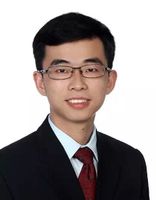
This dissertation consists of three chapters related to international trade and industrial policies.
The first chapter establishes that international trade and the market size affect institutional quality positively. Institutions, such as contract enforcements and rule of law, are arguably one of the most important determinants of economic development. I adopt an incomplete-contract approach to model institutions. Due to contract incompleteness, a firm can hold up its suppliers and distort production. When the effective market size facing firms is larger, due to trade liberalization, or increases in population or numbers of trading partners, benevolent governments have incentive to improve institutional quality to facilitate production to meet the larger demand. Interestingly, in my multiple-country framework, the competition in institutional quality also matters in a Nash-equilibrium sense. Institutional quality increases in trade-liberalized countries whereas those in the non-liberalized ones may decrease. This chapter also empirically shows the positive impact of real effective market size on institutional quality, supporting the model.
The second chapter finds that foreign direct investment (FDI) affects China’s industrial agglomeration negatively by utilizing the differential effects of FDI deregulation in 2002 in China on different industries. This result is somewhat counter-intuitive, as the conventional wisdom tends to think that FDI attracts domestic firms to cluster around them for various agglomeration benefits, technological spillovers in particular. To reconcile our empirical findings and the conventional wisdom, we develop a theory of FDI and agglomeration based on two counter-veiling force. Technology diffusion from FDI attracts domestic firms to be around them, but fiercer competition drives firms away. Our theory indicates that which force dominates depends on the scale of the economy. When the scale of the economy is sufficiently large, FDI discourages agglomeration. We find various evidence on this competition mechanism.
The third chapter studies the Chinese industrial subsidy policy from 1998 to 2007. Our industry equilibrium model establishes that the optimal policy should be positively correlated with various input distortions confronting firms. Based on this prediction, we evaluate the effectiveness of subsidy policy in China and document four stylized facts: (1) The efficiency of subsidy policy in China has grown by around 50% over the ten years, with a notable increase at the ascendance of Hu Jingtao into presidency; (2) Subsidy policy tends to have differential efficiency effect on the sector level, with more downstream sectors experiencing higher efficiency; (3) Provinces in the ‘Western Development Program’ received more subsidies compared to their eastern counterparts; (4) Labour and materials distortions have been properly corrected in the western regions, and materials distortion can explain most of the variation of subsidies in China. Finally we quantify the effect of the policy on welfare.
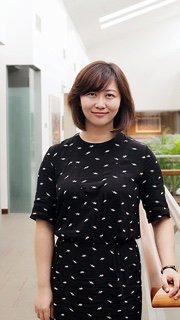
Three Essays on Social Insurance
This dissertation consists of three chapters on the economics of social insurance. Each chapter explores an aspect of the evaluation and design of social insurance in terms of nutrition, healthcare and unemployment.
The first chapter, Kim, Fleisher and Sun (2016) report evidence of long-term adverse health impacts of fetal malnutrition exposure of middle-aged survivors of the 1959-1961 China Famine using data from the China Health and Retirement Longitudinal Study. We find that fetal exposure to malnutrition has large and long lasting impacts on both physical health and cognitive abilities, including the risks of suffering a stroke, physical disabilities in speech, walking and vision, and measures of mental acuity even half a century after a tragic event. Our findings imply that policies and programs that improve the nutritional status of pregnant women yield benefits on the health of a fetus that extend through the life cycle in the form of reduced physical and mental impairment.
In Chapter 2, I evaluate the welfare benefits of the New Cooperative Medical Scheme (NCMS), the main public health insurance plan for the rural population in China. I find that the value of the NCMS to recipients is slightly higher than the government’s costs of implementation. Household benefits from the insurance through its value in transfer and insurance function. The estimated moral hazard costs are small compared to the total benefits. The findings suggest that behavioral changes due to health insurance (i.e. increase of medical service utilization) are in large welfare improving among low-income households.
In Chapter 3, I examine the effect of a two-tiered unemployment insurance system, combining both the UISA and the current unemployment insurance. Unemployment insurance savings account (UISA) is a mandatory individual savings accounts that can be used only during unemployment or retirement. Different from unemployment insurance, UISA does not lead to moral hazard problem but also provide no public insurance to workers. Workers are mandated to save when employed and can withdraw from the account when unemployed. Once the account is exhausted, the unemployed worker receives the usual unemployment benefits. The two-tiered unemployment insurance works more efficiently than an unemployment insurance system since it provides government benefits only to individuals who are not capable of consumption smoothing themselves. Fitting the model to the US economy, I find that, relative to the existing unemployment insurance system, the proposed two-tiered unemployment insurance leads to a welfare gain of 1% and reduce unemployment duration for younger workers.
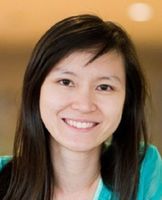
This dissertation comprises four papers that evaluate the effectiveness of public policies in Singapore. The first paper studies the non-tangible effect of a new non-contributory pension in Singapore on subjective well-being. The second investigates the effect of the same pension on labour supply, work expectations, private cash transfers and expenditure. The third evaluates a pragmatic randomised controlled trial of a new transitional care programme in a large quaternary hospital, while the fourth examines the effect of a large-scale housing upgrading programme on the resale prices of upgraded flats.
Chapter 2 looks at the non-tangible effect of a new non-contributory pension (the Silver Support Scheme, or SSS) on subjective well-being. This chapter separately estimates the announcement and disbursement effects, and finds that SSS recipients experienced greater life satisfaction at announcement, and the difference between announcement and disbursement effects is not statistically different from zero. The improvement in life satisfaction appears to be driven by social, household income, and economic satisfaction. These effects are heterogeneous, with less financially prepared individuals exhibiting larger increases in well-being.
Chapter 3 examines the effect of SSS on labour supply, work expectations, private cash transfers and expenditure, one year after its implementation. There is no evidence that receiving SSS payouts led to a fall in labour supply, work expectations or the receipt of private cash transfers – these outcome estimates are statistically insignificant and are either close to zero or positive. The expenditure estimates are positive, but unfortunately too imprecise for definitive conclusions. These results support Chapter 2’s findings that SSS improves the welfare of recipients as it has not led to substantial crowding out of private transfers or changes in labour market behaviour.
Chapter 4 evaluates a pragmatic randomised controlled trial of a new transitional care programme (TCP), CareHub, in a large quaternary hospital. CareHub merges an assortment of existing transitional care services to protocolise post-discharge patient encounters, with the goal of improving patient outcomes and containing costs. In the six months after the index hospital admission, CareHub reduced the number of unplanned cardiac-related readmissions and total unplanned cardiac-related days in hospital by 39% and 56% respectively, which translate into a decrease of 0.23 readmissions and 2.2 days of hospitalisation. Net costs were reduced by about SGD1,300 per CareHub patient. It also reduced patient anxiety/depression and improved the quality of care transition.
Chapter 5 estimates heterogeneous hedonic prices for different levels of housing space, by exploiting a unique space-adding project in Singapore that added a uniform amount of 6m2 of space to each existing housing unit regardless of the original size of the unit. This space adding was part of a large scale urban renewal and housing upgrading policy. The resale price of a housing unit increased by 7% on average, and the absolute extent of price appreciation varied significantly across the original size of the units. The total house price appreciation can be attributed to the combined effect of changes in housing space and average price per unit housing space.
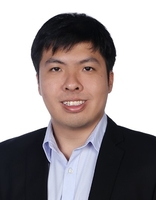
Crowdfunding is a method of raising funds to support a venture, typically by raising small amounts from a large number of investors (backers or patrons). This whole process is conducted on an online platform that facilitates interactions between project creators and potential contributors. We explore in the dissertation, the determinants of the success of crowdfunding projects. The first essay, using data from Kickstarter (the leading crowdfunding platform), explores how backers to a project are interconnected with other backers through their backing of common projects thus forming an implicit backer network. We find that backers that are in central positions within the network have an impact on other backers and, through them, affect the outcomes of projects by increasing the likelihood of project success, increasing funding and decreasing the time taken to reach the funding goal. The second essay explores the unique phenomenon of patronage. Unlike the one-time contribution that backers make in Kickstarter, patrons fund the creator and their projects in a recurring manner. We use data from a leading patronage crowdfunding platform to explore what project characteristics lead to changes in patterns of patron growth and recurring contributions in crowdfunding. We find that several project characteristics not only have an impact on the change in patron and contribution functions but also in the velocity and acceleration of these functions. Both essays uncover determinants that have not been considered thus far in their respective crowdfunding context and provide recommendations for project creators and platforms to maximize the funding generated within each specific context.
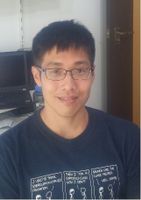
Essays in the Economics of Health and Ageing
This dissertation consists of four papers in applied microeconomics / the economics of health and ageing that analyse the causal effect of public policies, or of events / issues amenable to policy intervention. Chapter 1 provides an overview of the papers in this dissertation.
Chapter 2 investigates whether a major and growing environmental disamenity – dengue fever – leads to protective behavior that increases residential electricity consumption. Being near a dengue cluster leads to a persistent increase in electricity consumption in 4-room and 5-room/bigger flats (by 1.7% and 1.1% respectively). In addition, electricity consumption rises discontinuously when a dengue cluster’s risk classification is upgraded from yellow to red. This increased electricity consumption cost $11.9 to 16.3 million per annum (in 2015 Singapore dollars), or 7% – 12% of the overall costs of dengue in Singapore.
Chapter 3 studies the effect of in-utero exposure to mild nutritional shocks during Ramadan on an individual’s later-life outcomes. In-utero exposure to Ramadan leads to poorer subjective well-being across a broad range of domains (overall life, social and family life, daily activities, economic, and health satisfaction), self-rated health condition, and poorer mental well-being. In addition, exposed individuals report higher rates of diagnosed cardiovascular conditions and higher body mass index (among women). We find no evidence that these results are driven by selective timing of pregnancies, differing survey participation rates, or seasonal effects.
Chapter 4 examines the effect of an exogenous permanent income shock on subjective well-being. This permanent income shock is the introduction of Singapore’s first national non-contributory pension, the Silver Support Scheme. The pension improved the life satisfaction of recipients; this effect appears to be driven by social, household income, and economic satisfaction. Consistent with the predictions of the permanent income hypothesis, well-being improved at announcement, but did not improve significantly further at disbursement of the pension. Lastly, we find evidence that the marginal utility of income varies – recipients who reported being less financially prepared for retirement exhibited larger increases in well-being.
Lastly, Chapter 5 reports results from a pragmatic, randomized controlled trial of CareHub, a new transitional care program (TCP) in Singapore’s National University Hospital that aims to contain costs, reduce re-hospitalizations, and improve patient quality of life. CareHub reduced unplanned cardiac-related readmissions by 39% and unplanned cardiac-related days in hospital by 56%. In addition, we found suggestive evidence that CareHub reduced patient anxiety and depression, and improved the quality of transitional care. In all, CareHub achieved net cost savings of about S$1,300 per patient over six months, suggesting that a carefully designed TCP can reduce resource utilization while improving quality of life.
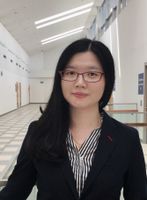
Dispersion and Uncertainty in Density Forecasts: Evidence from Surveys of Professional Forecasters
This dissertation studies patterns of dispersion in density forecasts as reported in surveys of professional forecasters. We pay special attention to the role of uncertainty in explaining dispersion in professional forecasters’ density forecasts of real output growth and inflation. We also consider the relationship between survey design and forecaster behavior. The last chapter describes future research exploring the characteristics of forecaster expectations using probability integral transforms.
