 U.S.-China trade war on the Chinese economy, following the micro-to-macro approach of Fajgelbaum et al. (2020) and analyze the impacts of the 2018–2019 U.S.-China trade war on the Chinese economy. We use highly disaggregated trade and tariff data with monthly frequency to identify the demand/supply elasticities of Chinese imports/exports, combined with a general equilibrium model for the Chinese economy (that takes into account input-output linkages, and regional heterogeneity in employment and sector specialization) to quantify the partial and general equilibrium effects of the tariff war. This complements the studies focused on the ex post response of the U.S. economy by Amiti et al. (2019), Flaaen et al. (2020), Fajgelbaum et al. (2020), and Cavallo et al. (2021).
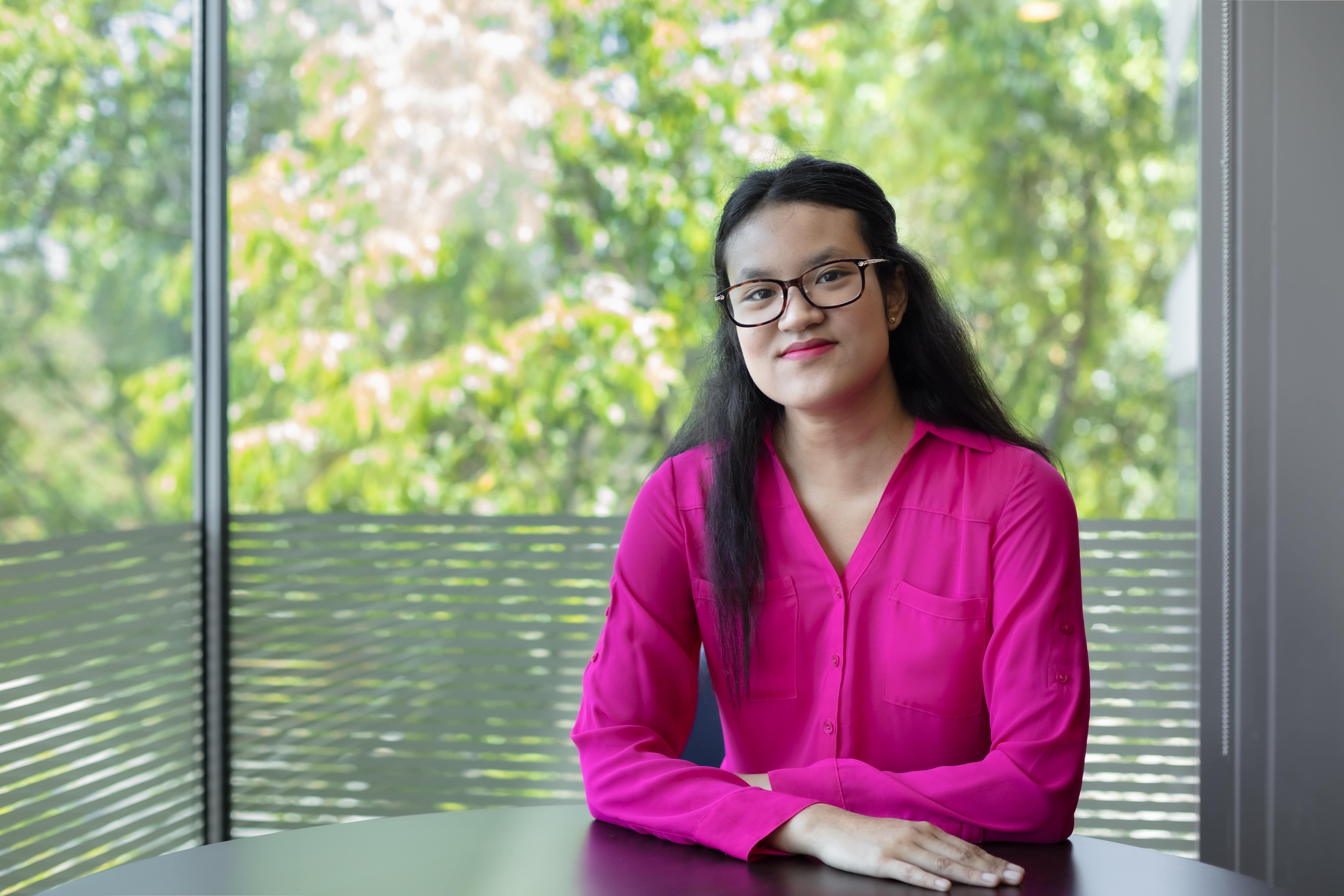
Research on the interpersonal effect of anger expressions on others’ concessionary behaviour has found conflicting results about whether anger expressions increase or decrease concessionary behaviour. The Emotions as Social Information (EASI) model (Van Kleef, 2009, 2014) proposed that these conflicting findings can be resolved by looking at inferential and affective processes. Specifically, anger expressions increase concessionary behaviour via inferential processes but decrease concessionary behaviour via affective processes. However, previous research has mainly focused on dominance-related inferences and reciprocal anger reactions. I propose that the relationship between anger expressions and concessionary behaviour is determined by the type of inferential and affective processes, and not just whether inferential or affective processes are occurring. I explore other inferential processes, such as affiliation-related inferences, and other affective processes, such as complementary fear reactions, together with dominance-related inferences and reciprocal anger reactions, as possible mediators of the relationship between anger expressions and others’ concessionary behaviour. I also propose that the relative influence of these mediators depends on the perceived appropriateness of the anger expression and investigate the proposed model in a transgression setting. I found support for the mediating effect of dominance-related inferences and partial support for the mediating effect of reciprocal anger reactions, but not the other mediators. I also found partial support for the moderating effect of a counterpart’s transgression role on the relationship between anger expressions and perceived appropriateness. I also did not find any moderating effects of perceived appropriateness. Implications of these findings and future research plans for further testing of the EASI model are discussed.
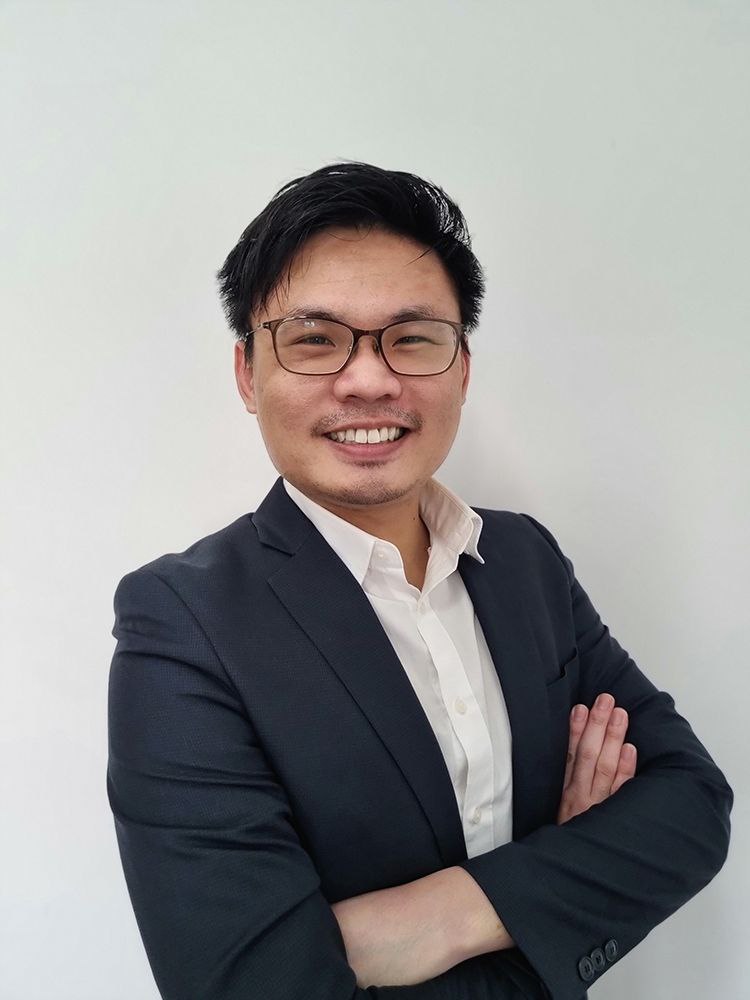
Common across current research in healthcare operations is the conclusion that there exist many inefficiencies in today’s healthcare systems. Governments and healthcare organisations have sought to address these inefficiencies through the introduction of new policies and operational procedures or by relying on incentives to encourage specific behaviour. However, despite these attempts to reduce inefficiencies in the healthcare systems, the problem persists and is further exacerbated by growing medical complexities coupled with a rapidly ageing population. Against this backdrop, this dissertation investigates two issues within healthcare operations: (i) colorectal cancer (CRC) screening adherence, and (ii) blood donor management. A distinguishing feature of this dissertation looks at the incentivization of participants’ behaviours within the two main operations in healthcare operations management.
