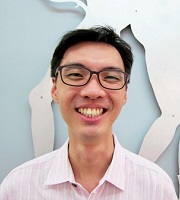
Three Essays on Corporate Finance
I focus on two areas of research for this dissertation. First, I look at incentives alignment, particularly of CEOs. Next I look at the effect of regulation on credit rating agencies. This dissertation has three essays on corporate finance. The first essay is co-authored with Gary Caton, Jeremy Goh and Scott Linn. The essay is motivated by the CEO pay slice of Bebchuk, Cremers, Peyers (2011) and explores if the negative effect of pay slice on Tobin’s Q, can be reduced or mitigated completely by the presence of friendly boards and equity incentive alignment. We find that this is the case, where connections between CEOs and board, and giving the CEO high equity portion of pay, reduces the negative effect of pay slice on Q.
The second essay is co-authored with Gary Caton and Jeremy Goh. We look at credit rating changes ability to predict operating profitability, particularly after Regulation FD and the Dodd-Frank Act. We find that this is the case, but only for specific sub groups of the rating changes.
The third essay looks at CEOs’ involvement in earnings conference calls. Relative to their pay among the top-five executives, I find that CEOs who speak more than they are paid generate abnormal returns in the conference calls they speak at, and add firm value via Tobin’s Q. They however are more susceptible to executive turnovers. Those who speak more than paid maintain their jobs
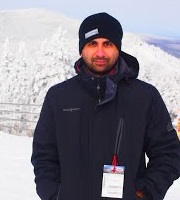
Today’s mobile phones represent a rich and powerful computing platform, given their sensing, processing and communication capabilities. These devices are also part of the everyday life of millions of people, and coupled with the unprecedented access to personal context, make them the ideal tool for conducting behavioural experiments in an unobtrusive way. Transforming the mobile device from a mere observer of human context to an enabler of behavioural experiments however, requires not only providing experimenters access to the deep, near-real time human context (e.g., location, activity, group dynamics) but also exposing a disciplined scientific experimentation service that frees them from the many experimental chores such as subject selection and mitigating biases.
This dissertation shows that it is possible to enable insitu real-time experimentation that require context-specific triggers targeting real participants on their actual mobile phones. I first developed a platform called Jarvis that allows experimenters to easily, and quickly create a diverse range of observational and treatment studies, specify a variety of opportune moments for targeting participants, and support multiple intervention (treatment) content types. Jarvis automates the process of participant selection and the creation of experimental groups, and adheres to the well known randomized controlled trial (RCT) experimental process. Of the many possibilities, a use case I envision for Jarvis, is providing retailers a platform to run lifestyle based experiments that investigate promotional strategies. Such experiments might entail the platform to provide the experimenter with the appropriate target population based on their preferences. To support this, I developed a matching and scoring algorithm that accurately factors participants’ preferences when matching experiment promotions and is capable of combining structured and unstructured promotion information into a single score. Doing so, will allow the experimentation system to target the right set of participants. Finally, I developed techniques for capturing and handling context uncertainty within Jarvis. As the opportune experiment-intervention moments are identified from sources such as sensors and social media, which have inherent uncertainties associated with them, it is crucial that such information is recorded and/or processed. More specifically, Jarvis defines a confidence metric for the location predicate as well as dynamically computes the sample size for a given experiment under context uncertainty. In doing so it provides adequate information to the experimenter to process the results of an experiment in addition to maximizing the statistical power. I validated my dissertation in the following way.
Through a series of live experiments I showcase the diversity of the system in supporting multiple experiment designs, the ease of experiment specification, and the rich behavioural information accessible to the experimenter in the form of a report. The matching and scoring algorithm was evaluated in two different ways; First, an in-depth analytical evaluation of the ranking algorithm was conducted to understand the accuracy of the algorithm. Second, I ran a user study with 43 undergraduate students to understand the effectiveness of the algorithm. Finally, I validate the context-uncertainty handling capabilities of Jarvis through simulations and show that using overlap ratios to represent location confidence is reliable and that the algorithm to estimate the number of false positives has minimal errors. Both these values are important in understanding the outcome of an experiment and in turn defining it’s success criteria.