As a starting point, chapter one gives a summary of the literature that tries to answer, using data from survey of forecasters, the following three key questions: Why do forecasters disagree? What do density forecasts reveal in addition to point forecasts? Does disagreement serve as a good proxy for forecaster uncertainty? This chapter provides an overview of the studies and briefly discusses how this dissertation can make contributions to the literature.
Chapter two explores the role of uncertainty in explaining dispersion in professional forecasters’ density forecasts of real output growth and inflation. We consider three separate notions of uncertainty: general macroeconomic uncertainty (the fact that macroeconomic variables are easier to forecast at some time than others), policy uncertainty, and forecaster uncertainty. The main finding is that dispersion in individual density forecasts is related to overall macroeconomic uncertainty and policy uncertainty, while forecaster uncertainty (which we define as the average in the uncertainty expressed by individual forecasters) appears to have little role in forecast dispersion.
Chapter three examines the relationship between survey design and forecasters’ behavior by exploiting changes to the probability bins provided to forecasters at the solicitation of density forecasts. We consider three important surveys, namely Survey of Professional Forecasters by the Philadelphia Fed (USSPF), Survey of Professional Forecasters by the European Central Bank (ECBSPF), and Survey of External Forecasters by Bank of England (SEF). While the adjustment of forecast bins can reasonably arise from the fluctuation of underlying macroeconomic variable, there are also cases where the modification is neutral to the economic environment. Our analysis examines how disagreement and forecaster uncertainty respond to these two different categories of survey changes. The results suggest that disagreement only responds to changes caused by real economy. Uncertainty responds to both and the effect is more persistent. These empirical facts highlight the importance of behavioral perspective when inferences are drawn from professional forecasts.
I summarize our conclusion in Chapter four, and describe future research plan exploring the features of forecaster uncertainty using probability integral transforms (“z-statistics”), a commonly-used test for density forecast optimality. We focus on the shape of the distribution of z-statistics, which is informative about the confidence level as well as bias (optimism or pessimism) of forecasters. There is evidence of significant hysteresis and that survey scheme greatly affects the performance of density forecasts.
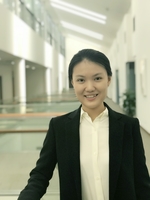
Three Essays in Corporate Finance
My dissertation aims to better understand managers and financial analysts’ behavior, incentives, and constraints, as well as their impacts on firm decisions and financial markets. In Chapter 1, I show that peer firms play an important role in determining U.S. corporate cash saving decisions. Using an instrument variable identification strategy, I find that one standard deviation change in peer firms average cash savings leads to a 2.63% same-direction change in firm’s own cash savings, which exceeds the marginal effects of many previously identified determinants. The economic implications of such peer effects are large, which can significantly alter cash savings in a representative industry by 7.2%. In cross-sectional tests, I find that peer effects are stronger when the product market is highly competitive and when the economy is in recession. In addition, less powerful, smaller, and financially constrained firms respond more actively to their peers’ cash saving decisions. Finally, I provide evidence that such peer effects are asymmetric — cash-rich firms, who already hold enough cash, are less likely to mimic peers’ cash policies compared to cash-starved firms.
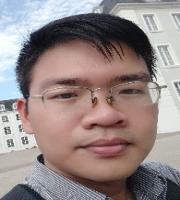
Debugging programs and writing formal specifications are essential but expensive processes to maintain quality and reliability of software systems. Developers often have to debug and create specifications manually, which take a lot of their time and effort. Recently, several automated solutions have been proposed to help developers alleviate the cost of manual labor in the two processes. In particular, fault localization techniques help developer debug by accepting textual information in bug reports or program spectra (i.e., a record of which program elements are executed for each test case).
Their output is a ranked list of program elements that are likely to be faulty. Developers then inspect the ranked list from beginning of the ranked list until root causes of the fault are found. On the other hand, many systems have no or lack of high quality formal specifications. To deal with the issue, researchers have proposed techniques to automatically infer specifications in a variety of formalism, such as nite state automation (FSA). The inferred specifications can be used for many manual software processes, including debugging.
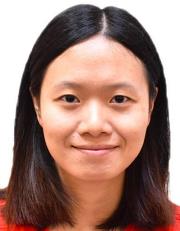
In this thesis, we are broadly interested in solving real world problems that involve decision support for coordinating agent movements in dynamic urban environments, where people are agents exhibiting different human behavior patterns and preferences. The rapid development of mobile technologies makes it easier to capture agent behavioral and preference information. Such rich agent specific information, coupled with the explosive growth of computational power, opens many opportunities that we could potentially leverage, to better guide/influence the agents in urban environments.
The purpose of this thesis is to investigate how we can effectively and efficiently guide and coordinate the agents with a personal touch, which entails optimized resource allocation and scheduling at the operational level.
More specifically, we look into the agent coordination from three specific aspects with different application domains: (a) crowd control in leisure environments by providing personalized guidance to individual agents to smooth the congestions due to the crowd; (b) mobile crowdsourcing by distributing location-based tasks to part-time crowd workers on-the-go to promote the platform efficiency; (c) workforce scheduling by better utilizing full-time workforce to provide location-based services at customers' homes.
For each, we propose models and efficient algorithms, considering agent-level preferences and problem-specific requirements. The proposed solution approaches are shown to be effective through various experiments on real-world and synthetic datasets.
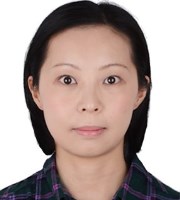
In this dissertation, I strived to understand the role of individuals’ affect in the development processes of interpersonal trust within organizations. To achieve the goal, I conducted two studies, one conceptual framework and one empirical investigation. Trust scholars have long recognized the affective component of trust experience. However, previous theoretical arguments and empirical findings are not well integrated to provide a cohesive understanding on various dynamic roles that emotions and moods can play in the trust development. Recognizing that the trustor and trustee may face diverse relational problems at various stages of their trust relationship, I first suggested a Phase Model based on well-recognized trust development models.
In the phase model, a trust development process encompasses pre-encounter, first impression, trust interaction, trust maintenance, and trust disruption/deterioration phases. I also recognized that individuals’ affect may impact trust development through multiple ways based on two perspectives on the roles of affect: The Affect Cognitive perspective and the Social Functional perspective. I delineated various mechanisms that emotions and moods can play in each phase and whether the mechanism is based on the Affect Cognitive perspective or the Social Functional perspective. In addition, I suggested that the trust development can go back from a latter phase to an earlier phase and that various affective mechanisms can phase in, out, and back in again as relationships are initiated, develop, and perhaps are even disrupted and restored.
As a result, the conceptual framework could help guide future research on affect and trust development. After delineating the conceptual paper, I conducted an empirical investigation on how newcomers develop trust in their supervisors. The literature on leader behaviors and employee trust in leader has suggested that interactional justice could promote employee trust through impacting the social exchange processes between employees and their leaders. Integrating the Social Exchange theory and findings from affect literature, I investigated how supervisor interactional justice and newcomer agreeableness impact the development of newcomer trust in supervisor through influencing newcomer anxiety level and anxiety reduction. Findings of an experience sampling study suggested that high supervisor interactional justice could lead to high levels of newcomer trust through low levels of newcomer anxiety.
Newcomer anxiety reduction (i.e., negative change over the encounter period of two weeks) could promote newcomer trust improvement (i.e., positive change over the encounter period), which in turn impacted the final levels of newcomer trust in supervisor at the end of encounter stage. In addition, supervisor interactional justice and newcomer agreeableness interacted to impact newcomer anxiety reduction. For low agreeable individuals, higher supervisor interactional justice led to more newcomer anxiety reduction in the encounter stage. Taken together, the empirical study offers insights into the process of interpersonal trust development starting from the first day at work, and uncovers the role of affective mechanisms underlying initial trust development.
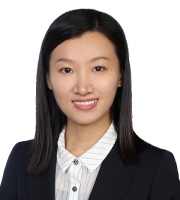
Essays in Corporate Cash Holdings
This dissertation addresses three topics in corporate cash holdings. The first paper provides a new determinant of cash holdings by examining the impact of earnings transparency on corporate cash holdings. Motivated by Barth et al. (2013), who show that firms with less earnings transparency tend to have higher cost of equity, this paper shows that the cross-section differences in earnings transparency cause variations in firm cash holdings because firms with less earnings transparency have more incentives to hold cash in order to avoid costly external financing.
Using data of US firms from 1980 to 2013, it is found that earnings transparency is significantly negatively associated with cash reserves. This impact remains significant when corporate governance measures, accounting-based earnings quality, geography diversity and other information asymmetry measures are accounted for. And this impact is more pronounced in firms with more growth opportunities, more R&D expenses and more financial constraints. It is further found that firm with lower earnings transparency have a higher value of cash holdings, suggesting that cash held by firms with lower earnings transparency are expected to be used to invest, which is also a verification that firms with less earnings transparency hold more cash for precautionary motivation.
The second paper studies on the channel of the relation between corporate cash holdings and stock return. Corporate cash holding is found to be able to predict stock return. Some scholars attribute this to the association of cash with systematic risk with respect to growth options. Others find that the relation is a mispricing effect. I try to test whether the relation between cash and return is driven by systematic risk that captured by cash. The empirical results do not support the risk explanation of cash-return relation. First, the risk loading on CASH factor cannot predict returns, which is not consistent with rational frictionless asset pricing models. Second, CASH factor cannot reflect future GDP growth. Third, CASH and its factor loading exhibit no association with implied cost of capital derived from analysts’ earnings forecasts. Additionally, I find institutional investors tend to buy in more stocks of firms with more cash, and the cash-return relation is less pronounced in firms with more institutional investors, providing evidence supporting the mispricing explanation. Overall, this study casts doubt on the argument that cash can serve as a proxy of systematic risk in the explanation of cross sectional variation in stock returns while finds evidence of the mispricing story.
The third paper studies the monitoring role of sovereign wealth funds on corporate cash holding policy and uses Temasek Holdings as the case. We find that Temasek’s presence has a negative effect on cash for companies with poor governance quality while its cash effect becomes positive for well-governed firms. Temasek’s discerning effect on cash policies highlights the effective monitoring role of sovereign funds.
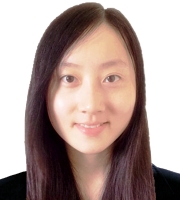
The financial services sector has seen dramatic technological innovations in the last several years associated with the “fintech revolution.” Major changes have taken place in channel management, credit card rewards marketing, cryptocurren-cy, and wealth management, and have influenced consumers’ banking behavior in different ways. As a consequence, there has been a growing demand for banks to rethink their business models and operations to adapt to changing consumer be-havior and counter the competitive pressure from other banks and non-bank play-ers. In this dissertation, I study consumer behavior related to different aspects of financial innovation by addressing research questions that are motivated by theo-ry-focused research literature and managerial considerations in business practice. I seek to understand how technology is reshaping financial services, and how finan-cial institutions can leverage big data analytics to create deep insights about con-sumer behavior for decision support.
The first essay studies credit card-based partnerships between banks and re-tailers. I test a number of hypotheses that assess the indirect effects related to the impacts of credit card programs in my research setting. I use publicly-available data, together with proprietary data from a large financial institution, to examine the impact of card-based promotions on consumer behavior and merchant perfor-mance. The results show that such promotions create positive indirect effects, leading to increased purchases from customers of other banks, in addition to the bank running the promotion. This research creates insights that banks can leverage to optimize their card-based reward programs, and paves the way forward for strengthening credit card merchant partnerships.