The first chapter of the dissertation empirically examines the determinants and barriers of CRC screening adherence. Using responses drawn from a nationwide survey, the data highlights that CRC screening adherence levels continue to remain low despite the government’s implementation of nationwide screening programs. To study the reasons behind the low adherence rate, I conduct a stepwise logistic regression model and identify several key predictors of the screening adherence. I found that age and individual perceived risk of developing CRC have significant quadratic trends towards screening participation. The results further show that participant's proficiency in probability literacy has an impact on perceiving an individual's risk of developing CRC towards screening adherence. Linear predictors consisting of CRC knowledge and factor of trust in government are also significant predictors towards screening participation. Motivated by the significant quadratic trend of age, I further investigate the nonmonotonic relationship between age and the adherence rate and provide policymakers with insights on possible interventions to CRC screening policies via a mediation model. I found that policy mediation factors in the form of financial means - CPF account balance and ownership of private insurance were statistically significant mediators that drives the nonmonotonic relationship.
The second chapter studies the strategic management of blood inventory through donor incentivization policies where under-incentivization may lead to shortage of critical blood supply while over-incentivization potentially causes excessive wastage. Incorporating key features of the blood donation such as perishable inventory, observation queue and stochastic demand and supply, I propose an optimization model to solve the donor incentivization decisions in the blood donor management problem by modelling both the blood inventory and donor flow process. Building on the techniques of the Pipeline Queues framework, the optimization model can be reformulated into a convex problem and be efficiently solved. Numerical experiments were further conducted to study how the structure of the optimal policies can change with respect to donors’ responsiveness, inventory levels, changes in demand for blood, new donor recruitment rate and distribution of donors in the observation window. Based on the results, the study also puts forward important practical implications relevant in supply chains with social impact.
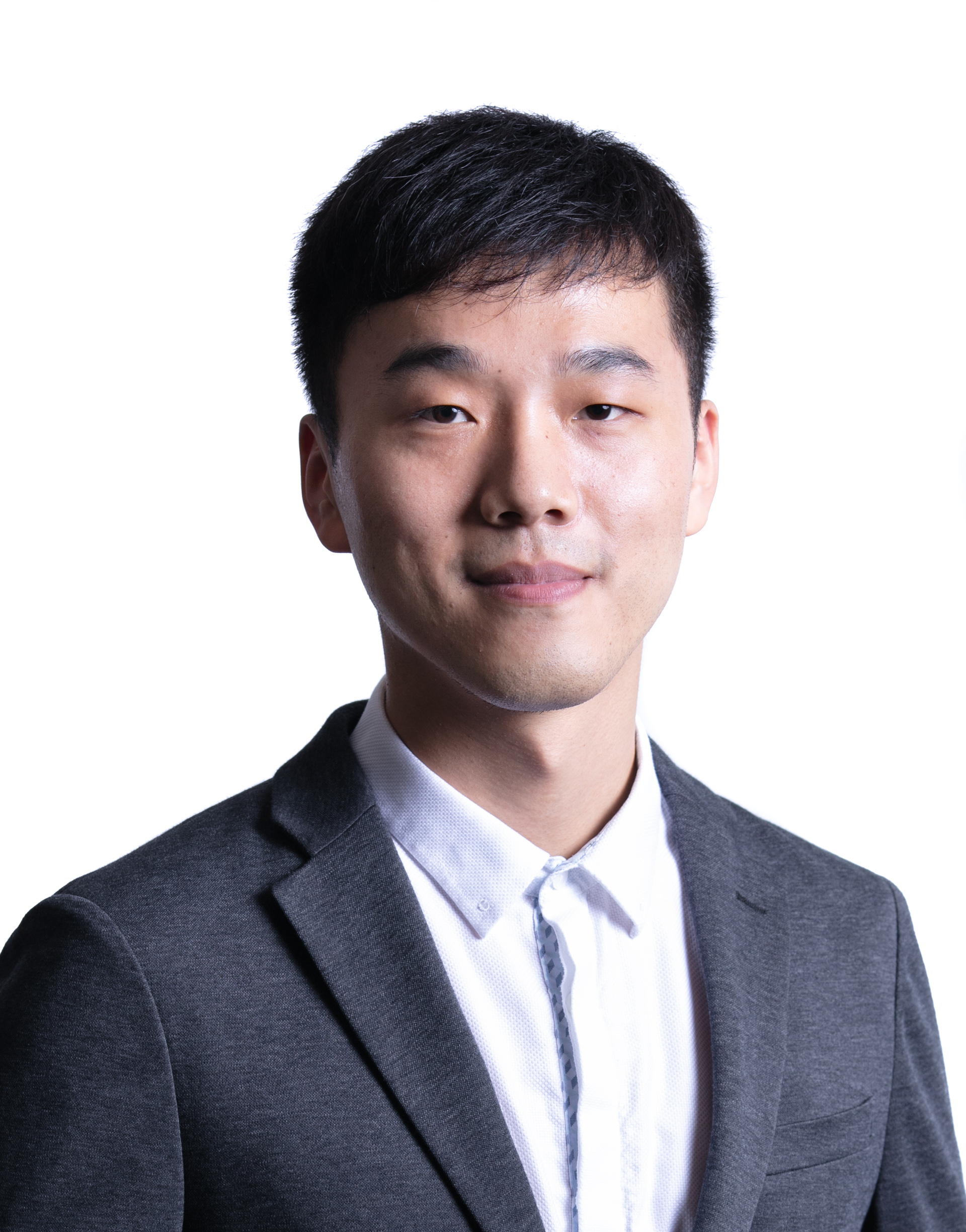
Resource flexibility hedges against uncertainty in service and production systems. However, flexibility also brings complexity and difficulty in allocating resources. The thesis mainly studies managing flexible resources in two scenarios. The first scenario is a type of coordination of workers in a production or assembly line – bucket brigade. Specifically, the study shows how to manage a stochastic bucket brigade with discrete work stations. The second scenario is a service system with flexible service resources. The study proposes a distributive decision rule for the allocation of resources under both supply and demand uncertainty.
Chapter 2 studies a J-station, I-worker bucket brigade with preemptible work content. The time duration for each worker to serve a job at a station is exponentially distributed with a rate that depends on the station’s work content and the worker’s work speed. We analytically derive the throughput and the coefficient of variation (CV) of the inter-completion time. We study the system under three cases. (i) If the work speeds depend only on the workers, the throughput gap between the stochastic and the deterministic systems can be up to 47% when the number of stations is small. (ii) If the work speeds depend on the workers and the stations such that the workers may not dominate each other at every station, the asymptotic throughput can be expressed as a function of two factors. (iii) The work speeds depend on the workers, the stations, and the jobs. There is a trade-off between the intensification of the learning experience and the diversification of the skills.
Chapter 3 further studies a J-station, I-worker bucket brigade with non-preemptible work content. If the work content is non-preemptible, the work on each station can not be preempted and has to be processed by the same worker. We properly denote the waiting workers, re-analyze the state of the system, and the transition probability matrix of the reset vectors. Finally, we derive the average throughput. In the numerical experiments, we first verify the theoretical results by simulations. Then we compare the throughput difference between a non-preemptible line and a preemptible line. If the workers are sequenced slowest-to-fastest, the preemptible line dominates the non-preemptible line. However, if the workers are sequenced fastest-to-slowest, the non-preemptible line can possibly dominate the preemptible line. As such, the management needs to consider the actual setting to enhance the performance.
Chapter 4 studies a resource allocation problem, where the planner needs to decide simultaneously on both the supply and the allocation policy to fulfill the uncertain demand over a multi-period horizon. We introduce a distributive decision rule, which decides on the proportion of jobs awaiting dispatch to each of the possible resource supply pools. Our model has a convex reformulation that can be solved efficiently. Through simulations, we illustrate that the optimal solution evolves with changes in service distribution, initial conditions, temporal fluctuations in demand, and resource availability. At last, we benchmark our model against the static rule and a fluid model. In doing so, we justify the adaptivity of the proposed distributive decision rule and show the robustness of our model to different settings.
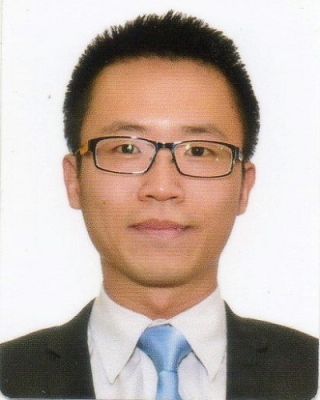
Comparison sites are widely used by consumers. Theory assumes that consumers visit these sites to discover new alternatives, raising questions about the role of the initial consideration set (alternatives considered at the start of search) when comparison sites are available. Will consumers ignore their initial consideration set and directly explore new alternatives? Will consumers with large initial consideration sets avoid comparison sites? Utilizing search and incomplete knowledge theories, the authors intuit that consumers first search their initial consideration set, and visit a comparison site to reduce the search costs of doing so. If a suitable alternative is absent, consumers subsequently visit a comparison site to discover new alternatives. The authors test their expectations on unique data capturing consumers’ initial consideration sets and online search and find strong support. Specifically, consumers search a greater proportion of their initial consideration set at the start of search and are more likely to visit a comparison site when their initial consideration set is large. Additionally, consumers are more likely to visit a comparison site when they expect to find a better deal, particularly at the end of search. Finally, only consumers expecting to find a better deal are more likely to explore alternatives not in their initial consideration set.