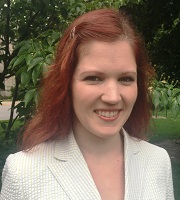
Bioecological exchange theory is proposed, which resolves contradictions between sexual strategies theory and social role theory. People are hypothesized to flexibly shift their mate preferences in response to the percentage of resources they can provide within a couple, but not limitlessly. Men are hypothesized to facultatively shift between 25-100% of provisioning and women from 0-75% of provisioning, as seen in foragers. Both sexes are then hypothesized to trade provisioning for a reciprocal amount of childcare in a partner.
Study 1 uses a sample of undergraduate Singaporean women (n = 197) to demonstrate that the more women expect to contribute to their household income, the less important social level becomes in a long-term mate. Study 2 uses an international community sample (n = 155) to show that both men and women expect to make less than their spouses when low in income, women expect to make the same as their spouses when high in income, and men expect to make more than their spouses when high in income. Women expect greater equality of provisioning and childcare the more they make, while men expect to make more than their spouses and do less childcare the more they make. Study 3 primed Singaporean undergraduates (n = 546) to feel like they would be high-earners or low earners when they graduate, and tested the effects of these conditions on preference for relative income across five levels of homemaking.
It was revealed that women want men who make more than them even when husbands are willing to do 100% of childcare when low in income, but are willing to marry men who make less than them if husbands are willing to do 50% or more of housework and childcare when high in income. Men want potential wives to make more than them when low in income unless their wives do 100% of housework and childcare, but when high in income men find women making less than them to be acceptable across all levels of homemaking, except when women are unwilling to do any. These studies provide initial support for bioecological exchange theory, and highlight the importance of considering relative income within potential couples instead of simply between intrasexual competitors, as well as the underestimated role of parental care on human mate choice.
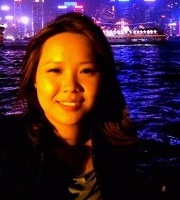
Recent research on compensatory control indicate a motivation seek out external sources of control (e.g., hierarchical structures) when subjective control is threatened. As exiting/formation of interpersonal relationships within low relational mobility environments is likely to be beyond personal choice and may threaten subjective control, three studies were conducted to investigate whether the compensatory control account could explain the negative relationship found between hierarchy endorsement and low relational mobility.
Study 1 provided initial evidence for the link; low personal-low environmental mobility individuals (vs. high personal-high environment mobility participants) were more likely to indicate higher internal control when they had higher (.vs lower) hierarchy endorsement. Study 2 and Study 3 extended Study 1 by showing (a) the different patterns of percieved internal control gain among high and low relational mobility individuals after hierarchy exposure (Study 2), and (b) how a macro-level threat (i.e., system threat) moderates the compensatory control phenomenon among high and low relational mobility individuals (Study 3).
Altogether, the studies inform us of how social ecology and individual experiences may interact to influence the individual psyche.
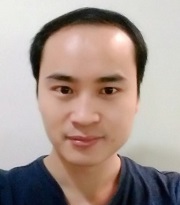
Mining User Viewpoints in Online Discussions
Online discussion forums are a type of social media which contains rich usercontributed facts, opinions, and user interactions on diverse topics. The large volume of opinionated data generated in online discussions provides an ideal testbed for user opinion mining. In particular, mining user opinions on social and political issues from online discussions is useful not only to government organizations and companies but also to social and political scientists.
In this dissertation, we propose to study the task of mining user viewpoints or stances from online discussions on social and political issues. Specifically, we will talk about our proposed approaches for these sub-tasks, namely, viewpoint discovery, micro-level and macro-level stance prediction, and user viewpoint summarization. We first study how to model user posting behaviors for viewpoint discovery. We have two models for modeling user posting behaviors. Our first model takes three important characteristics of online discussions into consideration: user consistency, topic preference, and user interactions. Our second model focuses on mining interaction features from structured debate posts, and studies how to incorporate such features for viewpoint discovery. Second, we study how to model user opinions for viewpoint discovery. To model user opinions, we leverage the advances in sentiment analysis to extract users opinions in their arguments. Nevertheless, user opinions are sparse in social media and therefore we propose to apply collaborative filtering through matrix factorization to generalize the extracted opinions. Furthermore, we study micro-level and macro-level stance prediction. We propose an integrated model that jointly models arguments, stances, and attributes. Last but not least, we seek to summarize the viewpoints by finding representative posts as one may find the amount of posts holding the same viewpoint is still large.