The second essay emphasizes the importance of investigating bank branch network changes, including branch openings and closures, and their impacts on customers’ omni-channel banking behavior. I find that branch openings create customer awareness and lead to synergetic increases in transactions across chan-nels, and branch closures result in a migration pattern from alternative channels to online banking due to the joint effects of negative perceptions and substitution. This essay contributes to our knowledge of multi-channel services in the IS and Management literature, and provides strategic implications on branch network re-structuring in omni-channel financial services.
In the third essay, I draw on social contagion theory and a spatiotemporal per-spective to explore the global penetration of bitcoin, and how security events have been influential in this process. This essay uses transaction data from Mt.Gox, one of the largest bitcoin exchange platforms in the world before its bankruptcy in 2014, and other publicly-available data sources for county-level information. The results suggest that the global penetration of bitcoin is jointly influenced by the economy, technology and regulatory situation of a country. And news about the occurrence of security incidents related to the cryptocurrency has a negative im-pact on its cross-country diffusion. This study contributes to the literature on the diffusion of emerging financial technologies, as well as business practices at the early stage of the development of a digital currency.
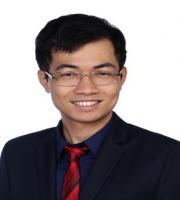
That consumers share similar tastes on some products does not guarantee their agreement on other products. Therefore, both similarity and dierence should be taken into account for a more rounded view on consumer preferences. This manuscript focuses on mining this diversity of consumer preferences from two perspectives, namely 1) between consumers and 2) between products.
Diversity of preferences between consumers is studied in the context of recommendation systems. In some preference models, measuring similarities in preferences between two consumers plays the key role. These approaches assume two consumers would share certain degree of similarity on any products, ignoring the fact that the similarity may vary across products. We take one step further by measuring different degrees of similarity between two consumers.
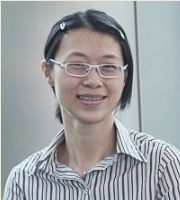
When Pursuing Multiple Goals, People Prioritize Attaining Minimum Requirements Over Aspiration Levels
When pursuing multiple goals over time, the amount of time (i.e., resources) available affects which goal is pursued: people prioritize (i.e., spend time on) the goal furthest from the aspiration level when there is plenty of time available to attain the aspiration level on the multiple goals but switch to prioritize the goal closest to the aspiration level when the time available starts to run out (e.g., Schmidt, Dolis, & Tolli, 2009). Although the aspiration level is the most commonly examined goal level, other goal levels possessing different psychological meanings (e.g., minimally acceptable or status quo goal levels) also exist.
I examined the effect of multiple goal levels (i.e., the minimally acceptable level and the aspiration level) on goal prioritization decisions. I hypothesized that when people were provided with both the minimally acceptable level and the aspiration level, they would prioritize attaining the minimally acceptable level over the aspiration level. Participants (N=316) engaged in a fully within-persons decision-making task where they repeatedly decided which of two goals to allocate their time to. The amount of time available for allocation was systematically varied.
Results indicated that people first strived for the minimally acceptable level on one goal. When they attained the minimally acceptable level on that goal, they switched to striving for the minimally acceptable level on the second goal. Only when people attained the minimally acceptable levels for both goals did they strive for the aspiration level (on one of the goals). The only exception is when they had insufficient time to attain both minimally acceptable goal levels; in that case, they focused only on one goal and strived for the aspiration level on that goal. Results imply that when choosing which goal to prioritize, people consider multiple goal levels. Implications of multiple goal levels for goal pursuit, goal revision, and theories of motivation are discussed.
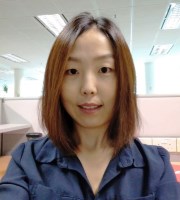
Most urban infrastructures are built to cater a planned capacity, yet surges in usage do happen in times (can be either expected or unexpected), and this has long been a major challenge for urban planner. In this thesis, I propose to study approaches handle surges in urban crowd movement. In particular, the surges in demand studied are limited to situations where a large crowd of commuters/visitors gather in a small vicinity, and I am concerned with their movements both within the vicinity and out of the vicinity (the egress from the vicinity). Significant crowd build-ups and congestions can be observed in a number of cases I studied, and when capacity expansion is not a viable strategy (either because of budget or physical constraints), smoothing these demand surges would be the only practical solution.
To handle such demand surges in urban crowds, we can either:
1. Distribute demands temporally: by slowing down the flow rate of incoming demands to the congested region through providing incentives or distractions.
2. Distribute demands spatially: by redirecting overflowing demands to other parts of network where spare capacities are still available. This might require additional investment in establishing complementary connection service.
My thesis aims at proposing computationally efficient strategies to tackle these issues. The first strategy targets on distributing demands temporally in a proactive way. In other words, this strategy is designed to prevent demand peaks from forming by slowing down crowd from congregating to areas of concern. As an example, I propose to study the strategy of crowd management in the context of theme park; in particular, the delay of flow rate towards congested areas is achieved by providing distractions (or incentives). It is demonstrated that crowd build-ups can be mitigated by utilizing this strategy. However, it might not always be possible to delay the crowd movement. For example, after major sports events that end late, most of crowd would just want to leave the stadium and reach home as soon as possible, and they will not slow down their egress pace, regardless of distractions/incentives. In these cases, I propose to study the use of the second strategy, which distributes crowds spatially to other parts of network so as to avoid clogging the vicinity that is closest to the demand node. More specifically, I propose to provide parallel services complementing existing ones so that more commuters can leave overcrowded areas and start their trips from other less crowded nodes. Consequently, there should be much fewer people queuing for services at the origin node.
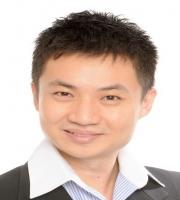
Motivated by the centrality measures constructed in Larcker, So and Wang (2013), I affirm that board connectedness positively affect firm performance in Singapore, and even if we were to measure firm performance by Tobin's Q. The impact on firm performance persists over at least four years. Controlling for Corporate Governance using a proprietary database, the Singapore Corporate Governance Index, only the Eigenvector centrality under simple-weighted and hyperbolic-weighted projections survives the robustness test, suggesting that firstly, the local proxy of Corporate Governance based on OECD principles possibly controls for what is proxied by the Betweenness, Closeness and Degree centrality measures, and secondly, there is a strong case not to ignore multiple ties when projecting interlocking boards.
The jury is hung on which weighting method is superior – the hyperbolic weighted projection has stronger results for return-onassets while the simple weighted method has stronger results for Tobin's Q. These results collectively provide additional support that some Corporate Governance indices may already impute the effects of connected boards to a certain extent. Using the methods for measuring social networks in interlocking boards as a basis, I extend the methodology to the space of ownership networks, a new endeavor since it considers the network distribution and connectedness of firm ownership, rather than focusing solely on the ultimate owners as has been the norm in the existing literature. Contrary to initial expectations, I find that simple methods, disregarding the directedness of the ownership linkages, are sufficient to yield strong results.
This paper is the first to document that ownership centrality has a direct impact on corporate performance. Controlling for Corporate Governance using the Singapore Corporate Governance Index, I find that the results for Tobin's Q are fully explained away. However, the results for return-on-assets remain mostly undiluted, with Degree and Eigenvector more significant for the unity-weighted network, and Betweenness and Closeness more significant for the stake-weighted network, making the N-score composite centrality measure a suitable compromise. Composite centrality shows significant influence on firm return-on-assets in the short to medium term.
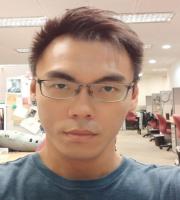
Self-esteem occupies an esteemed position in psychological research, but the self-esteem scholarship has often raised more questions than it has answered. Recent alternative approaches to self-esteem have made decent strides in resolving the mixed findings abound in the literature, such as a call for greater focus on self-esteem’s functionality and domain-specific components of self-esteem.
However, the lack of a well-grounded, parsimonious theory of self-esteem has kept these proximate theories and findings disparate and our overall understanding of self-esteem incomplete. The current dissertation sought to address these issues by developing a model of self-esteem based on the evolutionarily driven sociometer and life history theories such that important, unanswered issues concerning self-esteem research might be parsimoniously addressed, including what domains should affect self-esteem, how domains might be prioritized, and how our self-worth or value in those domains is managed. In particular, life history theory may answer these questions and also offer a way of mapping other classifications of life domains meaningfully according to two fundamental strategies, specifically mating versus somatic effort. According to the proposed model, life history determines the domains in life that a person may prioritize, and self-esteem hinges on his or her worth or value in those prioritized domains.
The current dissertation also developed and tested a measure that specifies how people will respond to either low or high value in the domains they prioritize, which can resolve questions about when people will exert effort to self-enhance or self-protect, or reduce effort and devalue the domain. Two studies served as an introductory investigation of the theoretical propositions of the current work and the findings were discussed in light of the predictions made. Overall, the current research extends our understanding of self-esteem and provided some evidence for the ideas proposed. Possible improvements to the current investigation are suggested in the discussion.
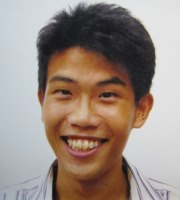
Drawing on the traditional internal-external dichotomy embraced by attribution research in other non-relational domains, research on attributions in romantic relationships has largely focused on distinguishing between the impact of making partner (internal) and external attributions. Given that past research on relationship cognitions showed that people think in relationship specific ways (e.g., relational schemas; Baldwin, 1992), I propose the need for the inclusion for attributions that capture relationship-specific causes.
With that in mind, the present research explored the incremental value of interpersonal attributions, which refer to the perception that a partner’s behaviors are caused by their love and care (or lack of) for the self and/or the relationship. To establish the importance of interpersonal attributions in relationship research, the aims of the present research are fourfold: 1) to develop a new measure of interpersonal attributions; 2) to demonstrate the unique predictive value of interpersonal attributions on relationship outcomes, beyond internal and external attributions; 3) to illuminate the process through which interpersonal attributions predict relationship satisfaction; and 4) to explore the boundary conditions of the effects of interpersonal attributions. Findings from three studies highlight the importance of moving beyond the dichotomy of internal-external attributions in relationship research. First, factor analyses of data from longitudinal (Study 1) and cross-sectional (Study 2) studies demonstrate that interpersonal attributions represent a discrete factor not captured by the internal-external distinction. Second, regression results showed that interpersonal attributions predict relationship satisfaction, over and above internal and external attributions. Taken together, these two findings provide evidence for the incremental value of interpersonal attribution.
Next, with the aim of explicating the direct effects between attributions and relationship satisfaction, Study 3 tests a moderated mediation model. Study 3 showed that the effects of interpersonal attributions on relationship satisfaction were mediated by cognitive and affective responses [Perceived Relationship Quality Component Index (PRQC index); Fletcher, Simpson, & Thomas, 2000] as well as partner perceptions [Interpersonal Qualities Scale (IQS); Murray, Holmes, & Griffin, 2000]. Furthermore, these effects were not moderated by the belief that effort can cultivate a successful relationship (i.e., growth theory; Knee, 1998). Overall, the findings suggest that the inclusion of interpersonal attributions contribute meaningfully to the discourse on the impact of divergent attribution patterns for partner’s behaviors in close relationships.