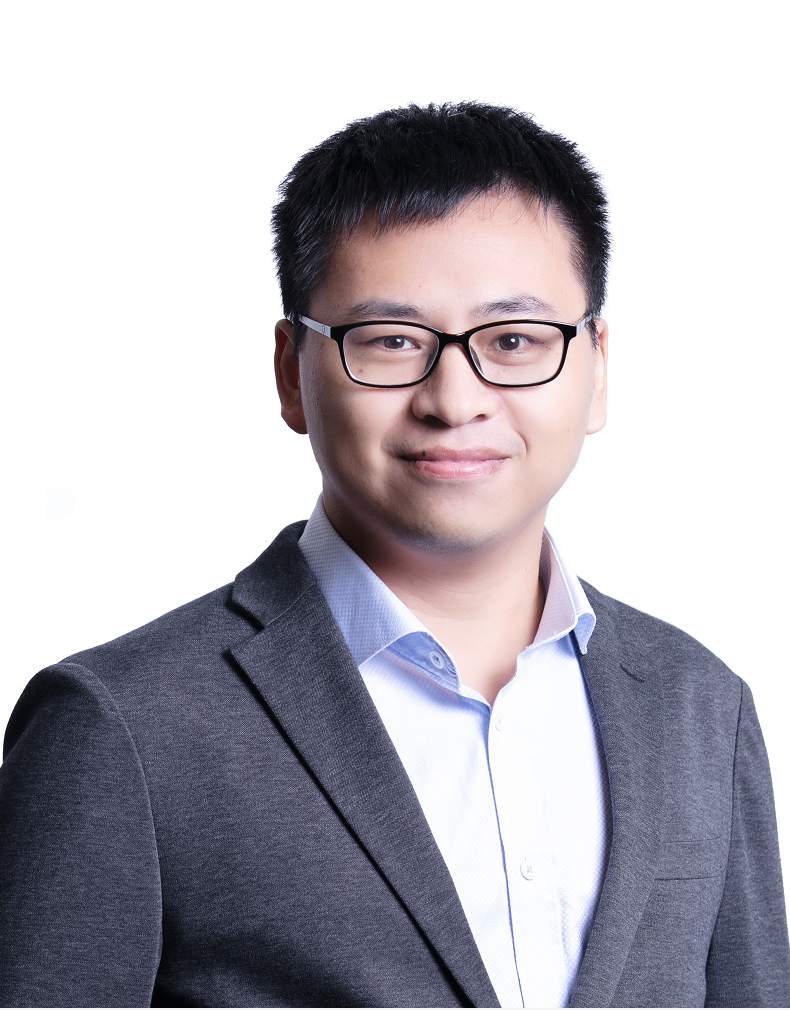
Essays on Management of Scarce Resources
Efficient management of scarce resources is critical to the improvement of economic, social and/or environmental performances. In this dissertation, I focus on the management of two scarce resources: i) healthcare resources and ii) water, and investigate two important problems: i) the estimation of patients’ health transition to support healthcare resources control under the context of sequential medical treatments, and ii) the urban water system control with a specific focus on the wastewater recycling capacity investment in the presence of climate change and urban water scarcity. The first chapter studies how to estimate patients’ health transition considering the effects of treatment-effect-based policies. Treatment-effect-based decision policies are increasingly used in healthcare problems, which leverage predictive information on patient health transitions and treatment outcomes for specific medical treatment decisions. However, treatment-effect-based policies will significantly censor patients’ observed health transitions and distort the estimation of transition probability matrices (TPMs). I propose a structural model to recover the underlying true TPMs from censored transition observations to address this issue. I show that the estimated TPMs from the structural model are consistent, asymptotically normally distributed and maximize the log-likelihood function on observed censored data. I compare the proposed model with other estimation methods through numerical experiments and demonstrate its advantages in various performance metrics, e.g., deviations from the ground truth TPMs. I also implement the proposed model to estimate patient health transitions using real censored data in ICUs extubation problems. Formulating the extubation problem as a classical optimal stopping Markov Decision Process model, I show that the proposed model, with more accurate estimated TPMs considering censored data, can reduce the length of stay of patients in ICU compared to other benchmark transition estimation methods. In the second chapter, considering multiple urban water resources (e.g., freshwater from reservoirs, recycled water, and desalinated water/imported freshwater) and multiple streams of urban water demand (e.g., household and non-household demands), I examine the economic and sustainable implications of wastewater recycling capacity investment under rainfall and recycling cost uncertainties. To this end, I formulate the problem as a two-stage stochastic minimization model and characterize the optimal wastewater recycling capacity. I find that the optimal recycling capacity first decreases and then increases in the freshwater capacity, suggesting that they are substitutes when the freshwater capacity is relatively small and complements otherwise. I also perform sensitivity analysis on how the uncertainties (rainfall and recycling cost variabilities and their correlation) affect the optimal recycling capacity and the optimal expected cost and find that the water utility always benefits from a higher correlation coefficient but a lower rainfall variability. In this chapter, I also discuss urban water sustainability using the measures such as urban water vulnerability and characterize the specific conditions under which urban water may become more vulnerable. The third chapter calibrates the economic model presented in the second chapter based on the publicly available data from the urban water supply practice in Adelaide, the capital city of South Australia. To complement the analytical results, I conduct comprehensive numerical analysis in this chapter to investigate the effects of uncertainties on the optimal expected cost and optimal recycling capacity. Moreover, I study the value of wastewater recycling and how rainfall and recycling cost variabilities, correlation and demand expansion affect it. For example, the results show that the value of wastewater recycling increases in the correlation coefficient and decreases in the rainfall variability. Based on the calibrated baseline scenario, I find that the expansion of both the household and non-household demands increase the value of recycling; moreover, the expansion of non-household demand tends to have a larger impact when the deviations from the baseline scenario become relatively large. I further study the leakage reduction, water vulnerability and overflow risk. The insights from the numerical analysis in this chapter complement the analytical results presented in Chapter 2. I put forward important practical implications relevant to both urban water utilities and water policymakers based on the findings.
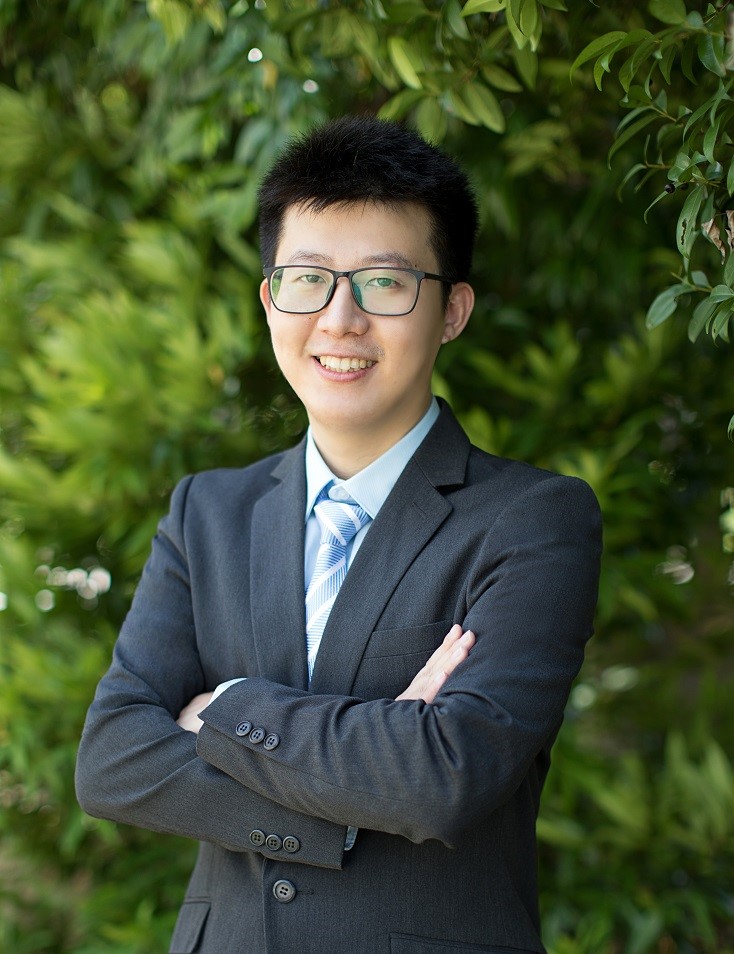
Two Essays on Innovation and Growth in China
This dissertation studies China’s economic growth from a perspective of industry dynamics. In chapter 1, I introduce the background and policies relating to China’s economic growth after 1978. In chapter 2, I find that the elasticity of the average R&D expenditure of firms on competition is -0.29 in weak-IPR (intellectual property right) provinces, and -0.06 in strict-IPR provinces. Next, I use the Schumpeterian growth model to explain this finding: When the market becomes more competitive, a firm prefers imitation to innovation to a larger extent, as a means of getting new technology. Due to enforcement of IPR laws, the imitation replaces innovation more slowly in strict-IPR provinces, compared to weakIPR provinces. In chapter 3, I estimate the TFPs of exporting and importing varieties for 6827 firms from 2002 to 2007 in the garment industry. I present three main channels of the growth of the aggregate TFPR(revenue) of continuous exporters: technology upgrade, reallocation of resources within continuous-exporting products, and switch of products. These three channels explain 27.2%,15.3%, and 9.46% of the aggregate TFPR growth, respectively. From the import side, the adjustment of import counts by firms explains 0.1% of the aggregate TFPR growth.