In summary, this dissertation discusses a number of key problems in mining user viewpoints in online discussions and proposes appropriate solutions to these problems. We also discuss other related tasks and point out some future work.
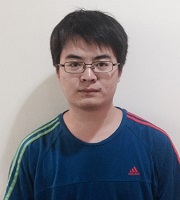
Event Identification and Analysis on Twitter
With the rapid growth of social media, Twitter has become one of the most widely adopted platforms for people to post short and instant messages. Because of such wide adoption of Twitter, events like breaking news and release of popular videos can easily capture people’s attention and spread rapidly on Twitter. Therefore, the popularity and importance of an event can be approximately gauged by the volume of tweets covering the event. Moreover, the relevant tweets also reflect the public’s opinions and reactions to events. It is therefore very important to identify and analyze the events on Twitter.
In this dissertation, we introduce our work which aims to (1) identify events from Twitter stream, (2) analyze personal topics, events and users on Twitter, and (3) summarize the events identified from Twitter. First of all, we focus on event identification on Twitter. We observe that the textual content coupled with the temporal patterns of tweets provides important insight into the general public’s attention and interests. A sudden increase of topically similar tweets usually indicates a burst of attention in some events that has happened offline (such as a product launch or a natural disaster) or online (such as the spread of a viral video). Based on these observations, we propose two models to identify events on Twitter, which are extended from LDA and a non-parametric model. These two models share two common assumptions: (1) similar tweets emerged around the same time are more likely about some events, and (2) similar tweets published by the same user over a long term are more likely about the user’s personal background and interests. These two assumptions help separate event-driven tweets from the large proportion of personal-interests-driven tweets. The first model needs to predefine the number of events because of the limitation of topic models. However, events emerge and die out fast along the time line, and the number can be countable infinite. Our non-parametric model overcomes this challenge. In the first task described above, we aim to identify events underlying the Twitter stream, and we do not consider the relation between events and users’ personal interest topics. However, the concept of events and users’ personal interest topics are orthogonal in that many events fall under certain topics. For example, concerts fall under the topic about music. Furthermore, being social media, Twitter users play important roles in forming topics and events on Twitter. Each user has her own topic interests, which influence the content of her tweets. Whether a user publishes a tweet related to an event also largely depends on whether her topic interests match the nature of the event. Modeling the interplay between topics, events and users can deepen our understanding of Twitter content and potentially aid many predication and recommendation tasks. For the second task, we aim to construct a unified model of topics, events and users on Twitter. The unified model is a combination of a topic model, a dynamic non-parametric model and matrix factorization. The topic model part is to learn users’ personal interest topics. The dynamic non-parametric model is to identify events from the tweets stream, and finally matrix factorization is to model the interaction between topics and events. Finally, we aim to summarize the events identified on Twitter. In the previous two tasks, we utilize topic models and a dynamic non-parametric models to identify events from tweets stream.
For both methods, events are learnt as clusters of tweets featured by multinomial word distributions. Therefore, users need to either read the clusters of tweets or the word distribution to interpret the events. However, the former is time-consuming and the latter cannot accurately represent the events. In this case, we propose a novel graph-based summarization method that generates concise abstractive summaries for the events. Overall, this dissertation presents our work on event identification first. Then we further analyze events, users and personal interest topics on Twitter, which can help better understand users’ tweeting behavior on events. Finally, we propose a summarization method to generate abstractive summaries for the events on Twitter.