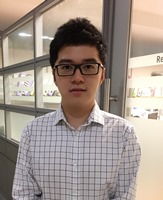
Essays on Asset Management
Hedge funds managed by listed firms significantly underperform funds managed by unlisted firms. We argue that since the new shareholders of a listed management company typically do not invest alongside the limited partners of the funds managed, the process of going public breaks the incentive alignment between ownership, control, and investment capital, thereby engendering agency problems.
In line with the agency explanation, the underperformance is more severe for funds that have low manager total deltas, low governance scores, and no manager personal capital, or that are managed by firms whose stock prices are more sensitive to earnings news. Post IPO, listed firms aggressively raise capital by launching multiple new funds. Consequently, despite the underperformance, listed firms harvest greater fee revenues than do comparable unlisted firms. Investors continue to subscribe to hedge funds managed by listed firms as they appear to offer lower operational risk.
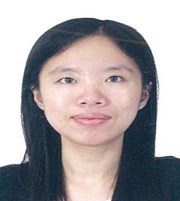
Essays on Investor Sentiment in Asset Pricing
The dissertation addresses three topics on investor sentiment in asset pricing. The first essay investigates the impact of market sentiment on the recent debate on equity premium forecasting. Particularly, market sentiment may break the link between fundamental economic predictors and equity premium. We find that economic predictors tend to lose their power and various remedies proposed in recent studies, such as non-negativity constraints, no longer work during high sentiment periods.
In contrast, economic predictors actually do have strong performances even without using any such remedies, as long as the sentiment stays low enough so as not to distort the link. Moreover, non-fundamental predictors, such as the 52-week high, work only when sentiment is high but not when sentiment is low since their performances rely on behavioural activities significant only during high sentiment periods. Finally, investors can be better-off by conducting paradigm shifts between using fundamental predictors in low sentiment periods and using non-fundamental predictors in high sentiment periods.
The second essay shows that there seems too much (more than 60%) fundamental related information in the Baker and Wurgler investor sentiment index (the BW index). Using a novel approach, we remove the fundamental related information in the BW index to obtain a purged sentiment (IS-P) index. The IS-P index outperforms the BW index in capturing the sentiment impact on cross-sectional stock returns and also beats various survey-based sentiment indices. Given that numerous studies are shadowed by the risk of producing misleading results by treating the potentially fundamental information dominated BW index as a behavioural variable, the IS-P index seems providing a safer choice for sentiment studies.
The third essay re-examines a classical topic in asset pricing: the risk and return tradeoff. Numerous studies have examined the risk and return relation, which should be theoretically positive according to Merton’s ICAPM model. However, the risk and return relation is surprisingly weak and even negative empirically. We argue that the theoretically positive risk and return relation might have been weakened or even reversed empirically by non-fundamental forces or “animal spirits”. Given that the animal spirits could be one key reason for the existing mixed and sensitive results, we measure the risk-return relation conditioning on fundamentals only. Now the impact of non-fundamental forces has been largely controlled and a positive risk and return relation can be restored.
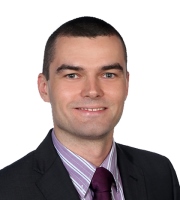
Present study compared the effect of two team efficacy (i.e., team members’ belief that the team can successfully perform a specific task) dispersion patterns in their effect on team creativity. Two dispersion patterns were manipulated such that the first one consisted of team members sharing an average level of team efficacy belief (i.e., shared team efficacy pattern), while the second dispersion pattern consisted of a majority of team members sharing a below average level of team efficacy belief and one minority member with relatively higher team efficacy belief (i.e., minority member team efficacy pattern) (DeRue, Hollenbeck, Ilgen, & Feltz, 2010).
Using motivated information processing in group model (De Dreu, Nijstad, & van Knippenberg, 2008), it was predicted that individuals who were assigned to minority members with a high team efficacy belief would engage in more discussion facilitating behaviors, which would induce higher information elaboration on the team level and consequently lead to higher team creativity. A laboratory team study (257 participants in 71 teams) was used to manipulate team efficacy patterns and measure their effect on team processes and team creativity during a brainstorming session.
The results showed that minority members expressed significantly more ideas when they perceived their efforts as indispensable for team effort. Theoretical implications of these findings for conceptualizations of team efficacy on team level and for motivated information processing in group model were discussed.
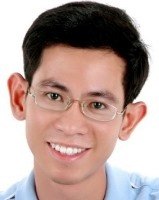
Modeling Adoption Dynamics in Social Networks
This dissertation studies the modeling of user-item adoption dynamics where an item can be an innovation, a piece of contagious information or a product. By “adoption dynamics” we refer to the process of users making decision choices to adopt items based on a variety of user and item factors. In the context of social networks, “adoption dynamics” is closely related to “item diffusion”. When a user in a social network adopts an item, she may influence her network neighbors to adopt the item. Those neighbors of her who adopt the item then continue to trigger more adoptions. As this progress unfolds over time, the item is diffused through the social network. This connection motivates us to study also item diffusion modeling.
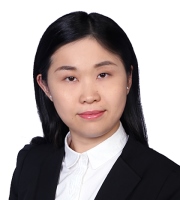
This dissertation comprises three papers that study how transportation cost affect price distribution across a city, how home equity affects the timing of pension withdrawal, and potential implications of macroprudential policies on the price informativeness. Specifically, the first paper examines how a change in the cost of car ownership affects housing price gradient with respect to distance from the central business district (CBD) in Singapore. The second paper investigates how household home equity affect the timing of claiming Social Security Retirement Income (SSRI) in the United States. The third paper explores how countercyclical policies in Singapore real estate market affect price informativeness.
Chapter 2 studies one important factor that helps to explain the price distribution of housing throughout a city. It is the acquisition cost of transportation. One key finding is obtained. When the cost of owning a car increases, the price of housing closer to the city center increases relative to housing farther away from the CBD, suggesting that increases in the price of a car cause individuals to increase their willingness to pay to locate closer to the CBD. This is consistent with the predictions from the monocentric city model that allows for two modes of transportation.
Chapter 3 examines the question that help to explain the timing when elderly individuals decide to receive SSRI benefits. The question investigates the trade-off between Social Security Retirement Income (SSRI) and home equity, two largest components among the various sources of financial assets of the elderly. Three key findings are obtained. An increase in the value of a home causes elderly individuals to delay SSRI claiming once they are eligible during the housing boom period, but we do not find a statistically significant impact on the claim decision during the bust period. Second, higher housing values have a positive effect on the likelihood of retirement in both the boom and bust period. Third, pension eligibility plays a role on the impact of home equity on retirement. Chapter 4 address one question that helps us to understand the consequences of macroprudential policies. It asks how the countercyclical policies that are designed to deter speculators by increasing transaction cost affect price informativenss in real estate market.
Two key findings are obtained. First, speculative trade decreases after dramatic increase in the transaction cost. Second, price trend along sales sequence shows significant increasing pattern. It suggests that price might not accurately reflect the true value of houses without market players who play a role in promoting informational efficiency.
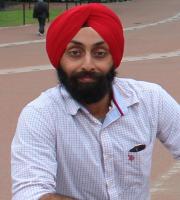
Testing and Debugging: A Reality Check
Testing and debugging are important activities during software development and maintenance. Testing is performed to check if the code contains errors whereas debugging is done to locate and fix these errors. Testing can be manual or automated and can be of different types such as unit, integration, system, stress etc. Debugging can also be manual or automated. These two activities have drawn attention of researchers in the recent years. Past studies have proposed many testing techniques such as automated test generation, test minimization, test case selection etc.
Studies related to debugging have proposed new techniques to find bugs using various fault localization schemes such as spectrum-based fault localization, IR-based fault localization, program slicing, delta debugging etc. to accurately and efficiently find bugs. However, even after years of research software continues to have bugs, which can have significant implications for the organization and economy. Often developers mention that the number of bugs they receive for the project overwhelms the resources they have. This brings forth the question of analyzing the current state of testing and debugging to understand its advantages and shortcomings. Also, many debugging techniques proposed in the past may ignore bias in data which can lead to wrong results. Furthermore, it is equally important to understand the expectations of practitioners who are currently using or will use these techniques. These analyses will help researchers understand pain points and expectations of practitioners which will help them design better techniques.
In this thesis, I take a step in this direction by conducting large-scale data analysis and by interviewing and surveying large number of practitioners. By analysing the quantitative and qualitative data, I plan to bring forward the gap between practitioners’ expectations and the research ouput. My thesis sheds light on current state-of-practice in testing in open-source projects, the tools currently used by developers and challenges faced by them during testing. For bug localization, I find that files that are already localized can have an impact on the results and this bias must be removed before running a bug localization algorithm. Furthermore, practitioners have a high expectation when it comes to adopting a new bug localization tool.
I also propose a technique to help developers find elements to test. Furthermore, through interviews and surveys, I provide suggestions for developers to create good test cases based on several characteristics such as size and complexity, coverage, maintainability, bug detection etc. In the future, I plan to perform a longitudinal study to understand the causal impact of testing on software quality. Furthermore, I plan to perform an empirical validation of good test cases based on the suggestions received from the practitioners.
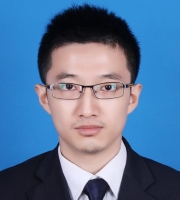
On Random Assignment Problems
This dissertation studies the standard random assignment problem (Bogomolnaia and Moulin (2001)) and investigates the scope of designing a desirable random assignment rule. Specifically, I ask the following two questions:
1. Is there a reasonably restricted domain of preferences on which there exists an strategy-sd-efficient proof, sd-efficient and sd-envy-free or equal-treatment-of-equals rule?
2. Moreover, if the answer is in the affirmative, what is that rule?
As a starting point, attention is restricted to the connected domains (Monjardet (2009)). It is shown that if a connected domain admits a desirable random assignment rule, it is structured in a specific way: a tier structure is fixed such that each tier contains at mosttwo objects and every admissible preference respects this tier structure. A domain structured in this way is called a restricted tier domain. In addition, on such a domain, the probabilistic serial (PS) rule is characterized by either sd-efficiency and sd-envy-freeness or sd-strategy-proofness, sd-efficiency, and equal-treatment-of-equals. Since these domains are too restricted, it becomes an important question whether we can find some unconnected domains on which desirable rules exist.
To facilitate such an investigation, the adjacency notion in Monjardet (2009) is weakened to block-adjacency, which refers to a flip between two adjacent blocks. Hence block-connectedness can be defined accordingly. Block-connected domains include connected domains as well as many unconnected ones. A sufficient condition called ”path-nestedness” is proposed for the equivalence between sd-strategy-proofness and the local sd-strategy-proofness on a block-connected domain, called the block-adjacent sd-strategy-proofness. Next, a class of domains, sequentially dichotomous domains, is proposed. A partition of the object set is called a direct refinement of another partition if from the latter to the former, exactly one block breaks into two and all the other blocks are inherited. Then a sequence of partitions is called a partition-path if it starts from the coarsest partition, ends at the finest partition, and along the sequence every partition is a direct refinement of its previous one. Hence a partition-path plots a way of differentiating objects by dichotomous divisions. Fix a partition-path, the corresponding sequentially dichotomous domain is the collection of preferences that respect all the partitions along the partition-path. Every such domain satisfies path-nestedness and hence the PS rule is shown to be sdstrategy- proof by verifying block-adjacent sd-strategy-proofness.