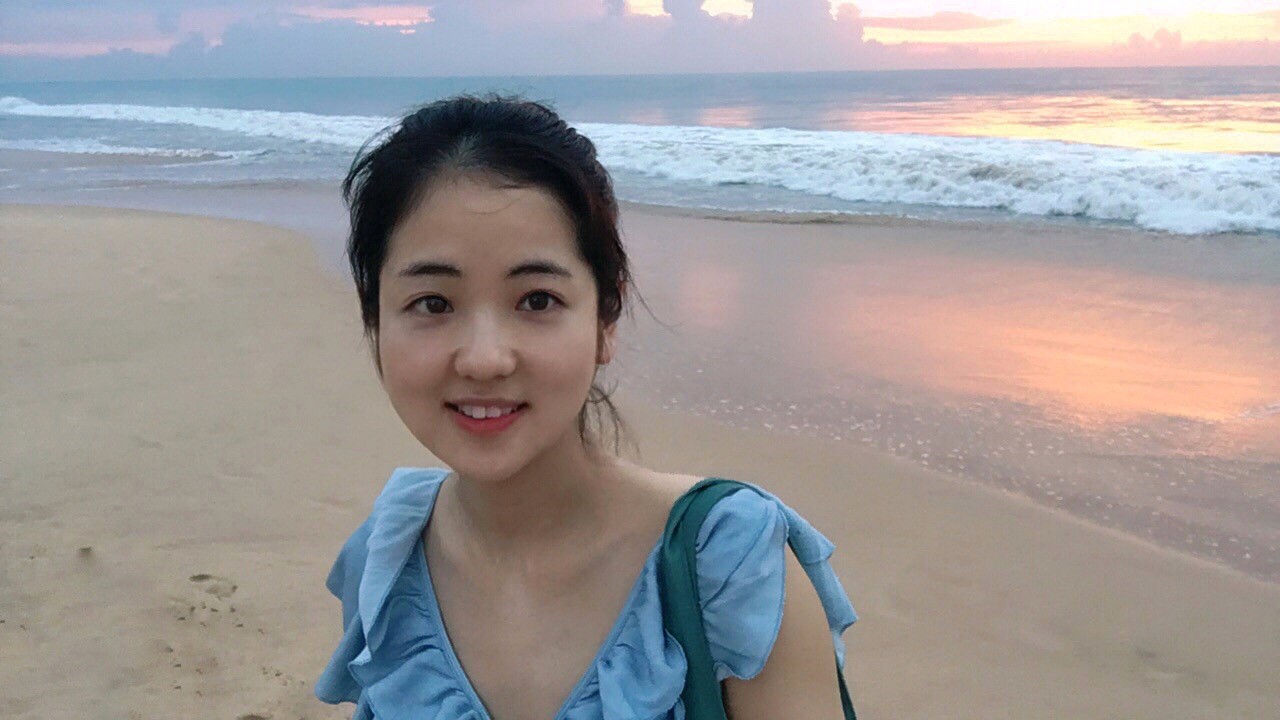
Three Essays on International Trade
In the first chapter, we develop an estimation procedure to identify the partial (direct) effects of the GATT/WTO membership on variable and fixed trade costs, respectively. This procedure extends the techniques of Anderson and Van Wincoop (2003) on the structural relationship of multilateral resistance terms and of Helpman, Melitz and Rubinstein (2008) on the structural modeling of trade incidence. We then develop a general equilibrium framework (that allows for the presence of zero trade) to simulate the impact of variable, fixed, and total trade cost changes on the firm-level trade structure (including the bilateral export productivity cutoff, the weighted/unweighted extensive margin of export, the intensive margin, and the mass of active firms), the bilateral trade flow, and the aggregate welfare due to the GATT/WTO system (given the trade cost effects estimated in the first stage) for the period 1978–2015.
Information asymmetry can create substantial frictions when importing firms find it difficult to acquire information about foreign products. In the second chapter, I use detailed China Customs Data to show that firms tend to import from countries with which they already have an importing relationship. Motivated by this fact, I develop a dynamic model describing firms’ decisions on their choice of sourcing country. This model incorporates both communication cost and satisfaction uncertainty, which are lower with familiar countries than with unfamiliar ones. Using this model, I estimate the benefits of importing from familiar countries measured by the probability improvement of receiving satisfactory products. I find that this probability can be improved by a maximum of 89.0 percent when importing from familiar countries instead of from unfamiliar ones. These results also support the prediction that the effective unit cost of intermediates is lower when importing from familiar countries.
The third chapter presents a heterogeneous firm model à la Melitz (2003) in which firms suffer from both the agency problem internally and financial constraints externally. We show that conditional on the same raw productivity draw, managers of potential exporting firms around the export cutoff in financially underdeveloped
countries exert more effort than their counterparts in financially developed countries, as to induce their owners to export. This finding has very positive policy implications, as firms in financially underdeveloped countries can compete with their peers in financially developed countries by exerting more managerial effort. We find clear empirical evidence for this theoretical prediction using the World Management Survey data for more than 7,000 firms in 20 countries during 2002-2012.
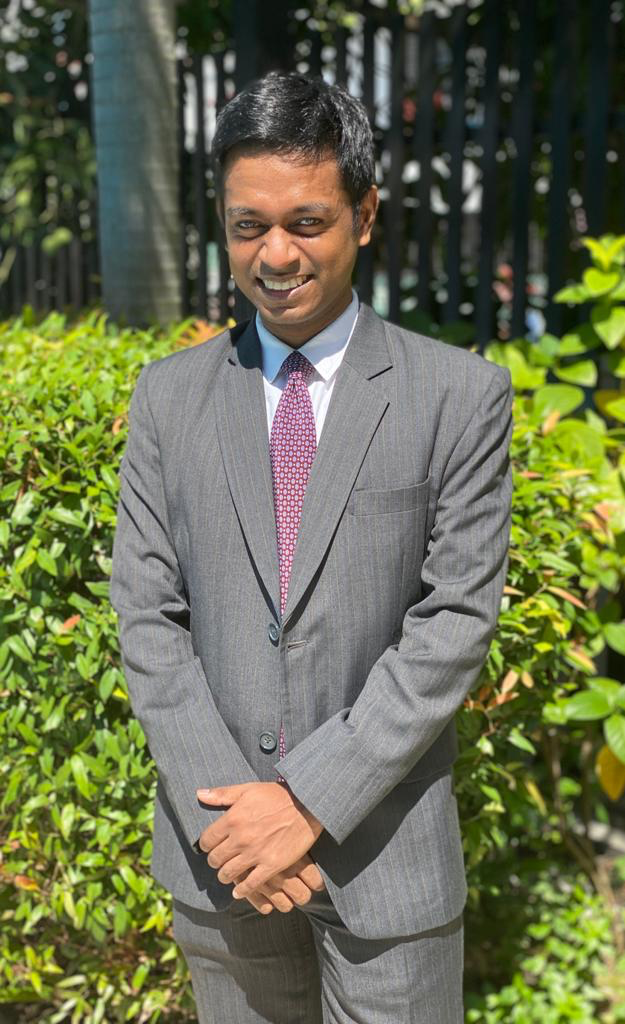
The first chapter is a randomized controlled trial study that uses loss framing and information nudges to increase secondary school attendance in Bangladesh. Conditional cash transfers (CCTs) have become one of the most common policy interventions to increase school attendance, but the cost-effectiveness of such interventions has not attracted the attention it deserves. Hence, in addition to a standard CCT implementation, our rich unique dataset on daily attendance allows us to experimentally study two potential ways to improve the cost-effectiveness of school attendance interventions: (i) SMS information nudges and (ii) loss framing in CCTs. The former provides school attendance information to parents and the latter exploits the endowment effect. Consistent with the existing literature, CCT intervention significantly increases school attendance. Though the difference between gain and loss framing is not statistically significant, the point estimate of the Loss treatment is consistently higher than that of the Gain treatment. The SMS treatment has a modest impact on school attendance but the overall cost of treatment is low. We also find diminishing marginal impact of cash transfer amount on attendance, indicating that the intensive margin matters. Thus, both loss framing and SMS nudges can be considered as alternative cost-effective approaches to promote attendance in schools in developing and less developed economies. In the second chapter, I study the causal impact of alcohol consumption on incidence of intimate partner violence in the Indian context. A study by the World Health Organization shows that about 35% of women in the world have been victims of physical or sexual intimate partner violence in their lifetime. Using an overidentified model where I exploit the spatial variation in alcohol ban and minimum legal drinking age across states in India to instrument for the husband's alcohol consumption, I find that alcohol consumption by the husband increases incidence of less severe physical violence by 55 percentage points and severe physical violence for women by 23.6 percentage points, and also has negative consequences on women empowerment in general. I further show that the results are not driven by worse gender attitudes in states where alcohol is allowed. A heterogeneity analysis reveals that there is a vicious cycle of intimate partner violence whereby individuals who are the most vulnerable in terms of having previous exposure to domestic abuse or residing in poorly constructed houses are often the victims. The third chapter explores the causal impact of the mid day meal program on parental investment in education for primary school going children in India. Using the first round of the Indian Human Development Survey (IHDS) and exploiting the staggered implementation of the mid day meal program across different states in India, we find that the amount spent on school fees reduces significantly by 16 percent for children who are eligible to receive the mid day meal. The significant decrease in school fees can, in part, be attributed to transfer of children from private to government schools. We further find that such transfers do not lead to any improvement in learning or health outcomes. However, there is no evidence of gender discrimination in school expenditures that might adversely affect the girl child. The fourth chapter outlines Singapore’s major sustainability challenges and its policy response in the areas of land use, transportation, waste management, water, and energy. We review the current and past Concept Plans from the perspective of sustainable land use and provide an overview of transportation policy in Singapore. We also examine Singapore’s policies to manage increasing wastes and review the four tap water management plan. Finally, we look at various initiatives by the government for sustainable use of energy. We discuss the opportunities that new technologies will bring about and the role that Singapore can play in building a sustainable city.