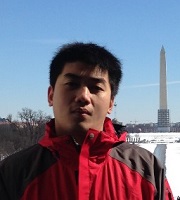
Multimodal Code Search
Today’s software is large and complex, consisting of millions of lines of code. New developers of a software project always face significant challenges in finding code related to their development or maintenance tasks (e.g., implementing features, fixing bugs and adding new features). In fact, research has shown that developers typically spend more time on locating and understanding code than modifying it. Thus, we can significantly reduce the cost of software development and maintenance by reducing the time to search and understand code relevant to a software development or maintenance task. In order to reduce the time of searching and understanding relevant code, many code search techniques are proposed. For different circumstances, the best form of inputs (i.e., queries) users can provide to search for a piece of code of interest may differ.
During development, developers usually like to search a piece of code implementing certain functionality for reuse by expressing their queries in free-form texts (i.e., natural language). After deployment, users might report bugs to an issue tracking system. For these bug reports, developers would benefit from an automated tool that can identify buggy code from the descriptions of the symptoms of the bugs. During maintenance, developers may notice that some pieces of code with a particular structure are potentially buggy. A code search technique that allows users to specify the code structure using a query language may be the best choice. In another scenario, developers may have found some buggy code examples and they would like to locate other similar code snippets containing the same problem across the entire system. In this case, a code search technique that takes as input known buggy code examples is the best choice. During testing, suppose developers have execution traces of a suite of test cases, they might want to use these execution traces as input to search the buggy code. Developers may also like to provide feedback to the code search engine to improve results. From the above examples, we could see that there is a need for multimodal code search which allows users to express their needs in multiple input forms and processes different inputs with different strategies. This will make their search more convenient and effective.
In this dissertation, we propose a multimodal code search engine, which employs novel techniques that allow developers to effectively find code elements of interest by processing developers’ inputs in various input forms including free-form texts, an SQL-like domain-specific language, code examples, execution traces, and user feedback. In the multimodal code search engine, we utilize program analysis, data mining, and machine learning techniques to improve the code search accuracy. Our evaluations show that our approaches improve over state-of-the-art approaches significantly.
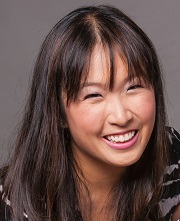
The (Un) Desirability of Happiness: Pathogen Threats Predict Differences in the Value of Happiness
People in some parts of the world find positive emotions more desirable than others. What accounts for this variability? We predicted that happiness would be valued less under conditions where the behaviors that happiness promotes would be less beneficial. We analyzed international survey data and United Nations voting records and found that happiness was valued relatively less in environments that had been historically pathogen-rich. Using a series of experimental studies, we showed that people who were experimentally primed by the threat of pathogens judged happiness in others less favorably and found happiness less appropriate. Our findings contribute to research on the function of positive emotions by providing insight into the boundary conditions under which happiness is deemed desirable.
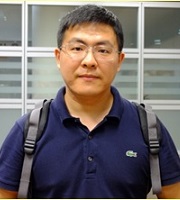
Two Essays on Corporate Finance
This dissertation investigates the impact of political factors on firm corporate policies. In the first essay, I investigate whether political uncertainty affects firm innovation, using United States gubernatorial elections as a source of plausibly exogenous variation in uncertainty. I find that firm innovation productivity, captured by patent counts and citations, declines 3.8% and 5.5% respectively in the year leading up to an election and quickly reverses afterward. This finding is robust to various specifications and endogeneity concerns. Incumbent Republican regime is negatively associated with innovation, and the negative effect of political uncertainty on innovation only exists in elections where the incumbent governor is a Republican. Finally, I find that the uncertainty effect is more pronounced in elections with high levels of uncertainty, in politically sensitive and non-regulated industries, and in firms subject to less binding financing constraints.
The second essay (jointly with Jerry Cao, Brandon Julio and Sili Zhou) examines the impact of political influence and ownership on corporate investment by exploiting the unique way provincial leaders are selected and promoted in China. The tournament-style promotion system creates incentives for new provincial governors to exert their influence over capital allocation, particularly during the early years of their term. Using a neighboring-province difference-in-differences estimation approach, we find that there is a divergence in investment rates between state owned enterprises (SOEs) and non-state owned enterprises (non-SOEs) following political turnover. SOEs experience an abnormal increase in investment by 6.0% in the year following the turnover, consistent with the incentives of a new governor to stimulate investment. In contrast, investment rates for non-SOEs decline significantly postturnover, suggesting that the political influence exerted over SOEs crowds out private investment.