In addition, every such domain is maximal for the PS rule to be sd-strategy-proof. Hence sequentially dichotomous domains significantly expand the scope of designing a desirable rule beyond what is indicated by the restricted tier domains. The last part of this dissertation investigates realistic preference restrictions, which are modeled as follows. Each object can be evaluated according to a large set of characteristics. The planner chooses a subset of these characteristics and a ranking of them. Then she describes each object as a list according to the chosen characteristics and their ranking. Being informed of such a description, each agent’s preference that is assumed to be lexicographically separable with respect to the ranking proposed by the planner. Hence a description induces a collection of admissible preferences. It is shown that, under two technical assumptions, whenever a description induces a preference domain which admits an sd-strategy-proof, sd-efficient, and equal-treatmentof-equals rule, it is a binary tree, i.e., for each feasible combination of the top-t characteristic values, the following-up characteristic takes two feasible values. In addition, whenever a description is a binary tree, the PS rule is sd-strategy-proof on the induced preference domain. In order to show sd-strategy-proofness of the PS rule on the domain induced by a binary tree, the domain is shown to be contained by a sequentially dichotomous domain and then the result stating the sd-strategy-proofness of the PS rule on sequentially dichotomous domains is invoked.
Research on adaptive memory demonstrates that words and objects are remembered better if they are evaluated in relation to their survival or reproductive fitness value. Using the error management theory as a framework to elucidate memory biases emerging from adaptive costs and benefits, the present research examined if memory is enhanced for faces of potential mates (i.e., opposite sex individuals) in an ancestral context when the facial attractiveness and the observer’s short-term mating motive were also considered (i.e., Adaptive mating memory).
In two studies, participant read scenarios depicting survival threats, mating, or modern environment, and were told to rate a set of faces based on these scenarios. After the rating task, they were given a surprise memory test. In both studies, participants were generally more accurate for unattractive faces than attractive faces, and they tended to falsely recognized attractive opposite sex faces more frequently compared to unattractive opposite sex faces.
In addition, women falsely recognized attractive female faces more frequently than other types of faces, consistent with the female intrasexual competition hypothesis. Across both studies, women demonstrated more accurate memory for faces compared to men, and context did not influence memory for faces, regardless of attractiveness, target sex, and participant sex.
Findings from the present research suggest that adaptive memory for potential mates’ faces emerges at the interface of costs and benefits associated with facial cues (i.e., face sex, and attractiveness), and is invariant of the context the faces are situated in.
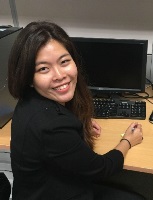
Understanding the Depleting and Replenishing Effects of Compassion on Resources and Stress Recovery
Existing literature on compassion in the workplace examines the antecedents of compassion and compassionate organizing with the underlying assumption that compassion for the suffering of others is a positive emotion and has desirable outcomes. I challenge this assumption by conceptualizing compassion as an ambivalent emotion and exploring the effects of compassion on individuals who feel compassion.
Using an experience-sampling methodology, outcomes such as helping behaviour, regulatory resources, personal resources, and stress response are examined. Feeling compassion for others can be distressing and requires emotional regulation. At the same time, feeling compassion can also motivate behaviours to alleviate suffering. Thus feeling compassion may initially be depleting yet be paradoxically later experienced as replenishing through the increased personal resources associated with helping others. However, whether there are constraints to helping also matters as feelings of compassion are not always acted on. Feelings of compassion should not translate into helping behaviour and should not lead to increased personal resources if constraints to helping are high. Further, conceptualizing compassion as an ambivalent emotion encompassing both pleasant and unpleasant aspects suggests that the emotion has some positive effects such as resilience and improved stress recovery.
Using an experience sampling method, or daily diary method, the effects of compassion on the aforementioned outcomes were examined on a sample of 80 university undergraduates over nine days. The results of the study suggest that compassion feelings and compassion behaviours have different effects on outcomes. The results of the study also suggest that feeling compassion for others has no significant effects on depletion whereas behaving compassionately is replenishes as it significantly increases personal resources. The results of the study also suggest that constraints to compassion behaviour can reduce the replenishing effects on personal resources. The results of the study also find that compassion increases mixed emotion which in is related to improved stress recovery. The study contributes in providing results that distinguish between compassion feelings and compassion behaviour, as well as being the first to examine within-person fluctuations of compassion feelings and behaviour. The study has implications on organizational citizenship behaviours as well as for organizations interested in building compassion cultures.
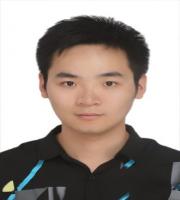
Essays on Corporate Finance
Economic, Policy uncertainty under political opaqueness imposes great impact in the capital market. I construct ex ante cross-section of firm sensitivity to China Economic Policy Uncertainty (CEPU) index from Baker, Bloom and Davis (2013). This measure of policy sensitivity is significantly negatively predictive of a firm’s market value and Tobin’s Q. Cross sectional tests show that the negative effects are stronger in SOEs= for firms with higher agency problems, and for firms operating in market with lower degree of competition or market disciplining. The evidence suggests that high level of policy influence causes significant value destruction in the capital market.
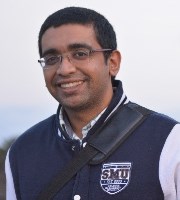
With the prevalence of sensors in public infrastructure as well as in personal devices, exploitation of data from these sensors to monitor and profile basic activities (e.g., locomotive states such as walking, and gestural actions such as smoking) has gained popularity. Basic activities identified by these sensors will drive the next generation of lifestyle monitoring applications and services. To provide more advanced and personalized services, these next-generation systems will need to capture and understand increasingly finer-grained details of various common daily life activities.In this dissertation, I demonstrate the possibility of building systems using off the- shelf devices, that not only identify activities, but also provide fine-grained details about an individual’s lifestyle, using a combination of multiple sensing modes.
These systems utilise sensor data from personal as well as infrastructure devices to unobtrusively monitor the daily life activities. In this dissertation, I have used eating and shopping as two examples of daily life activities and have shown the possibility to monitor fine-grained details of these activities. Additionally, I have explored the possibility of utilising the sensor data to identify the cognitive state of an individual performing a daily life activity. I first investigate the possibility of using multiple sensor classes on wearable devices to capture novel context about common gesture-driven activities. More specifically, I describe Annapurna, a system which utilises the inertial and image sensors in a single device to identify fine-grained details of the eating activity. Annapurna utilises data from the inertial sensors of a smartwatch efficiently to determine when a person is eating. The inertial sensors opportunistically trigger the smartwatch’s camera to capture images of the food consumed, which is used in building a food journal. Annapurna has been subjected to multiple user studies and we found that the system can capture finer details about the eating activity – images of the food consumed, with false-positive & false-negative rates of 6.5% & 3.3% respectively.
I next investigate the potential of combining sensing data from not just multiple personal devices, but also by using inexpensive ambient sensors/IoT platforms. More specifically, I describe I4S, a system utilises multiple sensor classes in multiple devices to identify fine-grained in-store activities of an individual shopper. The goal of I4S is to identify all the items that a customer in a retail store interacts with. I4S utilises the inertial sensor data from the smartwatch to identify the picking gesture as well as the shelf from where an item is picked. It utilises the BLE scan information from the customer’s smartphone to identify the rack from where the item is picked. By analysing the data collected through a user study involving 31 users, we found that we could identify pick gestures with a precision of over 92%, the rack where the pick occurred with an accuracy of over 86% and identify the position within a 1 meter wide rack with an accuracy of over 92%.
Finally, I explore the possibility of using such finer-grained capture of an individual’s physical activities to infer higher-level, cognitive characteristics associated with such daily life activities. As an exemplar, I describe CROSDAC, a technique to identify the cognitive state and behavior of an individual during the shopping activity. To determine the shopper’s behavior, CROSDAC analyses the shopper’s trajectory in a store as well as the physical activities performed by the shopper. Using an unsupervised approach, CROSDAC first discovers clusters (i.e., implicitly uncovering distinct shopping styles) from limited training data, and then builds a cluster-specific, but person-independent, classifier from the modest amount of training data available. Using data from two studies involving 52 users conducted in two diverse locations, we found that it is indeed possible to identify the cognitive state of the shoppers through the CROSDAC approach. Through these three systems and techniques, in this dissertation I demonstrate the possibility of utilising data from sensors embedded in one or more off-the-shelf devices to determine fine-grained insights about an individual’s lifestyle.
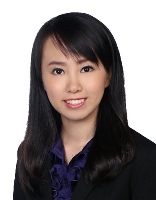
Techniques for Identifying Mobile Platform Vulnerabilities and Detecting Policy-violating Applications
Mobile systems are generally composed of three layers of software: application layer where third-party applications are installed, framework layer where Application Programming Interfaces (APIs) are exposed, and kernel layer where low-level system operations are executed. In this dissertation, we focus on security and vulnerability analysis of framework and application layers. Security mechanisms, such as Android’s sandbox and permission systems, exist in framework layer, while malware scanners protects application layer.
However, there are rooms for improvement in both mechanisms. For instance, Android’s permission system is known to be implemented in ad-hoc manner and not well-tested for vulnerabilities. Application layer also focuses mainly on malware application detection, while different types of harmful applications exist on application markets.
This dissertation aims to close these security gaps by performing vulnerability analysis on mobile frameworks and detecting policy-violating applications. As a result of our analysis, we find various framework-level vulnerabilities and we are able to launch serious proof-of-concept attacks on both iOS and Android platforms. We also propose mechanisms for detecting policy-violating applications and camouflaged applications. Our techniques are shown to improve the security of mobile systems and have several impacts on mobile industry.
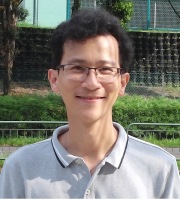
Visualization of high-dimensional data, such as text documents, is useful to map out the similarities among various data points. In the high-dimensional space, documents are commonly represented as bags of words, with dimensionality equal to the vocabulary size. Classical document visualization directly reduces this into visualizable two or three dimensions. Recent approaches consider an intermediate representation in topic space, between word space and visualization space, which preserves the semantics by topic modeling.
These approaches consider the problem of semantic visualization which attempts to jointly model visualization and topics. With semantic visualization, documents with similar topics will be displayed nearby. This dissertation focuses on building probabilistic models for semantic visualization by modeling other aspects of documents (i.e., document relationships and document representations) in addition to their texts.
The objective is to improve the quality of similarity-based document visualization while maintaining topic quality. In addition, we find applications of semantic visualization to various problems. For document collection visualization, we develop a system for navigating a text corpus interactively and topically via browsing and searching. Another application is single document visualization for visual comparison of documents using word clouds.
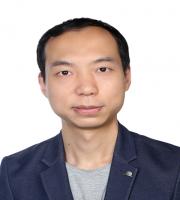
Microblogs such as Twitter have become the largest social platforms for users around the world to share anything happening around them with friends and beyond. A bursty topic in microblogs is one that triggers a surge of relevant tweets within a short period of time, which often reflects important events of mass interest. How to leverage microblogs for early detection and further impact analysis of bursty topics has, therefore, become an important research problem with immense practical value.
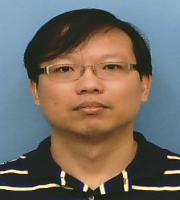
A major perspective in explaining involuntary unemployment is to recognize the existence of job market frictions, in particular, job market matching frictions. The workhorse model employed is the Diamond- Mortensen-Pissarides (DMP) model.