To Switch or Not to Switch: Individual Differences in Executive Function and Emotion Regulation Flexibility
Emotion regulation (ER) constitutes strategies that modulate the experience and expression of emotions. While past work has predominantly focused on each ER strategy independently, recent research has begun to examine individual-difference factors that are associated with the flexible implementation of ER strategies in line with environmental demands (i.e., ER flexibility). Considering that ER processes generally implicate executive function (EF)—a collection of adaptive, general-purpose control processes—it is plausible that EF could be involved in ER flexibility. Using a latent-variable approach based on a comprehensive battery of EF tasks, the present study investigated how the various aspects of EF (i.e., common EF, working-memory specific, and shifting-specific factors) are related to the flexible maintenance and switching of ER strategies in response to stimuli that elicit varying levels of emotional intensity. Results indicated that better working-memory-specific ability (i.e., the ability to manipulate and update information within a mental workspace) was associated with greater ER strategy variability and higher frequency of ER strategy switching in high-, relative to low-, intensity contexts. Further, more proficient common EF (i.e., the ability to sustain relevant goals in the face of competing goals and responses) corresponded to greater propensity to maintain ER strategy for contexts with low-, but not high-, negative intensity. The outcomes of this study offer a richer understanding of the cognitive mechanisms underlying ER flexibility.
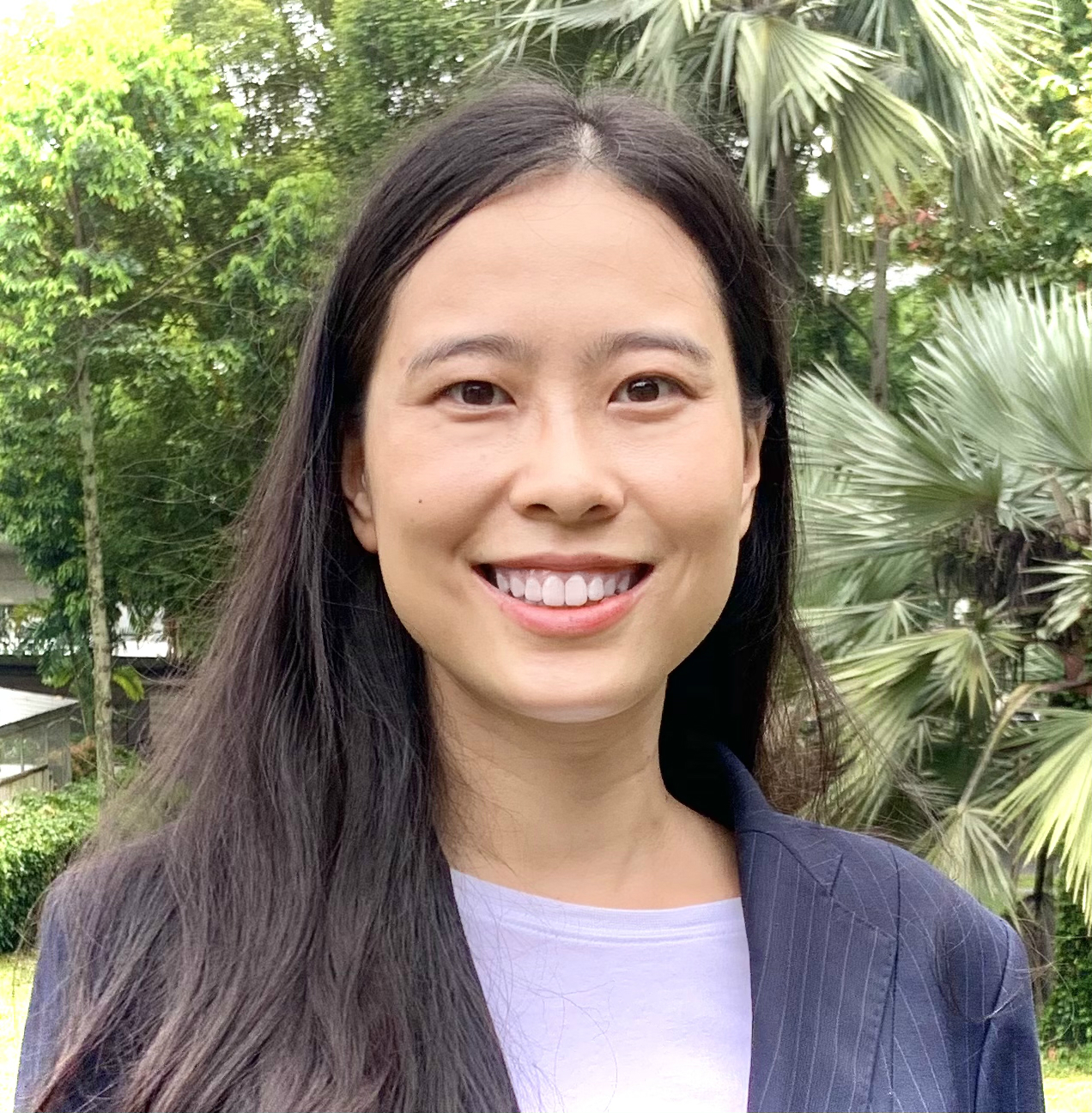
Three Essays on Empirical Asset Pricing
The dissertation consists of three essays on empirical asset pricing. The first chapter proposes a novel inter-firm link - similar employee satisfaction. Based on the employee satisfaction data on Glassdoor, the returns of similar employee satisfaction (SES) firms are documented to predict focal firm stock returns. A long-short portfolio sorted on the lagged returns of SES firms yields the Fama-French six-factor alpha of 135 bps per month. The observed predictability cannot be explained by risk-based arguments or subsumed by other known inter-firm momentums. According to the international tests, we observe stronger return predictability in countries with more flexible labor markets. The return predictability across SES firms may reflect a new type of cross-firm link derived from the knowledge spillover about employee welfare policies via social transmissions.
The second chapter discovers a novel firm characteristic that contains information about firm stock performance. Inspired by the psychological findings that demographic similarity can promote trust and coordination within a team, we propose and find that firm performance is positively related to the facial resemblance between top management team (TMT) members due to the higher managerial efficiency. A long-short value-weighted portfolio sorted on the TMT facial similarity yields a significant Fama and French (2018) six-factor alpha of 40 bps per month. In addition, the firm TMT facial similarity is also documented to be informative in firm operating performance. In addition, our tests suggest that investors’ limited attention and limits of arbitrage are the potential mechanisms behind the documented return predictability.
The last chapter studies the effects of CEO tweeting on firm stock performance by creating a measure of CEO tweeting skill. Based on the U.S. public firms sample from 2012 to 2018, we discover that if CEOs are good at communicating on social media, firms can benefit from CEOs’ high exposure on Twitter. However, if CEOs cannot handle well on social media, tweeting frequently can be harmful to the firm stock performance. We find the results hold across different countries (such as France, Germany, and the United Kingdom). The possible mechanisms behind our documented findings are shown to be limited attention and limits to arbitrage. And our documented effects are more likely to be explained by the behavioral bias other than risk explanations.
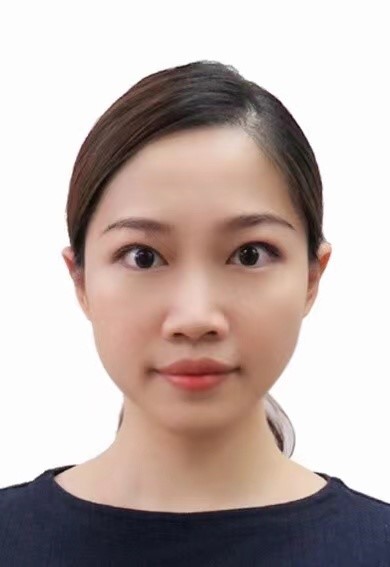
This dissertation consists of three essays that contribute to the theory of nonstationary time-series analysis.
The first chapter explores the inference procedures for predictive regressions with time-varying characteristics. We extend the self-generated instrumentation, called IVX, to incorporate persistent regressors of functional local-to-unity, functional mildly explosive, and functional mildly stationary roots. The asymptotic distributions of IVX estimators under time-varying parameters are novel and nonpivotal but lead to pivotal distributions of the corresponding Wald statistics that are robust across various roots. The numerical experiments justify the robustness of IVX testing procedures in finite samples. We also verify the existence of time-varying coefficients and the predictability of fundamentals with such unstable parameters using the S&P 500 data.
The second chapter proposes a functional local-to-unity model with autoregressive coefficients that vary smoothly over time. Two sieve estimators, namely a time series and a panel autoregression estimators, are considered to estimate the local-to-unity function. The property of consistency is established. Besides, a consistent specification test to detect parameter instability is proposed. Numerical simulations demonstrate the finite sample performance of the specification test. Finally, we apply the panel estimator and specification test to the price index of China's real estate market and obtain significant empirical results in measuring time-varying growth rates in the data.