The effects of political turnover on investment are mainly driven by normal turnovers, and turnovers with less-educated or local-born successors. Finally, we provide evidence that the political incentives around the turnover of provincial governors represent a misallocation of capital as measures of investment efficiency decline post-turnover.
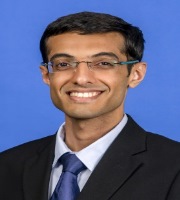
Cyclical Public Policy and Financial Factors
The Great Recession of 2009 motivated a growing body of research on the quantitative modeling of financial factors and appropriate policy responses. This dissertation is a part of that line of research and looks at the quantitative macroeconomic effects of financial factors on business cycles. The dissertation uses quantitative macroeconomic general equilibrium models (popular dynamic stochastic general equilibrium (DSGE)) that allow flexibility in micro-founded modeling of macroeconomic environments. The dissertation captures financial factors through explicit modeling of financial intermediation, featuring costly state verification and collateral constraints as financial frictions.
The first chapter offers a new quantitative model of credit cycles with endogenous leverage for financial intermediaries. Credit cycle dynamics emerge in a model with endogenous financial intermediary leverage and costly state verification. A trade-off between costly bank capital and a benefit of capital as a buffer against adverse shocks drives intermediary leverage. Bank capital functions as a buffer by reducing value-at-risk. Bank capital is costly as households require a premium to hold risky capital whereas deposits are insured. Changes in intermediary balance sheet size drive credit supply. The model displays three active credit channels: the business conditions channel, the bank net worth channel, and the funding cost channel. The model delivers empirically observed procyclical credit conditions. The second chapter investigates how bank monitoring dynamics evolve over the business cycle. The model features lognormal idiosyncratic productivity shocks for firms and endogenous default thresholds with costly state verification. The model presented in this chapter features financial intermediaries who engage in risk-shifting over the business cycle by reducing monitoring activity during business cycle upturns when the chances of loan losses are lower.
Bank monitoring is costly, but it can indirectly reduce loan default probabilities by preventing firm moral hazard. As aggregate default probabilities fall over the business cycle, the marginal benefit of loan monitoring drops. In addition, intermediary monitoring is inefficiently low because firms holdup part of the benefit of monitoring. The third chapter abstracts from financial intermediation and looks at how tax policy should vary across the business cycle in the presence of financial frictions. Financial factors in the model give rise to heterogeneity among households. Optimal income tax rates are more volatile for lower income households. The paper looks at the quantitative properties of Ramsey optimal income tax rates as well as optimal public goods provision.
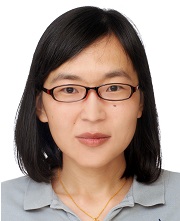
This dissertation studies the problem of preparing good-quality social network data for data analysis and mining. Modern online social networks such as Twitter, Facebook, and LinkedIn have rapidly grown in popularity. The consequent availability of a wealth of social network data provides an unprecedented opportunity for data analysis and mining researchers to determine useful and actionable information in a wide variety of fields such as social sciences, marketing, management, and security. However, raw social network data are vast, noisy, distributed, and sensitive in nature, which challenge data mining and analysis tasks in storage, efficiency, accuracy, etc.
Many mining algorithms cannot operate or generate accurate results on the vast and messy data. Thus social network data preparation deserves special attention as it processes raw data and transforms them into usable forms for data mining and analysis tasks. Data preparation consists of four main steps, namely data collection, data cleaning, data reduction, and data conversion, each of which deals with different challenges of the raw data. In this dissertation, we consider three important problems related to the data collection and data conversion steps in social network data preparation.