Similar to the labor market, the market for physical capital markets exhibits the same characteristics with a pool of unsold inventory as well as used capital that is sold and reallocated to other terms. Nevertheless, past research has highlighted several issues of the DMP model in matching the characteristics of the labor market. In a model enriched with labor participation flows and job separation, I evaluate the model performance in resolving the issues in the Krause and Lubik (2007) model in the presence of nominal price rigidity.
The model resolves the failure in generating the Beveridge curve in the presence of endogenous job destruction. Separately, in a RBC model with frictional labor and physical capital market and endogenous labor participation, I evaluate the model prediction in a context where labor disutility is procyclical under both contemporaneous shocks and news shocks.
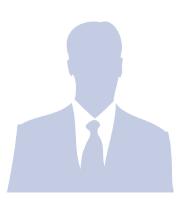
The service industry is a growing sector in most countries and emotional labor is a major component of service employees’ jobs. As such, it is important to understand how emotional labor influences employee discretionary behaviors such as counter-productive workplace behaviors (CWBs) and organizational citizenship behaviors (OCBs), both of which affect the well-being of employees and organizations.
This dissertation presents two studies that examined the mechanisms underlying, and boundary conditions surrounding, emotional labor and employee discretionary behaviors. Drawing on theories and research regarding ego depletion, inauthenticity, and behavior consistency, this paper proposed a theoretical model that hypothesized how two potential mechanisms (i.e., felt inauthenticity and emotional exhaustion) work interactively to connect emotional labor with discretionary behaviors.
Two multi-wave studies consisting of three measurement periods of 240 (Study 1) and 441 (Study 2) employees conducted on MTurk provided partial support for the hypothesized model. As hypothesized, felt inauthenticity and emotional exhaustion interacted to influence the two types of counterproductive workplace behaviors (CWBs). As such, the indirect effects between surface acting and CWBs through felt inauthenticity were moderated by emotional exhaustion. More specifically, the indirect effects were positive and stronger at low levels of emotional exhaustion but weaker at high levels of emotional exhaustion.
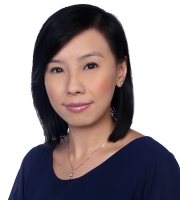
Women play an important role in business management (female businesspersons) but yet they face constraints in the workplace, such as in negotiations. As female businesspersons seem to be facing seemingly conflicting gender and business identities, the level of the integration between these identities, as captured by the construct gender-professional identity integration (G-PII), can be a critical factor that influences female businesspersons in negotiations. It is expected that the level of G-PII influences female businesspersons’ negotiation behaviors when their different identities (i.e., female identity, business identity or dual identities) are activated.
Hence, a DIAIM model that depicts how female businesspersons with different levels of G-PII may react to single versus dual identity primes behave is proposed. It is then applied to study female businesspersons in mixed-motive negotiations. A pilot study was conducted to develop an identity priming task for female businesspersons’ identity frame switching. Results of the pilot study showed that female businesspersons with high G-PII exhibited a reversed assimilation effect while low G-PIIs exhibited a reversed contrast effect.
In the main study, the propositions in the DIAIM were tested on female businesspersons’ negotiation behaviors. Results showed that identity cues moderated female businesspersons’ G-PII to affect their competition and personal negotiation outcomes, hence it provided some support to the DIAIM model. Overall, this research went beyond what past research had found on how people’s single identity activation and provided some evidence for the simultaneous activation of multiple identities.
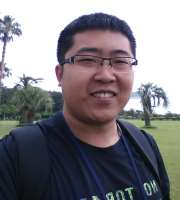
Aspect Discovery from Product Reviews
With the rapid development of online shopping sites and social media, product reviews are accumulating. These reviews contain information that is valuable to both businesses and customers. To businesses, companies can easily get a large number of feedback of their products, which is difficult to achieve by doing customer survey in the traditional way. To customers, they can know the products they are interested in better by reading reviews, which may be uneasy without online reviews. However, the accumulation has caused consuming all reviews impossible. It is necessary to develop automated techniques to efficiently process them. One of the most fundamental research problems related to product review analysis is aspect discovery. Aspects are components or attributes of a product or service. Aspect discovery is to find the relevant terms and then cluster them into aspects. As users often evaluate products based on aspects, presenting them with aspect level analysis is very necessary. Meanwhile, aspect discovery works as the basis of many downstream applications, such as aspect level opinion summarization, rating prediction, and product recommendation.
There are three basic steps to go through for aspect discovery. The first one is about defining the aspects we need. In this step, we need to understand and determine what are considered aspects. The second one is about identifying words that are used to describe aspects. This step can help us concentrate on analyzing information that is most relevant to aspect discovery. The third one is about clustering words into aspects. The main goal of this step is to cluster words that are about the same aspect into the same group. There has been much work trying to do the three basic steps in different ways. However, there still exist some limitations with them. In the first step, most existing studies assume that they can discover aspects that people use to evaluate products. However, besides aspects, there also exist another type of latent topics in product reviews, which is named “properties” by us. Properties are attributes that are intrinsic to products, which are not suitable to be used to compare different products. In the second step, to identify aspect words, many supervised learning based models have been proposed. While proven to be effective, they require large amounts of training data and turn to be much less useful when applied to data from a different domain. To finish the third step, many extensions of LDA have been proposed for clustering aspect words. Most of them only rely on the co-occurrence statistics of words without considering the semantic meanings of words.
In this dissertation, we try to propose several new models to deal with some remaining problems of existing work: 1. We propose a principled model to separate product properties from aspects and connect both of them with ratings. Our model can effectively do the separation and its output can help us understand users’ shopping behaviors and preferences better. 2. We design two Recurrent Neural Network (RNN) based models to incorporate domain independent rules into domain specific supervised learning based neural networks. Our models can improve a lot over some existing strong baselines in the task of cross-domain aspect word identification. 3. We use word embeddings to boost traditional topic modeling of product reviews. The proposed model is more effective in both discovering meaningful aspects and recommending products to users. 4. We propose a model integrating RNN with Neural Topic model (NTM) to jointly identify and cluster aspect words. Our model is able to discover clearer and more coherent aspects. It is also more effective in sentence clustering than the baselines.
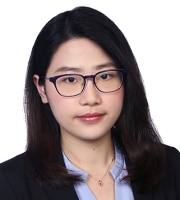
Mining Bug Repositories for Automatic Software Bug Management: From Bug Triaging to Patch Backporting
Software systems are often released with bugs due to system complexity and inadequate testing. Bug resolving process plays an important role in development and evolution of software systems because developers could collect a considerable number of bugs from users and testers daily. For instance, during September 2015, the Eclipse project received approximately 2,500 bug reports, averaging 80 new reports each day. To help developers effectively address and manage bugs, bug tracking systems such as Bugzilla and JIRA are adopted to manage the life cycle of a bug through bug report. Since most of the information related to bugs are stored in software repositories, e.g., bug tracking systems, version control repositories, mailing list archives, etc.
These repositories contain a wealth of valuable information, which could be mined to automate bug management process and thus save developers time and effort. In this thesis, I target the automation of three bug management tasks, i.e., bug prioritization, bug assignment, and stable related patch identification. Bug prioritization is important for developers to ensure that important reports are prioritized and fixed first. For automated bug prioritization, we propose an approach that recommends a priority level based on information available in bug reports by considering multiple factors, including temporal, textual, author, relatedreport, severity, and product, that potentially affect the priority level of a bug report. After being prioritized, each reported bug must be assigned to an appropriate developer/ team for handling the bug.
This bug assignment process is important, because assigning a bug report to the incorrect developer or team can increase the overall time required to fix the bug, and thus increase project maintenance cost. Moreover, this process is time consuming and non-trivial since good comprehension of bug report, source code, and team members is needed. To automate bug assignment process, we propose a unified model based on learning to rank technique. The unified model naturally combines location-based information and activity-based information extracted from historical bug reports and source code for more accurate recommendation. After developers have fixed their bugs, they will submit patches that could resolve the bugs to bug tracking systems. The submitted patches will be reviewed and verified by other developers to make sure their correctness.
In the last stage of bug management process, verified patches will be applied on the software code. In this stage, many software systems prefer to maintain multiple versions of software systems. For instance, developers of the Linux kernel release new versions, including bug fixes and new features, frequently, while maintaining some older “longterm” versions, which are stable, reliable, and secure execution environment to users. The maintaining of longterm versions raises the problem of how to identify patches that are submitted to the current version but should be backported to the longterm versions as well. To help developer find patches that should be moved to the longterm stable versions, we present two approaches that could automatically identify bug fixing patches based on the changes and commit messages recorded in code repositories. One approach is based on hand-crafted features and two machine learning techniques, i.e., LPU (Learning from Positive and Unlabeled Examples) and SVM (Support Vector Machine). The other approach is based on a convolutional neural network (CNN), which automatically learns features from patches.
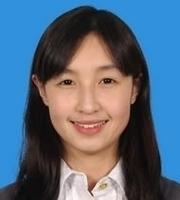
Essays in Corporate Finance
Based on a constructed index measuring the corporate governance quality of public firms, this paper focus on the role of corporate governance and its association with the performance of Singapore Exchange listed firms. We investigate the following three aspects centred on the topic of firm corporate governance in Singapore.
First, we examine the performance of the Chinese firms listed on the Singapore Exchange (S-Chips) and the role of their corporate governance. S-Chips indeed underperform local firms in terms of Tobin’s Q within both univariate and multivariable frameworks, as well as base on size matched and Propensity Score Matching samples. Higher index value indicating good governance is found to be positively related to higher Tobin’s Q for the full sample; however, when firms are separated into difference groups based on firm’s location characteristics, we find that the above positive relationship vanishes and even reverse sign when applying to firms falling into the S-Chip group. Such negative result is future supported by robustness tests that take size, firm holdings pattern and other firm characteristics into account. The results thus support our view that S-Chips are suffering from low valuation due to their “notorious reputations” caused by the scandalous actions of the managements of some former companies involved with scandals and that governance quality revealed by the S-Chips’ managements through public resources are not regarded as trust worthy by the market.
Second, we investigate the relationship between firm’s government ownership, particularly the fractions of common stocks held by Temasek Holdings, and firm value within the corporate governance framework based on a sample of Singapore Exchange listed firms. We make comparison between firm valuation and corporate governance index (SCGI) of firms that have Temasek investments (TLCs) and firms that do not (non-TLCs) and found that TLCs tend to have both higher corporate governance score and Q value and the results are consistent based on the analyses of both multivariable regression and simultaneous regressions. Temasek stock holdings are found to be related to higher firm Tobin’s Q value beyond the level of Q that is associated with good corporate governance for the full sample. Better corporate governance of TLCs are robust to matched samples based on firm size and PSM score; however, results for firm value based on matched samples indicates that it is the differences in firm characteristics such as size, leverage and profitability that drive the different firm values between TLCs and their non-TLC counterparties. No statistical significance differences are found for the positive relationship of firm value and corporate governance between TLCs and non-TLCs.
Third, we look at the impact of firm’s corporate governance practice on reducing agency costs. We choose two proxies to quantify agency costs, namely asset turnover as an inverse measure, and free cash flow as a direct measure. Controlling for growth prospects, we find a positive linear relationship between firm asset utilization (asset turnover) and governance quality, consistent with the notion that effective corporate governance can help mitigate agency problems. Furthermore, we find a nonlinear inverted U-shaped relationship between agency costs and corporate governance when using the interaction of free cash flow and firm growth opportunities as the direct agency costs proxy. Free cash flows are not kept with firms that have the best corporate governance performance when no positive NPV investment opportunities are available. Sub-indices analysis reconfirms the importance of roles of stakeholders and the responsibilities of the board of directors.