The third chapter discusses about time-varying predictive regressions, which are useful in the applications of empirical finance. The relevant theory in this area is mainly restricted to the case in which the model contains the local-to-unity (LUR) or locally stationary regressors only. It is not universal as the prevalent evidence indicates the existence of both time-varying predictability and the mixed-root phenomenon. We investigate a nonparametric predictive regression model with mixed-root regressors and time-varying coefficients, evolving smoothly over time. Further, we present a new variant of the self-generated instrument, called Sieve-IVX, which attains robust inference irrespective of various degrees of persistence. We establish its consistency and provide a Wald test to detect the temporary predictability of economic fundamentals. Numerical simulations show satisfactory finite-sample performances, which support our results.
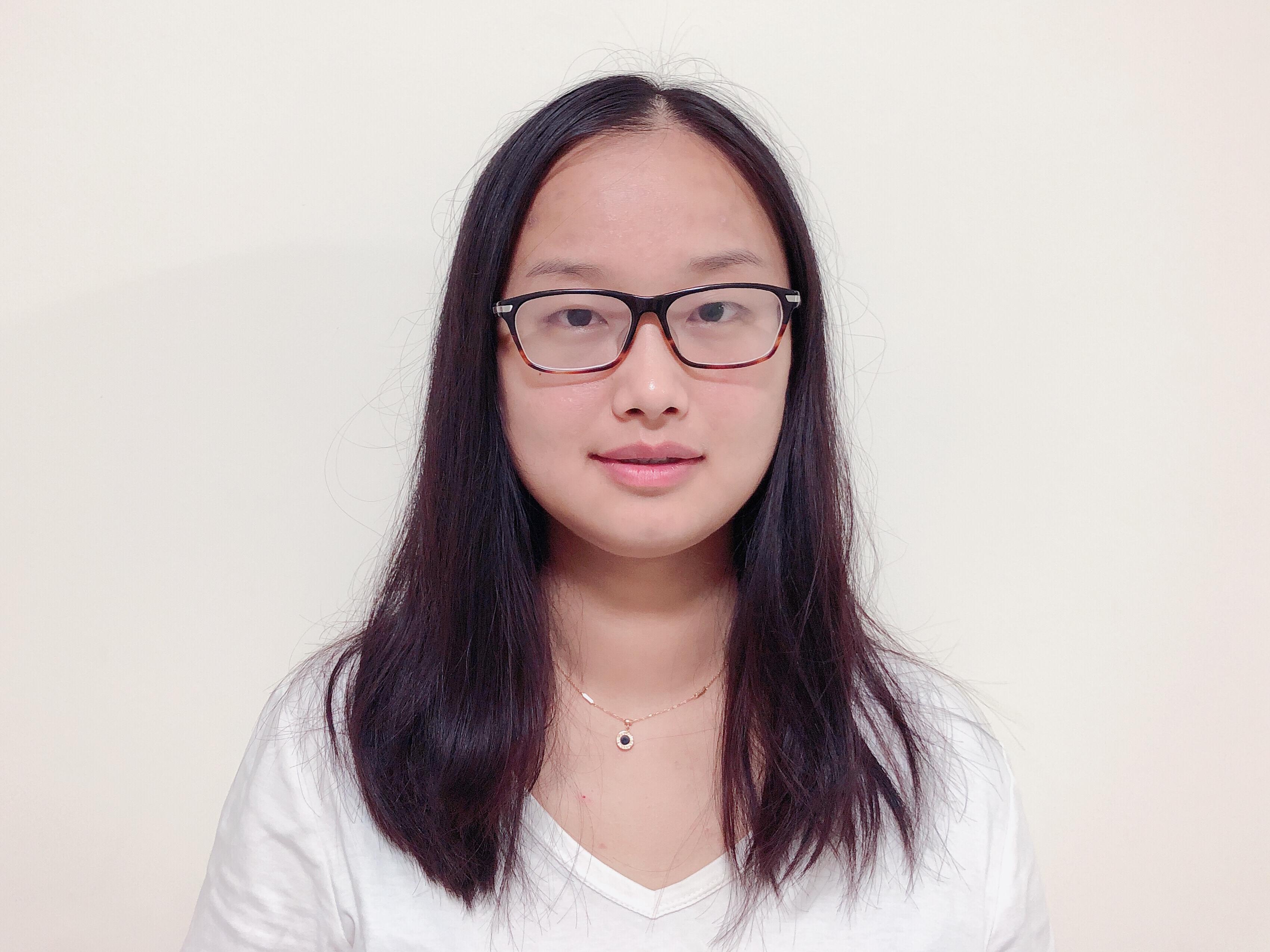
Due to increased aging populations and changes in lifestyles, we have witnessed an increased prevalence of various chronic and acute diseases and a drastic rise in healthcare expenditures in recent years. It is of paramount importance for public health to promote regular screening and close monitoring to detect the early onset of diseases. On the other hand, the increasing availability of healthcare data and advancement in data analytics offer a huge potential to facilitate this goal. We can analyze the vast amount of data and recommend more personalized diagnostic tests after receiving results and signals from screening tests and monitoring systems, which are critical decisions for the effective and efficient implementation of such screening programs and monitoring systems. Meanwhile, it is also necessary to consider human behavioral issues and their impact in making the recommendations. In particular, individual adherence to the recommended diagnostic tests can significantly affect the effectiveness and efficiency of the programs. This dissertation aims to integrate predictive analytics, optimization techniques, and behavioral models to improve risk monitoring and decision-making in patient monitoring systems and population screening programs. This dissertation first studies the real-time risk monitoring problem for patients in intensive care units (ICUs). We identify a critical lag in the provision of information due to the long lead time to measure some laboratory test variables (e.g., creatinine, platelets, and bilirubin) used in calculating the Sequential Organ Failure Assessment (SOFA) score, a well-established and important risk measure for patients in ICUs. We develop machine learning models to estimate such variables using easily measured bedside variables, the rate of changes in bedside variables, and time lag from the previous laboratory test, which mimics how physicians assess patient conditions in practice. Then the predicted laboratory test variables can be used to calculate an estimate of the real-time SOFA score. We further take advantage of the estimated standard deviations from these models to construct intervals of the real-time SOFA scores. We hypothesize that the estimated score intervals could capture the uncertainty in patient condition since the previous test and provide valuable information in a new dimension that complements the nominal SOFA scores. Using a dataset collected from an ICU in a tertiary hospital in Singapore, we calibrate our model and validate the hypothesis by comparing the prognostic accuracy of the proposed approach on patients’ 24-hour mortality and 30-day readmission with those from the SOFA score calculated using the conventional approaches. The proposed methodology could be applied to other risk measures to improve their prognostic accuracy and provide more reliable early warning for timely intervention. The methodologies developed in the previous chapter can help raise a warning of potential deterioration in a patient’s health condition, but the exact problem still has to be confirmed through follow-up diagnostic tests, which are typically more invasive and expensive. Medical resource overuse has become increasingly common in recent years and caused diverse problems, including unnecessary and risky diagnostic tests and overly intensive or expensive treatments. There is a growing call for more evidence-based decisions to reduce unnecessary diagnostic tests. The next part of the thesis dives into this problem to optimize the prescription of diagnostic tests during the health monitoring process, leveraging the improved risk monitoring tools developed in the previous chapter. In particular, we develop a finite-horizon, partially observable Markov decision process model to optimize the time to initiate a diagnostic test. Our model captures both measured and estimated clinical variables (including estimated intervals) in real-time to update the belief on a patient’s underlying health condition. We apply the model to monitor patients’ blood glucose levels to detect hyperglycemia, a common complication of critical illness. We calibrate the model using the same ICU dataset as in the previous chapter and demonstrate that the new approach can advance the detection time with fewer diagnostic tests. The methodology can also be applied to many other health monitoring systems, especially those powered by smart wearable health devices for chronic diseases. However, to optimally design the warning signals and recommend the diagnostic tests for such a monitoring system, one must consider the impact of human behavioral issues, especially individuals’ perception of the warning signals and adherence to the recommendations. We address this challenge in the next chapter in the optimal design of population screening programs for cancer surveillance and screening. Cancer remains one of the leading causes of human death, while early detection enables timely intervention and reduction in mortality rate. Two-stage screening programs are broadly implemented in practice among large average-risk populations to effectively and efficiently detect cancer in the early stages. Individuals receiving positive results in first-stage (initial) tests are recommended to undergo second-stage tests for further diagnosis. Notably, individuals’ adherence to the second-stage tests, which is closely associated with the initial test design (sensitivity and specificity) and personal characteristics, varies considerably across individuals and leads to different cancer detection rates and demands for second-stage tests. We adopt a Bayesian persuasion framework to model the optimal initial test design problem in the context of colorectal cancer screening. Our goal is to balance the trade-off between test effectiveness (i.e., detection rates of cancer incidences) and test efficiency (i.e., demands for second-stage tests), considering individuals’ adherence behavior. We conduct a nationwide survey in Singapore to calibrate the individual’s response to changes in the test design. With the embedded behavioral model, we next optimize the threshold selection in the initial test design (which decides the test sensitivity and specificity). We characterized the structural properties of an optimal initial test design. Using various data and information collected locally in Singapore and from the literature, we demonstrate that a well-designed initial test can detect more cancer incidences with fewer second-stage tests than the current practice. We further explore the benefits of using heterogeneous initial tests for different sub-populations and use the interpretable clustering technique to search for implementable rules to partition the population. We find that customized tests with simply an age-gender partition rule could bring significant extra benefits. To conclude, this thesis studies the optimal design of real-time patient monitoring systems and population screening programs, using a combination of techniques from machine learning, optimization, game theory and survey design. By analyzing the comprehensive datasets collected from various sources, we showcase that well-designed monitoring systems and screening programs can benefit individuals, healthcare service providers, and health systems through improved effectiveness and efficiency in healthcare service delivery.