The first problem is the sampling issue for social network data collection. Restricted by processing power and resources, most research that analyzes user-generated content from social networks relies on samples obtained via social network APIs. But the lack of consideration for the quality and potential bias of the samples reduces the effectiveness and validity of the analysis results. To fill this gap, in the first work of the dissertation, we perform an exploratory analysis of data samples obtained from social network stream APIs to understand the representativeness of the samples to the corresponding complete data and their potential for use in various data mining tasks.
The second problem is the privacy protection issue at the data conversion step. We discover a new type of attacks in which malicious adversaries utilize the connection information of a victim (anonymous) user to some known public users in a social network to re-identify the user and compromise identity privacy. We name this type of attacks connection fingerprint (CFP) attacks. In the second work of the dissertation, we investigate the potential risk of CFP attacks on social networks and propose two efficient k-anonymity-based network conversion algorithms to protect social networks against CFP attacks and preserve the utility of converted networks.
The third problem is the utility issue in privacy preserving data conversion. Existing k-anonymization algorithms convert networks to protect privacy via modifying edges, and they preserve utility by minimizing the number of edges modified. We find this simple utility model cannot reflect real utility changes of networks with complex structure. Thus, existing k-anonymization algorithms designed based on this simple utility model cannot guarantee generating social networks with high utility. To solve this problem, in the third work of this dissertation, we propose a new utility benchmark that directly measures the change on network community structure caused by a network conversion algorithm. We also design a general k- anonymization algorithm framework based on this new utility model. Our algorithm can significantly improve the utility of generated networks compared with existing algorithms.
Our work in this dissertation emphasizes the importance of data preparation for social network analysis and mining tasks. Our study of the sampling issue in social network collection provides guidelines for people to use or not to use sampled social network content data for their research. Our work on privacy preserving social network conversion provides methods to better protect the identity privacy of social network users and maintain the utility of social network data.
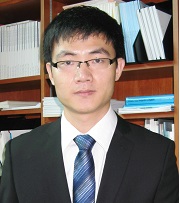
Essays on High-frequency Financial Data Analysis
This dissertation consists of three essays on high-frequency financial data analysis. I consider intraday periodicity adjustment and its effect on intraday volatility estimation, the Business Time Sampling (BTS) scheme and the estimation of market microstructure noise using NYSE tick-by-tick transaction data. Chapter 2 studies two methods of adjusting for intraday periodicity of highfrequency financial data: the well-known Duration Adjustment (DA) method and the recently proposed Time Transformation (TT) method (Wu (2012)). I examine the effects of these adjustments on the estimation of intraday volatility using the Autoregressive Conditional Duration-Integrated Conditional Variance (ACD-ICV) method of Tse and Yang (2012).
I find that daily volatility estimates are not sensitive to intraday periodicity adjustment. However, intraday volatility is found to have a weaker U-shaped volatility smile and a biased trough if intraday periodicity adjustment is not applied. In addition, adjustment taking account of trades with zero duration (multiple trades at the same time stamp) results in deeper intraday volatility smile. Chapter 3 proposes a new method to implement the Business Time Sampling (BTS) scheme for high-frequency financial data using a time-transformation function. The sampled BTS returns have approximately equal volatility given a target average sampling frequency. My Monte Carlo results show that the Tripower Realized Volatility (TRV) estimates of daily volatility using the BTS returns produce smaller root mean-squared error than estimates using returns based on the Calendar Time Sampling (CTS) and Tick Time Sampling (TTS) schemes, with and without subsampling.
Based on the BTS methodology I propose a modified ACD-ICV estimate of intraday volatility and find that this new method has superior performance over the Realized Kernel estimate and the ACD-ICV estimate based on sampling by price events. Chapter 4 proposes new methods to estimate the noise variance of high-frequency stock returns using differences of subsampling realized variance estimates at two or multiple time scales. Noise-variance estimates are compared and the new proposed estimates perform the best in reporting lower mean error and root mean-squared error. This chapter shows significant estimation error of noise-variance estimates when transactions are selected at too high or too low frequencies. For a typical New York Stock Exchange stock, the noise-to-signal ratio is around 0.005% in the period from 2010 to 2013.