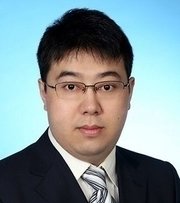
Three Essays on Random Mechanism Design
This dissertation develops several econometric techniques to address three issues in financial economics, namely, constructing a real estate price index, estimating structural break points, and estimating integrated variance in the presence of market microstructure noise and the corresponding microstructure noise function. Chapter 2 develops a new methodology for constructing a real estate price index that utilizes all transaction price information, encompassing both single-sales and repeat-sales. The method is less susceptible to specification error than standard hedonic methods and is not subject to the sample selection bias involved in indexes that rely only on repeat sales.
This dissertation focuses on RSCFs which provide every voter incentives to truthfully reveal her preference, and hence follows the formulation of strategyproofness in [26] which requires that the lottery under truthtelling (first-order) stochastically dominates the lottery under any misrepresentation according to every voter’s true preference independently of others’ behaviors. Moreover, this dissertation restricts attention to the class of unanimous RSCFs, that is, if the alternative is the best for all voters in a preference profile, it receives probability one. A typical class of unanimous and strategy-proof RSCFs is random dictatorships. A domain is a random dictatorship domain if every unanimous and strategyproof RSCF is a random dictatorship. Gibbard [26] showed that the complete domain is a random dictatorship domain. Chapter 2 studies dictatorial domains, i.e., every unanimous and strategy-proof Deterministic Social Choice Function (or DSCF) is a dictatorship, and shows that a dictatorial domain is not necessarily a random dictatorship domain. This result applies to the constrained voting model. Moreover, this chapter shows that substantial strengthenings of Linked Domains (a class of dictatorial domains introduced in [1]) are needed to restore random dictatorship and such strengthenings are“almost necessary”. Single-peaked domains are the most attractive among restricted voting domains which can admit a large class of “well-behaved” strategy-proof RSCFs.
Chapter 3 studies an inverse question: does the single-peakedness restriction naturally emerge as a consequence of the existence of a well-behaved strategy-proof randomized voting rule? This chapter proves the following result: Every path-connected domain that admits a unanimous, tops-only, strategy-proof RSCF satisfying a compromise property is single-peaked on a tree. Conversely, every single-peaked domain admits such a RSCF satisfying these properties. This result provides a justification of the salience of single-peaked preferences and evidence in favor of the Gul conjecture (see [3]). One important class of RSCFs is the class of tops-only RSCFs whose social lottery under each preference profile depends only on the peaks of preferences. The tops-only property is widely explored in DSCFs, and more importantly, is usually implied by unanimity and strategy-proofness in DSCFs (e.g., [52], [15]).
In Chapter 4, a general condition is identified on domains of preferences (the Interior Property and the Exterior Property), which ensures that every unanimous and strategy-proof RSCF has the tops-only property. Moreover, this chapter provides applications of this sufficient condition and use it to derive new results.
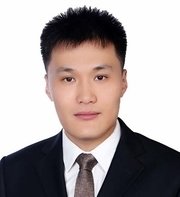
The past twenty years have been a time of many new technological developments, changing business practices, and interesting innovations in the financial information system (IS) and technology landscape. As the financial services industry has been undergoing the digital transformation, the emergence of mobile financial services has been changing the way that customers pay for goods and services purchases and interact with financial institutions. This dissertation seeks to understand the evolution of the mobile payments technology ecosystem and how firms make mobile payments investment decisions under uncertainty, as well as examines the influence of mobile banking on customer behavior and financial decision-making.
Essay 1 examines recent changes in the payment sector by extending the research on technology ecosystems and paths of influence analysis for how mobile payments technology innovations arise and evolve. Three simple building blocks, technology components, technology-based services, and the technology-supported infrastructures, provide foundations for the related digital businesses. I focus on two key elements: (1) modeling the impacts of competition and cooperation on different forms of innovations in the aforementioned building blocks; and (2) representing the role that regulatory forces play in driving or delaying innovation. I retrospectively analyze the past two decades of innovations in the mobile payments space, and identify the industry-specific patterns of innovation that have occurred, suggesting how they have been affected by competition, cooperation and regulation.
Innovations involving IT provide potentially valuable investment opportunities for industry and government organizations. Significant uncertainties are associated with decision-making for IT investment though. Essay 2 investigates a firm’s mobile payment technology investment decision-making when it faces significant technological risks and market uncertainties. I propose a new option-based stochastic valuation modeling approach for mobile payment technology investment under uncertainty. I analyze a mobile payment system infrastructure investment on the part of a start-up, and report on several sensitivity analyses and the use of least-squares Monte Carlo valuation to demonstrate some useful management findings.
Essay 3 examines the impact of the mobile channel on customer services demand across banking digital channels, and investigates how the use of the mobile channel influences customer financial decision-making. My findings suggest that the use of the mobile channel increases customer demand for digital services. The mobile phone channel serves as a complement to the PC channel, and the tablet channel substitutes for the PC channel, and the mobile phone channel and the tablet channel are complementary. In addition, my analysis indicates that customers acquire more information for financial decision-making following the use of the mobile channel. Compared to the PC-only users, mobile phone and tablet users are less likely to incur overdraft and credit card penalty fees. This study has implications for banks’ managers related to the design and management of service delivery channels.
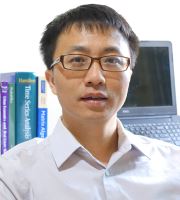
Three Essays on Financial Econometrics
This dissertation develops several econometric techniques to address three issues in financial economics, namely, constructing a real estate price index, estimating structural break points, and estimating integrated variance in the presence of market microstructure noise and the corresponding microstructure noise function. Chapter 2 develops a new methodology for constructing a real estate price index that utilizes all transaction price information, encompassing both single-sales and repeat-sales. The method is less susceptible to specification error than standard hedonic methods and is not subject to the sample selection bias involved in indexes that rely only on repeat sales.
The methodology employs a model design that uses a sale pairing process based on the individual building level, rather than the individual house level as is used in the repeat-sales method. The approach extends ideas from repeat-sales methodology in a way that accommodates much wider datasets. In an empirical analysis of the methodology, we fit the model to the private residential property market in Singapore between Q1 1995 and Q2 2014, covering several periods of major price fluctuation and changes in government macroprudential policy. The index is found to perform much better in out-of-sample prediction exercises than either the S&P/Case-Shiller index or the index based on standard hedonic methods. In a further empirical application, the recursive dating method of Phillips, Shi and Yu (2015a, 2015b) is used to detect explosive behavior in the Singapore real estate market. Explosive behavior in the new index is found to arise two quarters earlier than in the other indices.
Chapter 3, based on the Girsanov theorem, obtains the exact finite sample distribution of the maximum likelihood estimator of structural break points in a continuous time model. The exact finite sample theory suggests that, in empirically realistic situations, there is a strong finite sample bias in the estimator of structural break points. This property is shared by least squares estimator of both the absolute structural break point and the fractional structural break point in discrete time models. A simulation-based method based on the indirect estimation approach is proposed to reduce the bias both in continuous time and discrete time models. Monte Carlo studies show that the indirect estimation method achieves substantial bias reductions. However, since the binding function has a slope less than one, the variance of the indirect estimator is larger than that of the original estimator.
Chapter 4 develops a novel panel data approach to estimating integrated variance and testing microstructure noise using high frequency data. Under weak conditions on the underlying efficient price process and the nature of high frequency noise contamination, we employ nonparametric kernel methods to estimate a model that accommodates a very general formulation of the effects of microstructure noise. The methodology pools information in the data across different days, leading to a panel model form that enhances efficiency in estimation and produces a convenient approach to testing the linear noise effect that is conventional in existing procedures. Asymptotic theory is developed for the nonparametric estimates and test statistics..
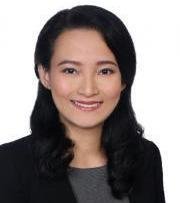
Asymptotically refined and heteroskedasticity robust inferences are considered for spatial linear and panel regression models, based on the quasi maximum likelihood (QML) or the adjusted concentrated quasi score (ACQS) approaches. Refined inferences are achieved through bias correcting the QML estimators, bias correcting the t-ratios for covariate effects, and improving tests for spatial effects; heteroskedasticity-robust inferences are achieved through adjusting the quasi score functions.
Several popular spatial linear and panel regression models are considered including the linear regression models with either spatial error dependence (SED), or spatial lag dependence (SLD), or both SED and SLD (SARAR), the linear regression models with higher-order spatial effects, SARAR(p; q), and the fixed-effects panel data models with SED or SLD or both. Asymptotic properties of the new estimators and the new inferential statistics are examined. Extensive Monte Carlo experiments are run, and the results show that the proposed methodologies work really well.
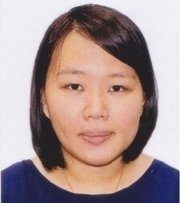
Multimedia codestreams distributed through open and insecure networks are subjected to attacks such as malicious content tampering and unauthorized accesses. This dissertation first addresses the issue of authentication as a mean to integrity - protect multimedia codestreams against malicious tampering. Two cryptographic-based authentication schemes are proposed to authenticate generic scalable video codestreams with a multi-layered structure. The first scheme combines the salient features of hash-chaining and double error correction coding to achieve loss resiliency with low communication overhead and proxy-transparency. The second scheme further improves computation cost by replacing digital signature with a hash-based message authentication code to achieve packet-level authentication and loss-resiliency.
Both schemes are robust to transcoding, i.e., they require only onetime authentication but allow verification on different transcoded versions. A comprehensive analysis is performed on the proposed schemes in comparison to existing work in terms of their authentication and verification delays, communication overhead, and buffer sizes needed for authentication/verification. Scalable video codestreams encoded by the H.264/SVC standard are made up of frames with spatial and quality layers while each frame belongs to a specific temporal layer. Taking into account the dependency structure of an H.264/SVC codestream, a secure and efficient cryptographic-based authentication scheme that is fully compatible with such a structure is proposed. By integrating the temporal scalability structure with a combination of double error correction coding and packet replication techniques, the proposed scheme is highly loss-resilient with a low communication overhead under burst loss condition. Performances of the proposed scheme under different encoding settings are further analyzed and the results showed that the proposed scheme outperforms an existing scheme in terms of its loss-resiliency. The proposed scheme also exhibits low authentication and verification delays, which is an important performance factor for real-time multimedia applications.
The third work in this dissertation studies the security of content-based authentication for non-scalable video codestreams. Based upon the video coding concept, it is shown that existing transform-domain content-based authentication schemes exhibit a common design flaw, where the transform-domain feature extracted is not sufficient to represent the true semantic meaning of the codestreams. Consequently, although the schemes are able to detect semantic-changing attacks performed in the pixel domain, they are unable to detect attacks performed in the transform domain. A comprehensive discussion on how the flaw can be exploited by manipulating transform domain parameters is presented and several attack examples are demonstrated. In addition, the concept behind attacks that manipulate the transform-domain header parameters and the conditions of the attacks, given the attacker's desired attack content, are discussed in depth.
Finally, the issue of access control as a mean to regulate unauthorized accesses to protected codestreams is studied. For generic scalable codestreams, a secure and efficient access control scheme is presented, where symmetric encryption is used to protect the codestreams, and attribute-based encryption is used to disseminate access keys to users. We further extend the scheme to address access control for H.264/SVC codestreams. The proposed schemes are secure against collusion attack and employ access keys generation hierarchy that is fully compatible to the dependency structures of generic and H.264/SVC codestreams, respectively. As a result, they are efficient in the way that each user needs to maintain only a single access key regardless of the number of layers he/she is entitled to access. The proposed schemes also eliminate the use of an online key distribution center by employing attribute-based encryption for access keys dissemination.