Novel Techniques in Recovering, Embedding, and Enforcing Policies for Control-Flow Integrity
Control-Flow Integrity (CFI) is an attractive security property with which most injected and code-reuse attacks can be defeated, including advanced attacking techniques like Return-Oriented Programming (ROP). CFI extracts a control-flow graph (CFG) for a given program and instruments the program to respect the CFG. Specifically, checks are inserted before indirect branch instructions. Before these instructions are executed during runtime, the checks consult the CFG to ensure that the indirect branch is allowed to reach the intended target. Hence, any sort of controlflow hijacking would be prevented. There are three fundamental components in CFI enforcement. The first component is accurately recovering the policy (CFG). Usually, the more precise the policy (CFG) is, the more security CFI improves, but precise CFG generation was considered hard without the support of source code. The second one is embedding the CFI policy securely. Current CFI enforcement usually inserts checks before indirect branches to consult a read-only table which stores the valid CFG information. However, this kind of read-only table can be overwritten by some kinds of attacks (e.g., Rowhammer attack and data-oriented programming). The third component is to efficiently enforce the CFI policy. In current approaches, no matter whether there are attacks, the CFI checks are always executed whenever there is an indirect control-flow transfer. Therefore, it is critical to minimize the performance impact of the CFI checks. In this dissertation, we propose novel solutions to handle these three fundamental components. We systematically study how compiler optimization would impact CFG recovery by investigating two methods that recover CFI policy based on function signature matching at the binary level and propose our novel improved mechanism to more accurately recover function signature. We also propose an enhanced deep learning approach to recover function signature by including domain-specific knowledge to the dataset. To embed CFI policy securely, we design a novel platform which encodes the policy into the machine instructions directly without relying on consulting any read-only data structure by making use of the idea of instruction-set randomisation. In it, each basic block is encrypted with a key derived from the CFG. To efficiently enforce CFI policy, we make use of a mature dynamic code optimization platform called DynamoRIO to enforce the policy so that it only requires to do the CFI check when needed.
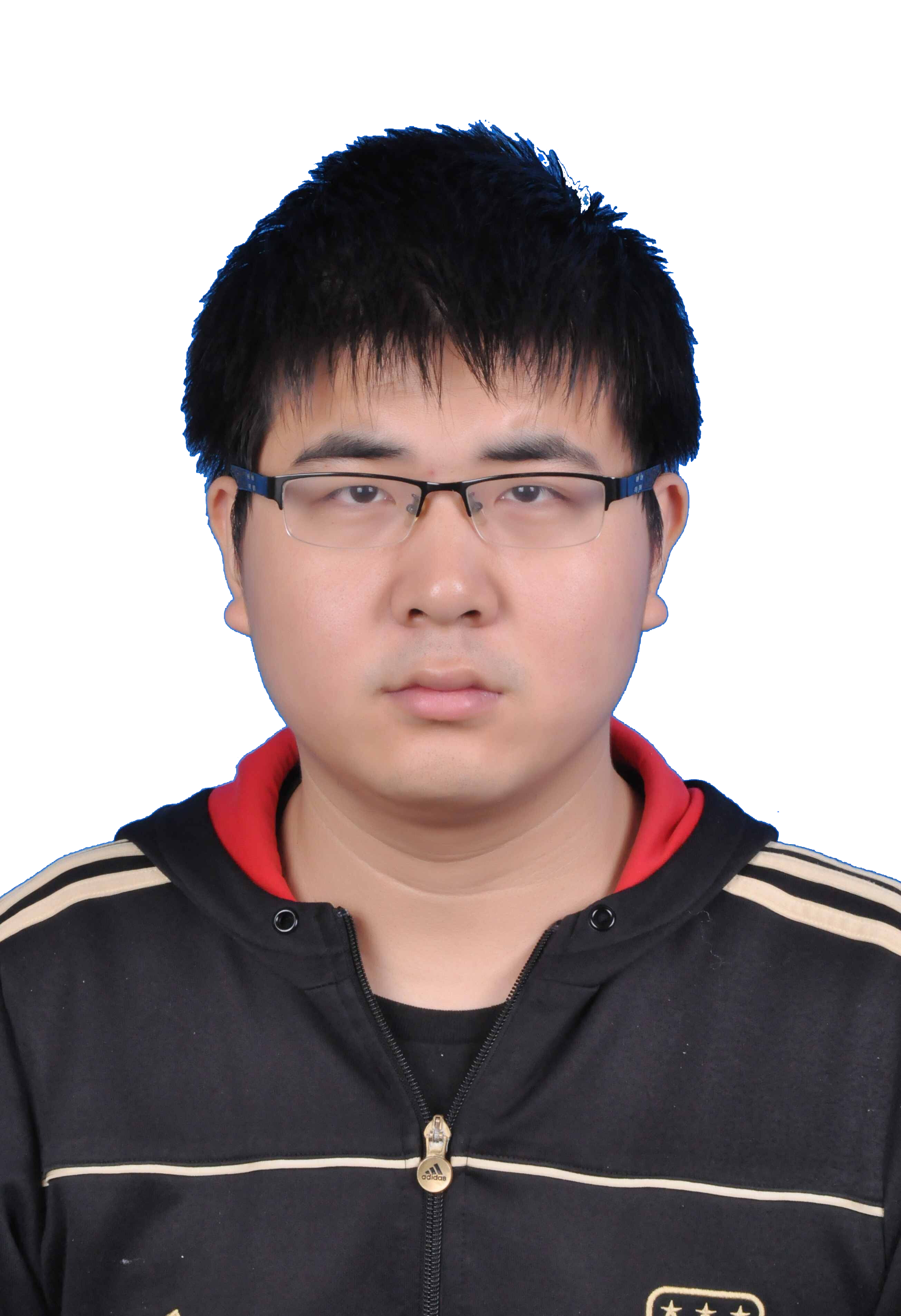
Monetary incentives, such as matching subsidies, are widely used in traditional fundraising and crowdfunding platforms to boost funding activities and improve funding outcomes. However, its effectiveness on prosocial fundraising is still unclear from both theoretical (Bénabou and Tirole, 2006; Frey, 1997; Meier, 2007a) and empirical studies (Ariely et al., 2009; Karlan and List, 2007; Rondeau and List, 2008). This dissertation aims to examine the effectiveness of matching subsidies on prosocial fundraising in the crowdfunding context. Specifically, I study how the presence of matching subsidies affects overall funding outcomes and funding dynamics in the online prosocial crowdfunding environment.
The first essay utilises a quasi-experiment on a prosocial crowdfunding platform to examine the effectiveness of matching subsidies, in which third-party institutions provide a dollar-for-dollar match of private contributions on selected campaigns, on funding outcomes, and lender behavior. Although matching subsidies offer matched loans competitive advantages over unmatched loans, we find that the total private contributions to both matched and unmatched loans increase compared to their pre-matching counterparts, suggesting a positive spillover effect on unmatched loans. However, matching subsidies lead to decreased private contributions on the platform after the matching event, showing an intertemporal displacement effect on existing loans. Furthermore, we find matching subsidies effectively attract previously inactive lenders to contribute to matched loans, leading to a motivational crowding-out effect on active lenders to unmatched loans. These findings shed new light on the overall effectiveness of matching subsidies on the online crowdfunding platforms. These findings provide policy support to offer matching subsidies on prosocial crowdfunding websites to increase overall funding.
The second essay examines how matching subsidies affect the dynamics of prosocial crowdfunding, driven by herding behaviour and payoff externalities. First, in contrast to the previous literature documenting that prior contributions may crowd out subsequent contributions in prosocial crowdfunding, we find that both herding behaviour and positive payoff externalities exist, which suggests that higher cumulative contributions lead to an increase in the subsequent funding amount. Second, we identify the existence of the bystander effect, where the positive effect of prior contributions drops sharply when the campaign is close to success. Finally, we find a substitution effect between matching subsidies and prior cumulative contributions. Matching subsidies not only increase private contributions but also moderate the herding behaviour and payoff externalities. Our findings shed new light on the effective strategies to boost fundraising on prosocial crowdfunding platforms.
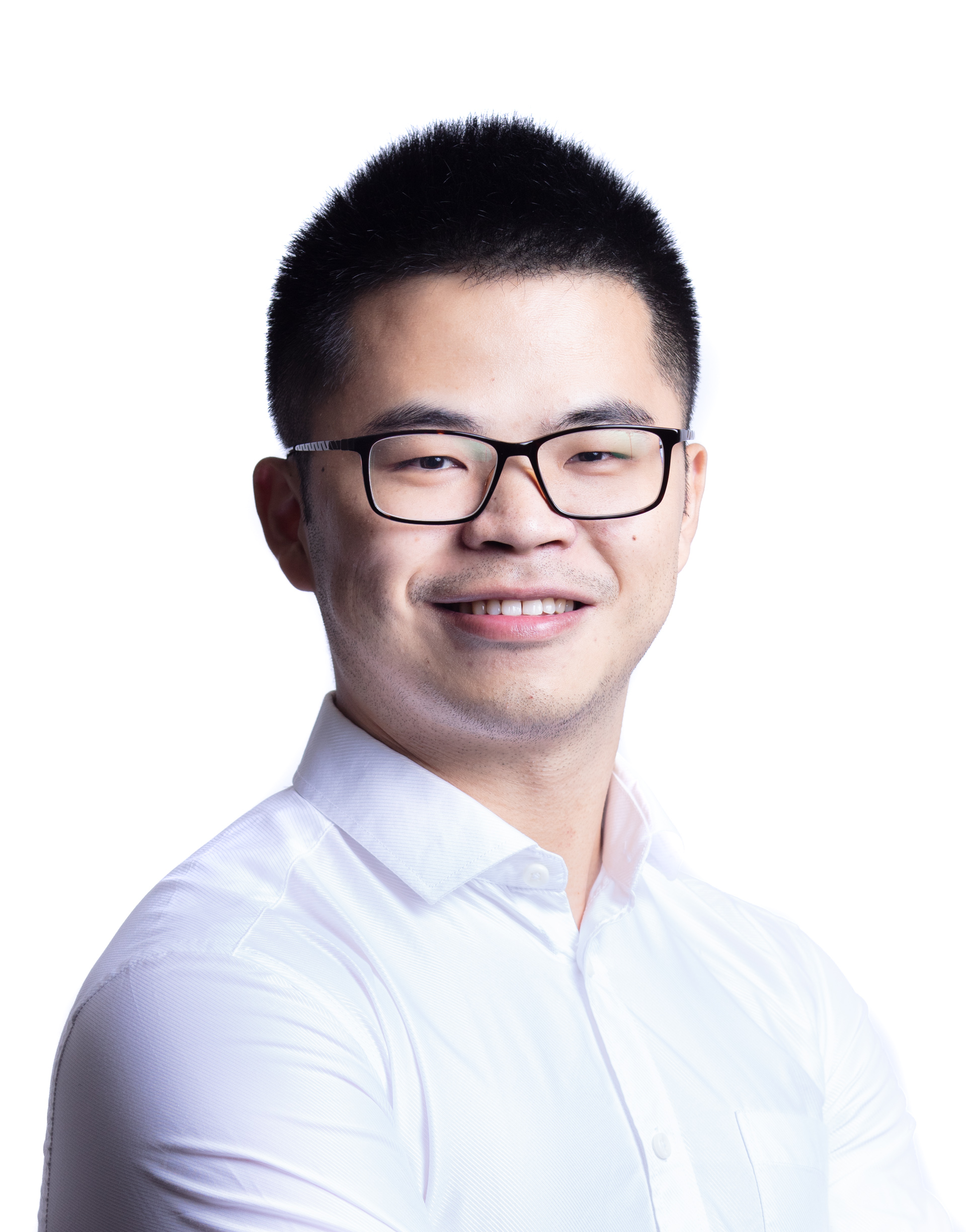
Essays on Empirical Asset Pricing
The dissertation consists of three chapters on empirical asset pricing. The first chapter examines whether the cross-sectional variation in private subsidiaries’ information disclosure predicts the cross-sectional dispersion in future equity returns of public parent firms. Information disclosure on private subsidiaries is not mandatory for public firms in the U.S., and thus these subsidiaries could be a good choice for public firms to hide bad news. We construct a private subsidiaries’ information disclosure (PSID) measure and find that a value-weighted portfolio that longs stocks in the highest PSID quintile and shorts stocks in the lowest PSID quintile yields a Fama and French (2015) five-factor alpha of 0.60% per month. This return predictability is robust controlling for various firm-specific characteristics and is stronger for stocks that receive less investor attention and stocks that are costlier to arbitrage, consistent with the hypothesis that PSID information is slowly incorporated into stock prices. The second chapter investigates whether locations of firms’ economically-important public subsidiaries contain valuable information about parent firms’ stocks returns. Stock returns of firms in the same headquarter state tend to move together (Pirinsky and Wang (2006)). Parsons, Sabbatucci, and Titman (2020) find that the return comovement of firms headquartered in the same state extends to a predictable lead-lag effect because investors are not able to fully process information arising from firms’ peers located in the same place. We reexamine whether returns of geographic peers based on the locations of both headquarters and economically relevant subsidiaries are useful for predicting the stock returns of focal firms. We find that focal firms whose geographic peers experience higher (lower) returns in the current month will earn higher (lower) returns in the next month. A strategy exploiting this pattern is distinct from other wellknown cross-firm momentum strategies, and it is more pronounced among firms that receive less investor attention and firms that are more costly to arbitrage, consistent with slow information diffusion in the geographic network into stock prices. The third chapter focuses on the well-known presidential puzzle, which refers to the striking empirical fact that stock market returns are much higher under Democratic presidencies than Republican ones. Since first noted by Huang (1985) and Hensel and Ziemba (1995) and carefully documented by Santa-Clara and Valkanov (2003), the pattern remains robust. It is only recently that Pastor and Veronesi (2020) provide an ingenious solution to this puzzle. In this paper, we document a different presidential puzzle in the cross-section of individual stocks. We construct a monthly Presidential Economic Approval Rating (PEAR) index from 1981 to 2019, by averaging ratings on president’s handling of the economy across various national polls. In the cross-section, stocks with high betas to changes in the PEAR index significantly under-perform those with low betas by 0.9% per month in the future, on a risk adjusted basis. The low-PEAR-beta premium persists up to one year, and is present in various sub-samples (based on industries, presidential cycles, transitions, and tenures) and even in other G7 countries. It is also robust to different risk adjustment models and controls for other related return predictors. Since the PEAR index is negatively correlated with measures of aggregate risk aversion, a simple risk model would predict the low PEAR-beta stocks to earn lower (not higher) expected returns. Contrary to the sentimentinduced overpricing, the premium does not come primarily from the short leg following high sentiment periods. Instead, the premium could be driven by a novel sentiment towards presidential alignment.
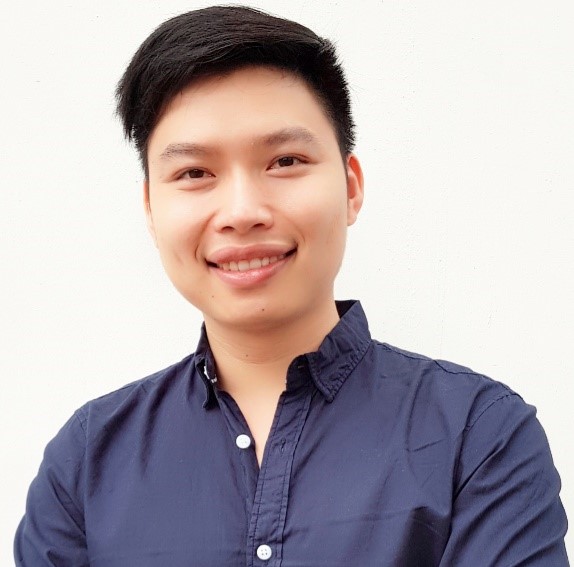
Personalized recommendation, whose objective is to generate a limited list of items (e.g., products on Amazon, movies on Netflix, or pins on Pinterest, etc.) for each user, has gained extensive attention from both researchers and practitioners in the last decade. The necessity of personalized recommendation is driven by the explosion of available options online, which makes it difficult, if not downright impossible, for each user to investigate every option. Product and service providers rely on recommendation algorithms to identify manageable number of the most likely or preferred options to be presented to each user. Also, due to the limited screen estate of computing devices, this manageable number maybe relatively small, yet the selection of items to be recommended is personalized to each individual users.
The basic entities of a personalized recommendation system are items and users. Personalization can be achieved through custom alternatives for delivering the right experience to the right user at the right time on the right device. Therefore, personalized recommendation can appear in many forms, depending on the characteristics of the items and the desired experience that the system wants users to have. In this thesis, we encompass two perspectives on personalized recommendation: preference learning and similarity learning. The former refers to the personalization in which the recommendation is tailored towards users' preference. The latter, on the other hand, refers to personalization approach in which recommendation is generated based on the users' personal perceptions of similarity between the items.
In the preference learning perspective, we focus on the task of retrieving recommendations efficiently and propose two techniques for this objective. For the first technique, we rely on Euclidean embedding to learn user and item latent vectors from users' ordinal preferences. Since they operate in the Euclidean space, these latent vectors natively support efficient nearest neighbor search using geometric structures such as spatial trees. For the second technique, our key idea is to desensitize the effect of vector magnitudes when modelling users' preferences over items. That effectively reduces the recommendation retrieval problem to the nearest neighbor search problem with cosine similarity, which can be solved efficiently with various indexing methods such as locality sensitive hashing, spatial trees, or inverted index. Extensive experiments on publicly available datasets show significant improvement of proposed techniques over the baselines.
In the similarity learning perspective, we are interested in the setting where there are multiple similarity perceptions in the data. Towards modelling these perceptions effectively, we propose two approaches that are natively multiperspective. One is a graph-theoretic framework that yields a similarity measure for any pair of objects for a perspective. Another is a geometric framework that learns multiple low-dimensional representation of objects, each for one perspective. Experiments in both studies show that the adoption of multiperspective approach allows us to better model the similarity between objects, as compared to classical uniperspective methods, which ignore the multiperspectivity in the data.