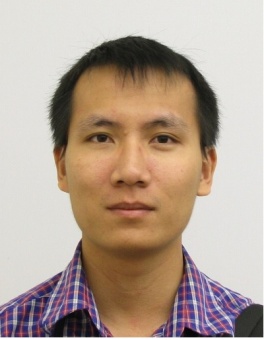
This dissertation addresses the modeling of factors concerning microblogging users' content and behavior. We focus on two sets of factors. The first set includes behavioral factors of users and content items driving content propagation in microblogging. The second set consists of latent topics and communities of users as the users are engaged in content generation and behavior adoptions. These two sets of factors are extremely important in many applications, e.g., network monitoring and recommender systems.
In the first part of this dissertation, we identify user virality, user susceptibility, and content virality as three behavioral factors that affect users' behaviors in content propagation. User virality refers to the ability of a user in getting her content propagated by many other users, while user susceptibility refers to the tendency of a user to propagate other users' content. Content virality refers to the tendency of a content item to attract propagation by users. Instead of modeling these factors independently as done in previous research, we propose to jointly model all these factors considering their inter-relationships. We develop static, temporal, and incremental models for measuring the factors based on propagation data. We also develop a static model for modeling the factors specific to topics. In the second part of this dissertation, we develop topic models for learning users' topical interest and communities from both their content and behavior.
We first propose a model to derive community affiliations of users using topics and sentiments expressed in their content as well as their behavior. We then extend the model to learn both users' personal interest and that of their communities, distinguishing the two types of interests. Our model also learns the bias of users toward their communities when generating content and adopting behavior.
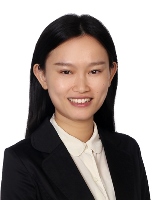
Social media has become a popular platform for millions of users to share activities and thoughts. Many applications are now tapping on social media to disseminate information (e.g., news), to promote products (e.g., advertisements), to manage customer relationship (e.g., customer feedback), and to source for investment (e.g., crowdfunding). Many of these applications require user profile knowledge to select the target social media users or to personalize messages to users. Social media user profiling is a task of constructing user profiles such as demographical labels, interests, and opinions, etc., using social media data. Among the social media user profiling research works, many focus on analyzing posted content.
These works could run into the danger of non-representative findings as users often withhold some information when posting content on social media. This behavior is called selective self-disclosure. The challenge of profiling users with selective self-disclosure behavior motivates this dissertation, which consists of three pieces of research works. The first work is that of profiling silent users in social media. Silent users (or lurkers) are the users who choose not to disclose any information. In this work, we analyze silent users’ behavior and profile silent users’ marital status, religion and political orientation by leveraging their neighbor content. The second work is that of profiling users with selective topic disclosure. Social media users may choose not to post some of their interested topics.
As a result, their posting and reading topics can be different. In this work, we analyze the difference between users’ posting and reading topics and profile users’ posting and reading topics separately even we do not know the content users read. The third work is that of profiling users with selective opinion disclosure. In social media, users may not disclose their opinions on a specific issue even when they are interested in this issue. We call these users issue-specific silent users. In this work, we investigate and profile the opinions of issue-specific silent users.
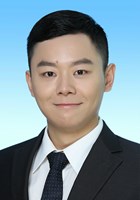
Essays about Corporate Finance
Innovation is vital to companies’ competitive advantages and is an important driver of economic growth. However, innovation is costly, since the innovation process is long, idiosyncratic, and uncertain, often involving a very high failure probability and great positive externalities .We thus launch the investigation from the following three aspects to explore how to create a better environment for producing innovation: Financing of innovation; dual-class share structure of innovation; and regulation and policy (e.g. SOX Act.)'s impact on innovation.
First of all, we study the effect of firms’ real estate collateral on innovation. In the presence of financing frictions, firms can use real estate assets as collateral to finance innovation. Through this collateral channel, positive shocks to the value of real estate collateral enhance firms’ financing capacity and lead to more innovation. Empirically, a one standard deviation increase in a firm’s real estate valuation is associated with an 8% increase in the quantity, quality, generality, and originality of its patents applied in the same year, and such positive effect is persistent over subsequent five years. The positive effect is more pronounced for firms that are financially constrained, dependent on debt finance, or belonging to hard-to-innovate industries. Our results suggest that corporate real estate collateral serves an important role in mitigating financial constraints, which leads to more innovation outputs.
Second, we try to explore how the dual-class share structure would affect the in production of innovation. Despite the risk of power abuse by corporate insiders with excessive control rights, technology companies are increasingly adopting dual-class share structures. In this paper, we show that such structures are negatively associated with corporate innovation measures. For dual-class firms, patents are increasing in Tobin’s Q, high-tech or hard-to-innovate industries, external takeover market threats or product market competition. Our findings are robust to reverse causality. To ensure that these findings are not the result of reverse causality, we examine a subsample of firms that switch from single-class.
Third, we investigate whether innovation by publicly listed U.S. companies deteriorated significantly after the adoption of the Sarbanes-Oxley Act of 2002. Using data on patent filings as proxies for firms’ innovative activities, we find firms’ innovation as measured by patents and innovation efficiency dampened significantly after the enactment of the Act. The degree of impact is related to firm specific characteristics such as firm value (Tobin’s Q) or corporate governance (G-Index) as well as firms’ operating conditions (i.e., high-tech industries, delisted or not). We find evidence that SOX’s impact on firms is more pronounced for growth firms, firms with low governance scores, firms operating in high-tech industries or firms that continued to stay listed. Overall, the results suggests that the SOX has an unintended consequence of stifling corporate innovation.
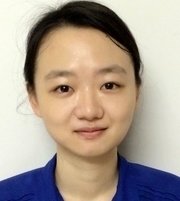
During the lock-up period, company insiders are prohibited from selling their shares for a set period immediately after initial public offerings (IPOs), usually 180 days. This strict prohibition limits the borrowing of securities by short sellers within this period. Therefore, upon reaching the lock-up expiry date, the short-sale constraint may be loosened and new investors may rush into the stock market, which affects asset price and stock return. This thesis focuses on the IPOs’ performance during the lock-up period and the reasons for the unusual performance.
The first section commences by questioning the role of the short seller and its relation to the stock return during the lock-up period. Since Regulation SHO required that short sale transaction data be made available during year 2005 to 2007, we are able to use the daily short selling transaction data to examine the trading behaviour of short sellers during the period around the lock-up expiry date. We find that transactions around the lock-up expiration are associated with a significant drop in the abnormal return. Furthermore, on the lock-up expiry day, the short selling percentage reaches the highest point, while stock return drops to the lowest level compared to the lock-up period. Hence, there is a connection between short sale and stock return on the lock-up expiry day. We then examine whether trading behaviour of short sellers around the lock-up expiration contains any information of future stock returns. The results all indicate a highly significant predictability of short seller trading activities on future stock returns. The findings lead us to develop the second section.
Since there is a dramatic drop of IPOs’ abnormal return during the lockup expiry day in the Regulation SHO period, in the second section, we investigate whether this is a universal phenomenon by using a comprehensive sample period from year 1990 to 2014. By implementing an event study with a wider event window, we discover that the abnormal returns indeed decrease significantly during most of the sample period. However, we reveal that the return decline trend ceases right after the lock-up expiration and even reverses to the highest level of return before the lock-up expiry day for several years. Therefore, we may assume that the lock-up expiration event does not have a permanent impact on stock returns.
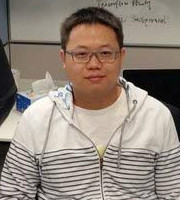
Rapid advances in mobile devices and cloud-based music services have brought about a fundamental change in the way people consume music. Cloud-based music streaming platforms like Pandora and Last.fm host an increasing huge volume of music contents. Meanwhile, the ubiquity of wireless infrastructure and advanced mobile devices enable users to access such abundant music content anytime and anywhere.
Consequently, there has been an increasing demand for the development of intelligent techniques to facilitate personalized and context-aware music retrieval and recommendation. Most of existing music retrieval systems have not considered users' music preferences, and traditional music recommender systems have not considered the influence of local contexts.
As a result, search and recommendation results may not best suit users' music preference influenced by the dynamically changed contexts, when users listen to music using mobile devices on the move. Current mobile devices are equipped with various sensors and typically for personal use. Thus, rich user information (e.g., age, gender, listening logs) and various types of contexts (e.g., time. location) can be obtained and detected with the mobile devices, which provide an opportunity to develop personalized and context-aware music retrieval and recommender systems.
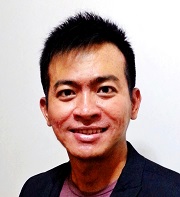
Role and Impact of Energy in the Business Cycle
Given the fundamental role of energy in the economy, the macroeconomic literature contains a large body of work on the impact of oil/energy on the business cycle, with much of the attention focusing on energy supply shocks, mostly modeled as exogenous oil/energy price increases. And yet, the oil price hikes pre-2008 suggest that other shocks to the energy market may be the source of such instance of price disturbances, so that their effects on the economy are no longer predicted by exogenous energy supply shocks.
In such scenario, it is no longer valid to treat energy price disturbances as exogenous shocks to an economic model that seeks to study the impact of energy on the business cycle.
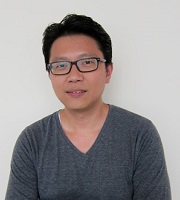
Three Essays in Corporate Finance
There are two foci in my research efforts to produce this dissertation. First, I explore and create novel datasets and methods that can expand our existing arsenal of empirical tools.1 Following that, I deploy these tools to analyze three aspects of information science in social networks and earnings-related voluntary disclosures: Social network connectedness, natural language, and management credibility. This dissertation has three essays on corporate finance.
The first essay is motivated by the friendly board framework of Adams and Ferreira (2007). In this study, we measure the value of board advisory activities using Centrality Slice (CS) - the ratio of the network connectedness of executive directors to non-executive directors. We find that this measure positively relates to firm value, performance-turnover sensitivity, management forecast accuracy, and market reaction to forecast surprises. The results from our instrumented regression suggest that CS is an optimal selection outcome that varies across firms. As such, firms will likely enjoy better advisory benefits if their policies can support high CS in an optimal manner.
The second essay is co-authored with Roger K. Loh. In this study, we add two novel approaches to a large literature on analysts’ conflicts of interests. Using analysts’ tones during peer conference calls, and returns co-movement between their brokerages and hosts to proxy for the level of information advantage, we find that analysts from high returns co-moving brokerages exhibit language patterns that neither signal competition nor collusion. Our results show that the market values tones, with increasing reactions to the level of returns co-movement, consistent with the notion of pricing for competence. We also find that the market is not naïve as it discounts sentiment tones from brokerages sanctioned during the Global Analyst Research Settlements.
The third essay is co-authored with Chiraphol N. Chiyachantana. Using a proprietary set of institutional trading data, we investigate how sophisticated investors utilize the information contained in management earnings forecasts characteristics to formulate their trading strategy. We find that these investors’ responses to a firm’s forecasts are not only increasing in the magnitude of earnings surprise, but also magnified by the firm’s prior forecast accuracy. We reveal transient institutions as the principal traders on these forecast characteristics and show that trading strategies using both forecast surprise and prior forecast accuracy are not only profitable to implement, but also outperform those that rely solely on forecast surprise.